AI Behavioral Tracking is transforming qualitative research by offering innovative solutions to age-old analysis challenges. Traditionally, researchers spend considerable time sifting through interview transcripts and survey responses, often resulting in biased insights and inconsistent findings. AI tools are now stepping in to automate these processes, enabling researchers to focus on what truly matters—understanding participant behavior.
In qualitative studies, AI Behavioral Tracking helps streamline data collection and analysis. It provides researchers with timely insights by systematically identifying patterns and shifts in behavior. This integration of technology not only enhances the efficiency of qualitative research but also ensures a more accurate representation of participant perspectives, leading to richer and more actionable conclusions.
Analyze qualitative data. At Scale.
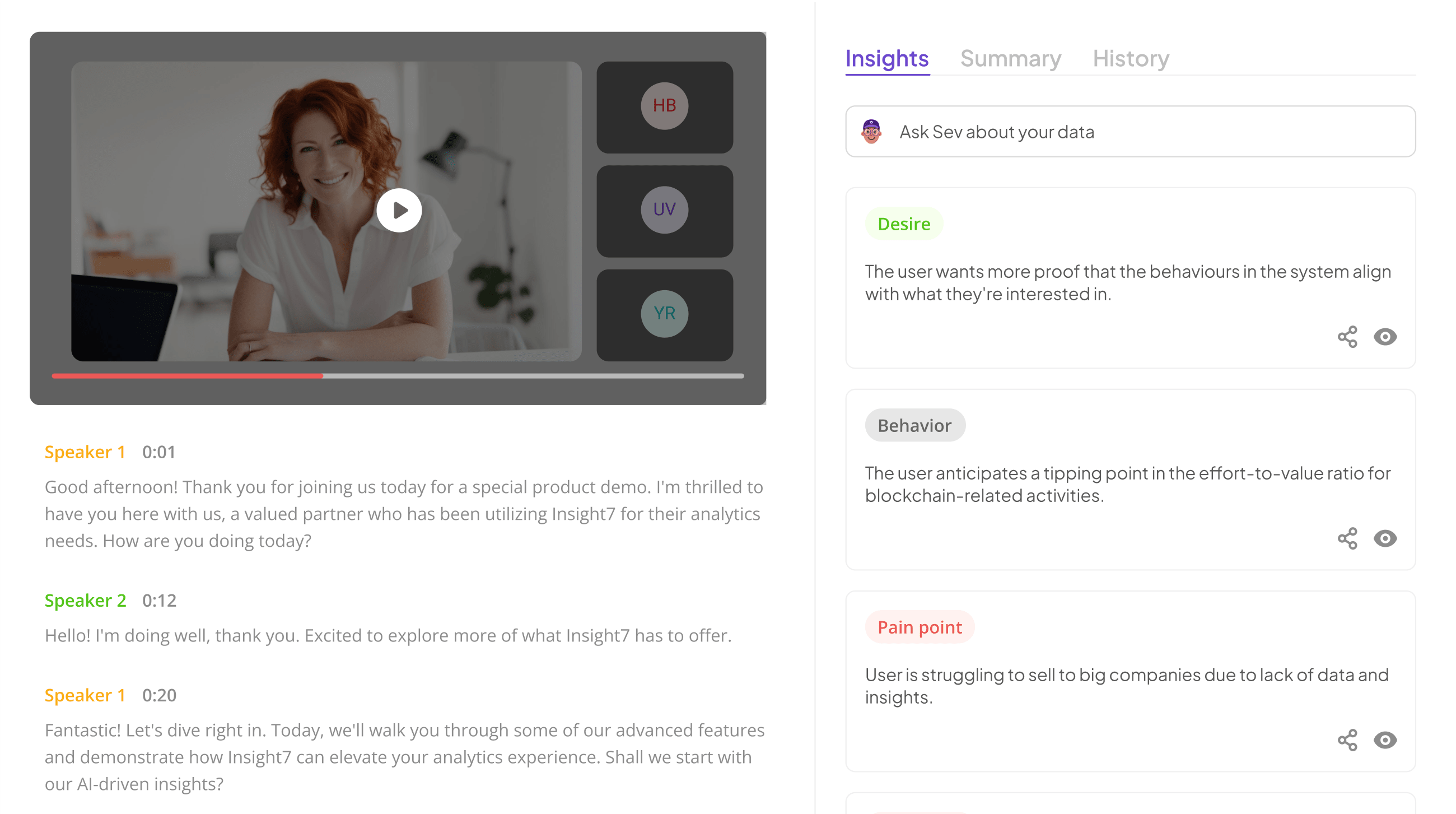
Understanding AI Behavioral Tracking Tools
AI Behavioral Tracking tools are instrumental in understanding the nuances of human behavior during qualitative studies. These tools use algorithms to analyze user interactions and patterns effectively. By leveraging large datasets, they identify trends and shifts in behavior that might not be readily apparent. This capability allows researchers to gain deeper insights into the motivations and sentiments of participants.
