Predictive Planning AI is transforming the way organizations approach service level management. As businesses face an overwhelming volume of customer data, traditional methods of analysis often fall short, leading to missed opportunities. This AI-driven approach provides the ability to anticipate service demands and optimize resource allocation, ultimately enhancing customer satisfaction.
Incorporating Predictive Planning AI enables teams to move from reactive to proactive service management. By harnessing real-time data insights, organizations can identify potential issues before they escalate, thus ensuring that service levels meet or exceed expectations. Embracing this technology can streamline processes and foster an agile response to customer needs, paving the way for improved operational efficiency.
Analyze qualitative data. At Scale.
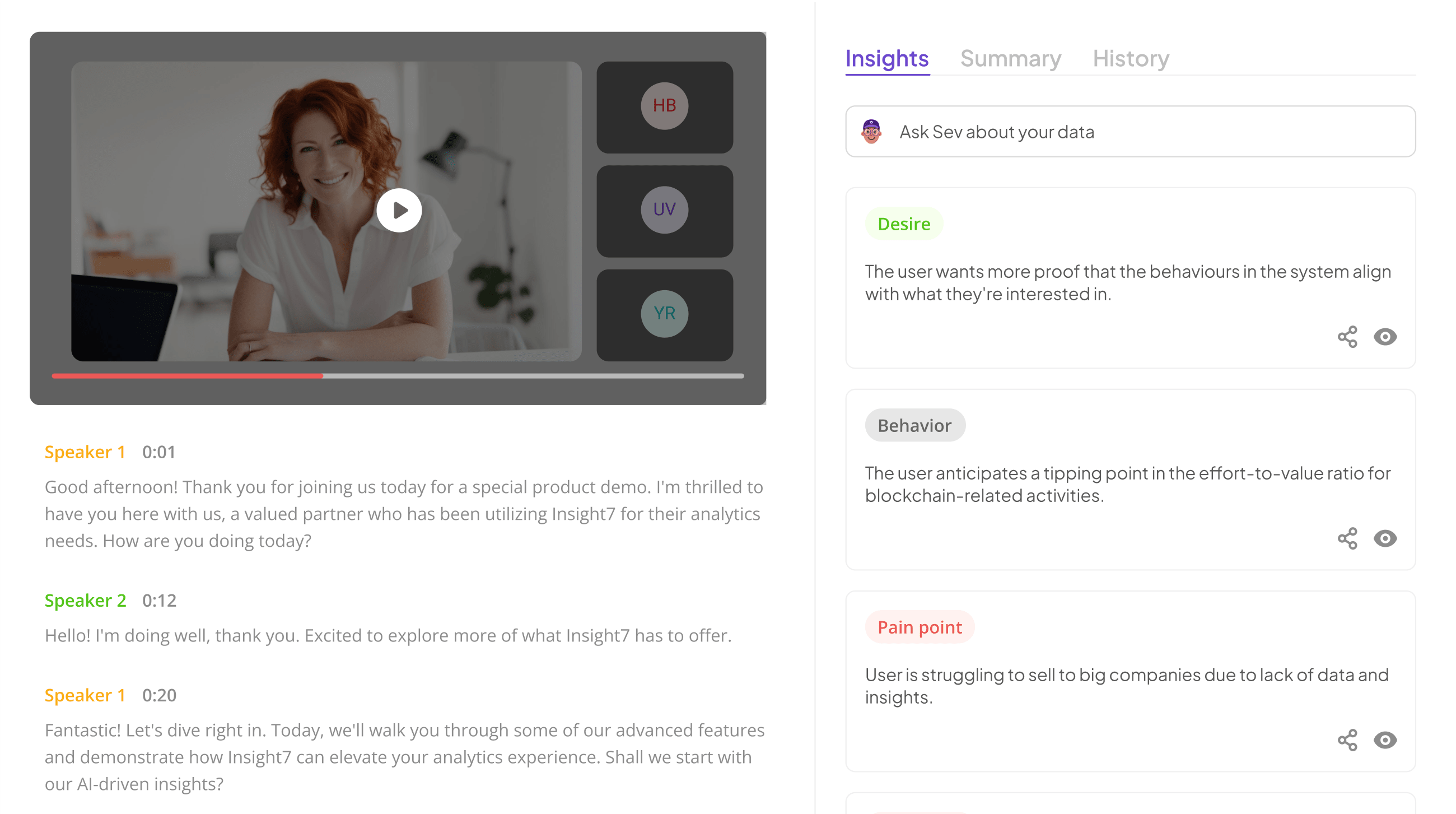
Exploring AIs Role in Predictive Planning for Service Levels
Artificial Intelligence (AI) transforms the landscape of predictive planning for service levels in significant ways. Predictive Planning AI analyzes vast data sets to identify patterns and trends, which helps organizations anticipate customer needs and optimize resources effectively. With AI tools at their disposal, businesses can integrate predictive analytics into their service management strategies, enhancing overall efficiency and service quality.
