AI-driven Qualitative Synthesis represents a groundbreaking approach to analyzing qualitative data. Traditional methods often involve tedious manual reviews of transcripts, interviews, and survey responses, leading to inconsistencies and biases. The emergence of AI tools offers researchers the ability to streamline this process, providing faster insights without sacrificing quality.
With the implementation of AI-driven Qualitative Synthesis, organizations can automate data reduction and analysis. This innovation not only saves time but also facilitates a more objective examination of perspectives, enabling teams to derive meaningful themes and recommendations swiftly. Embracing this technology presents an opportunity for greater efficiency in qualitative research.
Analyze qualitative data. At Scale.
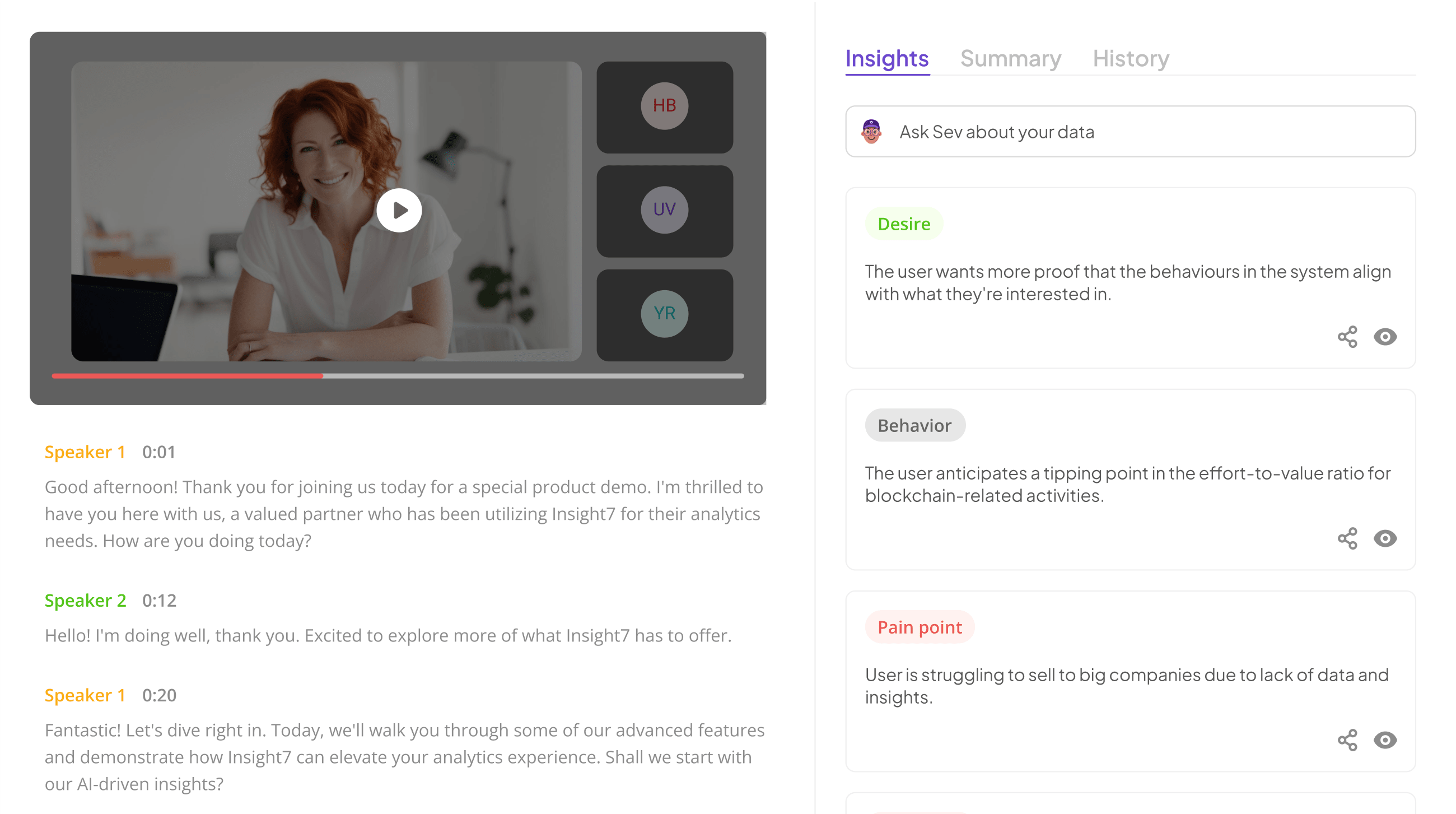
Understanding AI-driven Qualitative Synthesis in Data Reduction
AI-driven qualitative synthesis is revolutionizing how researchers manage vast amounts of qualitative data. It enables the transformation of extensive interviews and open-ended survey responses into organized, actionable insights. By automating the synthesis process, AI significantly reduces the time and effort required for data analysis, which is often bogged down by manual methods. The results can be achieved more consistently, addressing biases inherent in human interpretations.
