Trainable AI agents for real-time AI feedback are transforming the way organizations gather and analyze customer insights, providing instantaneous feedback that enables businesses to refine products, services, and strategies, while staying agile and responsive to evolving customerย needs.
The complexities of effectively training AI agents to utilize this feedback cannot be overlooked. Organizations can uncover valuable patterns and trends by training AI systems to interpret data dynamically. Understanding the intricacies of real-time AI feedback will enhance decision-making, enabling proactive responses that align closely with user expectations. This section will delve deeper into the methodologies and benefits associated with this transformative technology.
Analyze & Evaluate Calls. At Scale.
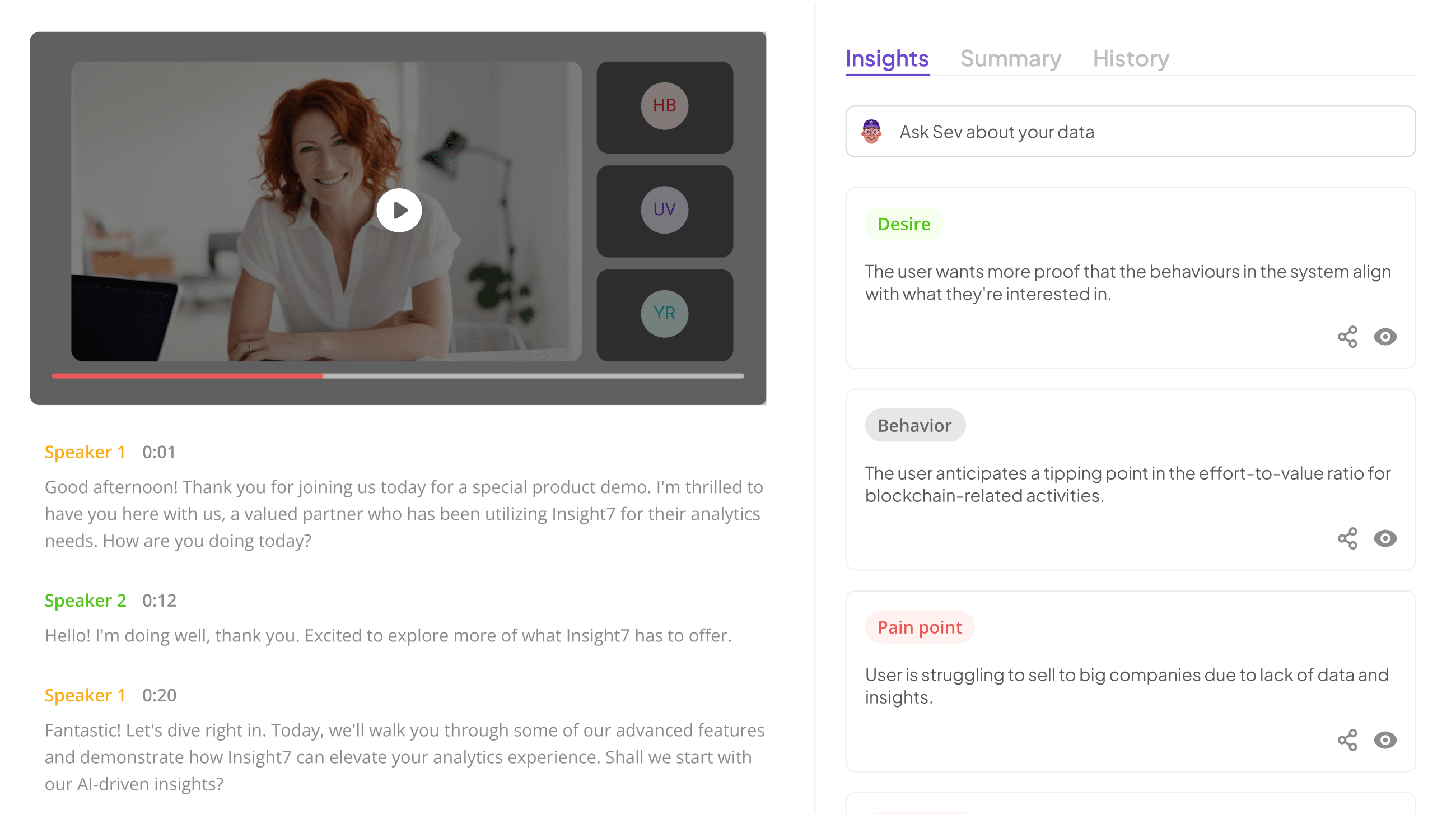
Harnessing Real-Time AI Feedback: Key Concepts
Real-time AI Feedback is pivotal to training AI agents for effective feedback analysis. By harnessing this immediate response mechanism, organizations can process insights rapidly, making timely decisions based on user interactions. Understanding how to implement and utilize this feedback allows teams to enhance their AI’s learning experience, leading to significantly improved outcomes.