First, AI empowers organizations to preemptively address potential service disruptions. By identifying patterns in historical data, companies can predict peaks in demand and allocate resources accordingly. Next, AI-driven insights enable organizations to quantify service level expectations, ensuring they meet or exceed customer satisfaction consistently. Furthermore, implementing AI tools requires structured processes, including meticulous data collection and model training. By committing to a systematic adoption of Predictive Planning AI, businesses can improve decision-making and achieve higher service levels, ultimately driving customer loyalty.
Understanding Predictive Planning AI Fundamentals
Predictive Planning AI involves using advanced algorithms and data analysis techniques to anticipate future service levels. This AI-powered approach allows organizations to assess their needs based on historical data, current trends, and various influencing factors. Understanding how this technology functions is crucial to maximizing its benefits and improving decision-making processes.
The core of Predictive Planning AI lies in its ability to collect, analyze, and interpret data effectively. Organizations can use this information to predict demand fluctuations, optimize resource allocations, and identify potential service interruptions before they impact customers. By embracing Predictive Planning AI, companies can enhance their service delivery, improve customer satisfaction, and ultimately drive growth. Key elements of this technology include data integration, model training, and continuous monitoring to refine predictions over time. Ultimately, understanding these fundamentals equips organizations to make informed decisions and improve operational efficiencies in service level management.
- Defining Predictive Planning AI
Predictive Planning AI involves using artificial intelligence to forecast and optimize service levels efficiently. This technology harnesses data analytics and machine learning to anticipate future service demands, allowing businesses to allocate resources more effectively. By analyzing historical data and recognizing patterns, Predictive Planning AI enables decision-makers to create informed, strategic plans to enhance service delivery.
Implementing Predictive Planning AI can significantly enhance operational efficiency. It allows organizations to identify potential challenges before they arise, avoiding disruptions and improving customer satisfaction. Moreover, businesses can fine-tune their service offerings based on anticipated trends, ensuring they stay competitive in a dynamic market. Overall, the integration of Predictive Planning AI into service level management empowers companies to respond proactively and adapt to changing demands.
- Importance of AI in Service Level Management
In the realm of service level management, the importance of AI cannot be overstated. Predictive Planning AI empowers organizations to anticipate customer needs and optimize service delivery. Traditional methods struggle to keep pace with the vast data generated from customer interactions. By harnessing AI, businesses can analyze this data effectively, providing timely insights that enhance decision-making.
The integration of Predictive Planning AI offers several key benefits. Firstly, it significantly reduces response times by quickly identifying patterns in customer requests. Secondly, it improves accuracy in service level expectations, enabling more effective resource allocation. Lastly, AI fosters continuous improvement by learning from past interactions, adapting strategies accordingly. By adopting these technologies, organizations can elevate their service level management, staying ahead of competitors and fostering customer loyalty.
In today's fast-paced market, leveraging AI is essential for businesses seeking optimal performance and customer satisfaction.
