How to Use AI to Extract Context-Specific Insights from Interviews
-
Hello Insight
- 10 min read
Understanding the intricacies of interviews can be challenging, especially when seeking deep insights that inform decision-making. In today's fast-paced environment, the need for Contextual Interview Insights has never been more pressing. AI offers innovative solutions for extracting rich, meaningful data from interviews, providing researchers with tools to streamline their processes and enhance accuracy.
By harnessing AI, businesses can transition from manual, time-consuming analysis methods to real-time extraction of critical themes and insights. This shift not only speeds up the reporting process but also minimizes bias and improves consistency across teams. As we explore how to effectively utilize AI, we will uncover the transformative potential it holds for gaining Contextual Interview Insights.
Analyze & Evaluate Calls. At Scale.
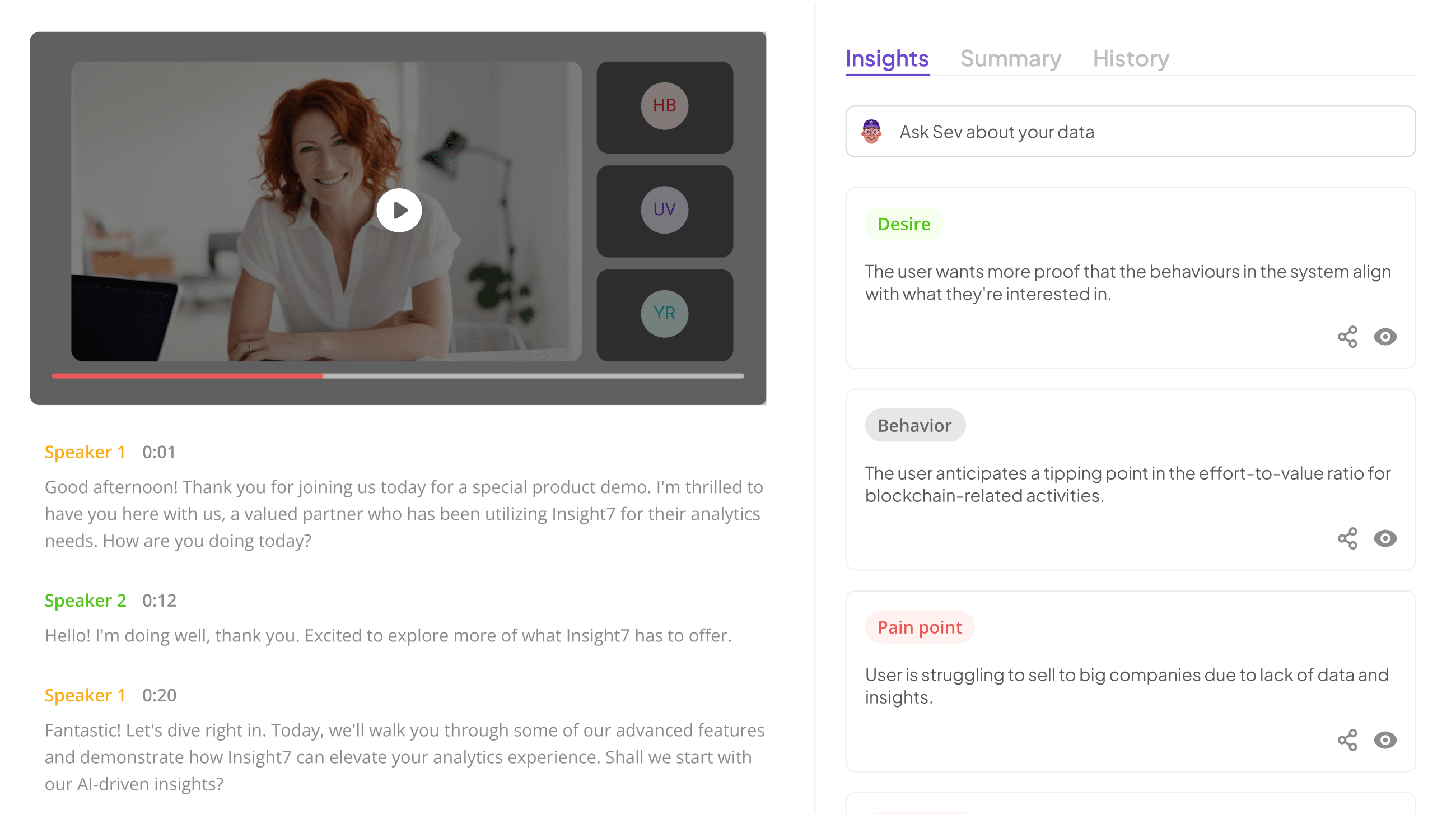
Understanding Contextual Interview Insights through AI
Understanding contextual interview insights through AI has become essential for organizations looking to maximize efficiencies and enhance accuracy. By employing AI algorithms, teams can analyze verbal cues and emotional context that traditional methods might overlook. This not only streamlines the process of data collection but also reduces biases that arise from individual interpretations. AI tools can quickly sift through massive amounts of data, delivering themes and insights that enable researchers to focus on strategic actions.
