Abandonment Pattern Recognition is a crucial skill in understanding user behavior during interviews. Imagine sifting through a wealth of insights, only to realize that key signs of abandonment slipped through your fingers. Recognizing these patterns can transform how you engage with users and refine your product offerings.
In this section, we will explore the significance of spotting abandonment patterns within interview transcripts. Understanding how to identify these indicators helps uncover the reasons behind user disengagement, ensuring a more informed approach to product development. Through effective recognition techniques, you can enhance the overall user experience and drive retention.
Analyze qualitative data. At Scale.
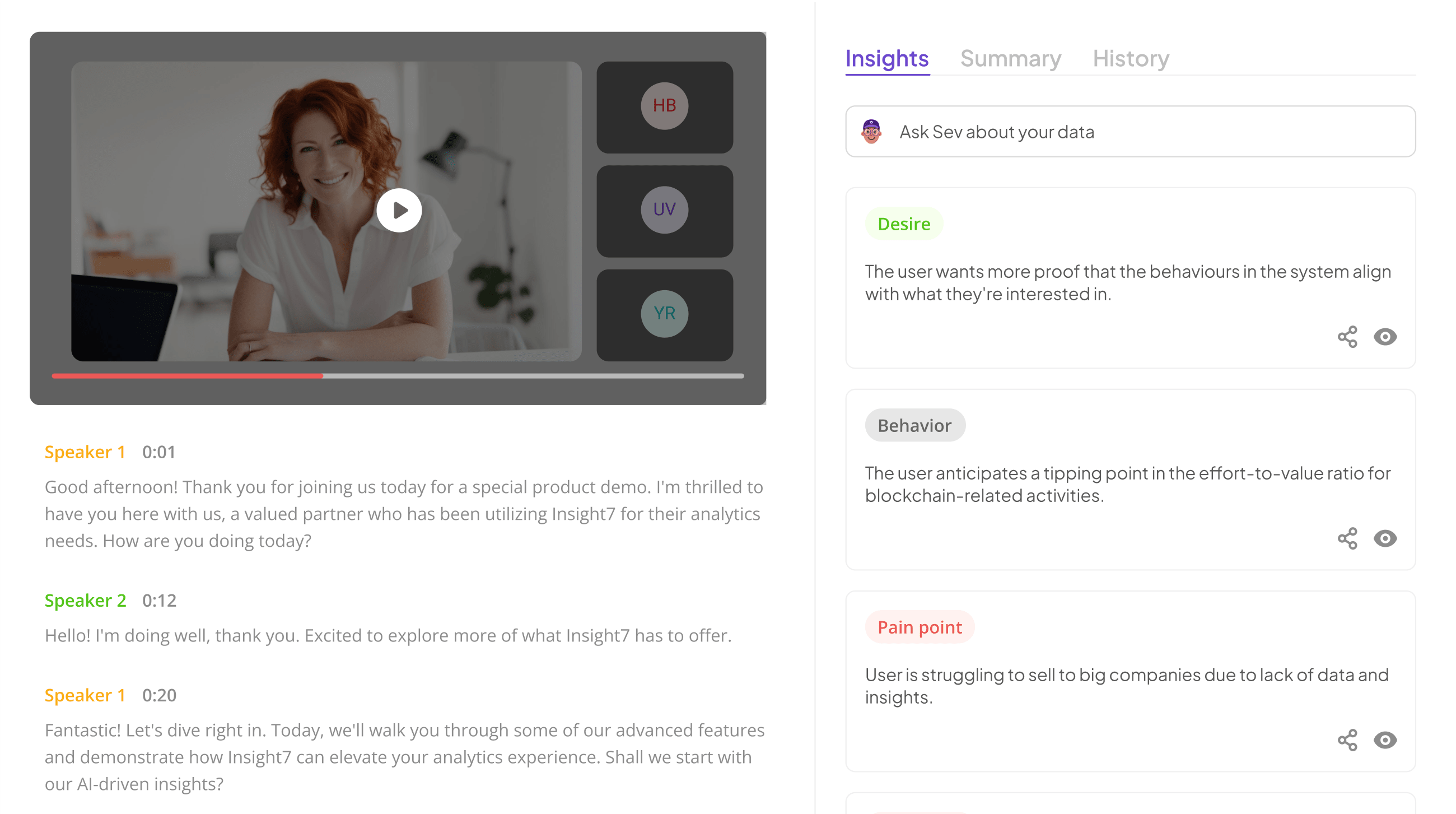
Understanding the Fundamentals of Abandonment Pattern Recognition
Recognizing abandonment patterns requires a foundational understanding of user behavior and motivations. Abandonment Pattern Recognition entails analyzing interview transcripts to uncover reasons why users disengage. This process starts with systematically organizing data, allowing for more efficient exploration of responses. The key is to look for recurring sentiments that signal discomfort or frustration, which frequently lead to abandonment.
