Thematic Response Segmentation serves as a crucial method for enhancing the analysis of survey data. By grouping responses into distinct themes, analysts can uncover deeper insights vital for decision-making. This process not only identifies key trends but also highlights underlying patterns within diverse data sets, transforming raw information into actionable intelligence.
As we explore Thematic Response Segmentation, it's essential to understand how leverage AI can streamline the process. With advanced techniques, AI can efficiently categorize responses, enabling quicker and more accurate analysis. This ultimately empowers organizations to respond more effectively to customer feedback and evolving market demands.
Analyze & Evaluate Calls. At Scale.
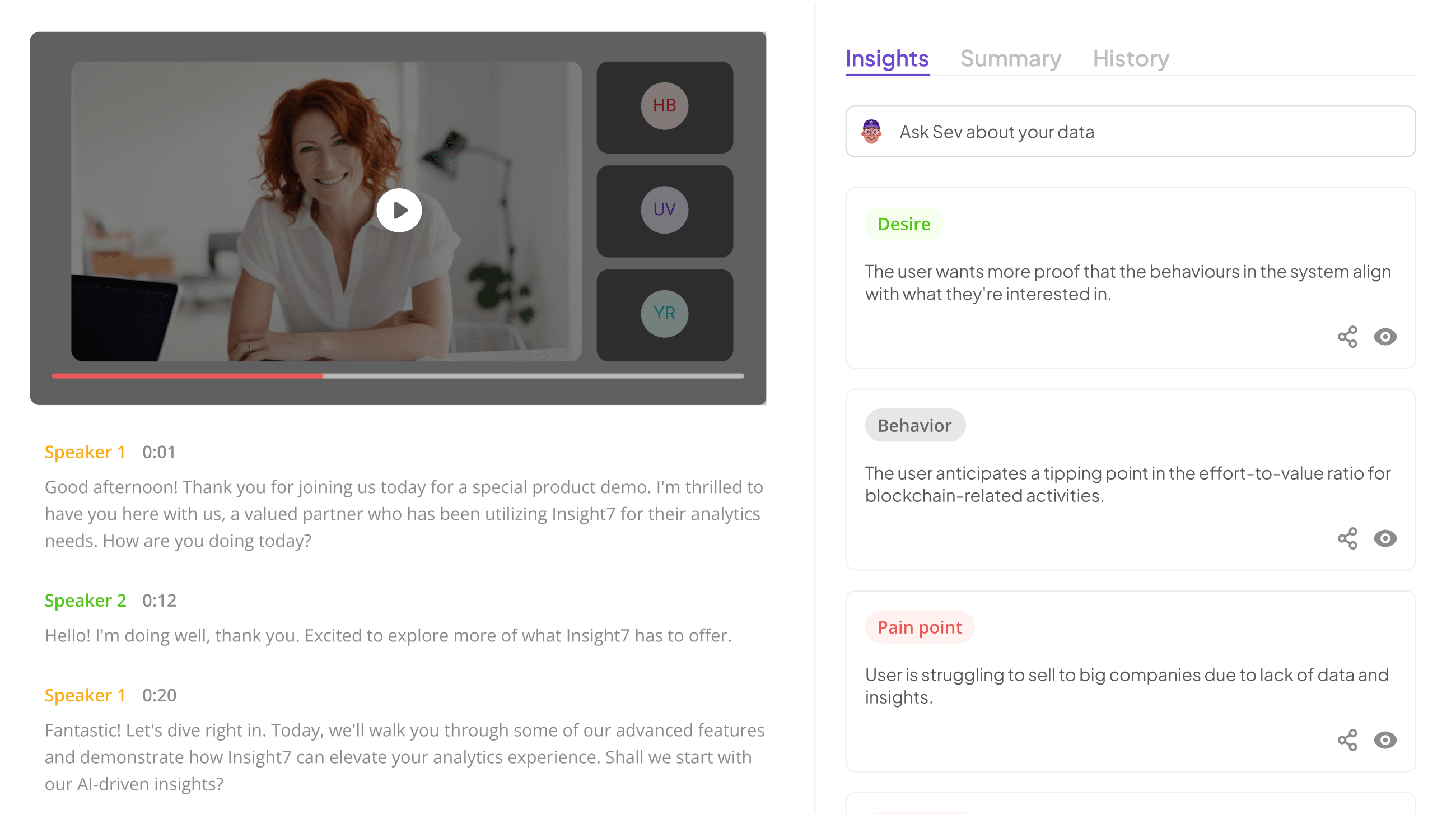
Understanding Thematic Response Segmentation with AI
Thematic Response Segmentation involves organizing survey responses based on shared themes and insights. Using AI for this purpose enhances efficiency and accuracy, allowing businesses to extract meaningful information from large volumes of data. By automating the segmentation process, organizations can gain deeper insights into consumer sentiment and preferences, which are crucial for informed decision-making.
