AI-Driven Topic Tagging is revolutionizing how researchers process interview data, transforming a once tedious task into a streamlined, efficient process. Traditional methods of analyzing interviews often lead to inconsistencies and significant delays in insights due to manual efforts. Researchers seek faster and more reliable ways to capture the essence of interviews, making AI an invaluable tool in this transformation.
Embracing AI for topic tagging enables teams to minimize bias and improve accuracy in their insights. By leveraging advanced algorithms, researchers can systematically identify key themes in interviews, allowing for quicker report generation. This innovation not only enhances the quality of insights but also empowers teams to focus on strategic decision-making instead of time-consuming analysis.
Analyze qualitative data. At Scale.
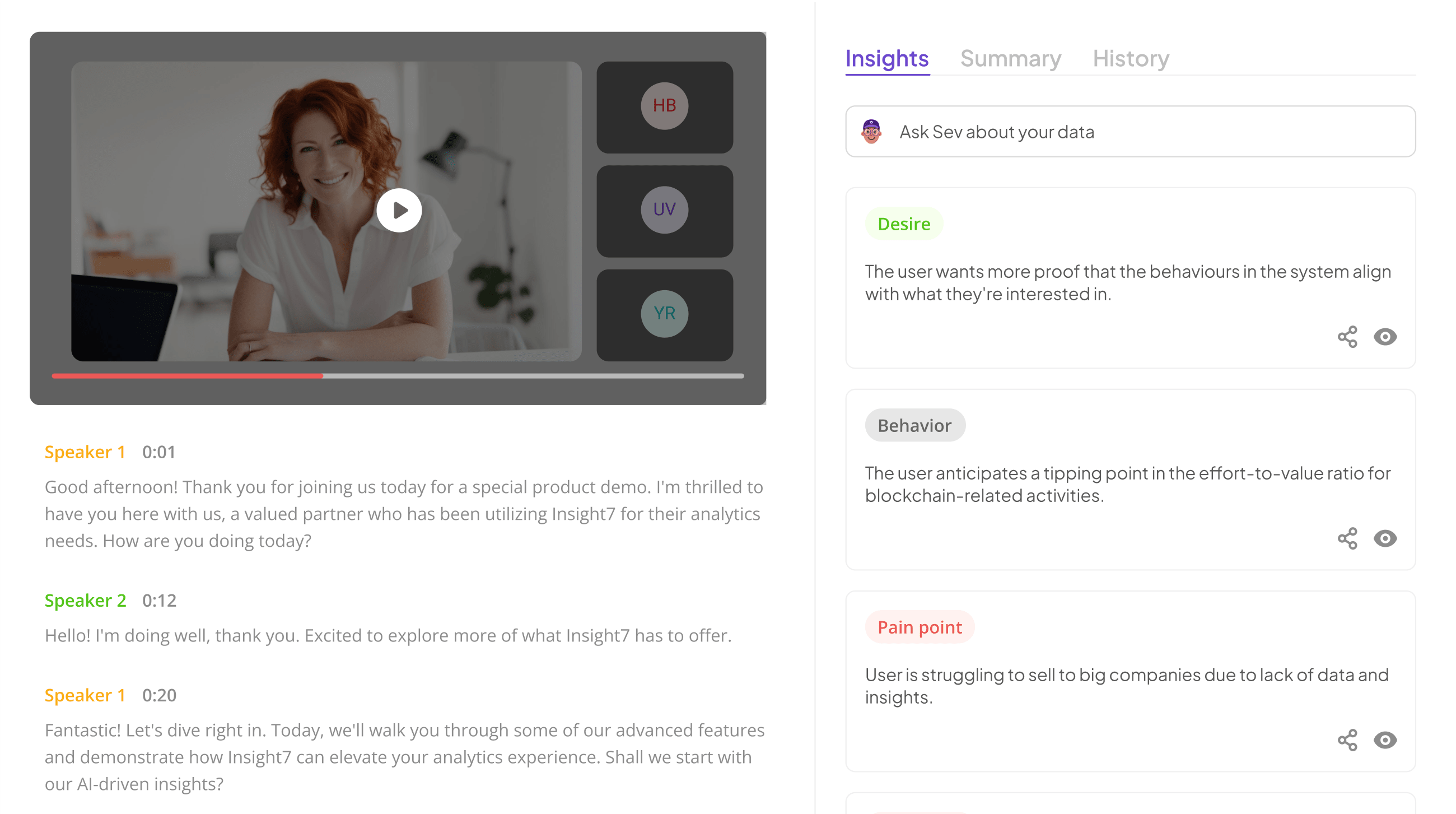
Understanding AI-Driven Topic Tagging
AI-Driven Topic Tagging transforms the way researchers handle data from interviews. By utilizing advanced algorithms, this technology enables the identification and categorization of key themes within spoken content efficiently. Researchers can process large amounts of qualitative data, making it easier to derive actionable insights and focus on essential findings.
