AI-driven Qualitative Summarization is transforming the landscape of longitudinal research, addressing critical challenges faced by researchers. Manually analyzing interviews and open-ended surveys often leads to inconsistencies and delays in insights. As research teams strive for quicker, more accurate reports, the integration of AI offers a promising solution to streamline the summarization process.
By leveraging AI-driven tools, researchers can effectively identify themes, trends, and insights within extensive qualitative data. This not only enhances the quality of analysis but significantly reduces the time it takes to generate comprehensive summaries, allowing teams to focus on interpreting results and making data-driven decisions.
Analyze qualitative data. At Scale.
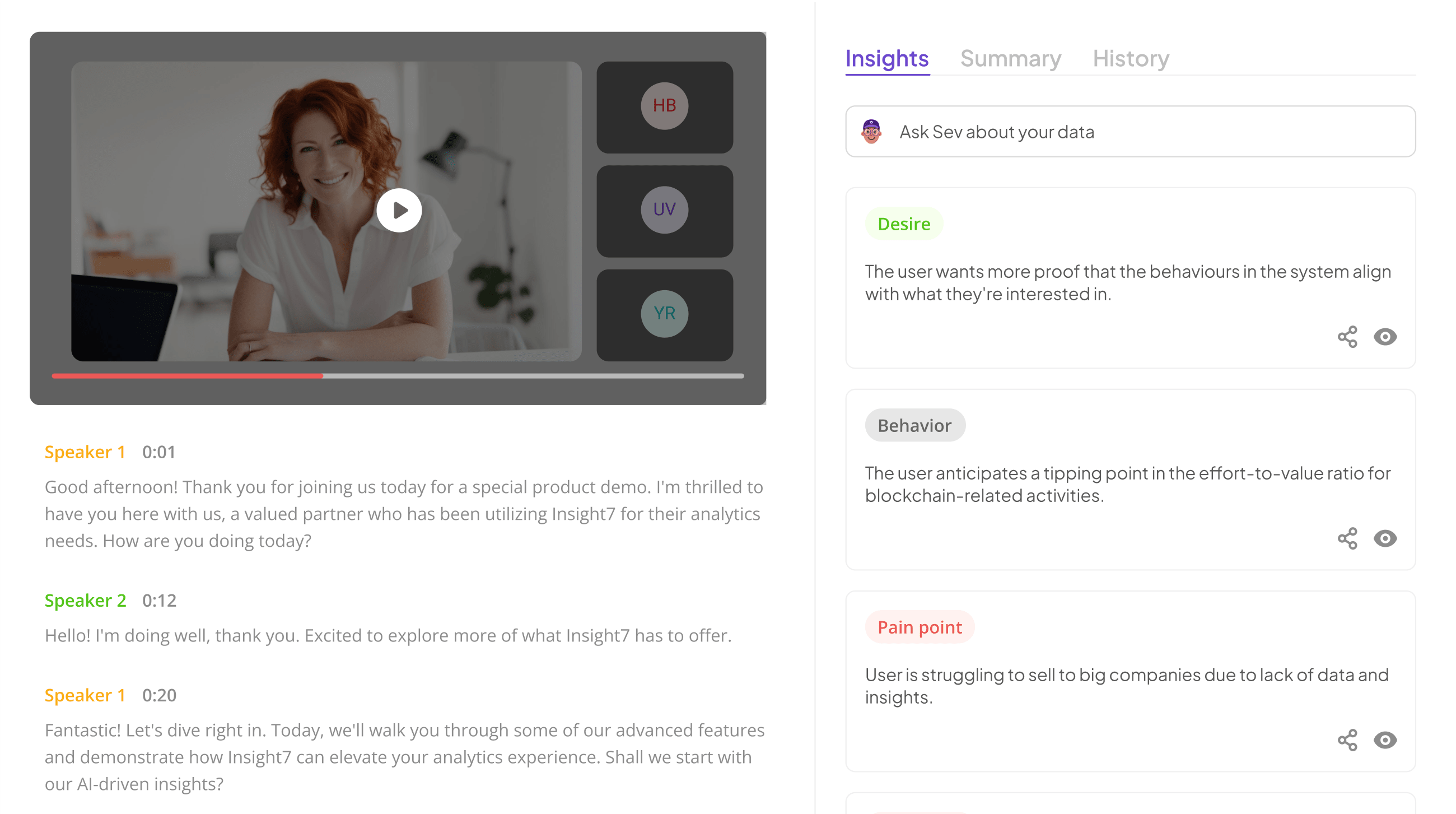
Understanding Longitudinal Qualitative Research
Longitudinal qualitative research involves collecting data from the same subjects over extended periods, allowing for an in-depth understanding of their experiences and perspectives. This method provides rich insights into how attitudes, behaviors, and feelings evolve over time. Researchers often employ interviews, focus groups, and other qualitative techniques to gather this data, which can reveal long-term trends and shifts in participant responses.
