How to Train AI Agents to Assist in Coaching Feedback Loops
-
Bella Williams
- 10 min read
AI Coaching Assistants have emerged as invaluable tools in enhancing feedback loops, revolutionizing how individuals and teams receive and process feedback. Imagine a team navigating multiple projects with conflicting insights; this is where AI Coaching Assistants play a pivotal role by providing cohesive support that fosters productive discussions and decision-making. By analyzing vast amounts of data, these assistants can clarify needs and streamline communication, allowing users to focus on actionable outcomes.
Training these AI agents to assist in coaching feedback loops requires careful thought and planning. Clear objectives must be set, and robust data collection methods need to be established to inform their programming. The development of feedback algorithms is crucial for customizing responses that align with user expectations. Ultimately, the effective integration of AI Coaching Assistants can lead to a more efficient, responsive coaching environment, enhancing both individual and team performance.
Analyze & Evaluate Calls. At Scale.
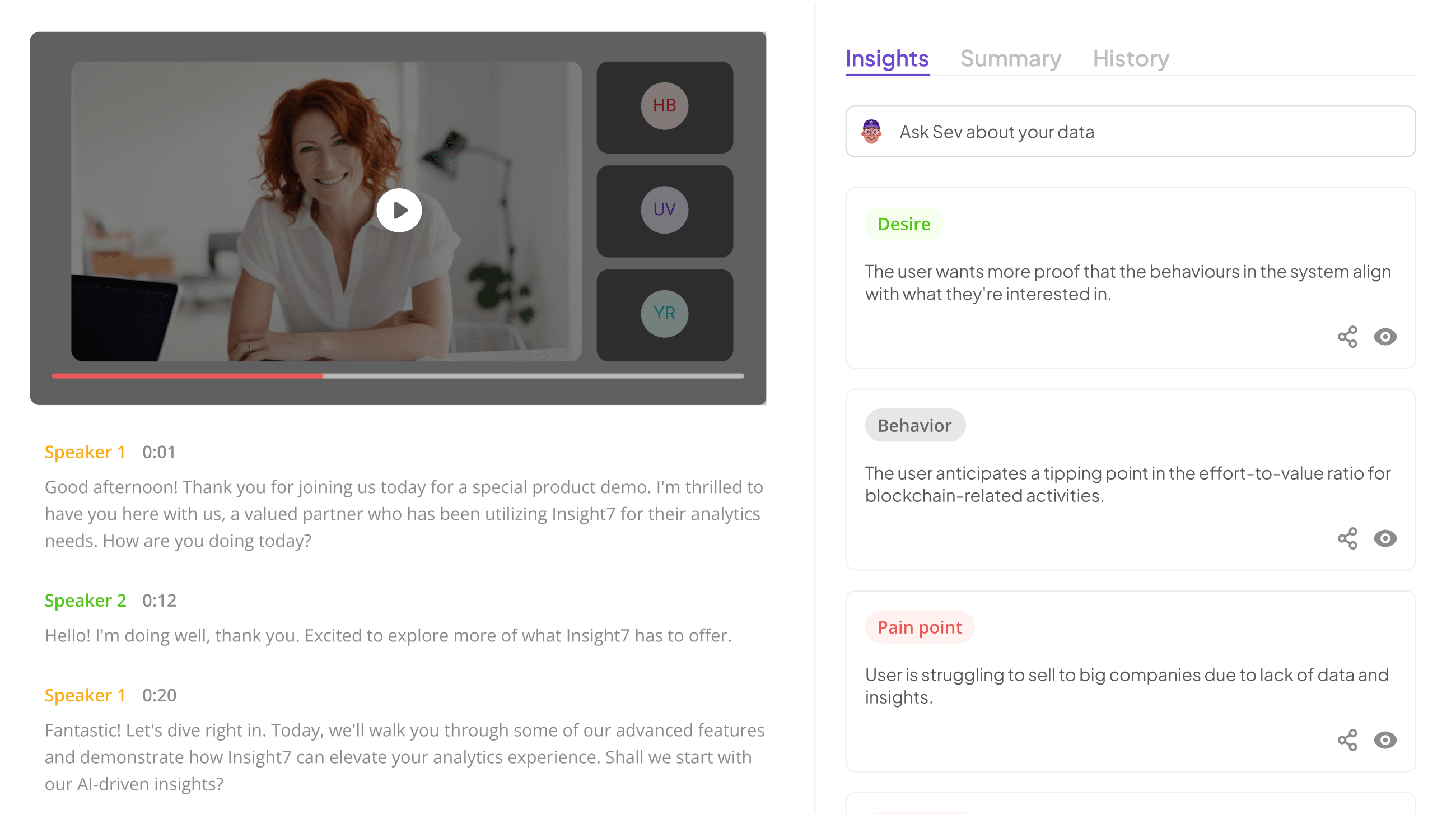
Understanding the Role of AI Coaching Assistants
AI Coaching Assistants are revolutionizing the way feedback loops function in various coaching environments. By closely analyzing data and interpreting user interactions, these AI systems can provide real-time insights that lead to improved performance. With their ability to adapt and learn from ongoing conversations, AI Coaching Assistants help coaches identify areas for growth and development. This dynamic interaction fosters a continuous feedback mechanism, allowing for timely adjustments and enhanced learning experiences.