The process begins with data ingestion, where audio recordings are transcribed and analyzed for recurring topics. This allows teams to cluster information and visualize connectivity between different insights. As a result, AI-Driven Topic Tagging not only saves time but also enhances the accuracy of research outcomes. By applying this method, researchers can streamline their workflow and present comprehensive reports that highlight the most relevant insights gleaned from their interviews. Embracing these innovative techniques will ultimately lead to richer research and informed decision-making.
The Role of AI in Modern Research
AI-Driven Topic Tagging streamlines the research process, transforming how we analyze qualitative data. One of the key roles of AI in modern research is enhancing efficiency by executing complex tasks like topic tagging. Researchers often face challenges in manually sifting through extensive interview transcripts, which can lead to inconsistencies and delays. AI tools adeptly manage these concerns by rapidly categorizing data into key themes, allowing researchers to focus on deriving meaningful insights.
Furthermore, AI facilitates a more objective approach to data analysis. It minimizes biases that can occur when multiple researchers interpret the same content differently. The result is a consistent, timely output of insights crucial for informed decision-making. Embracing AI-driven topic tagging not only accelerates the research workflow but also elevates the quality of analysis, leading to deeper understanding and impactful research outcomes.
Benefits of Using AI for Topic Tagging
Using AI-Driven Topic Tagging in research interviews offers substantial advantages. First, it significantly improves efficiency. Traditional methods of tagging topics can be time-consuming and often inconsistent. AI systems can quickly analyze large volumes of data, identifying patterns and key themes faster than a human can. This rapid processing frees researchers to focus on deeper analysis rather than manual tagging.
In addition to efficiency, AI-Driven Topic Tagging enhances accuracy. Human error often occurs in manual tagging, leading to unreliable insights. AI algorithms, trained to understand context and nuance, reduce these mistakes by consistently applying tagging criteria. Moreover, the transparency in AI systems allows researchers to trace back the origin of insights. This feature supports greater trust in the findings, ensuring that decisions based on these insights are well-founded. Overall, integrating AI into topic tagging optimizes both the quality and speed of data analysis in research interviews.
Extract insights from interviews, calls, surveys and reviews for insights in minutes
Implementing AI-Driven Topic Tagging in Your Research Workflow
Implementing AI-Driven Topic Tagging in Your Research Workflow begins with preparing your interview data. This initial step is crucial, as it involves cleaning and structuring your research material. Organize transcripts, audio recordings, or notes into a format that AI tools can effectively analyze. A well-structured dataset will enhance tagging accuracy and relevance.
Next, selecting the right AI tools is fundamental for successful implementation. Consider tools that offer customizable options, allowing you to train the AI on industry-specific language and themes. After selecting a tool, you will need to train the AI model using a sample of your tagged data. This training process enables the AI to understand the context and nuances within your interviews. Finally, evaluating and refining tagging accuracy ensures that the AI outputs align with your research goals. Adjust parameters and continuously assess results to maximize the benefits of AI-Driven Topic Tagging in your workflow.
Step-by-Step Guide to Automated Topic Tagging
Automated topic tagging enhances the efficiency and accuracy of analyzing research interviews. To implement AI-driven topic tagging effectively, begin by preparing your interview data. Ensure that all audio files are transcribed and cleaned up. This initial step lays the foundation for a smooth tagging process by removing any irrelevant information or noise.
Next, selecting the right AI tools is crucial. Look for platforms that specialize in natural language processing and offer robust analysis features. Once you've chosen a tool, focus on training the AI model with your data. This involves feeding the AI examples so it can learn to identify patterns and key themes. Finally, evaluating and refining the tagging accuracy ensures that the results are reliable and aligned with your research goals. Through these steps, AI-driven topic tagging can transform how you synthesize insights from your interviews.