To understand AI-driven qualitative synthesis better, consider the following key aspects:
Data Processing Efficiency: AI efficiently processes large datasets, enabling quicker identification of themes and patterns.
Consistency and Accuracy: Machine learning algorithms can deliver uniform insights, mitigating discrepancies that can arise from manual analysis.
Scalability: AI solutions can easily scale to accommodate growing datasets, making them ideal for organizations facing increasing data volumes.
By embracing these advantages, researchers can enhance their qualitative data reduction efforts, leading to more timely and reliable insights.
What is AI-driven Qualitative Synthesis?
AI-driven Qualitative Synthesis refers to the application of artificial intelligence techniques to analyze qualitative data effectively. This process transforms raw data, such as interview transcripts and open-ended survey responses, into actionable insights. By employing AI, researchers can automate the coding, categorization, and synthesis of qualitative information, significantly accelerating the analytic process. This results in quicker turnaround times for reports, allowing teams to respond to client needs rapidly.
The benefits of AI-driven qualitative synthesis extend beyond speed. It also enhances accuracy by reducing human bias, which often affects traditional qualitative analysis. By using AI algorithms, patterns and themes emerge more consistently, leading to more reliable findings. Thus, AI-driven qualitative synthesis is not just about efficiency; itโs about improving the quality and credibility of insights derived from qualitative research. Embracing this approach can revolutionize how organizations process and utilize qualitative data, empowering them to make informed decisions.
Understanding AI-driven Qualitative Synthesis in Data Reduction involves recognizing its transformative potential. This approach streamlines the qualitative data analysis process by utilizing advanced algorithms to uncover patterns and insights from vast datasets. Researchers can efficiently condense large volumes of information, making it easier to interpret and apply findings to relevant contexts.
The process begins with data collection and preparation, where raw data is organized for analysis. Following this, AI algorithms are applied to identify themes and significant trends, aiding in deeper understanding. Finally, interpreting these AI-driven results allows researchers to draw actionable conclusions, ultimately enhancing decision-making processes. By embracing AI-driven Qualitative Synthesis, organizations can harness data to reveal valuable insights that inform strategic initiatives and improve outcomes.
Benefits of Using AI for Qualitative Data Reduction
AI-driven Qualitative Synthesis provides significant advantages for managing qualitative data. One key benefit is efficiency; AI can quickly process vast amounts of data, making it easier to extract insights without the lengthy manual efforts traditionally required. By automating tedious tasks such as coding and theming, researchers can focus on deeper analysis and interpretation, ultimately enhancing productivity.
Another advantage is consistency. Human analysis often yields varying results due to personal biases or interpretation differences. AI algorithms offer standardized methodologies, minimizing inconsistencies and ensuring reliability in the insights produced. This is crucial in environments where teams collaborate and share research findings.
Furthermore, the scalability of AI-driven qualitative synthesis allows for handling larger datasets, enabling researchers to analyze complex data sources with relative ease. Ultimately, the integration of AI not only streamlines qualitative data reduction but also enriches the overall research process. With these benefits, AI promises a transformative impact on qualitative research methodologies.
Steps for Implementing AI-driven Qualitative Synthesis
Implementing AI-driven Qualitative Synthesis involves several key steps to enhance efficiency and reduce bias. The first step is data collection and preparation. Gather qualitative data, such as interview transcripts or open-ended survey responses, ensuring its quality and relevance. Organize the data systematically to facilitate analysis. Clear preparation sets the foundation for effective use of AI tools.
