How to Use AI to Categorize Survey Feedback by Intent
-
Hello Insight
- 10 min read
In today's fast-paced environment, organizations continuously seek effective ways to analyze their feedback. AI Intent Categorization emerges as a vital solution, enabling companies to understand and react to customer sentiments swiftly. By sifting through vast amounts of survey data, AI distinguishes intentions behind responses, offering insights that are both timely and actionable.
The process of categorizing survey feedback by intent not only enhances organizational decision-making but also deepens customer relationships. As businesses harness the power of AI, they can convert raw data into meaningful narratives. This transformation ultimately leads to improved products and services that resonate with their audience's needs and desires.
Analyze & Evaluate Calls. At Scale.
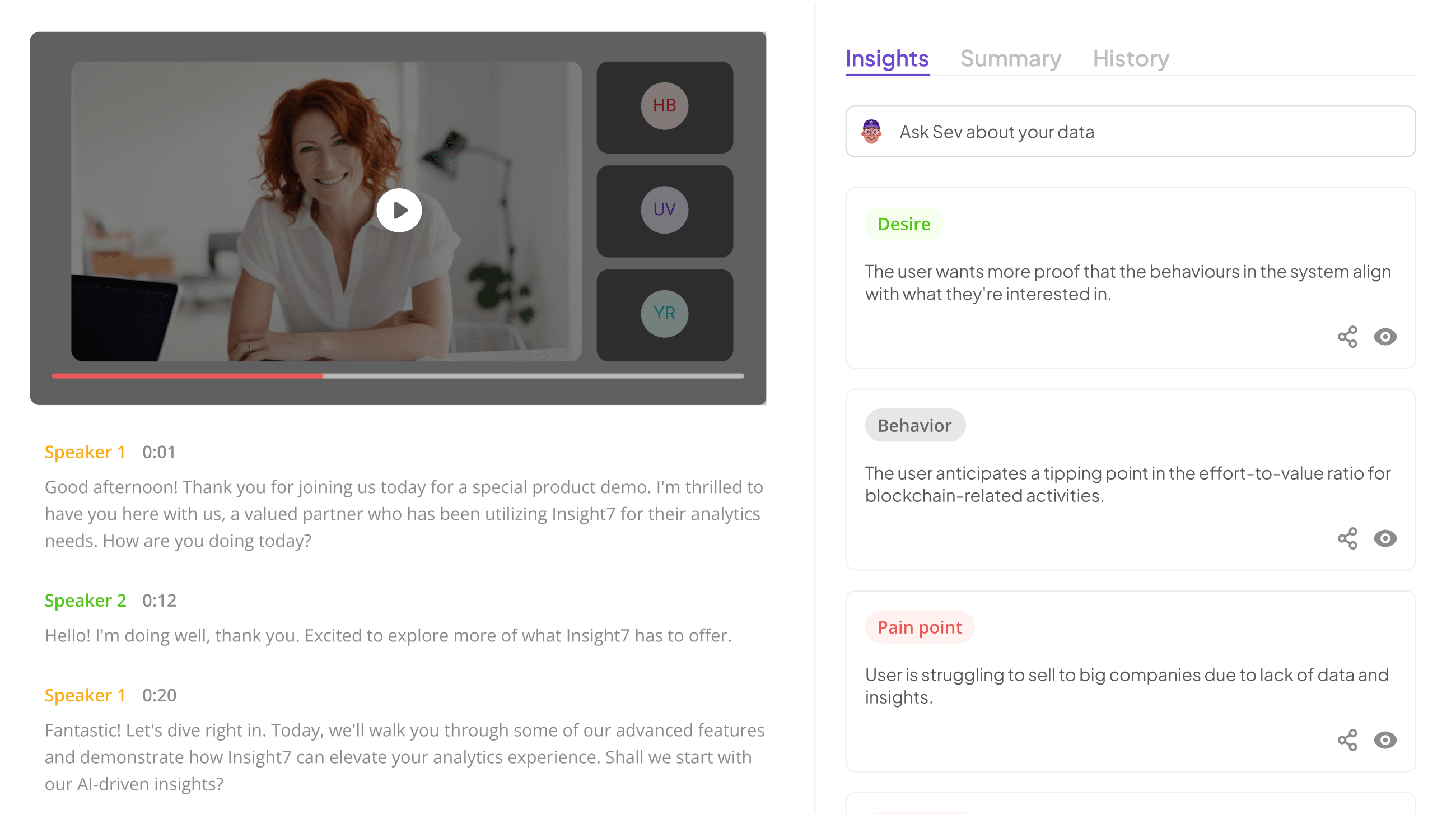
Understanding AI Intent Categorization
AI Intent Categorization serves as a transformative approach in analyzing survey feedback effectively. By understanding how AI categorizes intent, organizations can gain valuable insights into customer sentiments and preferences. This process involves training machine learning algorithms to identify underlying themes in survey responses, which enables better segmentation of feedback based on emotional context and intent.