Moreover, contextual interview insights are enriched when AI systems are trained to recognize the nuances of language and expression. For instance, recognizing sentiment can help researchers understand the underlying emotions tied to particular responses. This depth allows for more informed decision-making and powerful narrative development, providing a competitive advantage in various industries. Ultimately, utilizing AI for contextual interview insights transforms qualitative data into actionable strategies, paving the way for more effective research practices.
The Role of AI in Contextual Analysis
AI plays a pivotal role in contextual analysis, enhancing our ability to derive actionable insights from interviews. Through advanced data processing, AI tools can analyze transcripts quickly and efficiently, identifying key themes and sentiments which might otherwise be overlooked. By harnessing AI, researchers can transform raw interview data into structured, context-specific insights that inform decision-making.
Moreover, AI algorithms empower researchers to cluster data from multiple interviews, revealing broader trends and patterns across diverse perspectives. This enables a more nuanced understanding of interviewee experiences and opinions. By adopting these AI-driven methods, organizations can streamline their analysis process, reduce human bias, and significantly enhance the quality of their contextual interview insights. Ultimately, the integration of AI into contextual analysis fosters a more profound understanding of clients' needs and challenges, allowing organizations to respond with greater precision and agility.
Importance of Context in Interviews
Understanding the importance of context in interviews shapes the way insights are gathered and interpreted. Contextual Interview Insights go beyond simply recording responses; they delve into the surrounding circumstances that influence participants' thoughts. Factors such as environment, emotional state, and prior experiences can significantly shape an interviewee’s answers, providing richer and more nuanced data.
Recognizing these contextual elements allows for more robust analysis and actionable results. For instance, an interview conducted in a relaxed setting may elicit different responses compared to a formal atmosphere. Identifying these nuances leads to deeper understanding and can drastically enhance the relevance of findings. By leveraging AI tools, analysts can uncover these subtle yet impactful contexts, ensuring a comprehensive insight extraction process that is aligned with the true sentiments of interviewees. Therefore, integrating context-aware strategies into interviews is crucial for producing valuable insights that drive informed decision-making.
Extract insights from interviews, calls, surveys and reviews for insights in minutes
Steps to Extract Contextual Interview Insights Using AI
To extract contextual interview insights using AI, it's vital to follow a systematic approach. Start by collecting high-quality data from your interviews. Ensure that your questions are open-ended and cover a range of topics relevant to your research objectives. Recording interviews and transcribing them accurately will provide a solid foundation for insight generation. This step is crucial, as the richness of the data will significantly influence the complexity of the insights you can derive.
Next, apply AI algorithms tailored for contextual analysis. Utilize natural language processing (NLP) tools that can identify themes, emotions, and sentiments within the interview data. These technologies can analyze vast amounts of text quickly and efficiently, highlighting key trends that might go unnoticed with manual analysis. Finally, establish feedback loops to refine these insights. Consistently seek input from researchers and stakeholders, ensuring that the insights generated are actionable and aligned with your project goals. By following these steps meticulously, you can unlock profound contextual interview insights that drive informed decision-making.
Step 1: Key Data Collection Techniques
To effectively gather Contextual Interview Insights, it's crucial to implement robust data collection techniques. Start by designing your interviews with open-ended questions that encourage participants to share detailed experiences. This invites richer narratives and enables you to capture nuanced perspectives essential for contextual understanding. Additionally, employing recording tools allows you to revisit conversations, ensuring you capture all critical elements without missing any subtle cues.
