How to Train AI Agents for Predictive Strategic Intelligence
-
Bella Williams
- 10 min read
Predictive Strategy Training sets the foundation for AI agents to analyze complex data and make informed decisions. In today’s fast-paced landscape, organizations require AI agents capable of adapting to changing scenarios and strategic challenges. By implementing a structured training methodology, we can enhance AI’s understanding of predictive intelligence, enabling it to forecast outcomes effectively.
The essence of Predictive Strategy Training lies in merging data with innovative machine learning techniques. It empowers AI agents to simulate real-world scenarios, evaluate various strategies, and refine their performance through continuous feedback and adjustment. This training not only sharpens the agents' analytical capabilities but also fosters their ability to provide businesses with strategic insights that drive competitive advantage.
Analyze & Evaluate Calls. At Scale.
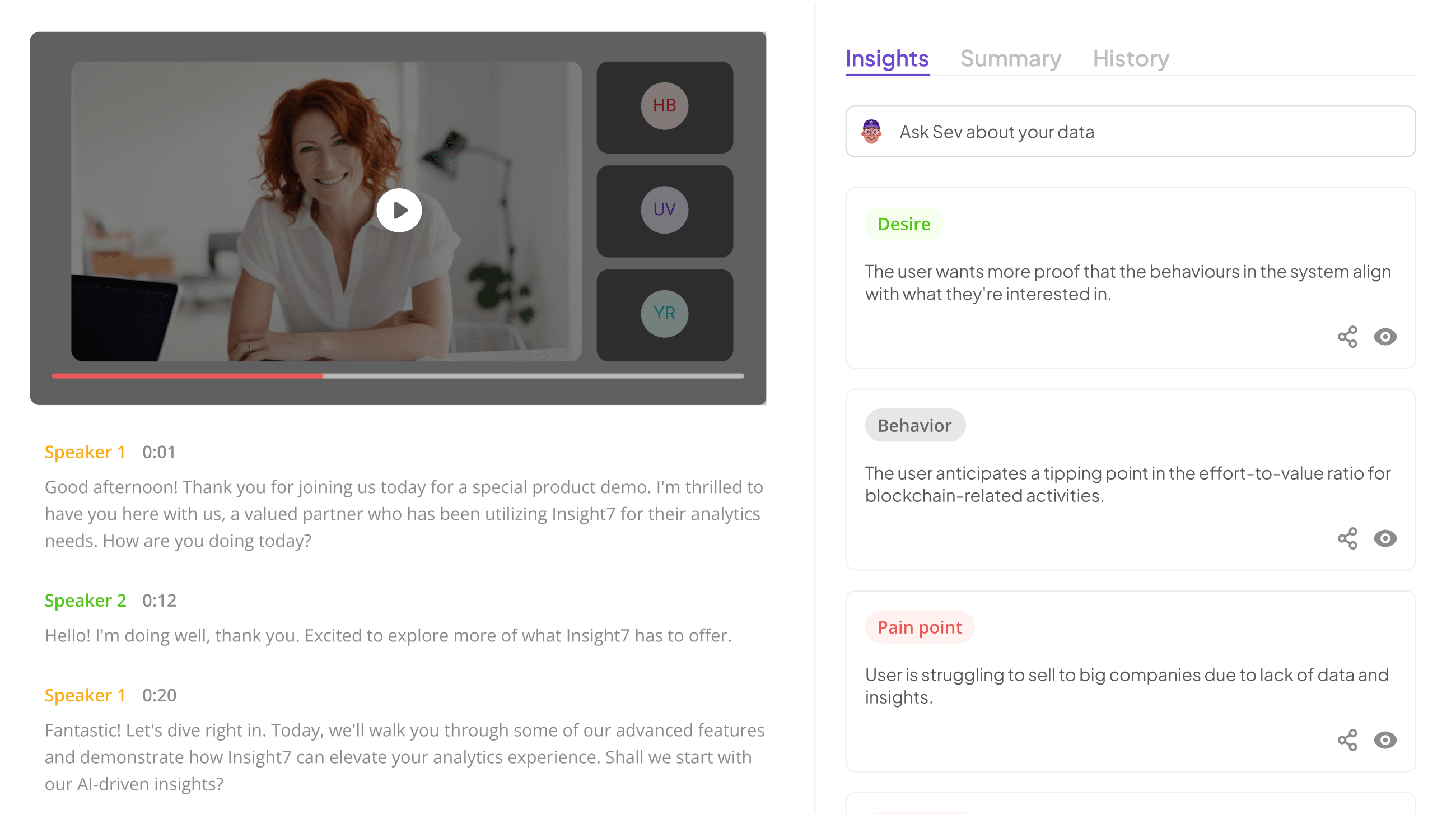
Understanding the Foundations of Predictive Strategy Training
In exploring the foundations of Predictive Strategy Training, it’s essential to understand the interplay between data, algorithms, and strategic insights. Predictive Strategy Training equips AI agents with the methodologies needed to analyze vast amounts of data and forecast outcomes accurately. This training encompasses essential principles that guide AI in making informed decisions based on available data trends and historical patterns.