To delve deeper, it’s essential to comprehend the steps involved in AI Intent Categorization. First, data preparation is crucial; the input data must be structured and cleaned to ensure accuracy. Next, selecting appropriate AI tools designed for intent detection is vital, as different platforms offer varying features. After choosing the right tools, organizations can train and test their AI models to refine categorization accuracy continually. Lastly, ongoing monitoring ensures that the system adapts to shifting trends, maintaining its relevance in delivering actionable insights from the collected feedback. This dynamic approach leads not only to improved customer understanding but also enhances overall decision-making processes.
The Role of AI in Intent Determination
AI Intent Categorization plays a crucial role in understanding and interpreting survey feedback. By analyzing the emotions, needs, and motivations behind responses, AI algorithms can categorize feedback effectively. This helps organizations pinpoint areas for improvement and identify customer pain points, leading to better service offerings.
To achieve accurate intent determination, AI leverages various techniques, such as natural language processing and machine learning. These technologies help process large volumes of feedback quickly, allowing for real-time insights. Organizations can then tailor their strategies based on categorized intents, enhancing their responses to customer needs. Furthermore, AI models continually learn from new data, ensuring that intent categorization remains relevant as customer preferences evolve. The integration of AI in feedback categorization not only boosts efficiency but also enriches the overall customer experience, laying the foundation for informed decision-making.
Benefits of Categorizing Feedback by Intent
Categorizing feedback by intent is vital for transforming data into actionable insights. By employing AI intent categorization, organizations can quickly identify trends and themes within feedback. This clarity helps improve decision-making processes. Understanding the underlying intent behind responses can reveal customer sentiment, preferences, and areas needing attention.
The benefits are manifold. Firstly, it enhances efficiency, allowing teams to focus on strategic initiatives rather than sifting through countless responses. Secondly, AI intent categorization provides personalized insights, tailoring services or products to specific customer needs. Lastly, it fosters a proactive approach, enabling prompt responses to challenges, thereby enhancing customer satisfaction. Embracing these advantages not only streamlines operations but also empowers businesses to create more meaningful interactions with their clients.
Extract insights from interviews, calls, surveys and reviews for insights in minutes
Steps to Implement AI for Intent Categorization in Surveys
To effectively implement AI for intent categorization in surveys, begin with comprehensive data collection and preparation. Gather qualitative feedback from various sources, ensuring a diverse range of responses. Clean and preprocess the data to eliminate noise and enhance quality, as this significantly impacts accuracy in AI intent categorization.
Next, select the appropriate AI tools tailored for intent analysis. Options like MonkeyLearn or Thematic offer specialized capabilities for processing open-ended survey responses. Once a suitable tool is chosen, invest time in training and testing your AI models. This iterative process helps refine categorization, ensuring the model correctly interprets different intents. Finally, establish a system for continuous monitoring and improvement. Regularly assess the performance of your models to adapt and optimize their functionality for evolving survey needs. Following these structured steps will streamline your survey analysis and boost the insights derived from categorizing feedback by intent.
Step 1: Data Collection and Preparation
Effective data collection and preparation are crucial for AI intent categorization. The first step involves identifying the sources of your survey feedback. This can include online forms, email responses, and social media comments. Before processing these responses with AI, it’s essential to organize the data systematically. Cleanliness and structure in your data allow for more accurate insights later on.
Once collected, prepare the data for analysis by removing any irrelevant or duplicate entries. Categorizing responses by the type of feedback—such as positive, negative, or neutral—can enhance understanding. Additionally, consider enriching your dataset with supplementary information, such as demographic details or context surrounding the feedback. This groundwork lays the foundation for effective AI intent categorization, ultimately leading to more precise and actionable insights derived from the feedback collected.
Step 2: Choosing the Right AI Tools for Intent Categorization
Choosing the right AI tools for intent categorization is crucial in ensuring accurate analysis of survey feedback. With many options available, it's beneficial to evaluate tools based on their features, ease of use, and compatibility with your existing systems. Key functionalities to consider include natural language processing capabilities, customization options, and analytics support. Selecting a tool that aligns with your specific needs enhances the categorization process and improves insights gained from survey responses.
Furthermore, reviewing user experiences and outcomes can guide your decision-making. Tools like Insight7, MonkeyLearn, Thematic, and RapidMiner each offer unique advantages. Insight7 specializes in customer feedback analysis, while MonkeyLearn allows for customizable text analysis. Thematic excels in deriving themes from open-ended responses, and RapidMiner provides a robust platform for data mining. By carefully considering these factors, you can choose the right AI tool to optimize your intent categorization strategy effectively.
Insight7
AI Intent Categorization enhances our ability to understand and analyze survey feedback effectively. By leveraging advanced algorithms, AI systems can scan and sort responses into distinct intent categories, such as complaints or suggestions. This categorization process allows businesses to identify trends rapidly, pinpointing areas requiring attention or improvement.
