AI-enhanced Mixed Research in Interviews represents a transformative approach to data analysis that combines qualitative and quantitative methods. Traditional interview analysis often reveals inconsistencies and biases, leading to delayed insights. With advancements in AI, researchers can streamline this process significantly, enhancing both accuracy and efficiency. Imagine being able to analyze hours of interview data in a fraction of the time, enabling quicker decision-making and improved insights for clients.
Incorporating AI technologies into mixed methods research offers various benefits. For instance, AI can automate data collection and transcription, minimizing human error and freeing up researchers to focus on strategic interpretation. Additionally, AI enables deeper engagement with qualitative data through coding and thematic analysis, allowing teams to uncover nuanced patterns that might otherwise go unnoticed. This integration not only accelerates the research process but also enhances the credibility and reliability of findings.
Analyze qualitative data. At Scale.
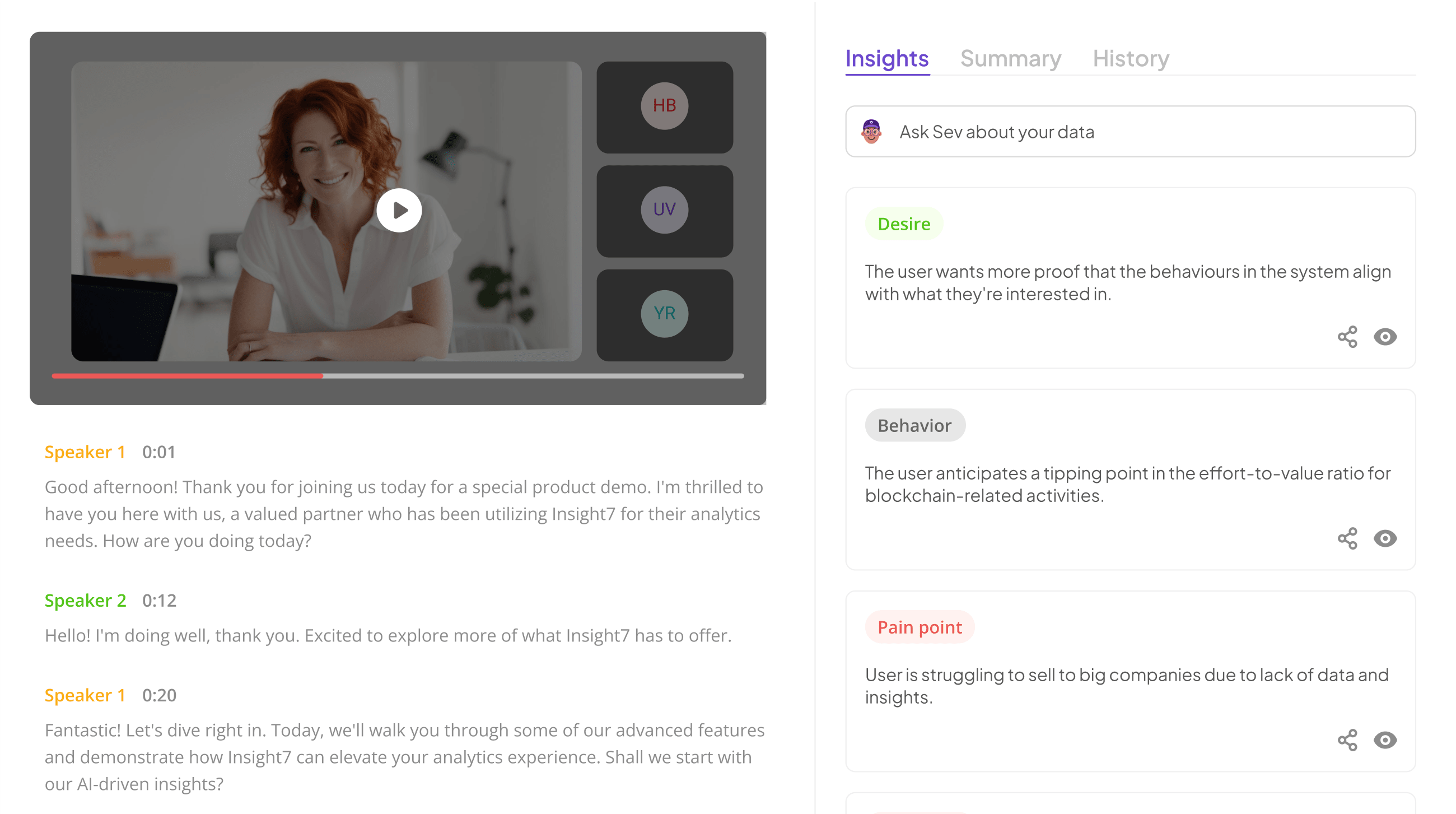
Leveraging AI Tools for AI-enhanced Mixed Research
AI-enhanced Mixed Research streamlines complex data collection and analysis processes, making research projects more efficient. By integrating intelligent tools, researchers can significantly improve the accuracy and speed of insights derived from interviews. One of the critical aspects of applying AI is its ability to automate data collection and transcription. This removes time-consuming manual steps, allowing researchers to focus more on analyzing the data itself.
Another essential application is the use of AI in coding and thematic analysis. Machine learning algorithms can analyze interview content and identify key themes, thus optimizing the qualitative analysis process. This not only decreases bias but also ensures consistency across research teams. Embracing these AI tools enables researchers to produce quicker and more reliable reports, meeting the increasing demands for timely insights in today’s fast-paced environment. Ultimately, adopting AI-enhanced Mixed Research fosters deeper understanding and better decision-making based on comprehensive data analysis.
Exploring Key Applications
AI-enhanced Mixed Research offers numerous applications that can streamline the research process from interviews. One significant application involves using AI for data collection and transcription. This automation not only saves time but ensures a higher level of accuracy when capturing participant responses. Consequently, researchers can focus on more complex analysis tasks instead of labor-intensive transcription duties.