Furthermore, AI Coaching Assistants personalize content and suggestions based on individual needs. They compute vast amounts of information quickly, creating tailor-made responses that resonate with each user's preferences. This personal touch not only encourages engagement but also inspires users to take actionable steps toward their goals. Understanding their role allows coaches to maximize the potential of AI Coaching Assistants, effectively transforming traditional coaching practices into a more connected and intuitive process.
Enhancing Feedback Mechanisms
To enhance feedback mechanisms in coaching, it is essential to establish a rhythm of continuous improvement. AI Coaching Assistants can play a pivotal role in this process by effectively monitoring interactions and providing real-time analysis. By harnessing data from customer service interactions, these assistants can identify common questions and issues that arise, enabling organizations to adjust their strategies and improve service offerings.
Furthermore, incorporating regular feedback reporting, such as monthly summaries, can illuminate trends, highlight training needs, and guide marketing efforts. With a systematic approach to analyzing customer inquiries, AI Coaching Assistants facilitate actionable insights, ensuring that organizations stay aligned with their customers' needs. This iterative feedback process ultimately allows for more responsive adaptations and better overall performance in coaching feedback loops.
Personalizing Learning with AI
AI Coaching Assistants hold immense potential for revolutionizing personalized learning. By analyzing individual preferences and behavioral patterns, these assistants can craft tailored experiences that adapt to the unique needs of each user. This adaptability fosters an environment where learners feel understood and supported, significantly enhancing engagement and motivation.
To achieve effective personalization, AI Coaching Assistants utilize several key approaches. First, they gather detailed data on user interactions, enabling them to identify specific learning styles and preferences. Second, they tailor content and feedback based on this data, ensuring that learners receive relevant insights that resonate with their current objectives. Lastly, through continuous interactions, AI can refine its understanding of learners, allowing it to offer ever-improving support. Embracing these capabilities can transform traditional learning experiences, making them more intuitive and effective.
Extract insights from interviews, calls, surveys and reviews for insights in minutes
Key Steps to Train AI Coaching Assistants for Effective Feedback
To train AI coaching assistants effectively, several critical steps must be taken. First, defining the objectives is paramount. Establish clear goals that align with the coaching strategy, ensuring the AI’s purpose is apparent. This clarity helps shape the subsequent processes and sets the foundation for success.
Next, data collection and preparation come into play. Gather a wide range of relevant coaching interactions, feedback examples, and performance metrics. Quality data is essential, as it enables the AI to learn and improve its feedback mechanisms over time. Developing effective feedback algorithms is the next step, focusing on how the AI interprets data and generates meaningful responses.
Finally, evaluating and iterating on feedback models ensures continuous improvement. Regular assessments and refinements allow the AI coaching assistants to adapt, thus enhancing their performance in real-time coaching situations. Following these steps establishes a robust system for effective, data-driven coaching feedback.
💬 Questions about How to Train AI Agents to Assist in Coaching Feedback Loops?