Incorporating thematic analysis is also vital. By breaking down collected data into categories, you can identify recurring themes, sentiments, and patterns. This will assist in structuring the insights in a way that aligns with your research goals. Lastly, consider using mind maps or analysis kits to visualize and organize your findings dynamically. This approach not only enhances clarity but also makes the analysis more accessible. Utilizing these techniques collectively ensures that your resulting insights are deeply contextual and relevant, driving better decision-making.
Step 2: Applying AI Algorithms for Contextual Analysis
When applying AI algorithms for contextual analysis, the goal is to unveil deeper layers of meaning from interviews. This process involves transforming raw data into nuanced insights that align with specific contexts. AI analyzes patterns within the conversations, identifying themes and sentiments that may not be immediately apparent to human analysts. By leveraging natural language processing (NLP) techniques, it can efficiently process vast amounts of data, allowing teams to focus on strategic decision-making rather than repetitive analysis.
To implement this effectively, it's vital to follow a structured approach. First, define the contextual parameters most relevant to your research objectives, ensuring clarity in what insights you seek. Second, utilize AI tools capable of sentiment analysis and topic modeling, which can offer a comprehensive view of interview responses. Finally, continuously validate findings through iterative feedback loops with stakeholders, enhancing the quality and relevance of the insights. This method not only streamlines the analysis process but also significantly enriches your Contextual Interview Insights.
Step 3: Refining Insights with Feedback Loops
Refining insights derived from interviews is an essential step in ensuring your findings are accurate and actionable. Feedback loops allow you to gather perspectives from different stakeholders, validating and enhancing the Contextual Interview Insights obtained. This process not only helps confirm your interpretations but also reveals potential gaps that may require further exploration.
Implementing effective feedback loops involves a structured approach. First, share preliminary insights with your team or relevant stakeholders to collect their reactions. Following their input, adjust your analysis by incorporating their views and suggestions. Finally, revisit the data, refining your initial hypotheses with the new information gathered. This iterative process fosters a more comprehensive understanding and maximizes the relevance of your insights, ultimately leading to better decision-making. Embrace these loops as a means to evolve your findings continually and align them closely with the context-specific needs of your audience.
💬 Questions about How to Use AI to Extract Context-Specific Insights from Interviews?
Our team typically responds within minutes
Choosing the Right Tools for Extracting Contextual Insights
Selecting appropriate tools for extracting contextual interview insights is critical for ensuring the process is effective and efficient. The right tools allow researchers to analyze vast amounts of interview data, identifying patterns and themes that may otherwise remain hidden. These tools help organize the insights, filter information based on various parameters, and generate comprehensive reports that encapsulate the essential findings.
When choosing tools, consider factors such as ease of use, adaptability to different types of data, and the ability to filter information effectively. For instance, advanced NLP tools can elevate the extraction process by interpreting nuances in spoken language, while reporting features allow users to compile findings effortlessly. Additionally, it’s vital to prioritize tools that provide transparency about the algorithms used, ensuring the reliability of the extracted insights. Investing in the right technology not only enhances data analysis but also streamlines the journey towards gaining valuable contextual interview insights.
Insight7: Leading AI Tool for Contextual Interview Insights
Incorporating AI into the realm of interviews revolutionizes our approach to deriving insights. The Insight7 tool stands out as a leading AI solution specifically designed for gathering contextual interview insights. This innovative application streamlines the analysis process, transforming vast amounts of qualitative data into meaningful, actionable insights. Users benefit from features that allow for detailed tracking of findings, enabling businesses to see progress transparently and efficiently.
With AI at the forefront, Insight7 employs sophisticated algorithms to identify key themes and patterns. This approach not only enhances the accuracy of insights but significantly reduces the time spent on data analysis. As teams harness this tool, they can expect a more organized workflow and consistent outputs, ultimately aiding strategic decision-making. By prioritizing context, Insight7 ensures that organizations can effectively interpret interview data and drive impactful changes within their operations.
Other Essential AI Tools
When extracting contextual interview insights, choosing the right AI tools is crucial. Various platforms can enhance the analysis and interpretation of interview data, making it easier to derive meaningful insights. Each tool offers unique capabilities that cater to different aspects of data processing, ensuring you can align the analysis with specific research goals.