With AI, the thematic analysis becomes more streamlined and insightful. AI algorithms can quickly identify patterns, helping to categorize responses into clear themes, making it easier to focus on specific areas of interest. For example, a survey on customer satisfaction might reveal common sentiments regarding product quality or service speed, allowing companies to address concerns effectively. Understanding these themes can guide marketing strategies and improve overall customer experiences. Using AI in thematic response segmentation not only saves time but also provides a comprehensive understanding of survey data, empowering organizations to respond to their audience's needs effectively.
The Importance of Thematic Segmentation
Thematic response segmentation plays a critical role in comprehending survey results. By segmenting responses according to themes, organizations can gain deeper insights into customer sentiments and preferences. This process allows researchers to identify recurring topics, which can lead to more targeted strategies for engagement and service improvement. When themes are accurately recognized, responses can be analyzed more meaningfully, driving actionable insights that align with specific business objectives.
Utilizing AI for thematic response segmentation further enhances this process. AI tools can quickly analyze vast amounts of data, pinpointing trends and patterns that manual analysis might overlook. This results in a more efficient analysis, enabling businesses to respond swiftly to customer needs. By focusing on thematic segmentation, organizations can not only improve their understanding of feedback but also enhance decision-making and strategic planning. Ultimately, thematic segmentation stands as a cornerstone of effective survey analysis, bridging the gap between data and informed business actions.
- Why segmenting survey responses by theme matters.
Segmenting survey responses by theme is an essential process that directly impacts data analysis and decision-making. By organizing responses into thematic categories, organizations can uncover insights that may often be hidden among raw data. This approach allows for a clearer understanding of participant sentiments, concerns, and preferences. When you employ Thematic Response Segmentation, it's possible to identify trends that can inform strategic initiatives and enhance user experiences.
Furthermore, segmenting responses by theme enables a focused analysis, making it easier to draw actionable conclusions. This targeted approach eliminates noise from unrelated data and highlights common threads that resonate across participant feedback. By leveraging AI tools in this process, you increase the precision of your analysis and save significant time, correlating insights with defined business goals. In essence, effective thematic segmentation leads to a deeper, more nuanced understanding of survey responses, paving the way for informed decisions and improved service outcomes.
- Benefits of using AI for thematic segmentation.
Using AI for thematic segmentation offers a range of significant benefits that can enhance the analysis of survey responses. One major advantage is efficiency. Traditional analysis can be time-consuming and labor-intensive, but AI algorithms can process large volumes of data quickly. This allows researchers to identify key themes and insights in a fraction of the time, enabling faster decision-making.
Another key benefit is the improved accuracy of thematic response segmentation. AI can recognize patterns and nuances in textual data that human analysts might overlook, leading to more reliable insights. Furthermore, AI tools can be customized to track specific goals, such as customer satisfaction or product feedback, ensuring that the analysis is aligned with the organization's objectives. By incorporating AI into thematic segmentation, users gain a powerful tool that not only saves time but also enhances the overall quality of insights derived from survey responses. Thus, utilizing AI transforms the process of analysis into a more insightful and streamlined experience.
Steps to Implement AI-Powered Thematic Response Segmentation
To implement AI-powered thematic response segmentation, begin by collecting and preprocessing your survey data. It is crucial to gather comprehensive responses while ensuring that the data is clean and organized for seamless analysis. This initial step lays the groundwork for effective thematic analysis and allows AI models to function optimally.