The success of Predictive Strategy Training hinges on two critical elements. First, defining what predictive strategic intelligence encompasses is vital. This includes identifying various types of data that can influence predictions. Second, the importance of data cannot be overstated; high-quality, relevant data enhances the training process significantly. As AI models learn from diverse datasets, they become better equipped to uncover insights and predict future scenarios effectively. By establishing a robust foundation, organizations can harness the power of predictive intelligence to drive strategic initiatives and improve decision-making processes.
The Key Elements of Predictive Strategy Training
Effective Predictive Strategy Training hinges on several key elements that ensure AI agents operate with high accuracy and relevance. First, a clear definition of predictive strategic intelligence is imperative. This involves understanding the specific goals of the training, such as enhancing the agent’s ability to analyze patterns and predict outcomes based on data inputs. Clarity in goals sets a strong foundation for a targeted training approach.
Another essential element is the importance of data. High-quality, relevant data serves as the backbone of predictive strategy training. The agents must be exposed to diverse datasets, enabling them to learn effectively and make accurate predictions. Data preprocessing techniques are vital, allowing for cleaning and organization, thus maximizing the training process. Additionally, incorporating real-world scenarios through simulations can greatly enhance practical understanding and adaptability of the AI agents in complex situations. By focusing on these elements, organizations can cultivate AI systems equipped with robust predictive capabilities.
- Defining Predictive Strategic Intelligence
Predictive Strategic Intelligence involves the ability to anticipate future trends and make informed decisions based on data-driven insights. This form of intelligence integrates techniques from various disciplines such as data analysis, machine learning, and scenario planning. By leveraging historical data and real-time information, organizations can identify patterns that allow them to forecast potential outcomes and navigate uncertainties.
Understanding Predictive Strategic Intelligence helps in optimizing resources and aligning strategies with anticipated market shifts. As organizations train AI agents using Predictive Strategy Training, they focus on developing models that can analyze vast datasets efficiently. This not only enhances the accuracy of predictions but also enables proactive measures in business strategies. By mastering this approach, organizations can position themselves advantageously in an ever-evolving marketplace, ensuring they stay ahead of competition and meet customer expectations effectively.
- Importance of Data in Training AI Agents
Data plays a crucial role in training AI agents aimed at predictive strategy training. The effectiveness of these agents directly relies on the quality and relevance of the data used. Reliable datasets help AI systems recognize patterns and draw insightful predictions, creating value in strategic decision-making. When training an AI agent, incorporating diverse datasets ensures robust learning, allowing the system to adapt to varying scenarios and user inputs.
Moreover, comprehensive data analysis enables the identification of critical trends and anomalies. This analysis aids in refining predictive models, thereby improving accuracy over time. For instance, businesses can analyze customer feedback to enhance products and services. The importance of data cannot be overstated, as it forms the foundation for a successful predictive intelligence strategy. Consequently, it becomes essential to prioritize data collection, preprocessing, and validation in this training process to maximize the potential of AI agents within any strategic framework.
Key Techniques in Predictive Strategy Training
Predictive Strategy Training involves several effective techniques aimed at enhancing AI agents' capabilities to make informed forecasts. Key methods, such as machine learning algorithms and simulation analysis, create a robust foundation for training. Machine learning algorithms allow AI agents to learn from historical data, revealing patterns that inform strategic decisions. By applying various algorithms, agents can enhance their predictive accuracy.