- Step 1: Preparing Your Interview Data
Preparing your interview data is a crucial initial step in the process of AI-driven topic tagging. Begin by ensuring that all data is gathered systematically and cleaned for analysis. This may involve transcribing recorded interviews, organizing files, and reviewing content for clarity. Clear and coherent data facilitates better insights, making it essential to document and format the information accurately.
Next, categorize your interviews based on themes or topics relevant to your research. This organization helps in identifying key areas where AI can tag relevant themes effectively. You may consider grouping interviews by participant demographics, subject matter, or even sentiment expressed during conversations. By laying this groundwork, you prepare your data for AI-driven topic tagging, allowing the technology to efficiently analyze patterns and extract meaningful insights. These insights can then be translated into actionable results for your research objectives.
- Step 2: Selecting the Right AI Tools
Selecting the right AI tools for AI-Driven Topic Tagging is crucial to maximizing the efficiency and accuracy of your research. Begin by identifying your specific needs, such as the volume of data or the complexity of themes you wish to tag. Each tool offers unique functionalities, such as filtering by speaker or type, which can significantly enhance your analysis. Understanding these features upfront can streamline your workflow and improve the quality of insights you derive.
Next, evaluate tools based on their ease of integration with your existing systems and the level of customization they offer. Itโs beneficial if the tool allows you to define custom themes or topics relevant to your research area. Ultimately, the right AI tools can help you quickly identify and tag key topics in your interviews, converting raw data into actionable insights efficiently and reliably. As you explore options, ensure you read user reviews and documentation to find the best fit for your research goals.
- Step 3: Training the AI Model
Training the AI model is a pivotal step in implementing AI-driven topic tagging effectively. Start by gathering a diverse and comprehensive dataset that reflects the various themes and responses typical in your research interviews. This dataset will serve as the foundation for teaching the AI model to recognize relevant topics accurately. Itโs essential to ensure the data is clean and organized to facilitate efficient learning.
Next, configure the model parameters to align with your research needs. You can choose from various algorithms tailored for text analysis, such as natural language processing or machine learning methods. Each algorithm will affect how the AI interprets the data and ultimately impacts the tagging process. Conduct training sessions iteratively, refining the model based on its performance during evaluations. Through this methodical approach, the AI will learn to tag key topics with precision, enhancing your research insights and outcomes significantly.
- Step 4: Evaluating and Refining Tagging Accuracy
Evaluating and refining tagging accuracy is a crucial aspect of AI-driven topic tagging in research interviews. To ensure that the AI system effectively identifies and categorizes key themes, continuous assessment is necessary. Start by comparing the AI-generated tags with the actual content of the interviews. This process can reveal discrepancies, highlighting areas where the AI may struggle with context or nuance.
Next, gather feedback from researchers who use these tags. Their insights will guide adjustments in the tagging algorithms. Itโs also essential to establish a set of criteria for what constitutes a successful tag, such as relevance, precision, and consistency. By regularly updating the AI model based on these evaluations, you can greatly enhance its performance. This iterative approach not only improves accuracy but also increases confidence in the insights derived from the research data. Ultimately, fostering a process of evaluation and refinement contributes to the effectiveness of AI-driven topic tagging and ensures more reliable outcomes from research interviews.
Top Tools for AI-Driven Topic Tagging
When considering the implementation of AI-Driven Topic Tagging, selecting the right tools is critical for success. Numerous tools offer features like semantic analysis and easy data integration, which can enhance the accuracy and efficiency of your tagging process. For instance, Insight7 allows users to extract insights and generate reports swiftly, tailoring the output based on specific themes or keywords. This flexibility makes it easier to focus on particular areas of interest during analysis.
Another noteworthy tool is MonkeyLearn, which excels in text classification and provides users with customizable templates tailored to specific research needs. Tagtog is renowned for its user-friendly interface, enabling users to annotate and categorize data effortlessly. Likewise, platforms like Keatext and Thematic leverage natural language processing to uncover hidden insights, creating a more nuanced understanding of the conversation data collected. By choosing tools that best align with your research objectives, you can maximize the benefits of AI-Driven Topic Tagging, transforming raw interview data into meaningful insights.
- Insight7
AI-Driven Topic Tagging can significantly transform how researchers analyze and interpret qualitative data gathered from interviews. At its core, this technology automates the process of identifying key themes and topics, saving time and increasing accuracy. By employing advanced algorithms, AI can process vast amounts of textual data, pinpointing recurring subjects that may not be immediately apparent to human analysts. This capability enhances the overall research process, allowing teams to focus on interpreting insights rather than sorting through data.