Focusing on emotional cues can provide deeper insights into user experiences. Notable patterns can emerge from how users articulate their thoughts about a product or service. By identifying common phrases or sentiments, one can form a clearer picture of friction points. Exploring these aspects will not only enhance your understanding of user behavior but also inform future product improvements. By mastering these fundamentals, you can effectively diagnose why users abandon your service and what can be done to retain them.
Common Triggers of User Abandonment
User abandonment frequently stems from several common triggers that manifest during user interactions. These triggers can include confusing user interfaces, prolonged loading times, and unclear navigation paths. Each of these issues can discourage users, leading them to abandon their journey with your product. Understanding these triggers allows you to better recognize patterns of user abandonment, which is essential for improving user experience.
Additionally, emotional responses can be a significant factor in user abandonment. Frustration from inadequate support or unmet expectations may prompt users to disengage. By analyzing interview transcripts, particularly focusing on user sentiments expressed, you can identify key abandonment pattern recognition elements. Listening closely to users' experiences helps pinpoint these frustrating moments, offering valuable insights to prevent potential drop-offs in the future.
Early Warning Signs in Interview Responses
Recognizing early warning signs in interview responses is crucial for preventing user abandonment. Analysts should look for indicators that suggest users are disengaging or dissatisfied. Phrases expressing confusion, frustration, or a lack of enthusiasm can signal potential abandonment patterns. For example, if users frequently say, โI donโt understand how this works,โ it suggests a deeper issue that may lead to attrition.
Another critical sign is the use of closed-ended questions that don't allow for elaboration. When users provide simple, one-word answers, it often reflects disengagement. Investigating these responses can help pinpoint the reasons behind abandonment behaviors. Patterns like repetitive complaints or hesitation about specific features can also indicate a need for further exploration. By accurately identifying these early warning signs, researchers can take proactive steps to address concerns before they escalate into user abandonment.
Extract insights from interviews, calls, surveys and reviews for insights in minutes
Tools and Techniques for Effective Abandonment Pattern Recognition
Effective Abandonment Pattern Recognition involves employing specific tools and techniques to accurately analyze interview transcripts. The first step is pre-processing and organizing your data. This step ensures that the information is structured and manageable, allowing for easier identification of patterns. By categorizing responses and jotting down notable comments, the groundwork for identifying abandonment indicators becomes clearer.
Next, identifying key themes and trends within the data is critical. This can involve looking for recurring phrases or sentiments that signal dissatisfaction or disengagement. Finally, pinpointing strong indicators of abandonment can reveal underlying issues that need addressing. Combining these structured steps with the right tools, such as Insight7, Rev.com, or Otter.ai, ensures efficient analysis. Together, these methods and technologies provide a robust framework for recognizing user abandonment patterns, ultimately helping improve user experience and retention.
Steps to Analyzing Interview Transcripts
Analyzing interview transcripts is a critical step in recognizing abandonment patterns effectively. Begin by pre-processing and organizing the data. This means cleaning up transcripts, ensuring clarity, and formatting them for easier readability. Carefully sorting responses based on themes can streamline your analysis. Adequate organization helps to connect relevant pieces of feedback and surface important insights regarding user interactions.