Next, select suitable AI tools to facilitate thematic response segmentation. Tools such as insight7, MonkeyLearn, and Thematic specialize in different aspects of data analysis, offering various features that can enhance your segmentation efforts. Then, implement AI models that are capable of recognizing patterns and themes within your data. Regularly evaluate the performance of these models to ensure that the segmentation is accurate and reliable. By following these steps, you can effectively harness the power of AI to derive valuable insights from survey responses.
Extract insights from interviews, calls, surveys and reviews for insights in minutes
Step 1: Collect and Preprocess Survey Data
To effectively begin the process of thematic response segmentation, it is crucial first to collect and preprocess your survey data. This initial step sets the stage for analyzing and extracting meaningful insights from the responses. Begin by gathering comprehensive survey data, ensuring it encompasses a wide range of participant inputs to capture diverse perspectives. This breadth of information is vital, as it will help identify recurring themes and nuanced opinions.
Once the data is collected, focus on cleaning and organizing it. Preprocessing involves removing extraneous information, correcting errors, and standardizing responses to maintain consistency. This step is necessary to ensure the data is as accurate as possible, which enhances the effectiveness of AI analysis later on. Only through meticulous collection and preprocessing can you unlock the full potential of AI in thematic response segmentation, ultimately transforming raw data into actionable insights.
- Gathering comprehensive survey responses.
Gathering comprehensive survey responses is crucial for effective thematic segmentation analysis. Quality responses ensure that the data reflects the diverse views and experiences of participants. To enhance the richness of your data, it's vital to design surveys that encourage detailed feedback. Open-ended questions can facilitate deeper insights, allowing respondents to share their thoughts freely, rather than simply selecting predefined options.
Once you collect these responses, the next step involves cleaning and organizing the data. This process ensures all information is coherent and ready for analysis. Pay attention to inconsistencies or omissions that may lead to skewed results. An organized dataset can improve the performance of AI tools designed for thematic response segmentation, producing more accurate and actionable insights that lead to informed decisions. Effective gathering and processing of survey responses set the foundation for successful analysis and understanding of underlying themes.
- Cleaning and organizing the data for analysis.
Cleaning and organizing the data for analysis is a crucial first step in the process of thematic response segmentation. It begins with collecting comprehensive survey responses and ensuring that they are devoid of errors or inconsistencies. This preliminary work helps to establish a clean dataset, making it easier to identify key themes later during analysis. Utilizing tools for data cleaning can streamline this process, automatically detecting duplicates and filling gaps that may distort results.
Once the data is clean, organizing it into relevant categories is essential. This can involve tagging responses based on predefined themes or allowing for an open-coding approach, where new themes emerge during the analysis. Clear organization aids in the subsequent stages, providing a solid foundation for employing AI tools that will highlight and extract critical insights effectively. Following these steps ensures that analysis yields relevant results, ultimately leading to actionable conclusions.
Step 2: Selecting the Right AI Tools for Thematic Segmentation
Selecting the right AI tools for thematic response segmentation is crucial to harnessing the insights from your survey data effectively. Begin by assessing the specific needs of your analysis and identifying the tools that align with your objectives. Some tools specialize in text analysis, while others excel in sentiment analysis or qualitative data categorization. Consider factors such as user-friendliness, scalability, and integration capabilities with your existing systems.
Furthermore, prioritize AI tools that offer advanced functionalities like customizable themes and automated coding to streamline the thematic segmentation process. For example, tools that cluster insights into meaningful categories will not only save time but also enhance the analysis quality. Remember, the ultimate goal is to extract actionable insights from your survey responses, enabling better decision-making and improved user experience. By thoughtfully selecting the appropriate AI tools, you can simplify the complexity of thematic segmentation and achieve deeper analytical insights.
Top AI Tools for Thematic Response Segmentation
Identifying the right AI tools is essential for efficient thematic response segmentation in survey analysis. Various platforms have emerged, each offering unique capabilities that streamline the process of categorizing responses by themes. Understanding these tools can significantly enhance your ability to extract meaningful insights from qualitative data, transforming raw responses into structured themes.