Another critical technique is simulation and scenario analysis, which enables agents to test different strategic paths in a risk-free environment. This approach not only facilitates skill development but also allows for the optimization of responses to various hypothetical situations. Together, these techniques empower AI agents to operate more effectively in decision-making processes, ultimately driving better outcomes in predictive strategic intelligence. Incorporating real-time data feeds can further enhance this training, ensuring that AI agents stay updated with the latest trends and insights for optimal performance.
- Machine Learning Algorithms
Machine learning algorithms play a crucial role in predictive strategy training, enabling AI agents to make informed decisions based on extensive data analysis. These algorithms identify patterns within large datasets, allowing agents to predict future trends and behaviors. Various types of algorithms, such as supervised, unsupervised, and reinforcement learning, each contribute unique strengths to the predictive modeling process, enhancing overall accuracy.
To effectively leverage machine learning algorithms for predictive strategy training, it is essential to understand their functionalities. Supervised learning focuses on labeled input-output pairs to make predictions, while unsupervised learning uncovers hidden structures in unlabeled data. Reinforcement learning, on the other hand, involves agents learning optimal actions through trial and error. Selecting the appropriate algorithm will significantly impact the efficiency and outcome of your predictive models, thus enhancing decision-making capabilities for strategic insights.
- Simulation and Scenario Analysis
Simulation and scenario analysis plays a pivotal role in predictive strategy training. By creating virtual environments, AI agents can explore various strategic decisions and their outcomes. This practice allows agents to understand complex relationships within data, facilitating enhanced learning and decision-making capabilities.
In executing simulation and scenario analysis, several key elements come into play. For instance, defining objectives allows clarity on what scenarios to test. Following this, generating diverse scenarios ensures that agents are exposed to a wide range of possibilities, from best-case to worst-case outcomes. Finally, evaluating performance after simulations provides insights into an agent’s effectiveness in real-world applications. This holistic approach not only enriches the learning experience but also significantly improves cognitive strategies in the AI agents trained for predictive strategic intelligence.
Extract insights from interviews, calls, surveys and reviews for insights in minutes
Implementing Predictive Strategy Training: A Step-by-Step Approach
To implement Predictive Strategy Training effectively, one must start with a thorough plan. The first step is to gather and prepare data from various sources. This requires identifying the most relevant data sets to your specific objectives, which may include historical performance metrics, market trends, and user interactions. Once collected, preprocessing and cleaning this data is essential to eliminate inconsistencies and ensure accuracy.
Next, develop predictive models by selecting suitable algorithms tailored to your goals. This involves training and tuning models based on the prepared data, optimizing parameters to enhance performance. After creating your models, it’s crucial to conduct rigorous testing and validation to assess their accuracy and reliability. Utilize defined performance metrics to evaluate how well your models perform in real-world scenarios. This iterative process of providing feedback and making improvements will ultimately refine the predictive capabilities of your AI agents and ensure they meet strategic goals effectively.
Step 1: Data Collection and Preparation
Effective data collection and preparation is crucial in the journey of Predictive Strategy Training. The first step involves identifying relevant data sources that will provide the insights needed to inform AI agents. These sources can range from customer feedback, sales calls, and surveys to large datasets from industry reports. By ensuring the data is diverse and rich, we lay a solid foundation for the AI models that will eventually use this information.
Once the data sources are identified, the next task is preprocessing and cleaning the data. This step ensures that the data is accurate, consistent, and free from errors or biases, which can compromise the training quality. Techniques—like filtering out irrelevant information and correcting inconsistencies—are vital in transforming raw data into a refined dataset ready for analysis. Properly prepared datasets enhance the performance of predictive models, leading to more reliable outcomes for strategic decision-making.
- Identifying Relevant Data Sources
Finding suitable data sources is crucial for effective predictive strategy training. Start by identifying internal data, like sales records and customer interactions, that can provide deep insights into your operations. Next, consider external sources such as market trends, social media analytics, and competitor performance data. Compiling this information will pave the way for a robust training framework for your AI agents.