Next, identify key themes and trends throughout the transcripts. Look for recurring phrases or sentiments that highlight user experiences and feelings about their interactions. Pay special attention to statements indicating frustration or confusion, as these often signal potential abandonment triggers. Finally, pinpoint specific indicators of abandonment by drawing connections between user feedback and exit points in their journey. These steps lay the groundwork for comprehensive abandonment pattern recognition, enabling you to derive actionable insights that enhance user engagement.
- Step 1: Pre-processing and Organizing Data
Before diving into abandonment pattern recognition, itโs essential to begin with pre-processing and organizing your data. First, gather all relevant interview transcripts in one place, ensuring you have a comprehensive view of the user interactions. This step involves cleaning the data by removing any irrelevant information, such as filler words or repeated phrases, which might create noise in your analysis.
Next, categorize the transcripts based on key themes or use cases. This organization helps unveil potential abandonment indicators buried within the data. Once organized, you can analyze how users expressed their frustrations or challenges during their interactions. By meticulously preparing your data, you set the stage for effective abandonment pattern recognition. This foundational work ultimately leads to insights that can drive design improvements and enhance user retention strategies.
- Step 2: Identifying Key Themes and Trends
Identifying key themes and trends is essential in abandonment pattern recognition. This process allows researchers to sift through interview transcripts and extract meaningful insights. Start by carefully reviewing interview responses to detect common phrases, sentiments, and recurring issues. Every participantโs experience may unveil significant patterns that point to why users abandon a product or service. By categorizing these insights, you will better understand the underlying reasons for user disengagement.
Once themes emerge, analyze how often they occur across transcripts. Look for notable trends, such as specific pain points or user expectations that consistently lead to abandonment. Utilizing tools like Insight7 can help streamline this analysis, providing a robust framework for organizing your findings. Appreciating these themes allows for strategic adjustments in product development, ultimately reducing abandonment and enhancing user experience.
- Step 3: Pinpointing Abandonment Indicators
Identifying abandonment indicators is crucial for insightful analysis of user behavior in interview transcripts. Begin by carefully examining the content of the responses to reveal any recurring sentiments or expressions of dissatisfaction. These emotions often signal that users may have disengaged or are nearing abandonment. Look for specific phrases that express confusion or frustration. Such indicators may serve as vital clues towards understanding the user's journey and motivations.
Next, prioritize the context in which these comments arise. Analyzing follow-up questions or prompts can shed light on the circumstances leading to a user's struggle. Pay attention to non-verbal cues or changes in tone, as they often underscore an experience of frustration or disappointment. Recognizing these patterns is essential for developing strategies that can mitigate user abandonment. By systematically pinpointing these abandonment indicators, you can better understand and enhance the user experience, promoting greater retention and satisfaction overall.
Top Tools for Analyzing Interview Transcripts
To effectively analyze interview transcripts for recognizing abandonment patterns, employing the right tools is essential. Insight7 stands out as a comprehensive option, capable of unveiling intricate user abandonment patterns. This tool streamlines the process of data management, making it easier to identify critical insights from interviews.
Other noteworthy tools also facilitate the analysis of interview transcripts. Rev.com excels in providing transcription services paired with robust analysis features, helping you capture and interpret user feedback. Otter.ai enhances the experience with AI-driven insights, significantly reducing the time needed for analysis. For those seeking varied export options, Sonix.ai offers a versatile solution for transcript analysis, while Temi.com provides fast transcription services alongside user-friendly editing capabilities. By leveraging these tools, you can effectively spot and understand the nuances of user abandonment, aiding in the refinement of your service or product.
- insight7: A comprehensive tool for identifying user abandonment patterns.
Abandonment Pattern Recognition is essential for understanding the moments when users disengage during their interaction with a product. insight7 serves as a comprehensive tool for identifying these user abandonment patterns by analyzing interview transcripts for crucial behavioral signals. By employing advanced analytics, insight7 uncovers specific phrases and sentiments that indicate frustration or confusion, providing valuable context to user experiences.