Some top AI tools to consider include comprehensive platforms like insight7, which specialize in thematic segmentation utilizing advanced AI features. User-friendly options such as MonkeyLearn assist in text analysis and segmentation tasks, making them accessible for teams with varying levels of expertise. Additionally, Thematic focuses on survey-specific analysis, while NVivo excels in handling qualitative data for thorough thematic categorization. Lastly, Clarabridge stands out for its detailed sentiment analysis and theme extraction capabilities. By integrating these tools, researchers can eliminate the challenges of manual categorization, ensuring timely and accurate results in thematic response segmentation.
- insight7: Comprehensive tool for thematic segmentation with advanced AI capabilities.
Thematic Response Segmentation empowers users to efficiently categorize survey responses, allowing for deeper insights into patterns and sentiments. One notable tool, insight7, stands out due to its advanced AI capabilities aimed at thematic segmentation. This powerful tool streamlines the analysis process by automatically identifying relevant themes within your survey data, enhancing both speed and accuracy.
Using insight7, users can define specific goals, such as improving customer experience or identifying challenges. The tool helps cluster insights into meaningful themes, providing sentiment analysis alongside each categorized response. Additionally, it features default tags while allowing for customization, ensuring a tailored experience that meets individual research needs. This comprehensive approach transforms raw data into actionable insights, ultimately saving time and improving outcomes in survey analysis.
- MonkeyLearn: User-friendly platform for text analysis and segmentation.
When considering thematic response segmentation, a user-friendly platform streamlines the process of text analysis and effectively categorizes responses. The intuitive interface simplifies data ingestion, allowing users to easily upload transcripts or survey responses. The platformโs capabilities enable users to extract meaningful themes and insights quickly without needing extensive technical knowledge.
The thematic response segmentation feature can enhance user experience significantly. Users benefit from straightforward data organization and analysis, which allows for deeper insights into survey results and customer feedback. By providing tools that can generate reports and highlight key themes, the platform supports effective decision-making processes. This ease of use ensures that even those without an extensive background in data analysis can harness the power of AI to derive actionable insights from their survey responses.
- Thematic: Specializes in survey analysis with AI-powered insights.
Thematic Response Segmentation is an essential process in understanding survey insights. By employing AI-powered analysis, you can efficiently categorize responses into distinct themes, allowing for a deeper understanding of your data. This method enhances your ability to extract valuable insights, ultimately guiding strategic decisions. Each theme clusters related responses together, helping to illustrate major trends, sentiments, and preferences from your respondents.
Moreover, using AI tools provides a significant advantage in this endeavor. With automated data processing, you can quickly and accurately identify themes without manual effort. Additionally, categorizing responses not only saves time but also reduces subjectivity in analysis. Thematic Response Segmentation enables you to uncover hidden patterns of customer behavior, making your findings actionable and relevant to your goals for improvement and innovation. Overall, this approach transforms raw survey data into meaningful insights that can influence decision-making and strategy development.
- NVivo: Offers robust qualitative data analysis and thematic categorization.
When exploring thematic response segmentation, NVivo stands out as a powerful tool for qualitative data analysis. It enables researchers to categorize complex survey responses into meaningful themes, enhancing the interpretation of qualitative data. By effectively organizing responses into thematic clusters, analysts can uncover insights that may not be immediately obvious during initial data reviews.
The software allows users to visually map out themes and sub-themes, facilitating a deeper understanding of the relationships among various responses. This capability is crucial as it streamlines the coding process, transforming vast amounts of data into organized, accessible insights. NVivoโs flexibility in handling diverse data types and integrating advanced analytical features makes it an invaluable asset. By utilizing NVivo for thematic response segmentation, researchers can significantly improve their analytical outcomes, ensuring that key themes are identifiable and actionable.
- Clarabridge: Provides detailed sentiment and theme analysis.