Key concepts include the importance of data visualization, where AI analyzes both positive and negative comments from users. This analysis helps in refining products and services based on real-user experiences. Another crucial element is querying across extensive datasets, enabling teams to identify patterns and trends that inform future strategies. By focusing on these essential aspects, organizations can ensure their AI systems are continually adapting and evolving in response to dynamic market demands, thus fostering an environment of continuous improvement and user satisfaction.
The Importance of Real-Time AI Feedback
Real-time AI Feedback plays a critical role in enhancing the efficiency of AI agents. By providing instant insights, agents can make data-driven decisions, improving their performance significantly. When AI systems receive immediate feedback, they can adapt quickly to changing circumstances, ensuring they remain relevant and effective in various operational contexts.
Moreover, real-time feedback fosters continuous learning, enabling AI agents to identify areas for improvement dynamically. This iterative process enhances accuracy and builds trust among users who expect swift and reliable responses. Ultimately, integrating real-time AI feedback into training protocols facilitates a deeper understanding of user interactions, ensuring AI solutions meet evolving demands effectively. By prioritizing this feedback mechanism, organizations can significantly elevate the quality of their AI systems and deliver enhanced user experiences.
Real-Time Feedback for Trainingย AIย Agents
Real-Time AI Feedback is revolutionizing the field of AI training by allowing agents to learn and adapt instantly. Traditional training methods often rely on static data sets, which can limit growth and adaptability. However, with real-time feedback, agents receive continuous input on their performance, enabling them to refine their responses and improve accuracy swiftly.
This dynamic process consists of several critical elements. First, immediate feedback allows AI agents to correct mistakes as they occur, fostering a more effective learning environment. Second, it supports personalized learning, as agents can adapt to distinct scenarios in real-time. Finally, the iterative nature of this feedback loop contributes to building more robust AI systems, ultimately enhancing their reliability and effectiveness in various applications. By embracing these principles, organizations can harness the power of Real-Time AI Feedback to elevate their AI training efforts significantly.
Steps to Train AI Agents for Real-Time Feedback Analysis
Training AI agents for real-time feedback analysis involves several critical steps that ensure the effectiveness and adaptability of the system. The journey begins with meticulous data collection and preprocessing to gather relevant information. Refining and structuring this data is essential, allowing the AI to learn from high-quality inputs that clearly represent user sentiments and behaviors.
Next, selecting an appropriate model tailored to your specific needs is vital. This choice impacts how well the AI understands and processes real-time feedback. Once the model is in place, use the actual feedback to train the AI agents. Here, the AI learns to adapt to new information continuously, enhancing its analytical capabilities. The final stage involves rigorous evaluation and iteration. During this phase, you assess the AI’s performance and refine its processes based on insights gathered, thereby fostering a cycle of continuous improvement and effectiveness in real-time AI feedback analysis.
Step 1: Data Collection and Preprocessing
Data collection and preprocessing are pivotal to ensuring effective real-time AI feedback in this initial step to train AI agents. The first task involves gathering relevant datasets, which can include customer feedback, reviews, and interactions across various platforms. Collecting data that accurately reflects user experiences is crucial, as this will guide the AI’s understanding and ability to deliver insightful feedback.