In addition to core business data, you can enhance your insights by integrating specialized datasets. These might include demographic data, industry reports, and customer feedback. Each type of data leads to a more nuanced understanding of market dynamics and consumer behavior. By focusing on a diverse array of sources, you can improve the predictive capabilities of your AI agents significantly, ensuring they are well-equipped to navigate future challenges effectively.
- Preprocessing and Cleaning Data
Preprocessing and cleaning data is a crucial step in predictive strategy training. This stage involves preparing the dataset to ensure it is free from errors, inconsistencies, and irrelevant information. The objective here is to enhance data quality, which directly influences the performance of AI models. A well-prepared dataset allows predictive models to learn effectively, leading to more accurate forecasts and strategic insights.
To achieve effective preprocessing, a few key actions must be performed. First, remove duplicate entries that may skew the results during analysis. Secondly, deal with missing values by either filling them in or removing the affected records. Finally, standardize data formats to maintain uniformity across the dataset. These steps collectively contribute to creating a solid foundation for successful predictive strategy training, enabling AI agents to yield reliable and actionable insights.
Step 2: Developing Predictive Models
Developing predictive models is a crucial phase in Predictive Strategy Training. This process begins with selecting the right algorithms that best fit the data and the specific objectives of your strategic intelligence tasks. Various machine learning algorithms, including decision trees and neural networks, can be employed to capture underlying patterns within data. Selecting an algorithm requires understanding your data's features and the insights you aim to extract.
Once you’ve selected suitable algorithms, the next step involves training and tuning your models. This is the phase where the models learn from historical data, and adjustments can be made to enhance their accuracy. Techniques such as cross-validation help in ensuring that the model generalizes well to unseen data. Throughout this stage, continuous monitoring and iterative refinement are necessary to adapt to changing variables or new insights, ultimately improving the predictive capacity of your AI agents.
- Selecting the Right Algorithms
In the realm of Predictive Strategy Training, selecting the right algorithms is a pivotal step in shaping effective AI agents. The algorithms you choose will determine how your model interprets data and makes predictions. Commonly used algorithms include decision trees, random forests, and neural networks, each offering unique strengths suited to different types of data and problems. Understanding the specifics of the data at hand, such as its structure and the relevant features, will significantly influence your choice of algorithm.
💬 Questions about How to Train AI Agents for Predictive Strategic Intelligence?
Our team typically responds within minutes
Furthermore, it's essential to consider the intended outcomes during the selection process. Algorithms must not only fit the current dataset but also align with the strategic goals of the project. Evaluating the performance of chosen algorithms through metrics like accuracy and recall will further aid in fine-tuning your models. With a thoughtful selection process, you can enhance the predictive capabilities of your AI agents and ultimately drive strategic decisions that align with your organizational objectives.
- Training and Tuning Models
Training and tuning models is a critical aspect of predictive strategy training for AI agents. In this phase, the selected algorithms undergo rigorous adjustments and enhancements to improve their predictive capabilities. Proper tuning involves fine-tuning hyperparameters to ensure optimal performance based on the data provided. This practice enables the AI agent to adapt better to the nuances of the training data, leading to more accurate predictions.
The process begins with setting clear objectives, followed by an iterative cycle of training and testing. Feedback from performance metrics allows data scientists to make informed decisions about changes needed in their models. Techniques, such as cross-validation, can highlight areas for improvement and guide the tuning process. Additionally, employing tools that automate aspects of model training can enhance efficiency and consistency. Ultimately, effective training and tuning models are indispensable in creating robust AI systems capable of delivering valuable strategic insights.
Step 3: Testing and Validation
Testing and validation are critical stages in the Predictive Strategy Training of AI agents. During this phase, it's essential to evaluate how well the models have learned from the data. This ensures that the predictions made by the AI align with expected outcomes in real-world scenarios. Establishing clear performance metrics allows trainers to gauge the efficiency and accuracy of the models effectively.