To navigate these tools effectively, it's essential to recognize their key features. First, they offer real-time data analysis, providing immediate feedback on behavioral trends. Second, visualization capabilities enhance comprehension by transforming complex data into digestible formats. Third, integration with existing research methodologies ensures that the transition to AI tracking is smooth and seamless. Understanding these features can significantly enhance the quality of qualitative research, ultimately driving more informed decision-making.
Key Features of AI Tools for Behavioral Analysis
AI tools for behavioral analysis are reshaping how researchers assess qualitative data. These powerful systems offer enhanced speed and accuracy, addressing key challenges in traditional analysis. One notable feature is automated data processing, which significantly reduces the time needed to analyze transcripts, interviews, and open-ended survey responses. This efficiency allows researchers to concentrate on drawing insights rather than getting bogged down by manual tasks.
Another important aspect of these tools is their ability to detect patterns and trends that may go unnoticed in conventional analysis. AI algorithms can analyze large volumes of qualitative data, delivering rich insights that inform decision-making. Furthermore, the use of AI in behavioral tracking also minimizes bias by standardizing the analysis process, ensuring consistent results regardless of the research team's size or experience. Overall, the integration of AI in behavioral analysis is not just a trend; it's a crucial step toward more effective and insightful research.
Top AI Tools for Tracking Behavioral Shifts
In today's evolving research landscape, a variety of AI tools specialize in tracking behavioral shifts within qualitative studies. These tools harness advanced algorithms and machine learning capabilities to provide nuanced insights from qualitative data, offering researchers the ability to analyze extensive datasets efficiently. They focus on patterns, sentiments, and trends, enabling more informed decision-making.
Highlighted below are some top AI tools for tracking behavioral shifts:
- Insight7: This platform streamlines qualitative data analysis, enhancing accuracy and speed.
- NVivo: Renowned for its user-friendly interface, it simplifies data coding and visualization.
- Dedoose: Ideal for mixed-method research, it permits robust collaboration and data integration.
- MAXQDA: This tool offers detailed analytics and visualization options, supporting deeper insights.
- ATLAS.ti: With its powerful qualitative analysis features, it assists in complex data coding tasks.
These AI behavioral tracking tools empower researchers to effectively identify shifts in participant behavior, ensuring that insights are both relevant and actionable. By integrating these technologies into your qualitative studies, you can enhance your analysis while minimizing biases commonly associated with manual data processing.
- Insight7
Insight7
In the realm of qualitative research, AI Behavioral Tracking emerges as a powerful tool for identifying and understanding behavioral shifts. By utilizing advanced algorithms, researchers can process extensive data, making it easier to spot trends and emerging patterns among participants' responses over time. This capability is vital for capturing nuanced insights that traditional methods may overlook.
The implementation of AI Behavioral Tracking requires careful strategy. First, selecting the appropriate AI tools tailored to your research needs ensures accuracy in data interpretation. Next, thorough data collection and preparation set the stage for successful analysis. Finally, applying AI tools to analyze and interpret the gathered behavioral data can reveal significant shifts in attitudes or preferences, fostering deeper engagement with your audience. Ultimately, AI Behavioral Tracking not only streamlines the research process but also empowers researchers to extract meaningful insights that inform decision-making.
- NVivo
NVivo is a popular tool in qualitative research, especially for analyzing complex data sets. Researchers use NVivo to organize and code their data, facilitating a thorough examination of behavioral shifts. By integrating AI functions, NVivo enhances the ability to track these shifts more effectively. Understanding how to harness NVivo for AI behavioral tracking can significantly improve the quality and depth of qualitative findings.