To effectively implement AI-Driven Topic Tagging, researchers should prioritize proper data preparation and select appropriate AI tools tailored to their specific needs. Training the AI model on relevant datasets ensures a more refined tagging accuracy, while ongoing evaluation helps maintain the effectiveness of topic tagging over time. By integrating these practices, researchers can leverage AI's potential to streamline their workflows and derive clearer insights from their interview data, ultimately fostering more informed decision-making.
- MonkeyLearn
In the realm of AI-driven topic tagging, a specific platform excels in transforming raw interview data into structured insights. This tool harnesses advanced algorithms to help users seamlessly categorize information, which significantly enhances the research process. By accurately identifying key themes, it enables researchers to focus on pivotal insights rather than getting lost in data.
To leverage this technology effectively, follow a strategic approach. First, prepare your interview data to ensure it's clean and organized. Next, select the appropriate AI tools that align with your specific needs, as each tool offers unique functionalities. Additionally, training the AI model with relevant examples will improve tagging accuracy. Finally, continuously evaluate the results and refine the model to ensure it evolves with your research requirements. Exploring these functionalities unlocks the immense potential of AI-driven topic tagging, making your research both efficient and effective.
- Tagtog
Tagtog serves as an exemplary tool in the realm of AI-Driven Topic Tagging, particularly when processing insights from research interviews. By utilizing advanced algorithms, the platform can categorize and tag key topics, transforming raw data into organized themes. This system not only streamlines the analysis process but also enhances the relevance of each insight, allowing researchers to focus on what truly matters in their findings.
The effectiveness of Tagtog is particularly notable when it comes to defining and refining tags based on specific project goals. Users can easily select default tags or customize them according to their needs, whether focusing on risks or collaboration themes. This flexibility is crucial for generating meaningful insights. With its ability to cluster insights and provide sentiment analysis, Tagtog stands as a powerhouse in ensuring that research efforts yield actionable outcomes, ultimately saving valuable time in the research process while maintaining high data integrity.
- Keatext
Keatext is an innovative tool that significantly enhances AI-driven topic tagging in research interviews. This platform processes large volumes of data, transcribing interviews efficiently and seamlessly into a format that can be analyzed. By harnessing advanced algorithms, Keatext identifies key themes and insights from textual responses, aiding researchers in understanding complex narratives.
The power of Keatext lies in its intuitive design, making it accessible even for those unfamiliar with technical processes. After transcription, users can extract quotes and relevant clips, allowing for a comprehensive analysis of sentiments and trends. This capability not only helps in organizing data but also facilitates the activation of insights across various channels. As a result, teams can refine their research strategies based on solid, data-driven inputs, ensuring that valuable perspectives are never overlooked.
- Thematic
Thematic analysis is crucial for identifying and understanding key concepts within qualitative data, particularly in research interviews. By harnessing AI-driven topic tagging, researchers can streamline this process effectively. When utilizing thematic analysis, the objective is to explore patterns that emerge from participants' responses, allowing deeper insights into their perspectives and the overall subject matter.
Firstly, defining your themes is essential in guiding the analysis. Themes should resonate with the research goals and provide clarity to the findings. Secondly, AI tools can assist in organizing data according to these themes, making it easier to extract meaningful insights. These tools analyze transcripts and highlight recurring ideas, offering visual contexts for better understanding. By automating this process, researchers can focus on interpreting results, ensuring more accurate outcomes while saving valuable time in their research workflow. Thus, AI-driven topic tagging serves as a powerful ally in enhancing the efficiency and depth of research analysis.
Conclusion: Maximizing the Impact of AI-Driven Topic Tagging in Research
To fully realize the benefits of AI-Driven Topic Tagging, researchers must embrace its capabilities beyond simple automation. The technology not only expedites the tagging process but also enhances the quality of insights drawn from interviews. By harnessing advanced algorithms, researchers can uncover thematic connections that might be overlooked in traditional analysis.
Moreover, maximizing the impact of this technology involves a commitment to refining tagging accuracy continuously. Engaging with feedback loops and iterating on the tagging model cultivates a more robust understanding of the research data. As a result, researchers can create richer, more nuanced narratives that resonate deeply with their target audience. AI-Driven Topic Tagging ultimately empowers researchers to navigate complex data landscapes with greater efficiency and precision.