Next, apply AI algorithms tailored for qualitative data analysis. Utilize AI tools designed to identify themes, sentiments, and patterns within the data. This automated approach significantly speeds up the analysis process compared to traditional methods. Following the analysis, it's crucial to interpret the results accurately. Review the AI-generated insights, cross-referencing them with your understanding of the context to ensure validity. This interpretation stage is essential to transform raw AI outputs into actionable findings. Each of these steps contributes to a more streamlined and effective qualitative synthesis process.
Extract insights from interviews, calls, surveys and reviews for insights in minutes
Step 1: Data Collection and Preparation
The initial phase of implementing AI-driven qualitative synthesis focuses on efficient data collection and preparation. This step is crucial, as the quality and structure of your data significantly influence the subsequent analysis. Begin by collecting qualitative data from diverse sources, such as interviews, surveys, or focus groups. Data must be carefully organized and formatted to ensure compatibility with AI tools.
Next, undertake thorough preparation. This includes transcribing audio or video recordings accurately, ensuring that the text reflects the original dialogue's nuances. In addition, consider the importance of data anonymization to protect sensitive information. Once your dataset is organized and prepped, you're ready for AI algorithms to analyze and synthesize insights effectively. By focusing on these preliminary steps, you lay a strong foundation for meaningful qualitative analysis.
Step 2: Applying AI Algorithms
Applying AI algorithms involves using advanced computational methods to analyze qualitative data efficiently. This stage is crucial in the process of AI-driven qualitative synthesis, transforming raw data into meaningful insights. First, algorithms such as natural language processing (NLP) can identify patterns and themes within large datasets. These techniques allow for the automatic classification of responses and the extraction of significant information that humans might overlook.
Next, machine learning algorithms can enhance accuracy by learning from previous analyses and adapting to new data inputs. They improve the consistency and reliability of insights generated from qualitative data. By employing these AI-driven tools, researchers can streamline their analysis, ensuring that critical themes and nuances are captured swiftly. This ultimately leads to more effective decision-making and a deeper understanding of the qualitative landscape.
Step 3: Interpreting AI-driven Results
Interpreting AI-driven results marks a pivotal stage in the qualitative data reduction process. It transforms vast amounts of data into actionable insights, allowing researchers to extract meaningful themes and trends. By leveraging AI-driven qualitative synthesis, you can quickly identify critical findings that inform decision-making, enhancing the overall value of your research. During this step, clarity is paramount; ensure that insights are presented transparently and comprehensively for optimal understanding.
To effectively interpret AI-driven results, consider the following key pointers:
- Data Contextualization: Understand the origin and background of the data to build trust in the insights derived.
- Theme Selection: Identify relevant themes that resonate with your research focus, as these will guide your narrative.
- Insight Validation: Validate insights with supporting quotes and contextual evidence to enhance credibility.
- Report Structuring: Organize findings in a cohesive manner, presenting insights alongside recommendations for practical application.
By focusing on these elements, you can ensure that the interpretation of AI-driven results leads to thorough and thoughtful insights.
Essential Tools for AI-driven Qualitative Synthesis
AI-driven Qualitative Synthesis relies on various essential tools that augment human analysis and streamline research processes. Popular software options include MAXQDA, NVivo, and Atlas.ti, each offering unique functionalities that cater to different aspects of qualitative research. For example, MAXQDA excels in document management, allowing users to organize, code, and analyze data effectively. NVivo provides advanced text analytics features, making it suitable for examining large datasets and extracting valuable insights.
In addition, Dedoose is known for its collaborative capabilities, enabling teams to work together seamlessly on qualitative projects. Each of these tools brings specific strengths to the table, enhancing the ability to derive themes and patterns from qualitative data. By adopting these solutions, researchers can improve consistency, reduce time spent on manual analysis, and ultimately produce richer insights. Embracing AI-driven qualitative synthesis through these tools ensures that teams are prepared to meet the increasing demand for timely and reliable research outputs.
Insight7
In the rapidly evolving landscape of qualitative research, Insight7 offers a potent framework for AI-driven qualitative synthesis. This approach effectively reduces vast amounts of qualitative data, transforming it into actionable insights. By utilizing artificial intelligence, researchers can expedite the synthesis process, thus revealing patterns and themes that might otherwise go unnoticed.