When working with NVivo, several features make it particularly effective. First, its coding capabilities allow for detailed categorization of qualitative responses. This aids in identifying themes that emerge as behavioral shifts occur. Second, NVivo supports data visualization, enabling researchers to create visual representations of their findings, which can reveal patterns over time. Lastly, NVivo integrates various data sources, including interviews, surveys, and social media content, providing a holistic view of behavioral changes. By adopting such tools, researchers can gain insights that drive strategic decisions and foster understanding in their studies.
- Dedoose
Dedoose plays a transformative role in AI behavioral tracking, particularly in qualitative research. Users can upload rich data from interviews and observations, making it easier to analyze behavioral shifts in a structured manner. The platform supports efficient data management, where researchers can engage with their qualitative data without overwhelming complexity.
One of its notable features is the ability to generate visualizations and comprehensive reports, which help in synthesizing insights. Furthermore, tools for theme extraction and quote identification simplify the analysis process, offering immediate access to pivotal data. Data privacy is also prioritized, ensuring compliance with regulations, which builds trust among researchers dealing with sensitive information. By employing AI behavioral tracking within Dedoose, researchers can foster improved collaboration and translation of insights into actionable strategies for their projects. This capability is essential for tackling the growing complexity of qualitative studies today.
- MAXQDA
MAXQDA enables researchers to harness AI for effective behavioral tracking in qualitative studies. This software is designed to facilitate the analysis of large amounts of text data, aiding researchers in identifying behavioral shifts among their subjects. By utilizing MAXQDA’s advanced text analysis features, users can streamline the process of categorizing and coding qualitative data, all while gaining valuable insights.
The platform offers integration with various AI tools that enhance data processing. Researchers can input raw data from interviews or questionnaires and let the software assist in automating initial analysis phases. This not only saves time but also allows for a more robust understanding of patterns and themes. Ultimately, by utilizing MAXQDA within the framework of AI behavioral tracking, researchers can better identify and interpret significant changes in behavior over time, leading to more informed conclusions and decision-making.
- ATLAS.ti
ATLAS.ti serves as a powerful tool for researchers focused on qualitative studies. Its advanced capabilities enable users to uncover intricate patterns and behavioral shifts within textual data. By utilizing AI behavioral tracking, researchers can efficiently analyze large volumes of qualitative information, allowing for deeper insights into participant attitudes and emotions.
This software offers features that facilitate the coding process, making it easier to categorize and explore data subsets related to behavioral changes. With visualizations and networking tools, the analysis becomes more intuitive, supporting a clearer understanding of emerging trends. Additionally, ATLAS.ti integrates with AI-driven methodologies, empowering researchers to monitor behavioral shifts in real-time. As such, it plays a crucial role in enhancing the overall research process and offers valuable insights into the dynamic nature of human behavior.
Extract insights from interviews, calls, surveys and reviews for insights in minutes
Implementing AI Behavioral Tracking in Research
Integrating AI behavioral tracking in research begins with understanding its potential to redefine qualitative studies. AI behavioral tracking tools help streamline data collection and analysis, leading to quicker and more consistent insights. This process can address challenges faced in traditional qualitative research where manual analysis often results in bias and delays. By implementing these tools, researchers can focus on more significant trends instead of getting lost in the minutiae of raw data.
To effectively implement AI behavioral tracking, researchers should follow several key steps. First, data collection and preparation involve organizing interviews or survey responses for AI processing. Next, selecting the right tools is crucial, considering factors like ease of use and analytical capabilities. Lastly, analyzing and interpreting the results requires critical thinking to convert raw data into actionable insights. Following these steps can ensure a smooth transition to AI-enhanced research, ultimately enriching the research outcome with more reliable behavioral shifts in qualitative data.
Step-by-Step Guide to Setting Up AI for Behavioral Tracking
Setting up AI for behavioral tracking is an essential process in qualitative research, allowing researchers to analyze nuanced human behaviors effectively. The first step involves thorough data collection and preparation; gather qualitative data from interviews, focus groups, or surveys, ensuring it spans diverse perspectives. Once the data is in place, it's crucial to select the right AI tools tailored for behavioral analysis. Various platforms offer unique functionalities, enabling researchers to choose based on specific project requirements.