Another key application is integrating AI into coding and thematic analysis. Researchers can set specific goals, such as identifying risks or improving member experiences, while AI tools categorically cluster insights based on predefined themes. Additionally, AI-driven sentiment analysis provides valuable context, allowing for deeper understanding and richer interpretations of qualitative data. By leveraging these applications, researchers can enhance their ability to draw meaningful conclusions, ultimately improving the robustness and reliability of their mixed methods research.
- Using AI for Data Collection and Transcription
AI-enhanced Mixed Research offers researchers innovative tools for data collection and transcription, particularly through automated processes. These AI innovations streamline how data from interviews is gathered and organized, significantly reducing the time and effort involved. Researchers can quickly transcribe interviews in bulk without relying on external software, allowing for seamless integration of textual data into their ongoing projects. This efficiency facilitates a more comprehensive analysis when comparing qualitative insights across various interviews.
Moreover, AI technology enhances the accuracy of transcriptions, minimizing human error and ensuring consistency. Once the data is transcribed, researchers can analyze themes and patterns effectively, generating actionable insights with precision. The ability to interrogate the data by extracting quotes and creating reports makes it a handy feature for comprehensive mixed methods research. Embracing AI for these tasks not only fosters a more dynamic research environment but also empowers researchers to draw deeper insights with greater confidence and speed.
- AI in Coding and Thematic Analysis
AI is transforming the field of coding and thematic analysis, making previously labor-intensive tasks more efficient. Through AI-enhanced mixed research, researchers can utilize advanced algorithms to identify patterns in qualitative data quickly. This lays the foundation for effective thematic analysis, where raw data is transformed into meaningful insights. By deploying AI tools, researchers can streamline the coding process, allowing them to categorize responses into predefined themes or develop new ones based on emerging data patterns.
