How to Train AI Agents for Predictive Qualitative Analysis
-
Bella Williams
- 10 min read
Predictive AI Training serves as a cornerstone in modern qualitative analysis, transforming how organizations derive insights from complex data. In an era where information is abundant, understanding how to effectively train AI agents is crucial for any business looking to harness qualitative data. By leveraging the strengths of AI, companies can identify patterns, generate insights, and make data-driven decisions that were once unfeasible.
The process begins with understanding both the potential and limitations of AI in qualitative analysis. Predictive AI training enables agents to interpret not just numbers but the narratives behind them, enabling a deeper understanding of consumer behavior. This foundational knowledge is essential for any organization aiming to navigate the intricacies of qualitative data successfully. Grasping these basics sets the stage for a more sophisticated approach to leveraging qualitative insights for strategic decision-making.
Analyze & Evaluate Calls. At Scale.
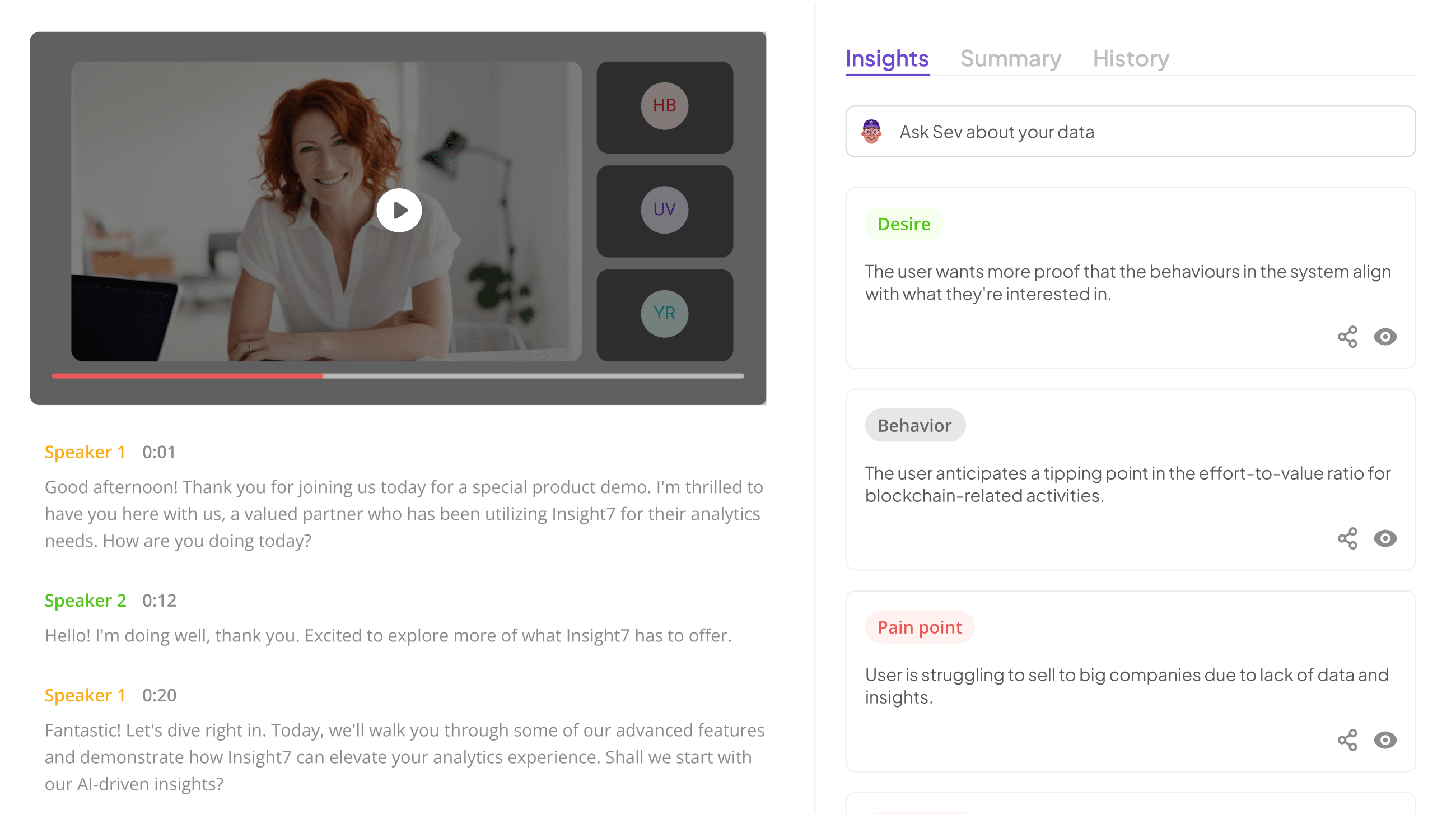
Understanding the Fundamentals of Predictive AI Training
Predictive AI Training serves as a crucial tool for harnessing the power of data in qualitative analysis. Understanding its fundamentals begins with recognizing the importance of specificity in training models. Being clear in your objectives allows AI agents to function effectively, minimizing instances of inaccuracies or "hallucinations," which can arise from vague guidelines.
