In today's fast-paced research environment, Interview Analysis Automation is transforming qualitative research. Traditionally, researchers relied on manual methods to analyze interview data, which proved to be time-consuming. These conventional approaches often resulted in delayed insights and ineffective collaboration. With the rise of automated tools, researchers can now efficiently process vast amounts of interview data, uncovering valuable insights more quickly.
Implementing Interview Analysis Automation brings several advantages. It streamlines the analysis process, allowing researchers to focus on meaningful interpretations rather than tedious data management. As the demand for rapid and accurate insights grows, automated analysis provides a significant competitive edge, enabling researchers to translate their findings into effective strategies. As we delve deeper into this subject, we will explore practical steps and considerations to successfully train AI agents for interview analysis in qualitative research.
Analyze qualitative data. At Scale.
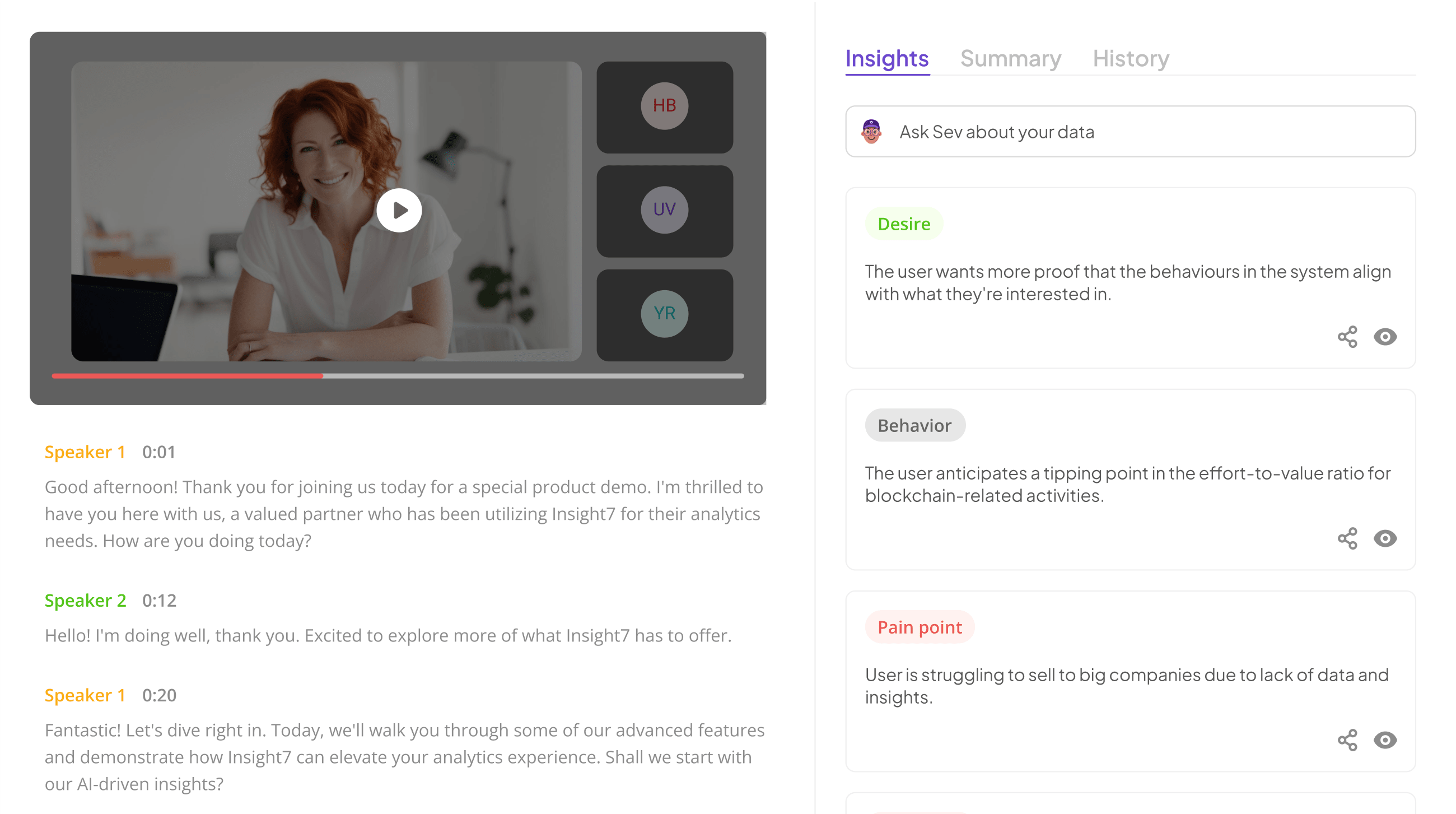
Understanding the Role of AI in Interview Analysis Automation
Artificial Intelligence plays a transformative role in interview analysis automation, altering how qualitative researchers extract insights from data. As researchers gather vast amounts of interview data, traditional analysis methods become increasingly inefficient and cumbersome. AI streamlines this process, enabling faster analysis and providing researchers with timely insights that can directly influence business strategies.
