AI-Driven Interview Analysis is transforming the landscape of academic research by enhancing how researchers process qualitative data. With the growing demand for efficiency and accuracy, traditional methods of manual analysis often fall short, leading to inconsistencies and delays. Researchers are increasingly turning to AI tools to streamline their workflow, ensuring that insights derived from interviews are both timely and reliable.
In this section, we will explore the fundamentals of AI-Driven Interview Analysis and its significance in academic research. By automating data processing and identifying key themes, these tools not only improve the reliability of findings but also allow researchers to focus on deeper analytical tasks. Understanding these innovations will equip academics with the strategies needed to harness AI effectively in their qualitative research endeavors.
Extract insights from Customer & Employee Interviews. At Scale.
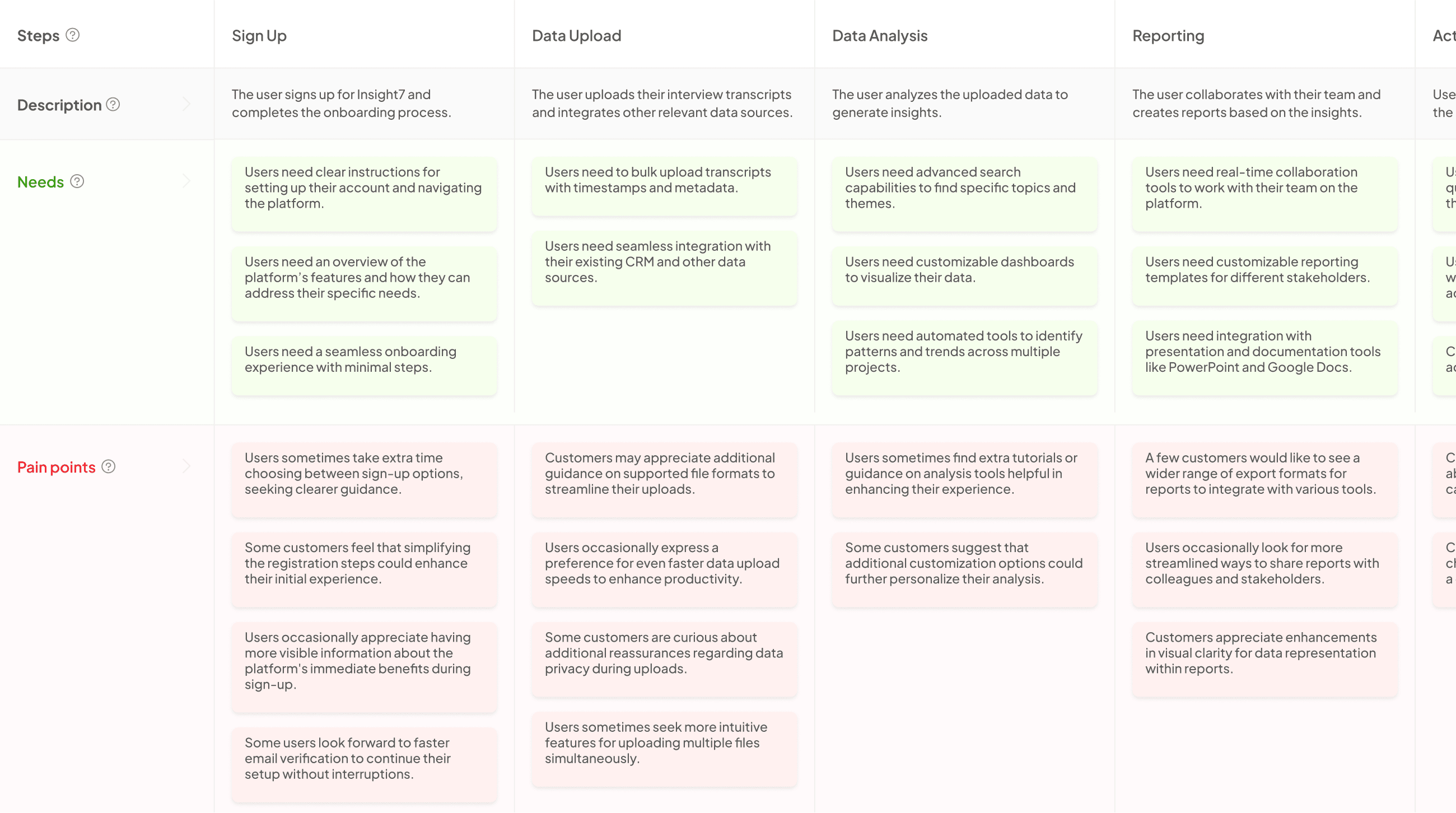
Understanding the Basics of AI-Driven Interview Analysis
AI-Driven Interview Analysis employs advanced algorithms to streamline and enhance the qualitative research process. By automating the analysis of interview transcripts, researchers can save significant time and reduce the risk of bias inherent in manual analysis. This technology aims to produce consistent insights across multiple interviews, thereby ensuring that researchers can focus on deriving conclusions rather than spending hours on data processing.