To implement AI Intent Categorization successfully, it is essential to follow key steps. First, data collection and preparation are critical, ensuring that the input data is clean and relevant. Next, selecting appropriate AI tools specifically designed for intent categorization will streamline the process. Tools like MonkeyLearn and Thematic provide robust options tailored for such tasks. Afterward, training and testing AI models are crucial for achieving accurate categorization results. Finally, continuous monitoring ensures that the system adapts and improves over time, ultimately leading to more insightful analysis of survey feedback.
MonkeyLearn
In the realm of AI Intent Categorization, one of the most effective tools available stands out for its intuitive design and powerful capabilities. Users can easily navigate through its interface to manage projects and analyze data. Within the platform, users can aggregate various formats of survey feedback and insights, enabling a comprehensive overview of responses. This seamless integration encourages collaboration, allowing team members to share and refine data collectively.
Notably, the platform offers specialized tools and kits tailored for specific analysis needs. These kits expedite the insight-gathering process by streamlining tasks, making it easier to categorize feedback by intent. By harnessing this technology, organizations can quickly identify patterns in user responses, enhancing their decision-making process. Ultimately, leveraging such a resource fosters a data-driven approach, ensuring that survey feedback analysis yields actionable insights for continuous improvement.
Thematic
Thematic analysis plays a crucial role in the process of AI intent categorization. By identifying and organizing recurring themes within survey feedback, this method helps uncover customers’ underlying sentiments and intentions. Understanding these patterns allows organizations to respond more effectively to needs and enhance overall engagement.
To implement thematic analysis effectively, follow these steps:
- Identify Key Themes: Start by reviewing your survey data to pinpoint common comments or sentiments.
- Group Similar Feedback: Cluster similar responses together under each identified theme. This creates a clearer picture of customer intent.
- Link Insights to Business Goals: Ensure that each theme relates to your project objectives, whether it’s increasing sales or driving engagement.
- Refine and Iterate: Continuously assess and refine your themes as feedback evolves. This allows for proactive adjustments in strategy based on real-time data insights.
These steps facilitate a structured approach to understanding customer feedback through thematic categorization, enriching the overall analysis and decision-making process.
RapidMiner
RapidMiner stands out as a powerful tool for AI intent categorization, streamlining how organizations process and analyze survey feedback. By using advanced analytics and machine learning, it helps users identify the underlying intents within large datasets. This speed and accuracy significantly reduce the manual effort required for categorizing feedback, allowing teams to focus on deeper insights and actionable strategies.
To effectively use RapidMiner for AI intent categorization, consider the following steps. First, prepare your data comprehensively by cleaning and organizing the feedback for processing. Then, utilize RapidMiner’s intuitive interface to configure your model, selecting appropriate algorithms for intent analysis. Next, validate your outcomes by testing the model's performance against historical data. Finally, continually monitor and refine the model to adapt to new trends in customer feedback. This structured approach ensures that you extract meaningful insights that align with the objectives of your organization.
Step 3: Training and Testing AI Models for Accurate Categorization
To effectively conduct AI Intent Categorization, training and testing AI models is essential for achieving accurate feedback categorization. This phase involves using curated datasets that showcase various intents derived from survey responses. By employing these datasets, you teach the AI system to recognize patterns and associate specific responses with their corresponding intents. Proper training enhances model accuracy, ensuring that your AI can consistently classify new feedback correctly.
Testing follows training and is equally crucial. It evaluates the model's performance by measuring its ability to categorize unseen data accurately. By analyzing test results, you can identify areas for improvement, fine-tune model parameters, or even adjust the training dataset. A well-trained and tested AI model ultimately leads to more reliable insights from survey feedback, enabling better decision-making for your projects. Regularly revisiting this process enhances the model’s capability to adapt to evolving language and intent patterns.
Step 4: Continuous Improvement and Monitoring
To ensure effective AI Intent Categorization, continuous improvement and monitoring are essential. This step focuses on regularly evaluating the performance and accuracy of the AI models used for categorizing survey feedback. Consistent assessment enables identification of areas for enhancement, ensuring the system remains aligned with evolving user intents and changing feedback patterns.
A proactive approach involves implementing a feedback loop where results are analyzed, and insights are used to refine the AI algorithms. Monitoring key performance metrics, such as accuracy and response times, holds significant importance in this process. Making adjustments based on these insights may involve retraining the models or fine-tuning the categorization criteria. By nurturing a culture of ongoing improvement, organizations can maintain high standards in AI Intent Categorization, thereby maximizing the relevance and value of their survey analysis.
Conclusion: Leveraging AI Intent Categorization for Improved Survey Feedback Analysis
In conclusion, AI Intent Categorization serves as a vital tool for enhancing survey feedback analysis. By systematically categorizing responses based on intent, organizations can streamline their data interpretation processes. This method not only saves time but also reduces bias, ensuring a more consistent analysis across diverse teams.
Furthermore, adopting AI for intent categorization provides a clearer understanding of customer sentiments. This clarity allows businesses to tailor their strategies effectively and respond promptly to market demands. Overall, the integration of AI technology empowers organizations to transform raw feedback into actionable insights, fostering smarter decision-making for future initiatives.