To effectively implement predictive AI training, it's essential to focus on several key areas. First, define your goals and understand the specific insights you require. Next, gather and preprocess relevant data, ensuring it's clean and suitable for analysis. Then, select algorithms that align with your objectives. Finally, continuously train, evaluate, and refine your models to adapt to changing data landscapes. By emphasizing these foundational aspects, businesses can greatly enhance their decision-making processes and gain valuable qualitative insights.
The Intersection of AI and Qualitative Insights
In today's world, the integration of AI in qualitative insights has become paramount for effective decision-making. Predictive AI training enables organizations to sift through vast amounts of unstructured data, transforming customer conversations into actionable insights. This intersection fosters a deep understanding of consumer behavior, allowing businesses to adapt quickly to changing market demands.
Furthermore, leveraging AI in qualitative analysis streamlines the process, making it more efficient and less time-consuming. Traditional methods often lead to scattered information and delayed responses. By combining advanced algorithms with qualitative research techniques, organizations can engage more meaningfully with their data. This synergy not only enhances the accuracy of insights but also fosters innovation and helps businesses stay ahead in competitive landscapes. As companies embrace this approach, they find that timely, data-driven decisions are crucial in creating strategic advantages.
Why Predictive AI Training is Essential for Businesses
Predictive AI Training is vital for businesses aiming to enhance analytical capabilities and decision-making processes. With an abundance of data available, companies must develop predictive models that can identify patterns, trends, and customer needs. This type of training equips AI agents to analyze qualitative data more effectively, allowing organizations to gauge consumer behavior and preferences. By implementing targeted training programs, businesses can boost the accuracy of forecasts and improve their operational efficiency significantly.
Moreover, Predictive AI Training fosters a proactive approach to market dynamics, helping businesses adapt swiftly to emerging trends. This adaptability not only strengthens competitive positioning but also drives innovation by uncovering new opportunities. Furthermore, empowering AI agents through comprehensive training ensures consistency and reliability in analysis, which is crucial for strategic decision-making. In summary, investing in Predictive AI Training is essential for businesses seeking to thrive in today's rapidly evolving market environment.
Tools and Techniques for Effective Predictive AI Training
Mastering tools and techniques is critical for effective predictive AI training, which allows organizations to harness qualitative insights for informed decision-making. To start, itโs essential to identify key tools that facilitate predictive analysis. Some of the most valuable tools include platforms like IBM Watson, Google Cloud AI, and DataRobot, which provide powerful features for data analysis and model deployment.
Next, implementing a structured approach ensures that the training process is efficient and effective. Initially, clear objectives should be defined to guide the training procedure. This is followed by thorough data collection and preprocessing to ensure quality inputs for model training. Choosing the right algorithms is equally important, as they dictate how the data will be interpreted. Finally, continuous evaluation and optimization of the models help refine accuracy, making predictive AI more robust in uncovering insights. By employing these strategies, organizations can significantly enhance their predictive qualitative analysis capabilities.
Extract insights from interviews, calls, surveys and reviews for insights in minutes
Key Tools for Predictive Qualitative Analysis
In the realm of predictive qualitative analysis, various tools play an essential role in enhancing the efficacy of predictive AI training. These tools enable practitioners to aggregate, analyze, and interpret qualitative data, leading to actionable insights that inform decision-making. Each of these tools offers unique features tailored to specific analytical needs, making it crucial to select the right one based on the project requirements.
The first tool worth noting is insight7, which excels in organizing and visualizing qualitative data. This platform streamlines the process of analyzing customer feedback, making it straightforward to extract valuable insights from large datasets. IBM Watson provides robust AI capabilities, allowing for advanced sentiment analysis and pattern recognition across diverse qualitative datasets. Similarly, Google Cloud AI offers powerful machine learning models capable of training on various qualitative inputs. RapidMiner and DataRobot are excellent options for those seeking to build, train, and optimize predictive models efficiently. Utilizing these key tools effectively will significantly enhance the predictive capabilities of AI agents, promoting better outcomes in qualitative analysis.