Next, the analysis and interpretation of behavioral data come into play. Employ AI algorithms that cluster themes, identify patterns, and generate insights from the collected data. This method accelerates the research process, enabling you to spot behavioral shifts more efficiently. By following these steps, you can harness AI behavioral tracking to transform qualitative studies and derive significant insights that inform decision-making.
Step 1: Data Collection and Preparation
In the journey of utilizing AI for tracking behavioral shifts, the first critical step is data collection and preparation. This phase sets the groundwork for effective analysis by ensuring that the right qualitative data is gathered systematically. Begin by identifying the specific behaviors you want to analyze and designing your data collection strategy accordingly. Utilize interviews, focus groups, and surveys to gather rich qualitative insights that are essential for AI behavioral tracking.
Once the data is collected, the next task is preparation. This involves cleaning and organizing the data to make it suitable for analysis. Transcribing interviews accurately, categorizing responses, and filtering out irrelevant information are all essential activities. The more structured your data is, the more effectively AI can help reveal behavioral insights. By focusing on careful collection and preparation, you'll ensure a robust foundation for the subsequent steps in analyzing and interpreting behavioral patterns.
Step 2: Selecting the Right AI Tools
To effectively harness the power of AI behavioral tracking, selecting the right tools is crucial. Start by evaluating the specific needs of your qualitative study. Consider the features offered by various AI tools, as they can significantly influence the insights gleaned from your data. Look for tools that excel in thematic analysis, sentiment detection, and data visualization. These features will enable you to identify behavioral shifts with greater accuracy and depth.
Next, assess user-friendliness and compatibility with your existing systems. The ideal AI tool should facilitate seamless integration and allow easy collaboration among team members. Once you narrow down your options, review each tool's performance through user testimonials or trial periods to ensure it meets your expectations. Making an informed decision in selecting the right AI tools will ultimately enhance your research quality and uncover meaningful behavioral insights.
Step 3: Analyzing and Interpreting Behavioral Data
In Step 3: Analyzing and Interpreting Behavioral Data, it's crucial to leverage AI Behavioral Tracking to derive meaningful insights. After collecting your qualitative data, the next step involves employing AI tools to analyze patterns and themes. Start by defining your analytical goals; for instance, are you interested in understanding user retention or pinpointing specific challenges? AI tools can categorize your data into themes, allowing for the extraction of valuable insights related to those goals.
Once the data is organized into themes, you can delve deeper into sentiment analysis. This step involves examining participant quotes and sentiments linked to each theme to identify underlying motivations or trends. By systematically analyzing the data, you'll uncover significant behavioral shifts, making your findings more actionable and relevant. This process not only enhances the understanding of qualitative insights but also supports decision-making based on solid data.
Evaluating the Effectiveness of AI in Behavioral Shift Analysis
Evaluating the effectiveness of AI in behavioral shift analysis involves a multi-faceted approach. First, it is critical to recognize how AI tools collect and analyze qualitative data to reveal behavioral trends. Effective AI behavioral tracking can enhance understanding by providing insights that would be difficult to discern through traditional methods. These tools can analyze large data sets rapidly and identify patterns, thus facilitating timely interventions.
Next, the reliability of AI insights must be considered. It is essential to assess how accurately AI detects and interprets behavioral shifts, as distorted insights may lead to misguided strategies. Regularly reviewing outcomes against set benchmarks ensures that AI-driven interpretations stay aligned with real-world behaviors. Additionally, integrating user feedback can refine AI models, enhancing their adaptability. Therefore, when evaluating AI's role in behavioral shift analysis, a comprehensive view of accuracy, usability, and adaptability is paramount to maximizing its potential and aligning it with user needs.
Conclusion: The Future of AI Behavioral Tracking in Qualitative Studies
The future of AI behavioral tracking in qualitative studies holds tremendous potential for enhancing research efficiency and accuracy. As AI tools continue to evolve, they will enable researchers to analyze vast amounts of qualitative data with remarkable speed. This advancement allows for more timely insights and reduces the potential for human bias, which often complicates manual analysis.
Furthermore, the integration of AI behavioral tracking can facilitate more nuanced understanding of consumer behavior, unveiling patterns that might remain hidden through traditional methods. By adopting these technologies, researchers will not only streamline their workflow but also enrich the depth of their qualitative findings. The journey ahead promises exciting developments in how we understand human behavior through qualitative studies.