By automating repetitive tasks such as transcription and sentiment analysis, AI frees researchers to focus on interpreting and contextualizing results. Furthermore, AI's ability to identify patterns and trends across multiple interviews enhances the depth and reliability of qualitative research findings. However, the effectiveness of AI in interview analysis hinges on the quality of data and appropriate algorithm training. Recognizing both the advantages and the limitations of AI can empower researchers to harness its full potential in qualitative analysis, ultimately paving the way for informed decision-making in various sectors.
Benefits of AI in Qualitative Research
Artificial intelligence offers transformative benefits for qualitative research, particularly in interview analysis automation. By automating the analysis process, researchers can significantly reduce the time spent on data examination. Instead of manually sifting through hours of dialogue, AI can quickly identify key themes, sentiments, and trends in interview transcripts, allowing researchers to focus more on interpreting and contextualizing findings.
Furthermore, AI enhances consistency and objectivity in qualitative analysis. Human interpretation can be influenced by biases and emotional responses, but AI algorithms follow predetermined criteria, ensuring a uniform approach. This impartiality allows for more reliable insights across multiple interviews, leading to more credible outcomes. With these advantages, the utilization of AI in qualitative research presents a compelling opportunity for researchers seeking efficiency and accuracy in their work.
- Efficiency and Time-Saving
Training AI agents for interview analysis automation streamlines the qualitative research process, enhancing efficiency and saving valuable time. By utilizing automated systems, researchers can transcribe and analyze vast amounts of interview data with a few clicks. This technology eliminates the tedious manual effort traditionally required for data review and thematic coding, allowing researchers to focus more on interpreting results.
Furthermore, leveraging AI enables quicker insights generation. Users can input multiple interviews simultaneously, facilitating a comprehensive analysis of themes, pain points, and desires. Agents can swiftly summarize conversations, highlighting critical excerpts and evidence to support conclusions. This efficiency not only optimizes the research timeline but also enhances accuracy, ensuring all significant details are captured without bias. Ultimately, interview analysis automation transforms the way researchers approach qualitative studies, making the process faster and more effective.
- Enhanced Consistency and Objectivity
In qualitative research, enhanced consistency and objectivity is crucial for maintaining the integrity of interview analysis automation. Human judgment can introduce bias, leading to skewed results. By implementing AI agents, researchers can achieve greater standardization in evaluating responses, minimizing variability across analyses. This structured approach ensures that each interaction is assessed using defined criteria, contributing to fairness and repeatability in assessments.
Moreover, AI-powered systems can document evidence supporting evaluations, which directly counters subjective claims and reinforces objectivity. Rather than relying solely on personal interpretation, researchers receive data-backed insights that elucidate how conclusions were drawn. This transparency not only builds trust in the analysis but also facilitates discussions around performance, particularly in reference to soft skills like tone of voice and engagement tactics. Ultimately, this balance of automated insights and objective metrics empowers researchers to produce reliable and actionable findings in their qualitative studies.
Limitations and Challenges
In the realm of interview analysis automation, several limitations and challenges present significant hurdles. First, handling complex and nuanced data remains a primary concern. Interviews often contain subtleties such as emotional tone, context, and cultural references that automated systems may struggle to interpret accurately. Consequently, extracting meaningful insights can become a tedious task, requiring human oversight to ensure that critical information is not overlooked.
Another challenge is ensuring ethical standards during the automation process. Issues related to privacy, informed consent, and data security must be addressed. Using AI in qualitative research necessitates contemplation of these ethical considerations to foster trust among participants and researchers. Moreover, as the landscape of AI technology evolves, staying compliant with regulations surrounding data use adds another layer of complexity. Therefore, developing robust frameworks is essential for effective interview analysis automation while upholding ethical integrity.