- insight7
Training AI agents for predictive qualitative analysis requires a nuanced understanding of data interpretation and insight extraction. Insight7 emphasizes the importance of asking the right questions and employing advanced AI technologies to uncover patterns in customer conversations. Through effective predictive AI training, agents are better equipped to analyze complex datasets, ultimately enhancing the decision-making process.
To train these AI agents successfully, consider the following steps:
Define Clear Objectives: Establish what you want to achieve with predictive AI. Clear goals ensure that the training process remains focused and effective.
Collect and Preprocess Data: Gather diverse qualitative data from customer interactions. Proper preprocessing helps the AI understand context and sentiment, which are crucial for generating reliable insights.
Choose Appropriate Algorithms: Select algorithms that align with your analysis objectives. Understanding the strengths and weaknesses of each algorithm is essential for successful predictive AI training.
Train, Evaluate, and Optimize Models: Continuously train the models with new data, evaluate their performance, and optimize based on feedback. This iterative process fosters improvement and adaptability in understanding customer needs.
These steps create a solid foundation that enables organizations to leverage predictive AI training, transforming raw data into actionable insights that drive business strategy.
- IBM Watson
IBM Watson offers advanced capabilities for predictive AI training, enabling businesses to transform qualitative analysis. By utilizing natural language processing and machine learning, it can identify patterns in large datasets, uncovering valuable insights from unstructured text. This technology allows users to efficiently analyze customer interactions, leading to more informed decision-making.
The process begins with training AI models on specific datasets, tailored to unique business needs. First, users define clear objectives, focusing on what insights they are seeking. Next, data is collected and preprocessed to ensure quality inputs for model development. Selecting appropriate algorithms follows, with options suited for various analytical tasks. Finally, the models undergo training, evaluation, and optimization, enhancing their predictive accuracy. Deploying such tools in predictive AI training empowers organizations to leverage qualitative data effectively, enhancing their overall analytical capabilities.
- Google Cloud AI
Google Cloud AI offers a comprehensive suite of tools specifically designed to enhance predictive AI training. It simplifies the process of building, training, and deploying models that can efficiently analyze qualitative data. By leveraging powerful machine learning capabilities, users can effectively mine insights from diverse data sources, enabling more informed decision-making.
To harness these features successfully, users should consider the following key aspects:
Data Ingestion: Start by integrating your data from various sources into the platform. This ensures that you have a comprehensive dataset for training your models.
๐ฌ Questions about How to Train AI Agents for Predictive Qualitative Analysis?
Our team typically responds within minutes
Model Training and Optimization: Utilize advanced algorithms to train your predictive models. Regularly evaluate their performance to fine-tune accuracy.
Real-time Insights: Once your models are deployed, leverage real-time analysis features. This allows for immediate insights that can influence strategic decisions.
Utilizing these facets can effectively enhance the capabilities of your AI agents in predictive qualitative analysis.
- RapidMiner
RapidMiner is a powerful tool that plays a significant role in predictive AI training. This platform aids users in transforming qualitative data into actionable insights. By utilizing its intuitive interface, you can effortlessly manage projects that compile various data sources, such as audio files and transcripts. Analyzing customer feedback becomes streamlined, allowing insights to emerge from large datasets swiftly.
In this environment, itโs possible to employ different analysis methods to identify trends and patterns among collected responses. The built-in functionalities simplify the process of summarizing results, enabling quick decision-making based on analytical findings. With RapidMiner, organizations can significantly enhance the efficiency of their predictive qualitative analyses. Its user-friendly nature makes it accessible for both novice users and experienced analysts, ensuring valuable insights can be gathered and utilized effectively. Mastering this tool positions teams to excel in predictive AI training initiatives.
- DataRobot
DataRobot serves as a significant tool in the realm of predictive AI training, enabling users to enhance qualitative analysis capabilities. By streamlining the process of building and deploying machine learning models, it allows analysts to focus on extracting meaningful insights without the burden of extensive technical expertise. The platform automates many aspects of model selection, evaluation, and optimization, making it easier to generate results quickly.
To effectively utilize DataRobot, users should follow a structured approach. First, clear objectives must be defined to guide the analysis. Next, relevant data should be collected and preprocessed to ensure quality inputs. After establishing the data foundation, appropriate algorithms can be selected based on the specific analytical goals. Finally, the models can be trained, evaluated, and optimized for accuracy and effectiveness. This comprehensive methodology empowers organizations to leverage predictive AI training successfully, leading to more informed decision-making.
Step-by-Step Guide to Implementing Predictive Models
Implementing predictive models is a structured process that empowers businesses to harness insights effectively. The first step involves defining clear objectives to outline what you want the predictive model to achieve. These objectives help determine the data needed and the analysis approach, guiding the entire project.