Additionally, AI can assist in sentiment analysis, providing a comprehensive understanding of the emotional tone within participant responses. By clustering insights into defined "buckets," researchers can visualize trends and sentiments with clarity, enhancing their analytical narratives. This dual approach not only saves time but also improves the reliability and depth of qualitative research findings. Ultimately, AI's role in coding and thematic analysis is pivotal to achieving richer insights in mixed methods research.
Top AI Tools for Mixed Methods Research
In the realm of AI-enhanced Mixed Research, selecting the right tools can significantly streamline the data analysis process. Various AI tools have emerged as essential for researchers who aim to combine qualitative and quantitative data insights effectively. Some of the top picks include NVivo, known for its robust coding capabilities, and MAXQDA, which allows for versatile data visualization. Dedoose offers user-friendly collaboration features, while ATLAS.ti specializes in handling complex qualitative data. Finally, insight7 stands out for its emphasis on centralizing customer insights.
These tools not only save time but also minimize bias in data interpretation. As researchers face growing demands for quicker insights and comprehensive reports, integrating these AI tools into the mixed methods research process becomes crucial. Embracing technology enhances the rigor of qualitative analysis while enabling researchers to stay agile in their methodologies.
- insight7
In the realm of AI-enhanced Mixed Research, the synthesis of qualitative and quantitative data provides a more nuanced understanding of interview outcomes. Insight7 illustrates how artificial intelligence can transform traditional interview methodologies into a more streamlined process. By integrating AI tools, researchers can automate data collection and analysis, effectively reducing bias and enhancing reliability in results.
To fully grasp the benefits of AI-enhanced Mixed Research, consider the following key aspects:
Data Collection Efficiency: AI can be employed to automate interview transcriptions, allowing researchers to focus on deeper analysis rather than labor-intensive tasks.
Thematic Analysis: With AI, coding qualitative data becomes faster and more systematic. Machine learning algorithms identify themes and patterns that may not be immediately apparent.
Enhanced Insights: The combination of AI with mixed methods allows researchers to gain actionable insights, driving informed decision-making processes more effectively.
Data Reliability: AI reduces human error in coding and analysis, fostering a more accurate representation of the data collected.
These elements illustrate how AI enhances the depth and breadth of gathered insights, fundamentally changing the landscape of research practices.
- NVivo
NVivo is a significant player in the realm of AI-enhanced mixed research, especially when it comes to analyzing qualitative data. Its capabilities allow researchers to manage, analyze, and visualize data derived from interviews effectively. This tool provides a platform for coding responses, identifying themes, and generating insightful visual representations of data relationships. By integrating AI algorithms, NVivo enhances the traditional coding process, making it more efficient and thorough.
Furthermore, NVivo simplifies collaboration among research team members by allowing them to share insights and findings easily. The tool can analyze large volumes of text, revealing patterns and trends that might be missed during manual analysis. This efficiency leads to a deeper understanding of the data and supports robust findings in mixed methods research. Essentially, NVivo not only streamlines the research process but also enriches the quality of insights generated, positioning itself as an invaluable resource for researchers.
- MAXQDA
MAXQDA serves as a powerful tool in the realm of AI-enhanced Mixed Research, especially when it comes to analyzing interview data. This software is designed to facilitate qualitative and quantitative data analysis, enabling you to make sense of complex information streams. With its robust features, researchers can code interviews, identify patterns, and extract meaningful insights swiftly. This streamlining aids in bridging traditional qualitative methods with quantitative metrics, promoting a more holistic view of research findings.
Incorporating MAXQDA into your research process allows for an efficient first pass on transcripts. Automated features assist in coding and thematic analysis, freeing researchers to focus on deeper analytical tasks. The integration of AI technology enhances your ability to manage large volumes of interview data, thereby supporting timely decision-making in Mixed Methods Research. Utilizing such tools can significantly reduce the time spent on data management, ultimately enriching the research experience and outcome.