To conduct a thorough testing and validation process, consider several essential strategies. First, utilize split datasets, where one part is used for training and another for testing to avoid bias. Second, employ cross-validation techniques to verify the model's robustness across diverse datasets, which can reveal its generalization capability. Lastly, integrate feedback mechanisms to iteratively improve model performance. By continuously refining the model based on these results, you enhance its predictive capabilities and ensure its reliability in strategic intelligence applications.
- Performance Metrics and Evaluation
Performance metrics and evaluation play a pivotal role in predictive strategy training, ensuring the effectiveness of AI agents. Assessing the performance of these agents begins with defining clear, measurable objectives aligned with strategic goals. This enables trainers to systematically evaluate how well the models perform in real-world scenarios and how effectively they leverage data to make informed predictions.
In this context, two essential evaluation components emerge. First, quantitative metrics such as accuracy, precision, and recall provide insights into the model's performance. They allow for a data-driven comparison of various models and help identify areas needing improvement. Secondly, qualitative assessments, such as user satisfaction and decision-making quality, offer a comprehensive view of the agents' practical impact. By continuously iterating on these performance metrics, trainers can refine predictive strategies, ensuring that the agents adapt to evolving market conditions and produce actionable insights.
- Iterative Feedback and Improvement
In the realm of Predictive Strategy Training, iterative feedback and improvement serve as vital processes. This approach emphasizes continuous refinement of AI agents by leveraging insights gained from each training cycle. By systematically evaluating the performance of predictive models, organizations can identify strengths and weaknesses, ensuring that the agents evolve in alignment with strategic objectives.
To effectively implement iterative feedback, several steps are crucial. First, establish clear performance metrics that assess the accuracy and relevance of AI outputs. Second, collect feedback from users and stakeholders to gain insights into areas requiring enhancement. Finally, integrate this feedback into the training cycle, adjusting algorithms and methodologies accordingly. This loop of evaluation and enhancement fosters an environment where AI agents steadily adapt, resulting in superior predictive capabilities tailored to strategic needs. Embracing these principles can lead to more reliable and effective AI-driven insights, ultimately benefiting organizational decision-making.
Essential Tools for Predictive Strategy Training
To effectively implement Predictive Strategy Training, selecting the right tools is essential. The landscape of AI has evolved significantly, offering various platforms designed to streamline the training process. These tools empower organizations to enhance their data analysis and modeling capabilities while democratizing accessibility for all team members.
A few of the top tools include insight7, DataRobot, H2O.ai, Google AI Platform, and IBM Watson. Each of these platforms brings unique features that support different aspects of Predictive Strategy Training. For instance, insight7 excels in providing an intuitive interface for analyzing customer insights rapidly. DataRobot focuses on automating machine learning, enabling users to build models efficiently without deep programming knowledge. H2O.ai is known for its open-source options and scalability, while Google AI Platform and IBM Watson offer robust cloud-based solutions with advanced analytics capabilities.
Adopting these tools facilitates a collaborative approach to data analysis, allowing teams to draw insights that drive strategic decisions. Whether you need to evaluate vast datasets or create sophisticated predictive models, having the right tools can transform your predictive strategy efforts.
Top Tools for Training AI Agents
Successful training of AI agents hinges on the tools utilized during the process. Using the right technology can significantly enhance Predictive Strategy Training, allowing for efficient model development and deployment. Key tools that stand out include DataRobot and H2O.ai, both known for their user-friendly interfaces and powerful machine learning capabilities. These platforms streamline the training process by automating much of the work involved, from data preparation to model evaluation.
Additionally, cloud-based solutions like Google AI Platform and IBM Watson provide scalable environments for AI training, ensuring that resources can be allocated efficiently. Insight7 offers specialized analytics features, enabling deeper insights into customer behavior, crucial for formulating effective predictive strategies. Integrating these tools not only optimizes training efforts but also enhances the overall performance of AI agents in predictive strategic contexts. By choosing the right tools, organizations can pave the way for more accurate and actionable insights.
- insight7
In the realm of predictive strategy training, understanding the nuances of data analysis is paramount. AI agents thrive on the wealth of information gleaned from customer conversations and market interactions. However, as companies face a deluge of customer signals, the traditional analytical methods fall short in fostering timely, actionable insights. Organizations must shift toward advanced data analysis techniques to maintain a competitive edge.