Applications of Predictive Planning AI in Service Levels
Predictive Planning AI plays a pivotal role in enhancing service levels through its ability to analyze vast datasets and generate actionable insights. One of its primary applications is in enhancing service efficiency. By utilizing advanced algorithms, organizations can anticipate customer needs and streamline operations, ultimately reducing wait times and improving satisfaction. This predictive capability not only empowers teams to allocate resources effectively but also ensures that service delivery aligns with customer expectations.
Another significant application is proactive issue detection and resolution. Predictive Planning AI enables organizations to identify potential service disruptions before they affect customers. With real-time monitoring and predictive analytics, companies can address issues swiftly, minimizing downtime and enhancing overall service quality. By combining these applications, businesses can foster a more responsive and customer-centric service environment, ultimately driving retention and loyalty. Such strategic use of AI transforms the service planning landscape, positioning organizations for future success.
- Enhancing Service Efficiency
In service efficiency, the focus is on ensuring that resources are maximally utilized to meet customer needs. Predictive Planning AI streamlines processes by analyzing data trends and patterns. This advanced technology allows businesses to anticipate service demands, leading to proactive adjustments in operations. By adopting these AI-driven tools, organizations can significantly reduce response times and optimize resource allocation.
Understanding service efficiency involves recognizing key factors such as customer feedback, service delivery times, and operational bottlenecks. AI tools help in identifying these patterns, allowing businesses to make informed decisions. Furthermore, predictive planning enables organizations to adapt swiftly to shifting customer expectations, thus enhancing overall service quality. Timely insights lead to improved communication and collaboration among teams, making it easier to implement effective strategies that benefit both the business and its customers.
- Proactive Issue Detection and Resolution
Proactive issue detection and resolution is crucial in maintaining smooth service levels. By utilizing Predictive Planning AI, businesses can foresee potential problems before they escalate. This involves collecting data and identifying patterns that signal upcoming issues, allowing for timely intervention. For instance, learning algorithms can analyze customer interactions and service metrics, helping teams pinpoint areas of concern that may impact user satisfaction.
To implement effective proactive detection and resolution strategies, organizations should consider the following steps:
Data Analysis: Continuously analyze historical data to identify trends and recurring issues. This helps in understanding the root causes of problems.
Feedback Loops: Establish channels for customer feedback, enabling real-time insights into potential service interruptions. Quick adjustments can be made based on direct input from clients.
Employee Training: Equip staff with the knowledge of predictive tools and empower them to respond to potential issues efficiently. Their expertise can bridge gaps that AI might not fully address.
By integrating these practices with Predictive Planning AI, companies can foster a proactive service environment, ensuring customer satisfaction remains a priority.
Extract insights from interviews, calls, surveys and reviews for insights in minutes
Utilizing AI-Powered Tools for Effective Predictive Planning
AI-powered tools are transforming how organizations approach predictive planning. These tools provide valuable insights into past and current data, enabling teams to make informed decisions for future service levels. By leveraging predictive planning AI, businesses can analyze trends, anticipate service demands, and enhance operational efficiency.
To effectively utilize these AI tools, the first step is data collection and integration. Gathering data from various sources ensures a comprehensive understanding of service dynamics. Next, model selection and training are crucial. Choosing the right AI model is essential for accurate predictions tailored to specific service environments. Finally, implementation and monitoring of the AI tools allow organizations to evaluate performance continually and adapt strategies as needed. By following these steps, companies can maximize the benefits of AI-driven predictive planning and optimize their service delivery.
Key Steps in Implementing AI Tools for Service Level Planning
Implementing AI tools for service level planning involves a structured approach to ensure effective integration and utilization. The first step is robust data collection and integration, which serves as the foundation for predictive planning AI. Gathering accurate and diverse datasets aids in building reliable models that reflect real-world scenarios. This stage also includes integrating diverse data sources to create a comprehensive picture of service performance.