Understanding the fundamentals of this technology begins with recognizing its capabilities. AI can identify themes, patterns, and sentiments within qualitative data, turning extensive transcripts into actionable insights. Additionally, it increases research efficiency, allowing teams to quickly report findings and respond to client needs. Ultimately, AI-Driven Interview Analysis represents a transformative step for academic researchers, ensuring both thoroughness and precision in the research analysis phase.
What is AI-Driven Interview Analysis?
AI-driven interview analysis is a cutting-edge approach that harnesses artificial intelligence to streamline the examination of qualitative data gathered from interviews. This method enables researchers to analyze vast amounts of textual data efficiently, minimizing the time-consuming labor traditionally involved in manual analysis. By utilizing natural language processing and machine learning algorithms, AI-driven interview analysis can uncover themes, sentiments, and patterns within interview responses that may be easily overlooked by human researchers.
The application of AI-driven interview analysis offers several advantages, particularly for academic research. It reduces bias by providing consistent analysis across different data sets and researchers. Additionally, researchers can access insights faster, allowing for timely decision-making and enhanced research output. By adopting this technology, academic researchers can transform their data analysis processes, ensuring that they remain competitive and effective in today’s fast-paced research environment.
Benefits of AI-Driven Interview Analysis in Academic Research
AI-Driven Interview Analysis offers pivotal advantages in academic research by enhancing data accuracy and reducing the time spent on manual analysis. By utilizing this technology, researchers can efficiently manage large volumes of qualitative data, allowing for quicker insights into complex topics. Furthermore, AI-driven tools help minimize human bias, ensuring a more consistent approach to interpreting interview transcripts.
Another significant benefit is the potential for richer thematic understanding. With AI's ability to discern patterns and key themes swiftly, researchers can uncover insights that may be overlooked in traditional analysis. This not only accelerates the research process but also elevates the quality of findings, leading to more robust conclusions. Embracing AI-Driven Interview Analysis ultimately empowers academics to focus on deeper inquiry and meaningful interpretation, significantly contributing to the advancement of their fields.
Tools for AI-Driven Interview Analysis in Academic Research
AI-driven interview analysis offers researchers a way to enhance the efficiency and accuracy of their qualitative data processing. Using advanced AI tools, researchers can automate the analysis of interview transcripts, significantly reducing time spent on manual tasks. These tools help identify patterns and themes more consistently, allowing for quick insights generation that meets the demands of today’s fast-paced research environment.
Several tools stand out in the realm of AI-driven interview analysis. Nvivo provides comprehensive qualitative data analysis, while Sonix focuses on accurate transcription services. Otter.ai excels in real-time transcription and collaboration, making it suitable for teams. Dovetail specializes in synthesizing insights from interviews and other qualitative data sources. Lastly, Transcribr ensures accurate and efficient transcription. Each tool caters to different research needs, helping academic researchers streamline their workflows and focus on generating impactful insights.
Generate Journey maps, Mind maps, Bar charts and more from your data in Minutes
Insight7: The Top Choice for Researchers
In the realm of academic research, choosing the right tools is crucial for effective outcomes. Insight7 stands out as a premier option for researchers seeking efficiency in analyzing interview data. This AI-driven tool streamlines the process, allowing researchers to convert extensive interview transcripts into actionable insights swiftly. Through advanced algorithms, Insight7 can automate the initial stages of analysis, freeing up valuable time for researchers to delve deeper into their findings.
Moreover, this platform not only enhances productivity but also ensures that researchers can maintain a comprehensive understanding of their data. By adopting Insight7 for AI-driven interview analysis, researchers benefit from robust support and features tailored specifically to academic needs. This approach transforms the often tedious task of interviewing and analyzing responses into a more manageable and insightful process, empowering researchers to focus on synthesizing knowledge and drawing meaningful conclusions from their work.
Other Prominent Tools for AI-Driven Interview Analysis
AI-Driven Interview Analysis is becoming increasingly vital in academic research, enabling researchers to derive insights efficiently from qualitative data. Various tools are available that cater to different analytical needs, improving productivity and reducing bias in the process. Among the prominent tools are Nvivo, known for its qualitative research capabilities; Sonix, which excels in transcription accuracy; and Otter.ai, appreciated for its user-friendly interface.