Key tools include MonkeyLearn, known for its versatility in text analysis and easy-to-use interface, perfect for beginners. IBM Watson Natural Language Understanding excels in sentiment analysis and categorization, providing comprehensive insights into emotional tones. Lexalytics focuses on deep semantic analysis, revealing complex relationships within the data. Meanwhile, Google Cloud Natural Language offers powerful entity recognition capabilities, enhancing your ability to identify key themes and topics from interviews. By leveraging these essential AI tools, analysts can significantly improve their ability to extract meaningful insights from interviews, ultimately driving better decision-making.
MonkeyLearn
In the quest for Contextual Interview Insights, utilizing advanced AI platforms can significantly enhance the analysis process. One notable tool streamlines data organization and insight extraction by simplifying the workflow for users. Its intuitive structure allows for seamless input of various data forms, whether through direct text entry or by uploading video interviews for transcription. Once the data is in, the real magic unfolds as users engage with the project's features to analyze, cluster, and extract relevant insights effortlessly.
To maximize your output, this platform comes equipped with predefined kits tailored for specific fields, allowing for quick initiation of analysis projects. Whether you're unpacking marketing strategies or evaluating customer feedback, these kits jumpstart your efforts by automatically generating key insights. The collaborative nature of the platform fosters a productive environment for teams, making it easy to consolidate and share findings. By effectively leveraging this powerful tool, you can discover vital Contextual Interview Insights that drive informed decision-making.
IBM Watson Natural Language Understanding
Understanding how to extract contextual interview insights is greatly enhanced by utilizing advanced natural language processing technologies. One such tool excels in analyzing text data to identify themes, sentiments, and relationships. This technology not only processes large volumes of interview data but also provides a nuanced understanding of the context behind interviewee responses.
To effectively leverage this tool, you must first input the interview transcripts and specify the data points you're interested in uncovering. The technology then analyzes the content, focusing on aspects like sentiment and key themes that emerge from participants' responses. The insights derived from this analysis can help identify trends, highlight important topics, and offer a deeper understanding of the subject matter. By tapping into contextual interview insights, businesses can make informed decisions and refine their strategies based on solid evidence and comprehensive data analyses.
Lexalytics
Lexalytics provides a sophisticated platform for extracting Contextual Interview Insights, allowing users to efficiently analyze qualitative data. The process begins with transcription, where audio or video interviews are converted into text, making it easier to work with the information. This initial step ensures that all nuances of the conversation are captured, forming a solid foundation for subsequent analysis.
Once transcribed, users can delve deeper into the data by identifying themes and extracting quotes that resonate with specific contexts. This analysis can be both individual and collective, enabling researchers to uncover broader trends and patterns. Moreover, the platform prioritizes data privacy and compliance, ensuring that sensitive information remains secure. By leveraging AI's capabilities, users can transform raw interview data into actionable insights, ultimately leading to more informed decisions and strategies in their respective fields.
Google Cloud Natural Language
The Google Cloud Natural Language tool captures and analyzes textual data, providing insights that help organizations make informed decisions. By processing the language used in interviews, it reveals sentiments, themes, and entities present in the responses. This capability is especially beneficial for extracting contextual interview insights that might not be immediately evident.
To utilize Google Cloud Natural Language effectively, first, upload your transcripts or recorded dialogues. The tool then annotates the text, offering a structured way to identify patterns and key sentiments. Secondly, it enhances the analysis by employing custom vocabulary to ensure relevant terminology is accurately interpreted. Finally, the visualization features allow for easy reporting and sharing of insights with your team, transforming raw data into actionable knowledge. By integrating this tool into your interview analysis process, you can improve the quality and relevance of your gleaned insights.
Conclusion: Maximizing Contextual Interview Insights with AI
Maximizing Contextual Interview Insights with AI involves a strategic blend of technology and human intuition. By integrating AI tools into the interview analysis process, you can significantly reduce time spent on manual data extraction. This efficiency enables teams to quickly identify patterns and trends that might otherwise go unnoticed, enhancing the overall richness of insights gathered.
Additionally, employing AI allows for greater consistency in themes and analyses across different researchers. A unified approach not only mitigates bias but also streamlines the delivery of actionable insights. As industries continue to demand rapid feedback and dynamic reporting, harnessing AI in this context will only grow in importance. Embracing these advancements equips your team to navigate the complexities of interview analysis more effectively.