Our team typically responds within minutes
Step 1: Defining the Objectives
Defining the objectives for AI Coaching Assistants is a foundational step in developing effective feedback loops. Start by identifying the specific goals these AI tools should achieve, keeping in mind the unique needs of users. For instance, objectives may include providing timely feedback, enhancing learning processes, or improving engagement. These clear goals serve as benchmarks for measuring success and refining AI behavior throughout the training process.
Next, it's vital to align these objectives with Key Performance Indicators (KPIs) that reflect user engagement and satisfaction. Establishing KPIs ensures that your coaching assistants can adapt and respond to changing user needs effectively. Regularly revisiting and updating these goals based on feedback and performance data facilitates a dynamic feedback loop, allowing the AI system to enhance its learning and support over time. Thus, a well-defined initial objective is critical for the long-term success of AI Coaching Assistants in feedback loops.
Step 2: Data Collection and Preparation
To effectively train AI Coaching Assistants, Step 2: Data Collection and Preparation is vital. This stage focuses on gathering essential data that will inform the AI’s learning process. It involves identifying relevant qualitative research findings that reflect customer insights. By synthesizing these insights, you develop a robust framework that will guide the AI in understanding user behavior and preferences.
During this phase, it's important to outline the customer journey through defined stages. These stages include Awareness, Interest, Consideration, Purchase, Usage, and Repurchase. Each stage informs the development of interrogation points pertaining to user sentiments and motivations. Gathering data through methods such as interviews and surveys enriches the dataset, allowing AI Coaching Assistants to be trained with a more comprehensive understanding. Adequate preparation ensures that the insights gleaned will directly impact the effectiveness of the feedback loops, making them more aligned with user needs and expectations.
Step 3: Developing Feedback Algorithms
Developing feedback algorithms is a crucial step in training AI coaching assistants. These algorithms enable the AI to process and analyze feedback effectively, facilitating the identification of patterns and insights. It’s essential to fine-tune these algorithms based on specific coaching objectives and user needs. By integrating user data and feedback history, the AI can deliver more relevant suggestions and insights.
To enhance the effectiveness of feedback algorithms, consider the following aspects:
- Data Segmentation: Focus on breaking down feedback into specific categories for targeted analysis.
- Needs Identification: Integrate methods for the AI to understand and extract user needs from feedback data.
- Pattern Recognition: Utilize machine learning techniques to identify trends and patterns across various feedback inputs.
- Feedback Personalization: Ensure that the AI adapts its responses based on individual user profiles and preferences.
By embracing these elements, AI coaching assistants can significantly improve their feedback loops, making the coaching experience more personalized and insightful for users.
Step 4: Evaluating and Iterating on Feedback Models
To effectively evaluate and iterate on feedback models used by AI Coaching Assistants, it is essential to implement a structured process. First, you should assess the performance of your feedback algorithms based on established criteria, such as accuracy, relevance, and user satisfaction. Collect data from actual coaching sessions, analyzing interactions to identify what works and what needs improvement. Evaluate not only the content of the feedback provided but also how well it engages users and encourages learning.
Once the evaluation phase is complete, it’s time to iterate on the models. This could involve refining algorithms, updating criteria based on user feedback, and integrating new data sources to enhance the coaching experience. Continuous iteration ensures that AI Coaching Assistants remain effective and relevant, adapting to evolving user needs. It is through this repetitive process of evaluation and refinement that the AI's coaching capabilities can be optimized for sustained success.
Conclusion: The Future of AI Coaching Assistants in Feedback Loops
The future of AI Coaching Assistants in feedback loops holds immense promise for enhancing coaching dynamics. As these intelligent systems evolve, their ability to provide real-time, personalized insights will redefine how feedback is delivered and processed. By integrating advanced analytics and user behavior data, AI Coaching Assistants can identify specific areas for improvement, fostering a more customized coaching experience.
Moreover, the continuous learning capabilities of these assistants can create dynamic feedback loops, making coaching more responsive and effective. As they adapt and refine their approaches based on user interactions, organizations can leverage AI Coaching Assistants to cultivate a culture of growth and development. Embracing this technology will not only streamline feedback processes but also empower individuals to reach their full potential.
💬 Questions about How to Train AI Agents to Assist in Coaching Feedback Loops?
Our team typically responds within minutes