Dovetail and Transcribr also stand out for their capability to transform interview data into actionable insights. Dovetail focuses on collaboration and sharing within research teams, ensuring a streamlined analysis process. In contrast, Transcribr simplifies transcription, allowing researchers to focus on analysis rather than manual note-taking. Each tool carries unique features that can significantly augment the effectiveness of AI-Driven Interview Analysis, catering to the diverse requirements of academic researchers.
- Nvivo: Features and Benefits
Nvivo offers powerful features designed to streamline the qualitative analysis process, making it an ideal choice for researchers. Its intuitive interface allows users to organize and code vast amounts of interview data efficiently. This helps in identifying themes and patterns without the confusion often associated with manual coding approaches. With AI-driven interview analysis, researchers can integrate advanced algorithms that assist in categorizing responses, ensuring no valuable insight is overlooked.
Additionally, Nvivo provides robust visualization tools, enabling researchers to create compelling data presentations. This functionality aids in translating complex findings into digestible formats for academic audiences. By harnessing these features, researchers can enhance their analytical rigor while saving time. Overall, the advantages of using Nvivo extend beyond mere data management; they significantly improve the quality and clarity of research outcomes, empowering users to draw meaningful conclusions from their interview analysis.
- Sonix: Features and Benefits
In academic research, the use of AI-driven interview analysis tools offers distinctive features and benefits that can significantly enhance the research process. These tools streamline data collection by providing an integrated transcription service that simplifies the ingestion of various media formats. Researchers can transcribe audio and video files without needing additional software, saving time and effort.
Once the data is transcribed, users gain access to a project management workspace where they can analyze transcripts collectively or individually. This capability allows researchers to extract key themes, relevant quotes, and clips efficiently. Furthermore, the built-in reporting features facilitate easy synthesis of insights derived from analysis, thereby enhancing the overall quality of the research. By prioritizing data privacy and employing advanced text analytics, these tools ensure a reliable and secure analysis environment tailored for academic needs.
- Otter.ai: Features and Benefits
Otter.ai offers a range of features designed to enhance the process of AI-driven interview analysis. Its automatic transcription capability allows users to capture spoken words in real-time, ensuring that no valuable insights are lost. This feature is particularly beneficial for academic research, as it transforms raw audio into searchable text, making it easier to analyze discussions and identify recurring themes. Users can also highlight and organize key points during interviews, which streamlines the process of synthesizing findings.
Another significant benefit includes collaboration tools that allow researchers to share transcripts and notes with team members seamlessly. This promotes a more integrated approach to data analysis by enabling collective insights. Furthermore, with integrated AI capabilities, researchers can gain deeper analytical perspectives, turning qualitative interview data into actionable, quantitative insights—a crucial step in academic research leveraging AI-driven interview analysis. By adopting this tool, researchers can greatly improve efficiency and effectiveness in their academic endeavors.
- Dovetail: Features and Benefits
Dovetail offers a comprehensive suite of features aimed at facilitating AI-driven interview analysis for academic research. One of its notable strengths is the ease of configuring project goals, allowing researchers to tailor their focus, whether it is improving engagement or driving sales insights. This flexibility ensures that users can derive meaningful outcomes directly correlated with their research objectives.
Additionally, Dovetail simplifies the process of extracting themes and insights from interview data. Users can view and edit insights and themes in a user-friendly manner, ensuring accuracy and context integrity. The ability to publish structured reports with a summary and key findings integrates seamlessly into academic workflows. Thus, Dovetail not only enhances the efficiency of analyzing interview data but also supports a clear presentation of findings, ultimately contributing to more robust academic research outcomes.
- Transcribr: Features and Benefits
Transcribr provides a seamless transcription service that elevates the process of analyzing interviews. One of its standout features is the ability to transcribe audio and video files directly into projects, allowing researchers to efficiently organize their data. This integration means that users can quickly access transcripts and begin analysis without the need for external tools. The platform supports bulk uploads, which is ideal when dealing with numerous interviews.
Another significant benefit is its focus on text analysis. Once transcripts are generated, researchers can explore themes, extract direct quotes, and compile reports for their academic work. The intuitive interface simplifies navigation between project folders and libraries, making it accessible even for those without extensive technical expertise. Overall, Transcribr supports researchers in harnessing AI-driven interview analysis effectively, enhancing both the quality and speed of data processing.
Steps to Implement AI-Driven Interview Analysis Effectively
To implement AI-Driven Interview Analysis effectively, start by selecting the right AI tool tailored to your needs. Your choice will significantly impact the quality and efficiency of the analysis. Research various tools, comparing their capabilities such as transcription accuracy, data visualization, and user interface. Clarity on user requirements will guide you in choosing a tool that enhances your research workflow.