Following this, model selection and training become crucial. Choosing the appropriate AI model depends on specific planning needs and service metrics. Training the model with historical data helps it learn patterns and predict future service levels accurately. This ensures that the predictive planning AI provides meaningful insights. Lastly, implementation and continuous monitoring are necessary to evaluate the AI tool's performance and effectiveness. Regular assessments help refine the model and adapt to changing market or service conditions, ultimately driving better decision-making and operational efficiency.
- Step 1: Data Collection and Integration
Data collection and integration are essential first steps in successfully implementing Predictive Planning AI for service level management. High-quality data serves as the foundation for accurate predictions and insights. Begin by gathering relevant data from various sources, including customer interactions, service performance metrics, and market trends. It is crucial to ensure that the data is clean, consistent, and organized. Employing automated tools can streamline this process, significantly reducing manual efforts and errors.
Once data is collected, integration follows. This involves consolidating data into a single platform where it can be uniformly analyzed. Utilize advanced data integration tools that facilitate real-time updates, providing a comprehensive view of service performance. By synthesizing diverse data points, Predictive Planning AI can identify trends and potential issues early, enabling proactive service adjustments. Ultimately, this robust data foundation empowers organizations to make informed decisions and optimize service levels effectively.
- Step 2: Model Selection and Training
Selecting the appropriate model for your Predictive Planning AI is crucial. This process involves evaluating various algorithms and methodologies that best suit your specific needs and objectives. Begin by analyzing the nature of the data you've collected, as different models excel in different scenarios. For instance, time-series analysis might be ideal for historical data trends, while classification algorithms could be better for categorical outcomes. Identifying your goals clearly will also guide your choices.
Once the model selection is made, focus turns to training. This phase entails feeding the chosen model with data to help it learn patterns and make accurate predictions. Pay careful attention to the quality of your training data, as it can significantly impact the modelโs performance. Regularly refine and validate your model during training, ensuring that it adapts to new information and continues to deliver reliable forecasts for service level planning. Consistent assessment and adjustment will help maintain the effectiveness of your Predictive Planning AI system.
- Step 3: Implementation and Monitoring
The implementation and monitoring phase is crucial for optimizing your predictive planning efforts. Begin by integrating the predictive planning AI tools into your existing systems. Ensure that all relevant data streams are connected, allowing the AI to analyze real-time metrics efficiently. Successful deployment requires thorough testing to confirm that the AI is functioning as intended and accurately providing insights.
Monitoring is equally important. Regularly review the performance of your predictive planning AI to identify any discrepancies or areas for improvement. Adjust parameters and update models based on feedback gathered during this phase. This ongoing evaluation will help you fine-tune the AI's capabilities, ensuring it continues to meet your organizationโs evolving needs. Consistent monitoring also facilitates proactive issue identification, thereby enabling timely problem resolution and maintaining optimal service levels. By establishing clear metrics for success, you can effectively harness the full potential of predictive planning AI in service level management.
Top AI Tools for Predictive Planning AI
Predictive Planning AI is revolutionizing how organizations manage service levels. As the demand for real-time insights increases, businesses must leverage advanced tools to enhance efficiency and responsiveness. There are several top AI tools designed specifically for predictive planning. These tools can automate data processing, analyze trends, and deliver actionable insights, transforming data into effective decision-making frameworks.
Key tools include IBM Watson AIOps, which facilitates IT service management through predictive analytics, and Google Cloud AI Platform, known for its robust machine learning capabilities. Microsoft Azure Machine Learning provides an extensive environment for building and deploying models, while H2O.ai offers accessible AI solutions for data-driven businesses. Each of these tools plays a pivotal role in predictive planning, allowing organizations to not only anticipate future challenges but also proactively address them, ensuring superior service delivery.
- insight7
Effectively harnessing Predictive Planning AI can significantly transform your approach to service level management. The journey begins by understanding core fundamentals, such as data collection and integration. Speaking to this process, successful predictive planning requires a holistic view of data inputsโfrom customer interactions to operational metrics. This data forms the basis for accurate forecasting and proactive decision-making.