Once data is gathered, preprocessing begins. This stage involves cleaning the data by removing any irrelevant information or noise, which allows for a clearer analysis. Standardizing formats and categorizing feedback into positive, negative, or neutral sentiments can significantly enhance the AIโs ability to analyze and interpret real-time insights. By systematically organizing the data, you set a solid foundation for the subsequent steps in training the AI agent, facilitating a more efficient journey toward actionable insights.
Step 2: Model Selection and Customization
To effectively train AI agents for real-time feedback analysis, selecting and customizing the right model is crucial for harnessing the full potential of trainable AI agent. Begin by assessing different machine learning algorithms, such as decision trees, neural networks, and support vector machines, based on the unique requirements of your data set. Matching the model’s capabilities to your specific tasks will significantly influence the performance of your AI agent in providing real-time insights.
Customization plays a critical role in tailoring the model to meet the nuances of your data. This involves adjusting parameters, modifying architectures, and even selecting features that align with your objective of real-time feedback. By understanding the intricacies of the modelโs behavior through continual fine-tuning, you ensure its effectiveness in delivering pertinent and timely insights. In this phase, validation is crucial; experimenting with various configurations will help identify the optimal settings, enhancing the overall feedback analysis process.
Step 3: Training the AI Model with Real-Time Feedback
In this crucial step, training the AI model with real-time feedback enables it to adapt and improve based on user interactions. The process begins by integrating continuous input from users, which serves as critical data for the AI. By capturing real-time feedback, the model can identify patterns and anomalies, allowing it to tune its responses effectively. As the model learns from actual user experiences, it evolves, becoming more adept at accurate analysis.
Incorporating real-time feedback not only enhances the AI’s accuracy but also fosters a more engaging user experience. Stakeholders must ensure that feedback channels remain open and accessible, enabling users to share their insights freely. This continuous cycle of learning and adaptation is essential for developing AI agents that exceed expectations and deliver actionable insights. Emphasizing real-time AI feedback transforms static models into dynamic systems, capable of evolving with their environment and meeting users’ needs effectively.
Step 4: Evaluation and Iteration for Improved Feedback
Effective strategies ensure that Real-Time AI Feedback mechanisms continuously improve in the evaluation and iteration phase. Start by analyzing the initial performance metrics derived from the AI agents’ feedback interactions. These metrics highlight strengths and weaknesses, providing a foundation for actionable insights. Next, develop a systematic approach to iterating your training model based on this feedback. Regularly update your evaluation criteria to reflect the evolving nature of customer interactions and expectations.
As new data becomes available, use it to refine the algorithms guiding your AI agents. This means assessing their responses and adapting them to new trends and user needs. By fostering a culture of continual evaluation, your agents will remain responsive and effective. Ultimately, the goal is to create a dynamic feedback loop where insights from real-time interactions inform ongoing development, leading to enhanced user experiences and improved performance over time.
Extract insights from interviews, calls, surveys and reviews for insights in minutes
Top Tools to Train AI Agents with Real-Time Feedback
To optimize real-time feedback AI training, various tools can significantly enhance the learning process. Each tool brings unique features to improve the accuracy and responsiveness of AI agents. For instance, TensorFlow offers robust frameworks designed for creating neural networks. It enables easy model modification and fine-tuning based on live data inputs, which is essential for real-time analysis.
Similarly, frameworks like PyTorch prioritize flexibility and speed, allowing quicker adjustments. IBM Watson stands out with its advanced natural language processing capabilities, making it ideal for sentiment analysis. Lastly, Azure Machine Learning Studio integrates seamlessly with other Microsoft services, enhancing data processing and visualization. Using these top tools contributes to effective training and empowers agents to analyze real-time feedback efficiently, ensuring they remain responsive to ever-changing user inputs and sentiment trends.
insight7
Training AI agents for Real-Time AI Feedback involves adeptly managing and interpreting data generated during interactions. Companies today are faced with a deluge of customer signals that traditional analysis methods struggle to comprehend quickly. To succeed, organizations need to harness AI capabilities that can provide immediate insights from data collected, making them responsive to customer needs and market dynamics.