- Handling Complex and Nuanced Data
Handling complex and nuanced data presents both opportunities and challenges in interview analysis automation. AI agents must accurately interpret various conversation dynamics, such as emotions, tone, and intention, which are often subtle. These factors can significantly influence the analysis and outcomes of qualitative research, making precision paramount.
To effectively manage this data, one can follow several strategies. Firstly, incorporate diverse datasets to enhance the AIโs learning experience. This includes transcripts from various contexts, enabling agents to adapt to different interview styles. Secondly, focus on iterative model training, allowing for continuous improvements based on feedback. Finally, utilize multi-dimensional analysis frameworks to better capture the richness of the data. These approaches not only streamline the analysis process but also ensure more reliable insights, ultimately enriching the research outcomes.
- Ensuring Ethical Standards
To achieve successful interview analysis automation, maintaining ethical standards in AI training is paramount. Firstly, ensuring informed consent from participants is crucial. Researchers must transparently communicate how their data will be used and require explicit permission to record or analyze interviews. This practice builds trust and aligns with ethical guidelines.
Secondly, protection of sensitive information is essential. AI models must be trained to recognize and handle confidential data, such as personal identifiers, social security numbers, and any sensitive content. Implementing data anonymization techniques can safeguard participants' identities. Finally, continuous monitoring of AI outputs is necessary to counteract biases. Regular audits should ensure AI models operate within established ethical boundaries, fostering trustworthy and responsible research practices.
Extract insights from interviews, calls, surveys and reviews for insights in minutes
Steps to Train AI Agents for Interview Analysis Automation
Training AI agents for interview analysis automation involves strategic and structured steps to ensure high-quality outcomes. The first step is data collection and preprocessing. This involves gathering a representative sample of interview data, which must then be cleaned and annotated to improve accuracy. Proper annotation should highlight key elements such as speaker identity, sentiment, and thematic content. This foundation is crucial as it directly impacts the effectiveness of the AI models.
Next, the focus shifts to designing and implementing AI algorithms. Selecting the right machine learning models is vital, as different models may yield varied results based on the intricacies of qualitative data. Following this, fine-tuning the models enhances their ability to identify patterns and insights from the interviews. By meticulously following these steps, researchers can effectively harness interview analysis automation, making the qualitative research process more efficient and insightful.
Step 1: Data Collection and Preprocessing
The first step in training AI agents for interview analysis automation involves meticulous data collection and preprocessing. This foundational stage determines the quality of insights generated by the AI. Begin by gathering representative interview data, ensuring that it captures diverse perspectives relevant to your research objectives. This step is critical because diverse data enhances the AI's understanding of various viewpoints.
Once the data is collected, the focus shifts to cleaning and annotating the text. Cleaning involves removing irrelevant information or errors that could skew analysis results. Annotation, on the other hand, entails tagging data with codes or labels to facilitate machine learning processes. Both actions allow the AI to learn patterns and nuances within the qualitative data, setting the stage for more effective analysis and interpretation. Properly executed, these preparatory steps pave the way for successful interview analysis automation.
- Gathering Representative Interview Data
Gathering representative interview data is crucial for effective interview analysis automation. This process aims to collect varied perspectives and insights from diverse participants, allowing AI agents to develop a comprehensive understanding of the subject matter. To ensure that the data gathered is representative, researchers should aim for a balanced sample. This means including participants from different demographics, industries, and experience levels. Well-rounded data enables the AI to better identify patterns and nuances within the interviews.
Once the data is collected, it must be carefully cleaned and annotated. This involves removing any irrelevant information and highlighting key themes. An appropriately annotated dataset enhances the AI's ability to learn accurately from the interviews. The importance of gathering representative interview data lies not only in the quantity but also in the quality of insights derived. By focusing on inclusivity and thoroughness, researchers can significantly improve the outcomes of their qualitative research using interview analysis automation.
- Cleaning and Annotating Text
Cleaning and annotating text are vital steps in preparing qualitative data for AI-driven interview analysis automation. Initially, this involves removing any irrelevant or extraneous content from transcripts. By focusing on the core content, researchers can effectively streamline the data, ensuring that the AI can analyze it more efficiently. This phase is crucial because clear and organized data directly influences the accuracy of later analyses.
Next, annotation plays a prominent role in enhancing data comprehension. It includes identifying themes, coding responses, and adding metadata to each entry, which helps the AI understand context and nuances better. Annotated texts lay a strong foundation for building AI models that can discern patterns and insights from the data.
Ultimately, thorough cleaning and annotating are essential to achieve meaningful insights in interview analysis automation. By preparing your data well, you can maximize the effectiveness of AI in generating insights from your qualitative research.