- Dedoose
In the realm of AI-enhanced mixed research, Dedoose stands out as a versatile tool for analyzing qualitative data from interviews. This platform simplifies the process of data collection, allowing researchers to upload audio files for automatic transcription. With its ability to support multiple languages, users can quickly convert spoken conversation into written text, making the initial step of analysis much more manageable. Dedoose also enables efficient organization, allowing researchers to categorize themes, quotes, and key insights all in one place.
Furthermore, its collaborative features foster teamwork among researchers, as they can share projects and collectively dive into data analysis. The tool offers powerful visualization capabilities, allowing users to generate comprehensive reports that highlight critical findings from their interviews. By streamlining the data analysis process, Dedoose enhances the overall efficiency and effectiveness of AI-enhanced mixed research, empowering researchers to extract actionable insights that inform decision-making.
- ATLAS.ti
ATLAS.ti serves as a vital tool in the realm of AI-enhanced mixed methods research, particularly in managing and analyzing qualitative data. By integrating AI functionalities into its framework, this software not only simplifies the management of extensive interview datasets but also aids in revealing deeper insights. Researchers can utilize its features for effectively coding data, allowing for the identification of patterns and themes that might otherwise go unnoticed.
The platform makes it easier to combine qualitative insights from interviews with quantitative data, facilitating a comprehensive understanding of research questions. This synergy between AI-enabled tools and mixed methods research promotes a thorough analysis of complex social phenomena. By relying on data visualization and network-building features, researchers can illustrate connections more clearly. Overall, ATLAS.ti empowers researchers to enhance their investigative efforts, making the journey from raw data to actionable insights both efficient and meaningful.
Extract insights from interviews, calls, surveys and reviews for insights in minutes
Steps to Implementing AI-enhanced Mixed Research
To implement AI-enhanced Mixed Research effectively, it’s essential to follow a structured approach. The first step involves preparing data for AI analysis. This includes organizing interview transcripts by ensuring they are accurately transcribed and formatted correctly. Data cleaning, such as removing irrelevant information or correcting transcription errors, is crucial to guarantee that the AI tools can analyze the raw data effectively.
The second step focuses on integrating AI into data analysis. You can utilize machine learning for pattern recognition, which enables the identification of trends and insights that might be missed through manual analysis. Additionally, employing text analysis and sentiment analysis with AI tools allows for a nuanced understanding of participant emotions and perceptions. This comprehensive approach ensures that AI-enhanced Mixed Research not only streamlines the analysis process but also enhances the quality of insights derived from interviews, ultimately leading to more effective decision-making in research.
Step 1: Preparing Data for AI Analysis
Preparing data for AI analysis begins with the meticulous organization of interview transcripts. The primary goal is to ensure that the data is clean and properly formatted for effective analysis. Begin by transcribing your interviews accurately to retain the interviewees' intent and nuances. This transcription process is vital as it forms the foundation for AI-enhanced mixed methods research.
After transcription, the next step is data cleaning. This involves removing irrelevant information, correcting errors, and standardizing formatting. In addition, consider categorizing responses based on themes or topics, which can facilitate deeper analysis later on. By following these steps, researchers can create a structured dataset that enhances the capabilities of AI tools, enabling them to extract valuable insights seamlessly. Ultimately, well-prepared data sets the stage for more effective AI applications, transforming qualitative interviews into actionable findings.
- Setting Up Interview Transcripts
To effectively set up interview transcripts, begin by ensuring that you have a reliable transcription method. This process is crucial for AI-enhanced Mixed Research, as accurate transcripts form the foundation for further analysis. Many platforms offer native transcription services, which can convert audio files into text with notable accuracy. Aim for platforms that support multiple languages and allow bulk uploads. This feature saves time, allowing you to focus on insights rather than manual data entry.
Next, organize your transcripts within a dedicated project framework. This organizational structure helps you to identify themes, quotes, and key clips efficiently. With your transcripts in place, you can apply AI tools to extract relevant insights and create visualizations. This systematic approach not only enhances the clarity of your data but also facilitates collaborative analysis, making it easier to draw actionable conclusions from the interviews you've conducted.