However, summarizing this wealth of information presents unique challenges. The extensive data collected can be overwhelming, and traditional analysis methods may be slow and inconsistent. To mitigate these issues, AI-driven qualitative summarization tools can enhance the analysis process, enabling faster and more accurate extraction of themes and key insights. These technological advancements can not only streamline data handling but also contribute significantly to the overall quality and reliability of the research findings. This synergy of AI and qualitative methods paves the way for a deeper understanding of complex human experiences.
The Nature and Purpose of Longitudinal Studies
Longitudinal studies are research approaches that observe subjects over extended periods to identify changes and development. This method is crucial for capturing the complexities of human behavior and experiences, as it allows researchers to collect data from the same participants multiple times. By doing so, the studies can reveal trends and trajectories that provide deeper insights into an individual's or population's evolution.
The purpose of these studies is to understand how variables interact and develop over time. They can inform various fields, including psychology, health, and social sciences. Incorporating AI-driven qualitative summarization into this research can enhance the efficiency and accuracy of data analysis. By automating the summarization process, researchers can focus on interpreting findings rather than spending excessive time on manual data review. This approach streamlines the analysis and offers a more coherent understanding of complex longitudinal data.
The Challenges of Summarizing Long-Duration Qualitative Data
Summarizing long-duration qualitative data presents several challenges that researchers need to navigate carefully. One of the primary hurdles is the sheer volume of data generated over extended periods, which can lead to information overload. AI-driven qualitative summarization tools can assist but require inputs that accurately represent participants' voices and insights. Without careful handling, the richness of data risks being lost or misinterpreted.
Another challenge arises from the evolving context in which long-term studies unfold. Participant responses may shift significantly due to changing circumstances. As a result, maintaining a consistent analytic framework becomes tricky. AI-based solutions can help by enabling real-time updates and adjustments in analysis, making it easier to track trends over time. Ultimately, harnessing AI for qualitative summarization demands a balance between automated efficiency and human interpretation to ensure meaningful insights are achieved.
Extract insights from interviews, calls, surveys and reviews for insights in minutes
AI-driven Qualitative Summarization Tools and Techniques
AI-driven Qualitative Summarization Tools and Techniques enable researchers to efficiently extract meaningful insights from extensive qualitative data. These tools utilize advanced algorithms to analyze transcripts and other qualitative materials, thus reducing human bias and enhancing consistency. By automating the summarization process, researchers can focus on interpreting results rather than being bogged down in manual analysis.
The effectiveness of AI-driven qualitative summarization emerges through several key techniques. Firstly, natural language processing (NLP) identifies patterns and themes within data, providing a coherent overview of findings. Secondly, machine learning algorithms continuously improve their accuracy based on user interactions, creating tailored summaries suited to specific research needs. Finally, visualization tools embedded in these platforms present data insights clearly, fostering better understanding and decision-making. By adopting these AI-driven techniques, qualitative researchers can streamline their workflows and deliver timely insights that meet client demands.
How AI Facilitates Summarization in Longitudinal Research
AI-driven qualitative summarization significantly transforms how researchers handle longitudinal studies. Traditional manual analysis can be labor-intensive and often results in inconsistencies. Researchers frequently sift through extensive interviews and open-ended responses, which can consume valuable time. By applying AI to this process, teams can streamline insights generation, leading to faster reporting without sacrificing quality.
AI facilitates summarization through a few key strategies. Firstly, it automates the identification of recurring themes, enhancing accuracy and reducing cognitive load on researchers. Secondly, algorithms help mitigate bias by providing consistent insights across various team members. Lastly, AI tools enable researchers to track changes over time in qualitative data, offering a richer perspective on evolving trends. These technological advancements not only expedite the summarization process but also enrich the overall research quality, making it a vital resource for anyone conducting longitudinal qualitative research.
Top AI Tools for Qualitative Summarization
Qualitative summarization has been greatly enhanced with the advent of AI-driven tools, streamlining the analysis of longitudinal qualitative research. Researchers often face the challenge of sifting through extensive data collected over time, making traditional methods increasingly inefficient. Fortunately, various AI tools have emerged to address these complexities and provide robust solutions.
One notable benefit of these tools is their ability to identify themes and patterns within large datasets quickly. For instance, software like NVivo and Atlas.ti can assist researchers in coding qualitative data and finding crucial insights more efficiently than manual methods. Other tools, such as MaxQDA and QDA Miner, are tailored to support thematic analysis, making it easier for teams to produce consistent and unbiased reports. By utilizing these AI-driven qualitative summarization tools, researchers can save both time and effort, enabling them to focus on drawing impactful conclusions from their findings.