Thematic response segmentation enhances the analysis of survey data through detailed insights. AI tools are designed to extract sentiment and categorize responses into meaningful themes. This process allows organizations to identify specific patterns within feedback, making it easier to pinpoint areas for improvement. By employing advanced algorithms, thematic segmentation provides a nuanced understanding of user sentiments, which is crucial for informed decision-making.
AI facilitates the grouping of insights by themes, allowing teams to focus on what truly matters. The clustering of data not only streamlines analysis but also connects responses to specific business goals. For instance, responses might reveal customer concerns about service quality, enabling targeted interventions. Utilizing AI for thematic response segmentation will empower organizations to respond more effectively to their audience's needs and improve overall experiences. By prioritizing thorough sentiment analysis and theme identification, businesses can create strategies that are insightful, relevant, and actionable.
Step 3: Implementing AI Models for Theme Identification
To implement AI models for theme identification, you must first understand the core functionality of these systems. AI models utilize natural language processing to analyze survey responses and extract prevalent themes. By training these models on historical data, they can learn to discern patterns indicative of specific themes. Thematic Response Segmentation enables efficient categorization of insights, allowing deeper understanding of participant sentiments.
Once the model is trained, it's crucial to evaluate its performance. Regular testing against known outcomes helps ensure its accuracy in theme identification. You might consider incorporating feedback loops where user insights refine the model further. Use detailed analytics to assess which themes are consistently recognized and how they align with your survey goals. This process facilitates a continuous improvement cycle, enhancing the modelโs reliability and the overall efficacy of your thematic segmentation strategy.
- Training AI models to recognize patterns and themes in the data.
Training AI models to recognize patterns and themes in the data is crucial for effective thematic response segmentation. This stage involves utilizing machine learning techniques to analyze and classify the rich textual information gathered from survey responses. Well-trained models can identify recurring themes and sentiments, which can inform decision-making.
First, it's essential to preprocess the survey data adequately. This process typically includes cleaning the data and converting it into a structure suitable for AI algorithms. Tokenization and removing stopwords are common practices. Next, selecting an appropriate AI tool can significantly impact the model's ability to recognize relevant themes effectively. Tools like NVivo or Thematic can streamline this process, enabling models to learn from a diverse range of responses.
Once a model is in place, ongoing evaluation is necessary. Continuous refinement ensures stability and accuracy in recognizing themes as new data comes in. This iterative approach ultimately strengthens thematic response segmentation, allowing organizations to derive deeper insights from their survey data.
- Evaluating AI performance to ensure accuracy in segmentation.
Accurate evaluation of AI performance is crucial to ensure reliable thematic response segmentation. By monitoring the capabilities of your AI tools, you can identify any weaknesses in their ability to categorize and analyze qualitative survey data. Regularly assessing these systems allows you to adjust parameters and enhance their accuracy, which is vital for meaningful insights. Ensuring that your AI effectively discerns patterns and themes leads to richer, more actionable data.
Key elements to consider in evaluating AI performance include the consistency of theme identification, precision in categorizing responses, and the ability to adapt to different types of data. First, conduct periodic performance tests to gauge whether the AI consistently identifies the same themes across similar datasets. Second, analyze how accurately the AI's categorizations align with human evaluations to ensure validity. Finally, gather user feedback on the AIโs performance to identify areas of improvement. Implementing a robust evaluation framework not only improves accuracy but also builds trust in the AI-driven segmentation outcomes.
Conclusion of Thematic Response Segmentation using AI
Thematic Response Segmentation using AI revolutionizes the way we analyze survey data, enabling more accurate insights and quicker decision-making. By embracing AI, organizations can efficiently categorize responses into recognizable themes, allowing for a clearer understanding of participant sentiments and motivations. This process not only speeds up data analysis but also uncovers hidden patterns that manual methods might miss.
Ultimately, effective thematic segmentation enhances both strategic planning and operational efficiency. As organizations increasingly demand timely insights, embracing AI-driven methodologies becomes crucial for remaining competitive in today's fast-paced environment. Leveraging these advanced techniques aids businesses in transforming raw survey data into valuable knowledge, making informed decisions that resonate with their target audience.