A systematic approach to predictive strategy training begins with effective data collection and preparation. This ensures that relevant and clean data fuels the AI models, allowing for precise predictions. Following this, developing robust predictive models involves selecting suitable algorithms and continually refining them based on performance metrics. With this framework, businesses can transform insights from sprawling datasets into strategic decisions, ultimately driving their growth. By prioritizing these steps, firms can successfully cultivate AI agents capable of delivering predictive strategic intelligence that aligns with real-time market demands.
- DataRobot
DataRobot serves as a pivotal tool in the journey of Predictive Strategy Training for AI agents. It provides a user-friendly interface for building and deploying machine learning models. By automating various aspects of model training, users can focus on formulating insights rather than just on the technicalities of algorithm development. This platform enables practitioners to easily access diverse algorithms, optimizing their predictions based on specific use cases.
The effectiveness of Predictive Strategy Training is significantly enhanced through the use of sophisticated tools like DataRobot. Model selection and performance tuning are simplified, allowing users to experiment with numerous strategies swiftly. The platform offers valuable capabilities, including automated feature engineering and model evaluations, fostering an environment where data-driven decisions can thrive. Emphasizing these automated features is integral, as they streamline the workflow, making transformative insights more accessible.
- H2O.ai
H2O.ai is a powerful tool for enhancing predictive strategy training in AI agents. By providing advanced machine learning capabilities, it simplifies the process of developing robust predictive models. This platform offers automated machine learning, enabling users to build, test, and deploy models with ease. The intuitive interface reduces barriers, making it accessible even to those with limited technical expertise.
In the context of predictive strategy training, H2O.ai stands out for its ability to handle large datasets efficiently. It supports various data formats and integrates seamlessly with multiple data sources, further enriching the training process. Additionally, the platform’s focus on transparency ensures that users can understand the decision-making processes behind their models. Utilizing H2O.ai not only accelerates the training timeline of AI agents but also enhances their predictive accuracy, ultimately leading to more informed strategic choices.
- Google AI Platform
The Google AI Platform serves as a powerful resource for implementing Predictive Strategy Training for AI agents. It offers a user-friendly interface designed to accommodate individuals across various skill levels, enabling businesses to democratize access to advanced AI capabilities. With this platform, users can quickly access tools that help transform unstructured data into actionable insights.
One of the standout features is its ability to process large volumes of data and extract critical insights efficiently. By organizing and analyzing multiple datasets, businesses can identify key trends and areas that require attention. Moreover, the platform supports collaborative workflows, ensuring that teams can work together seamlessly to develop predictive models. Such capabilities are essential for businesses aiming to refine their strategies based on customer feedback and evolving market dynamics. In this manner, the Google AI Platform becomes an essential partner in the journey of improving Predictive Strategy Training for AI agents.
- IBM Watson
IBM Watson exemplifies a pinnacle of advanced technology, particularly within the realm of predictive strategy training. This sophisticated platform employs cutting-edge algorithms to analyze vast datasets and derive actionable insights. As organizations strive to enhance their strategic capabilities, utilizing such technologies can significantly improve decision-making processes.
The platform's strengths lie in its ability to process unstructured data and integrate machine learning techniques. By training AI agents through data-driven methodologies, organizations can better predict trends and make informed decisions. Additionally, it allows users to customize models designed to meet specific industry needs, ensuring the focus remains on achieving strategic objectives. Through effective predictive strategy training, organizations can harness the power of AI, transforming data into valuable insights that drive success.
Conclusion: Future of Predictive Strategy Training in AI
The future of Predictive Strategy Training in AI looks promising as technology evolves. Enhancements in data processing, machine learning algorithms, and simulation techniques will refine how AI agents predict outcomes. These advancements will empower organizations to make informed decisions, adapting strategies based on accurate insights.
As AI capabilities grow, the role of predictive training will become more crucial. Training methodologies must remain flexible and responsive to new data types, ensuring AI agents receive comprehensive education. This evolution will foster more intelligent, consultative interactions in various fields, pointing toward a future where AI and human collaboration drives strategic excellence.
💬 Questions about How to Train AI Agents for Predictive Strategic Intelligence?
Our team typically responds within minutes