Analyze & Evaluate Calls. At Scale.
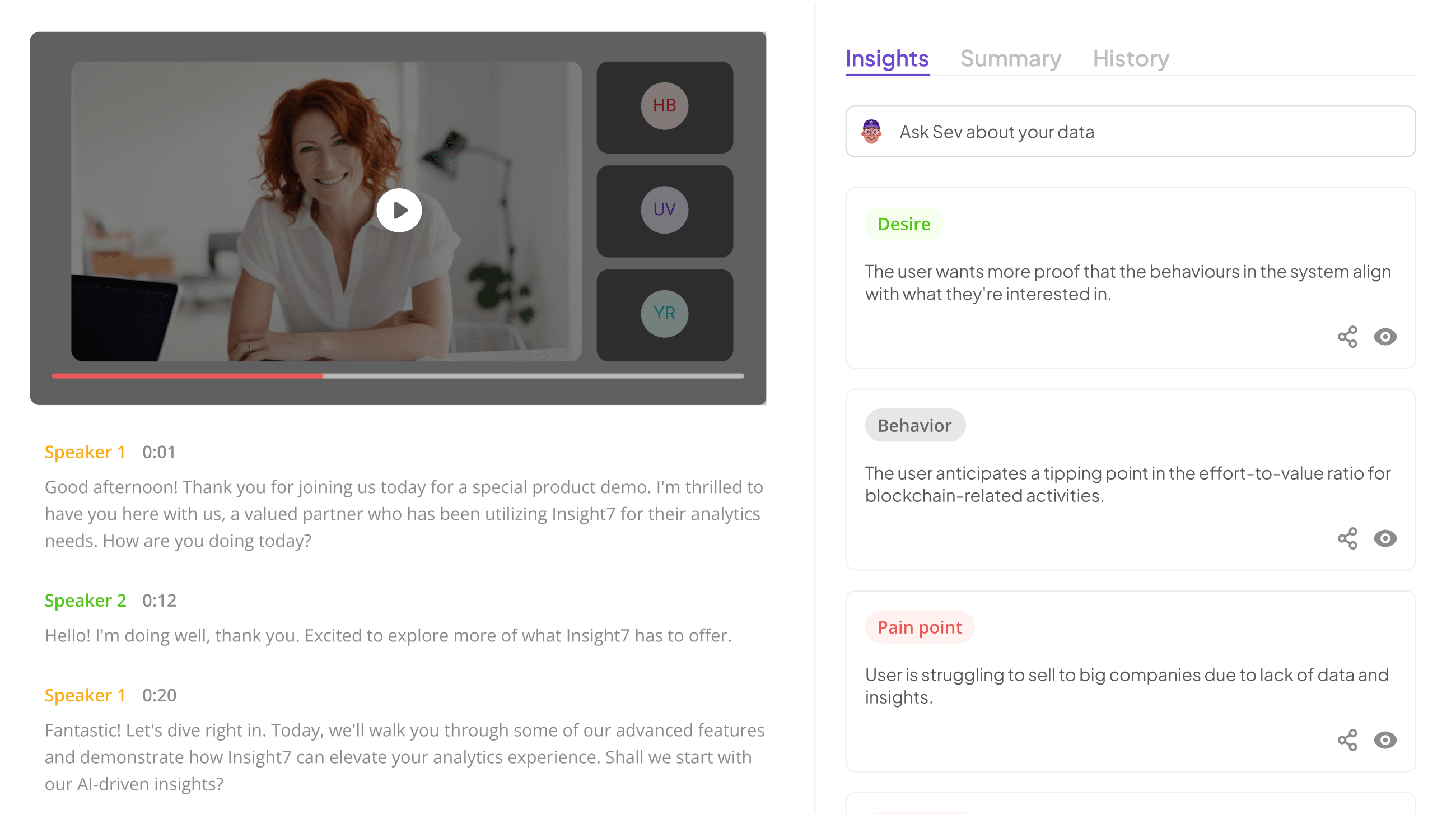
💬 Questions about How to Use AI to Extract Context-Specific Insights from Interviews?
Our team typically responds within minutes