The process begins with data collection and preprocessing, where raw customer interactions are organized for analysis. Next, selecting the right model tailored to specific needs enables AI systems to adapt better. Training these models effectively requires implementing real-time feedback mechanisms, ensuring continuous learning and improvement. Lastly, robust evaluation and iteration processes help refine agents further, making them increasingly adept at transforming insights into actionable strategies. When companies train AI agents this way, they can build a strong competitive edge in today’s fast-paced market, ultimately leading to enhanced customer satisfaction and business performance.
TensorFlow
TensorFlow is a powerful open-source library widely utilized for artificial intelligence and machine learning applications. Its flexible architecture allows developers to create complex neural networks while optimizing performance for real-time tasks. This capability makes TensorFlow an excellent choice for projects focused on Real-Time AI Feedback analysis, where speed and accuracy are critical.
When using TensorFlow, it’s crucial to consider several key elements. First, the seamless integration of various data types enables robust model training with extensive datasets. Second, the library’s support for CPUs and GPUs ensures efficient computations, essential for real-time feedback processing. Third, TensorFlowโs extensive community and wealth of resources provide valuable support for developers at all levels. By harnessing these aspects, organizations can effectively train AI agents to deliver timely insights, transforming raw data into actionable feedback for immediate decision-making.
PyTorch
PyTorch stands out as a powerful tool when training AI agents for real-time feedback analysis. Its dynamic computational graph allows flexibility, enabling developers to experiment with different neural network architectures. This versatility is particularly important in constructing models that need to adapt to changing data dynamics, a common challenge in feedback analysis.
Real-time AI feedback requires continuous learning from incoming data streams, and PyTorch facilitates this with its user-friendly interface and extensive library of pre-built functions. Developers can easily integrate their models with existing data pipelines, allowing for swift adjustments and enhancements based on real-time insights. Additionally, the community surrounding PyTorch is robust, offering support and shared resources that can accelerate development processes. Whether through fine-tuning existing models or creating new architectures from scratch, PyTorch is an excellent choice for harnessing the potential of AI in feedback analysis.
IBM Watson
IBM Watson provides powerful tools for harnessing Real-Time AI Feedback’s capabilities, enhancing AI agents’ training process. The technology employs sophisticated natural language processing and machine learning techniques to analyze feedback swiftly and accurately. By understanding the nuances of conversation, it enables businesses to gain valuable insights from interactions with customers in real time.
The system’s ability to track and evaluate individual agent performance is a game-changer. It can identify agentsโ strengths and weaknesses, leading to personalized training solutions. Additionally, automated report generation provides teams with clear, actionable insights in just minutesโstreamlining the feedback analysis process significantly. Training AI agents to utilize these insights fosters a culture of continuous improvement and customer-centric service, paving the way for enhanced decision-making and strategic growth.
Azure Machine Learning Studio
Azure Machine Learning Studio provides powerful tools for organizations looking to harness real-time AI feedback effectively. Offering a user-friendly interface simplifies the process of model creation and training, enabling users to build robust AI solutions without extensive coding knowledge. With its extensive library of machine learning algorithms, users can select the best models tailored for real-time feedback analysis.
Furthermore, Azure Machine Learning Studio allows for seamless integration with various data sources. This integration facilitates the immediate application of customer feedback into AI models, ensuring that insights are instantly actionable. By utilizing Azure’s capabilities, organizations can continuously refine their models through ongoing feedback, leading to more accurate predictions and enhanced decision-making. Thus, Azure Machine Learning Studio represents a crucial asset in the journey toward utilizing real-time AI feedback for optimized performance and actionable insights.
Conclusion: The Future of Real-Time AI Feedback in Feedback Analysis
The potential of Real-Time AI Feedback in feedback analysis is poised to reshape how organizations engage with their customers. In the coming years, advancements in AI technology will empower feedback systems to process and analyze customer opinions instantly. This real-time capability enables businesses to make data-driven decisions rapidly, fostering a more dynamic relationship with their audience.
As these AI systems evolve, they will allow for deeper insights and tailored responses, enhancing the overall customer experience. Continuous improvement in AI feedback mechanisms will drive innovation across industries. Embracing Real-Time AI Feedback streamlines feedback analysis and helps organizations stay ahead in meeting customer expectations.