- Insight7
In the context of AI-driven qualitative summarization, Insight7 plays a pivotal role in transforming how researchers synthesize data from longitudinal studies. This tool streamlines the deeper analysis of qualitative data, allowing users to identify key themes and insights quickly. By leveraging advanced algorithms, Insight7 can effectively analyze interview transcripts and observational data, providing a comprehensive overview that highlights trends and patterns over time.
When utilizing Insight7, researchers can expect several benefits. First, it significantly reduces the time required to sift through extensive qualitative data, enabling quicker decision-making. Second, the AI model continuously learns from new data, enhancing accuracy and relevance in summarization. Finally, the intuitive interface makes it accessible for users with varying levels of technical expertise. Overall, employing AI-driven qualitative summarization not only makes the research process more efficient but also enables richer insights that inform strategic decisions.
- NVivo
NVivo is a prominent tool in the realm of qualitative research, serving as a bridge between traditional analysis methods and innovative technological advancements. Researchers often seek intuitive solutions that efficiently synthesizes vast amounts of longitudinal qualitative data. NVivo enables users to organize, analyze, and visualize their qualitative information more effectively, greatly enhancing data interpretation.
One significant advantage of using NVivo lies in its capacity to integrate AI-driven qualitative summarization techniques. By harnessing machine learning algorithms, NVivo can streamline data coding and identify key themes, simplifying the overall analysis process. This offers researchers valuable insights without the burden of manual intervention.
As NVivo evolves, it plays a crucial role in the partnership between human analysis and AI capability. This helps ensure the accuracy of findings, offering researchers a more robust understanding of data collected over time. Embracing such tools not only aids in summarization but also fosters a deeper engagement with qualitative insights.
- Atlas.ti
Atlas.ti serves as a vital tool in AI-driven qualitative summarization, especially for longitudinal research. This software allows researchers to organize, analyze, and visualize data seamlessly. By using advanced functionalities, users can create analysis kits to address specific themes and questions, thereby providing contextually relevant insights that cater to their research goals.
In utilizing Atlas.ti, researchers can tag and code data efficiently, effectively categorizing findings into themes like challenges or member experiences. This process not only streamlines data management but also enhances interpretation, allowing for sentiment analysis and organized insights. Ultimately, incorporating this tool can significantly reduce the time needed to extract meaningful conclusions, making lengthy qualitative research more manageable and insightful.
- MaxQDA
MaxQDA stands out as a significant player in the realm of AI-driven qualitative summarization. This tool is designed to support researchers by enhancing their ability to manage lengthy qualitative data efficiently. With increasing demands from qualitative research, such as processing numerous interviews, MaxQDA streamlines data organization and analysis, freeing researchers from tedious manual tasks.
One of the key features of MaxQDA is its ability to conduct automatic text analysis, which expedites the summarization of extensive interview transcripts. Researchers can quickly identify themes, trends, and insights without getting bogged down in lengthy texts. Additionally, the tool integrates advanced visualizations that help convey complex data in an understandable manner, making it easier for teams to share findings. By utilizing such innovative software, researchers can enhance their productivity, ultimately leading to richer and more comprehensive insights from longitudinal studies.
- QDA Miner
QDA Miner is a powerful qualitative data analysis software that plays a vital role in facilitating AI-driven qualitative summarization. This tool enables researchers to efficiently manage and analyze large volumes of qualitative data often associated with longitudinal studies. By leveraging advanced algorithms, QDA Miner simplifies the process of extracting meaningful insights from extensive transcripts, interview notes, and observational data.
One of the standout features of QDA Miner is its ability to automate initial coding of qualitative data, which significantly reduces the manual effort typically required. This is particularly beneficial for researchers managing multiple interviews or focus groups, allowing them to focus on deeper analysis and interpretation. Additionally, its user-friendly interface ensures that researchers, regardless of technical proficiency, can utilize its capabilities effectively. Overall, QDA Miner exemplifies how AI-driven qualitative summarization can enhance the efficiency and effectiveness of longitudinal qualitative research.
Conclusion: Embracing AI-driven Qualitative Summarization for Better Insights
Embracing AI-driven Qualitative Summarization is a transformative step toward enhancing insights in qualitative research. As the demand for quicker and more accurate reporting grows, traditional methods often fall short, leading to inefficiencies and biases. By integrating AI into the qualitative analysis process, researchers can streamline data interpretation and elevate the consistency of insights derived from multiple interviews or focus groups.
The advantages of this technology are clear. AI-driven tools can rapidly analyze vast amounts of qualitative data, revealing themes and patterns that may go unnoticed in manual reviews. This allows researchers to focus more on strategic decision-making rather than tedious data processing. Ultimately, leveraging AI-driven Qualitative Summarization not only enriches research outcomes but also empowers teams to deliver valuable insights at an unprecedented pace.