- Data Cleaning and Formatting Techniques
Data cleaning and formatting are crucial steps in preparing your data for AI-enhanced mixed research. This process ensures that the data from interviews is accurate, consistent, and ready for analysis. First, begin by transcribing the audio or video recordings of your interviews. Utilize AI transcription tools for quicker and more precise results. Once transcriptions are complete, it’s essential to clean the data by removing any irrelevant information, correcting transcription errors, and ensuring that names or sensitive information is anonymized.
Next, focus on formatting the cleaned data. Establish a consistent structure for your files, applying headings and clear labels for questions and responses. Additionally, consider coding significant themes from interviews systematically without overlooking nuances. By using tags or categories, you can enhance the effectiveness of your analysis later. Ultimately, these data cleaning and formatting techniques not only prepare your data for AI analysis but also improve the reliability of insights gained from AI-enhanced mixed research.
Step 2: Integrating AI in Data Analysis
Integrating AI in Data Analysis enhances the capacity of mixed methods research significantly. AI can sift through large volumes of qualitative data, uncovering patterns and themes often missed in manual analysis. By employing machine learning algorithms, researchers can identify relationships within data, allowing for more robust conclusions drawn from interviews.
A few key strategies can be employed to leverage AI for analysis. First, utilize machine learning techniques to recognize patterns and trends across interview responses, enabling deeper insights. Second, implement text analysis to extract significant themes, facilitating a comprehensive understanding of participant sentiments. Lastly, sentiment analysis can assess emotional tones present in the interview data, further enriching the findings. These approaches ensure that the insights derived from mixed methods research are accurate, reliable, and profoundly impactful, guiding future decisions and strategies.
- Utilizing Machine Learning for Pattern Recognition
In the context of AI-enhanced mixed research, utilizing machine learning for pattern recognition plays a critical role. By employing machine learning algorithms, researchers can sift through large volumes of interview data, identifying recurring themes and significant patterns. This systematic approach allows for deeper insights that may not be readily observable through traditional qualitative analysis.
Machine learning excels at detecting nuances in language and sentiment, offering a comprehensive understanding of participants' perspectives. For instance, by analyzing word choice and emotional tone, researchers can uncover areas of agreement or contention among respondents. This capability not only streamlines the analysis process but also enhances the overall rigor of the findings. Incorporating machine learning into mixed methods research provides a pathway to generate more actionable insights, ultimately supporting better decision-making based on qualitative data.
- Text Analysis and Sentiment Analysis with AI
Text analysis and sentiment analysis with AI are transformative tools for enhancing mixed methods research. By leveraging these technologies, researchers can obtain deeper insights from interview transcripts. Text analysis involves breaking down large volumes of qualitative data into manageable themes, making it easier to identify trends and patterns. This process assists researchers in understanding participants' perspectives more effectively.
Sentiment analysis complements text analysis by assessing the emotional tone behind the words. Utilizing AI algorithms, researchers can gauge whether sentiments expressed in interviews are positive, negative, or neutral. This dual approach empowers researchers to paint a comprehensive picture of participants' feelings and opinions. Together, text and sentiment analysis enrich the thematic findings of AI-enhanced mixed research, paving the way for more robust and nuanced conclusions.
Conclusion: The Future of AI-enhanced Mixed Research in Interviews
The future of AI-enhanced mixed research in interviews is promising and transformative. As artificial intelligence continues to develop, we can expect the integration of advanced tools that streamline the research process and improve data accuracy. AI will increasingly assist in gathering, analyzing, and interpreting qualitative data, making it easier to draw meaningful insights from interviews.
Moreover, combining AI with traditional methods fosters a richer understanding of participant perspectives. This integration promotes a more iterative and responsive research approach, allowing researchers to continuously refine their methods based on initial findings. In this evolving landscape, embracing AI-enhanced mixed research will empower researchers to delve deeper into the voices of interviewees, paving the way for insights that drive impactful decision-making.