Step 2: Designing and Implementing AI Algorithms
In this critical phase of designing and implementing AI algorithms for interview analysis automation, selecting the right machine learning models becomes paramount. Understanding the characteristics of your data will guide you in choosing the most suitable models tailored for your specific qualitative research needs. Each model has its strengths and weaknesses, often relating to the complexity and volume of the data inputs, thus ensuring clarity in defining your research goals is essential.
Once you select the appropriate models, the next step focuses on training and fine-tuning these algorithms. This involves feeding the model with preprocessed interview data, allowing it to learn patterns, themes, and nuances inherent in the responses. By employing iterative adjustments, you can enhance the algorithmโs accuracy and reliability, making it adept at handling the qualitative intricacies typical in interview contexts. Properly trained AI agents can significantly streamline and enhance your interview analysis process, offering valuable insights previously hampered by manual analysis.
- Selecting Appropriate Machine Learning Models
Selecting the right machine learning models is crucial for effective interview analysis automation. The choice of model significantly impacts the performance of AI agents tasked with interpreting qualitative data. Initially, consider the type of insights you aim to extract from your interviews, as this will guide your model selection. For instance, if youโre focusing on sentiment detection, natural language processing (NLP) models like BERT or LSTM can be highly effective.
Once youโve identified potential models, evaluate them based on their capabilities to handle the complexity and nuances of qualitative data. Additionally, balance model performance with interpretability, as stakeholders may require clear explanations of how insights are generated. Testing various models through cross-validation can help you determine which one offers the best trade-off between accuracy and efficiency. By strategically selecting machine learning models, you empower your interview analysis automation process, leading to richer insights and actionable outcomes.
- Training and Fine-tuning Models
Training AI agents for interview analysis automation involves a structured approach to model development. First, it is crucial to gather diverse and representative interview data. This data not only serves as the basis for analysis but also informs the AI models' understanding of language nuances and context. Preprocessing this data is equally essential, as it involves cleaning, annotating, and organizing it into a format suitable for training. This initial step sets the stage for effective model training and performance.
Once the data is ready, the focus shifts to designing and implementing AI algorithms. The key here is to select suitable machine learning models that align with the goals of interview analysis. Training these models requires iterative fine-tuning, where parameters are adjusted based on performance metrics. By constantly refining the models with new data and feedback, researchers can enhance their agents' ability to automate interview analysis more accurately and efficiently. This thorough training process ultimately yields AI systems that can deliver insightful and actionable analysis in qualitative research.
Top Tools for Interview Analysis Automation
When exploring top tools for interview analysis automation, several options stand out due to their effectiveness and user-friendliness. These tools enable researchers to analyze qualitative data from interviews efficiently. They help transform unstructured data into structured insights, making it easier to identify patterns and derive conclusions.
One prominent tool is Atlas.ti, which excels in visualizing complex relationships in qualitative data. Its robust features allow users to code interviews systematically, facilitating in-depth analysis. Another noteworthy option is NVivo, known for its powerful data organization capabilities. Researchers appreciate its ability to manage large datasets and perform sophisticated queries. Dedoose, with its cloud-based platform, promotes collaboration among teams working on qualitative research. Finally, MAXQDA stands out for its user-friendly interface and versatile analysis options, making it suitable for both beginners and experienced researchers.
Selecting the right tool can significantly enhance the efficiency of interview analysis automation, ultimately leading to more actionable insights.
insight7: Leading the Way in Automated Interview Analysis
Automated Interview Analysis is revolutionizing qualitative research by enabling researchers to process vast amounts of interview data quickly and effectively. This innovative approach addresses common pain points faced by organizations that manually analyze customer conversations. Instead of sifting through extensive text files, researchers can employ AI to uncover key insights rapidly, allowing them to focus on strategic decision-making.
With Interview Analysis Automation, the efficiency of qualitative research improves dramatically. AI systems can learn from diverse datasets, ensuring that they can recognize patterns and themes that might otherwise go unnoticed. By streamlining this process, organizations not only save valuable time but also enhance their ability to translate insights into actionable business strategies. As the demand for timely insights grows, automated interview analysis stands out as a critical tool for staying competitive in todayโs market.
Other Notable Tools
In the realm of interview analysis automation, various notable tools can enhance the efficiency and accuracy of qualitative research. These tools facilitate the systematic examination of interview data, saving time while maintaining high levels of quality. Among the prominent options, Atlas.ti stands out for its intuitive interface, allowing researchers to easily code qualitative data and uncover meaningful patterns.