Implementing AI-driven qualitative synthesis begins with comprehensive data collection, followed by the application of advanced algorithms. These algorithms assist in interpreting the results, ensuring that critical insights are highlighted. This systematic process not only enhances the accuracy and reliability of findings but also allows researchers to focus on strategic decision-making. As organizations seek innovative solutions to analyze customer feedback, the integration of AI tools emerges as a strategic advantage in understanding user sentiments and market dynamics. Ultimately, embracing AI-driven techniques empowers researchers to glean deeper insights from their qualitative data.
MAXQDA
When it comes to MAXQDA, users encounter a powerful tool designed for qualitative data analysis. This software facilitates efficient organization and interpretation of vast amounts of qualitative data. With features that support text analysis, visualization, and coding, it can be particularly advantageous for researchers seeking AI-driven qualitative synthesis.
The integration of AI capabilities enhances MAXQDA's functionality, allowing for automated data reduction. Users can swiftly identify emerging themes and patterns in qualitative data, which significantly streamlines the research process. Moreover, by automating the initial stages of analysis, researchers can devote more time to nuanced interpretations and insights. In this ever-evolving digital age, leveraging such tools can dramatically enhance the quality and efficiency of qualitative research efforts, ultimately leading to more informed decisions.
NVivo
NVivo is a powerful tool designed to facilitate AI-driven qualitative synthesis, making the process of data reduction more efficient. By allowing researchers to organize, analyze, and visualize qualitative data effectively, NVivo streamlines the traditionally cumbersome tasks associated with qualitative research. The software employs advanced algorithms to identify themes and patterns, thus transforming raw data into insightful information.
Using NVivo, researchers can minimize manual tasks like tagging data or creating visual maps, as the tool automates many of these processes. This not only saves time but also increases the accuracy of the findings. By embracing NVivo in your qualitative research, you can merge human insight with AI capabilities, enabling a richer understanding of complex data. Consequently, the collaboration between AI and human intelligence becomes essential in enhancing qualitative synthesis and ensuring that research outcomes are both comprehensive and relevant.
Atlas.ti
When discussing tools for qualitative data analysis, one robust option stands out as an essential resource. This platform facilitates AI-driven qualitative synthesis by streamlining the coding process and enhancing analytical capabilities. It enables researchers to manage and explore their data efficiently, making it especially useful for reducing complex qualitative information into understandable insights.
Furthermore, the platform allows users to apply various analytical methods, from simple thematic analysis to more advanced AI algorithms. This adaptability can significantly enhance data interpretation, enabling researchers to derive actionable insights quickly. By utilizing such a powerful tool, practitioners can significantly improve the quality of their findings and the overall efficiency of their qualitative data reduction processes. Embracing AI-driven qualitative synthesis through this platform maximizes the potential for impactful insights and informed decision-making.
Dedoose
When exploring methods for qualitative data reduction, the role of specialized tools becomes essential. One robust option is geared towards fostering AI-driven qualitative synthesis to streamline the analysis processes. Users can efficiently upload various data types, allowing for a comprehensive examination of insights extracted from interviews, focus groups, or surveys. The integrated features help transform raw data into actionable insights, effectively supporting users in making informed decisions.
Another notable aspect of this tool is its ability to generate themes, quotes, and visual summaries from the analyzed data. This capability proves invaluable for users needing quick iterations on their findings. Additionally, the platform ensures compliance with data privacy regulations, safeguarding sensitive information throughout the analysis. By harnessing this software, researchers can enhance their qualitative synthesis efforts, ultimately making qualitative data reduction not only more manageable but also more impactful.
Conclusion: The Future of AI-driven Qualitative Synthesis
The future of AI-driven qualitative synthesis promises to revolutionize how researchers analyze and interpret qualitative data. As organizations increasingly demand rapid insights, AI-driven approaches will enable quicker decision-making and more consistent results. By automating data analysis, teams can eliminate bias and inefficiencies, ensuring that insights derived from interviews and surveys are both reliable and actionable.
Looking ahead, the integration of sophisticated AI tools will empower researchers to delve deeper into qualitative data, uncovering nuanced themes with greater precision. This technological evolution will not only enhance the quality of qualitative synthesis but also empower firms to adapt to the fast-paced demands of their industries, making informed decisions more accessible than ever before.