Next, prepare your interview data by organizing transcriptions and ensuring that they are clean and structured. This step is crucial, as messy or incomplete data can yield misleading results. After preparing your data, conduct the analysis using features offered by your chosen AI tool. This may involve identifying themes, generating insights, and visualizing trends.
Finally, interpret the results carefully. Understanding the implications of the AI analysis ensures the findings contribute meaningfully to your research objectives. By following these steps, you will harness the capabilities of AI efficiently, leading to more reliable and actionable insights.
Step 1: Selecting the Right AI Tool
Choosing the appropriate AI tool for interview analysis is a crucial first step in academic research. With numerous options available, researchers must carefully consider their specific needs and goals before making a selection. Key factors include the tool's ability to accurately analyze qualitative data, support for various file formats, and ease of use. A tool that integrates well with existing workflows can significantly enhance efficiency and accuracy in the analysis process.
When evaluating potential AI-driven tools for interview analysis, researchers should also consider the level of customization available. Features like thematic analysis, keyword extraction, and data visualization can provide deeper insights and facilitate a better understanding of the data. Furthermore, investing in a tool that offers strong reporting capabilities can enhance the presentation of research findings, ensuring they resonate with both academic and broader audiences. By thoughtfully selecting the right AI tool, researchers can unlock the true potential of their interview data, leading to richer, more informed conclusions.
Step 2: Preparing Your Interview Data for Analysis
Preparing your interview data for analysis is a crucial step in conducting effective academic research. Proper data organization is essential, as it allows AI-driven interview analysis tools to process and derive insights efficiently. Start by transcribing your interviews accurately, ensuring that each conversation captures the nuances of participants’ voices. Many AI tools offer automatic transcription services, which can save time while maintaining a high level of accuracy.
Next, categorize your data effectively. Group interviews by themes, topics, or questions to facilitate deeper analysis. This organization allows AI tools to highlight patterns and discrepancies effortlessly. Furthermore, you may want to clean your data by removing irrelevant content or clarifying statements for enhanced clarity. Establishing a consistent data formatting structure will also make it easier to analyze and interpret results later. Essentially, these preparatory steps set a strong foundation for leveraging AI-driven interview analysis to uncover valuable insights in your research.
Step 3: Conducting the Analysis
Conducting the analysis with AI-driven tools involves systematic steps to ensure rigorous examination of your interview data. Begin by uploading your transcribed interviews into the selected AI platform. This software, designed for academic research, employs robust algorithms to identify patterns and themes within your data. For effective analysis, it’s crucial to set defined objectives and choose relevant tags that capture the core of your research questions.
After inputting the data, the AI tool will assist you in clustering insights into themes, such as collaboration or challenges, which can be vital for your study. This process typically includes sentiment analysis, allowing you to understand participant emotions behind responses. As you interact with these outputs, take time to validate and refine the AI-generated insights against your research goals, culminating in a comprehensive analysis that adds depth to your academic inquiries.
Step 4: Interpreting the Results
In Step 4, interpreting the results obtained from AI-driven interview analysis is crucial for translating raw data into meaningful insights. After conducting the analysis, researchers should start by identifying key themes that emerged from the transcripts. This involves categorizing insights into relevant buckets, such as participant sentiments, challenges, or desired improvements. Each category can reveal unique perspectives necessary for understanding the broader implications of the research findings.
Next, researchers should examine the qualitative evidence supporting these themes, such as participant quotes. This not only provides context but also enables a richer understanding of the data. By mapping insights to predetermined goals, researchers can assess how well the findings align with their initial hypotheses. Ultimately, this step is about synthesizing the information to guide future decisions and enhance research quality. Emphasizing clarity and relevance, the process will significantly contribute to the research's overall impact and applicability.
Conclusion: Embracing AI-Driven Interview Analysis for Better Research Outcomes
Integrating AI-Driven Interview Analysis into academic research can significantly enhance the quality and efficiency of data interpretation. By employing these advanced tools, researchers can quickly identify themes and insights, allowing for a more robust analysis. This shift not only streamlines the data processing but also minimizes biases that often arise from manual interpretation.
As researchers embrace this innovative approach, they are likely to see improved outcomes in their findings and reports. Adopting AI-driven methods offers the potential for greater accuracy and faster turnaround times, meeting the increasing demands for timely research results. Ultimately, the transition to AI-driven analysis represents a pivotal moment in academic research, fostering a more informed and insightful understanding of complex interview data.