To effectively utilize insight7 for tracking user abandonment, consider these key steps. First, pre-process your data to ensure clarity and organization; this composition helps in identifying patterns more efficiently. Next, focus on recognizing prominent themes and trends present in user feedback. Finally, pinpoint the specific indicators of abandonment that emerge during the analysis. By systematically applying these techniques, you gain actionable insights that can drive necessary improvements, ultimately enhancing user retention and satisfaction.
- Rev.com: Offers transcription services with analysis features.
Transcription services play a vital role in analyzing user interviews, especially concerning identifying abandonment patterns. By converting spoken language into written text, these tools enable a detailed examination of user sentiments and behaviors. One such service provides not only accurate transcription but also various analytical features, enhancing the ability to derive insights from recorded interviews. With these features, users can search for specific phrases or evaluate content structure, making the identification of abandonment indicators much easier.
In the context of research, recognizing trends in abandoned user experiences is crucial. Users often provide hints within their transcripts, whether they're voicing concern or showing hesitation. These services help dissect those key moments, allowing researchers to spot patterns that indicate potential abandonment. Employing such transcription tools enables businesses to sift through vast amounts of feedback efficiently, transforming raw data into actionable insights that address user challenges and improve overall engagement.
- Otter.ai: Provides transcription and AI-powered insight generation.
Effective transcription and insight generation are crucial when addressing the nuances of user abandonment in interviews. The technology utilized offers automatic transcription services that convert spoken dialogues into text, creating a clear record for analysis. This streamlined process allows researchers to focus on the content, enabling the recognition of critical patterns related to user behavior, particularly concerning abandonment.
Moreover, AI-driven insights can spotlight underlying issues in user responses. For instance, analyzing the transcripts may reveal common phrases or sentiments tied to user drop-off points. By identifying such themes, teams can better understand potential gaps in user experience. Recognizing these abandonment indicators is vital for improving product offerings and retaining users. Ultimately, utilizing advanced transcription tools enhances the ability to spot abandonment pattern recognition effectively, leading to informed strategic decisions for product enhancements.
- Sonix.ai: Allows for transcript analysis with various export options.
Utilizing tools that facilitate transcript analysis is essential in recognizing abandonment patterns in user interviews. The selected platform offers various export options that allow users to process and review the data effectively. By analyzing transcripts with precision, organizations can uncover significant insights about where users tend to disengage. Many interview responses harbor clues indicating potential exit points, making it crucial to leverage robust analysis tools.
When transcripts are analyzed, users can spot recurring themes and specific indicators of abandonment. With features that allow for easy categorization and exportation of insights, the platform makes it straightforward to compile findings. These compiled results can then aid in identifying patterns that may have previously gone unnoticed. Ultimately, the ability to export this data in various formats enhances the workflow, allowing for deeper insights into user behaviors and preferences significantly impacting retention strategies.
- Temi.com: Fast transcription services with easy editing capabilities.
Fast transcription services dramatically streamline the process of analyzing interview transcripts, especially when you're focused on identifying user behavior patterns. With quick turnaround times and a straightforward editing interface, these services make it easy to refine transcripts for nuanced analysis. This is crucial when examining areas of user abandonment, as even small details in conversations can reveal significant insights about user engagement.
In the quest for effective abandonment pattern recognition, leveraging speedy transcription services can reduce the time spent on preparation. The ability to edit transcripts easily allows researchers to highlight critical moments and phrases that may signal user hesitance or disengagement. Identifying these indicators through well-processed transcripts can lead to actionable insights, enabling you to enhance user experiences and minimize abandonment rates. Ultimately, the combination of fast transcription and thoughtful analysis paves the way for improved understanding of user behavior, guiding future decisions and strategies.
Conclusion on Abandonment Pattern Recognition
Recognizing patterns of user abandonment is crucial for enhancing user experience and improving retention strategies. By thoroughly analyzing interview transcripts, one can unveil common themes and indicators that suggest when users disengage or lose interest. Such insights serve as valuable feedback for refining products or services based on real user input.
The process of abandonment pattern recognition is not merely a technical task but a proactive approach to understanding user behavior. By identifying specific triggers and warning signs within the data, organizations can take informed action to address concerns before they escalate. This analytical journey ultimately leads to a more user-centered design and stronger customer relationships.