Next, consider model selection and training. Organizations must choose the right algorithms tailored to their specific needs. This step ensures the AI tool learns from historical data patterns, enhancing its ability to predict future service needs. Once implemented, continuous monitoring is essential. Regular evaluations help adjust models and refine strategies based on real-time insights.
By mastering these steps, organizations can transition from reactive to proactive service level management. This shift not only improves efficiency but also supports a culture of innovation through timely, data-driven actions.
- Tool 2: IBM Watson AIOps
IBM Watson AIOps is a transformative tool that integrates seamlessly into predictive planning strategies. By harnessing the power of machine learning and AI, it can analyze vast amounts of data to predict service level performance. Its proactive capabilities enable organizations to anticipate potential issues before they escalate, streamlining service delivery and enhancing overall operational efficiency.
When implementing this solution, organizations can benefit from its ability to monitor IT environments in real time. Key features include automated problem resolution, which minimizes downtime, and enriched insights that inform decision-making. By utilizing predictive planning AI, businesses can optimize resource allocation while ensuring customer satisfaction remains a top priority. In an ever-evolving digital landscape, embracing tools like IBM Watson AIOps can prove essential for maintaining competitive advantage and achieving service excellence.
- Tool 3: Google Cloud AI Platform
The Google Cloud AI Platform provides an accessible way for businesses to enhance their predictive planning capabilities. It offers user-friendly tools that do not require specialized training, making it easy for anyone in the organization to leverage data effectively. With its intuitive interface, users can quickly generate actionable insights from various datasets, fostering a culture of data-driven decision-making.
This platform supports several critical features essential for predictive planning AI. For instance, it allows for the analysis of multiple data sources simultaneously, helping to identify trends and pain points across service delivery processes. Users can aggregate insights into comprehensive reports, aiding in strategic planning and operational efficiency. By democratizing access to advanced analytics, the Google Cloud AI Platform streamlines the process of turning data into valuable insights, leading to improved service level performance.
- Tool 4: Microsoft Azure Machine Learning
Microsoft Azure Machine Learning is a robust tool that empowers businesses to harness data for predictive planning. This platform supports the development, training, and deployment of machine learning models. Organizations can leverage Azure to create sophisticated models that forecast service level demands and optimize resource allocation more effectively.
When utilizing this tool, users should focus on three key areas: data preprocessing, model training, and performance monitoring. Data preprocessing ensures the data is clean and formatted correctly for insights to emerge. Next, model training allows organizations to build predictive models tailored to their specific needs, enhancing service delivery. Finally, performance monitoring helps businesses adjust their strategies based on real-time analytics. Embracing this comprehensive approach can significantly improve an organizationโs predictive planning AI capabilities. By prioritizing accuracy and efficiency, companies can better anticipate customer demands and streamline operations seamlessly.
- Tool 5: H2O.ai
H2O.ai offers a powerful platform for organizations aiming to enhance their predictive planning AI capabilities. Its user-friendly design democratizes access to advanced analytics, enabling team members at all levels to engage with data without requiring extensive training. Users can quickly import data and generate actionable insights, streamlining predictive service level planning that is both intuitive and effective.
One of the standout features of this platform is its ability to analyze large volumes of data across multiple files simultaneously. This capability allows for the identification of trends, pain points, and opportunities that are critical for optimizing service delivery. By leveraging machine learning and advanced algorithms, organizations can derive meaningful insights that drive decision-making processes, making it an invaluable tool in the arena of predictive planning AI.
Conclusion: Embracing Predictive Planning AI for Optimized Service Delivery
Predictive Planning AI is revolutionizing the landscape of service delivery, enabling organizations to enhance their operational efficiency. By implementing these advanced tools, businesses can anticipate needs and respond proactively to potential challenges. This predictive approach not only streamlines processes but also elevates customer satisfaction by ensuring timely and relevant service.
Embracing this technology requires a commitment to integrating insights and data into all planning phases. As organizations adopt Predictive Planning AI, they can expect to optimize resource allocation and improve service level agreements. Ultimately, this integration fosters a more agile and responsive environment, paving the way for sustained competitive advantage in an ever-evolving market.