Next, it's crucial to collect and preprocess your data rigorously. This step involves cleaning and organizing your data so it is suitable for analysis. Afterward, youโll choose appropriate algorithms based on your objectives and data type. Finally, train, evaluate, and optimize the models to ensure they yield the most accurate predictions. Each of these steps is fundamental in the Predictive AI Training process, making it essential for businesses focused on qualitative analysis. By applying these guidelines, you can maximize the effectiveness of deploying predictive models in your organization.
- Step 1: Define Clear Objectives
Establishing clear objectives is the foundational step in the journey of predictive AI training. Initially, itโs essential to pinpoint what specific qualitative insights need to be extracted from the data. This clarity helps in guiding the subsequent phases of the training process. Without well-defined goals, the entire training effort can become directionless and ineffective, ultimately yielding unsatisfactory results.
To ensure success, consider the following key objectives:
Identify Core Questions: Determine the specific qualitative variables of interest, such as customer sentiment or behavioral trends.
Set Clear Outcomes: Define what success looks like in measurable terms, like accuracy or relevance of insights.
Align with Business Needs: Ensure that your objectives are in sync with broader organizational goals to maximize the impact of your predictive models.
By taking the time to clearly define objectives, you set a strong foundation for the predictive analysis to follow, facilitating a more structured and effective training approach.
- Step 2: Collect and Preprocess Data
The second step in Predictive AI Training involves the critical processes of data collection and preprocessing. Data is the foundation of AI models, and its quality directly impacts performance. Begin by identifying relevant sources that feed meaningful data into your system. This may include customer feedback, market trends, and behavioral insights. Collecting diverse data enhances the robustness of your AI's predictive capabilities.
Once you've gathered the data, preprocessing becomes essential. This involves cleaning the data to remove inconsistencies and missing values. You should also standardize formats to ensure compatibility with your AI tools. Additionally, consider employing techniques like normalization and categorization to refine the dataset. By curating and refining the data, you ensure that your AI agent can accurately interpret trends and derive insights, ultimately enhancing its predictive accuracy. Thus, the effective collection and preprocessing of data are vital for successful Predictive AI Training.
- Step 3: Choose Appropriate Algorithms
Choosing appropriate algorithms is crucial in your journey towards effective predictive AI training. With a plethora of options available, the task can initially seem overwhelming. However, understanding the nature of your qualitative data and the specific insights you aim to extract simplifies this process. Start by assessing different algorithms such as decision trees, support vector machines, and neural networks, each offering unique advantages depending on your data's characteristics.
Next, consider the context of your analysis. For instance, if you're focusing on customer feedback, natural language processing models can yield valuable insights into sentiments and themes. Itโs also important to account for scalability, interpretability, and computational efficiency, as these factors significantly impact your modelโs performance. By methodically evaluating these algorithms, you empower your AI agents to enhance predictive accuracy and contribute meaningfully to qualitative analysis efforts. The right choice will not only drive insightful outcomes but also solidify the foundation of your predictive qualitative strategy.
- Step 4: Train, Evaluate, and Optimize Models
In this critical phase of the process, we focus on training, evaluating, and optimizing models, a cornerstone of predictive AI training. Initially, training the models involves inputting the preprocessed data into selected algorithms. This step is crucial as it establishes the foundation for how well the AI can learn from historical data. By ensuring diverse and representative training data, you enhance the model's ability to make accurate predictions in real-life situations.
Once the models are trained, evaluation becomes necessary to ascertain their performance. This involves using metrics such as accuracy, precision, and recall to gauge how effectively the models analyze qualitative data. Finally, optimizing the models may involve fine-tuning parameters and adjusting algorithms based on performance feedback. This iterative process enhances model reliability, ultimately leading to more informed decision-making and actionable insights in qualitative analysis.
Conclusion: Enhancing Decision-Making Through Advanced Predictive AI Training
Effective decision-making is critical in today's fast-paced environment, and Predictive AI Training can significantly enhance this process. By integrating advanced AI techniques, organizations can analyze qualitative data more accurately and derive actionable insights. These insights empower decision-makers to craft strategies that are not only informed but also adaptable to changing circumstances.
Moreover, the continuous improvement of AI agents through rigorous training allows for more nuanced understanding and responsiveness in various scenarios. As organizations refine their Predictive AI Training processes, they can expect to see improved outcomes and better alignment with customer needs. This ultimately fosters a more agile organizational culture, capable of navigating complexities with confidence.
๐ฌ Questions about How to Train AI Agents for Predictive Qualitative Analysis?
Our team typically responds within minutes