Another significant player is NVivo, which offers advanced features for the management and analysis of qualitative data. Its robust capabilities enable researchers to conduct detailed queries and visualize their findings effectively. Dedoose, with its user-friendly design, simplifies collaborative analysis, making it ideal for team-oriented research efforts. Lastly, MAXQDA provides a comprehensive toolset for coding and analyzing qualitative data, with unique visualization options that aid in interpreting complex insights. Each of these tools plays a vital role in automating the intricate process of interview analysis, helping researchers derive actionable insights from their qualitative data.
- Atlas.ti
Atlas.ti offers a powerful platform specifically designed for qualitative research and interview analysis automation. This tool enhances the way researchers analyze and visualize data, simplifying the process of extracting insights from interviews. With its user-friendly interface, researchers can create structured projects that bring together various data inputs, such as audio transcripts and other qualitative materials.
Researchers can easily upload and manage files, facilitating collaboration and enhancing project efficiency. For instance, it allows users to query data and summarize responses seamlessly, revealing patterns and trends essential for comprehensive analysis. These capabilities significantly streamline the process of interview analysis automation, enabling researchers to focus on interpreting insights rather than getting bogged down in data organization. In turn, this fosters a deeper understanding of qualitative data while ensuring the rigor and reliability of research findings.
- NVivo
In qualitative research, NVivo serves as a powerful tool for interview analysis automation. With its robust project management features, researchers can conveniently organize and analyze numerous audio and textual data. As you begin to compile interviews, creating a new project simplifies the process, allowing for seamless data uploads from various sources like your computer or cloud services. This streamlined workflow enhances the overall efficiency of data analysis, enabling researchers to focus on deriving insights rather than managing data logistics.
Additionally, NVivo's analytical capabilities allow users to visualize patterns and trends effectively. As each interview is transcribed and entered into the project, researchers can employ matrix analyses to extract specific responses to targeted questions. This functionality fosters a clear understanding of participant feedback, helping identify themes across multiple interviews. Ultimately, NVivo stands out as a vital asset in the toolkit for those seeking to automate their interview analysis and improve qualitative research outcomes.
- Dedoose
Dedoose serves as a vital tool in the quest for effective interview analysis automation. By offering a user-friendly platform, it supports qualitative researchers in organizing and analyzing data seamlessly. The software allows for collaboration among team members, enabling diverse perspectives to enhance the analysis process. This collaborative approach is particularly useful in identifying themes and patterns that may otherwise go unnoticed in traditional research settings.
Moreover, Dedoose simplifies the integration of multimedia files, such as audio and video recordings, into the analysis. This capability enriches the data context, allowing researchers to retrieve deeper insights from interviews. As AI technologies continue to evolve, platforms like this play a crucial role in transforming how qualitative data is processed and understood, paving the way for more refined and insightful interview analysis automation.
- MAXQDA
MAXQDA plays a significant role in interview analysis automation, particularly in qualitative research. This tool enhances the efficiency of data handling, enabling researchers to aggregate and analyze large volumes of qualitative data with ease. Researchers can create projects that bring multiple interviews together, effectively synthesizing insights and identifying trends. This functionality allows teams to focus on deeper analysis rather than getting bogged down in data management.
Furthermore, MAXQDA provides tools for transcribing audio files and importing data from various sources, making it easier to centralize and organize interview materials. By utilizing matrix analysis, users can quickly extract critical insights from numerous transcripts based on targeted questions. This innovative approach not only streamlines the analysis process but also ensures that findings are presented in a structured, easily interpretable manner. As such, incorporating MAXQDA into your workflow can significantly enhance the quality and speed of interview analysis automation in qualitative research.
Conclusion: Enhancing Qualitative Research with Interview Analysis Automation
Automating interview analysis can significantly transform qualitative research practices. By incorporating advanced technology, researchers can efficiently process large volumes of qualitative data, enabling them to extract actionable insights swiftly. This automation addresses the challenges often faced in traditional methods, such as time-consuming manual analysis and scattered information, allowing for more cohesive collaboration among teams.
Furthermore, interview analysis automation not only enhances efficiency but also supports greater consistency and objectivity in data interpretation. As a result, researchers can focus on strategic decision-making rather than spending excessive time on data management. Ultimately, integrating interview analysis automation presents a valuable opportunity for researchers to advance their qualitative methodologies, leading to meaningful insights that drive informed actions and strategies.