Analyze & Evaluate Calls. At Scale.
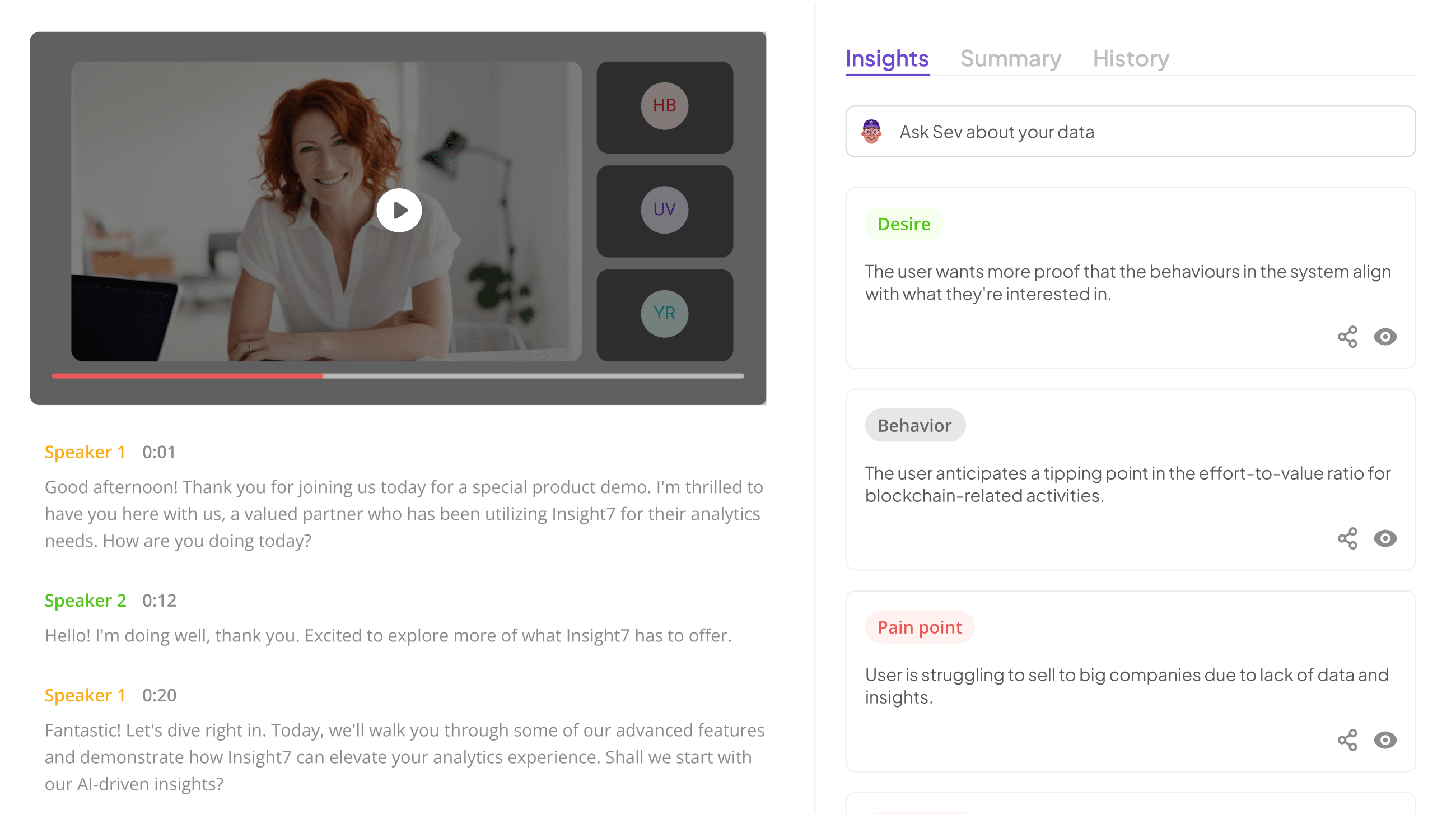