Introduction to Predictive QA Optimization begins with recognizing the transformative potential of data in enhancing quality assurance programs. Predictive QA Optimization utilizes advanced analytics to forecast potential quality issues before they arise, thereby improving efficiency and reducing costs. This proactive approach enables organizations to address problems at their source rather than in post-evaluation phases.
Creating a culture centered around predictive strategies shifts the focus from reactive to proactive quality management. Incorporating predictive analytics can lead to substantial improvements in QA processes, engagement with customers, and overall satisfaction. Understanding this concept is essential for organizations looking to stay ahead in an increasingly competitive landscape.
Analyze qualitative data. At Scale.
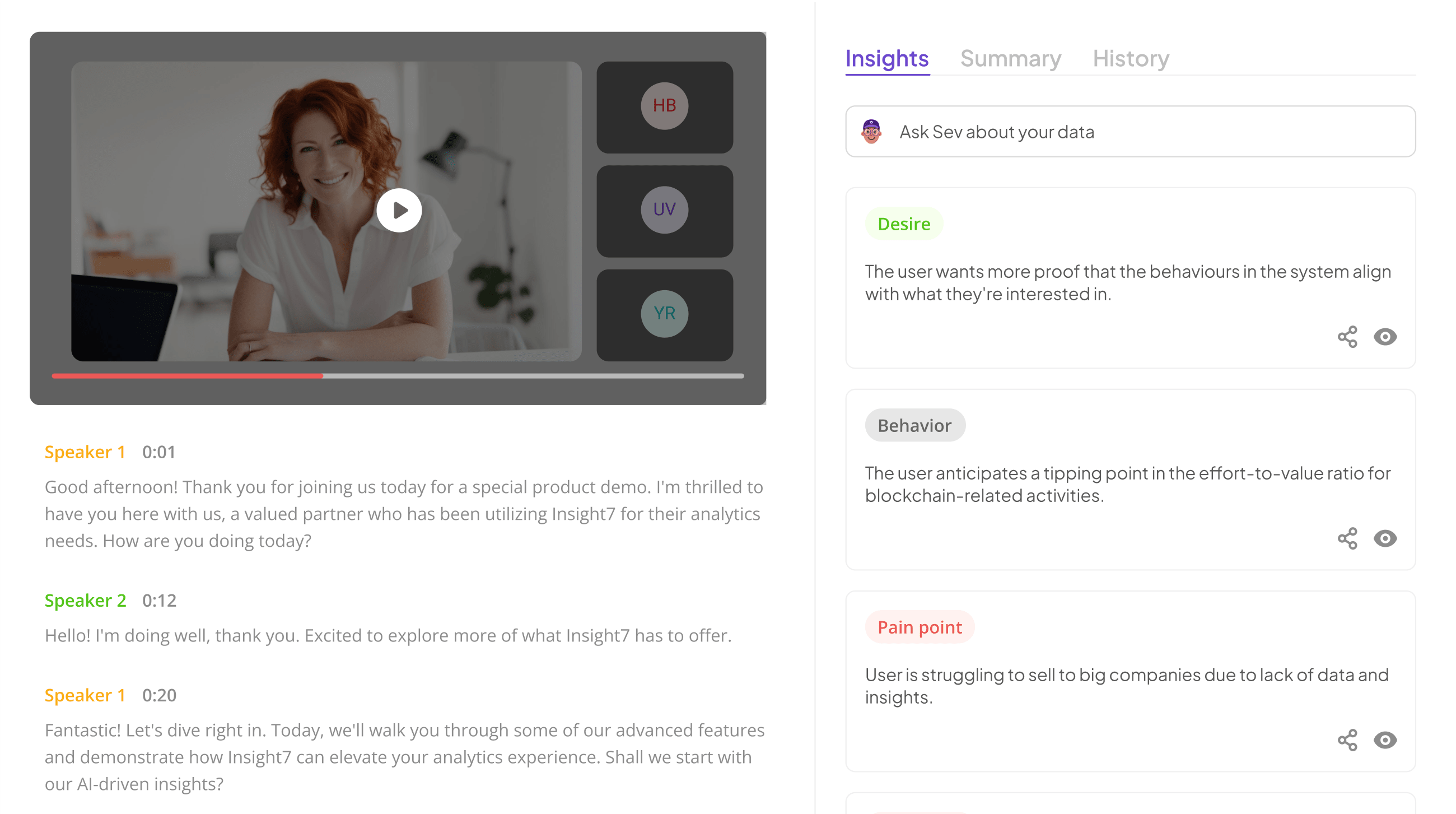
Understanding Predictive QA Optimization in Quality Assurance
Incorporating Predictive QA Optimization into quality assurance processes marks a significant shift in how organizations can improve product quality. It involves utilizing predictive analytics to identify potential quality issues before they arise. By analyzing patterns from historical data, teams can foresee areas of concern, thus sparing time and resources that would otherwise be spent on quality failures.
Organizations benefit immensely by integrating this approach. Predictive QA Optimization enables proactive problem-solving, enhancing compliance and efficiency. By effectively assessing trends and anomalies, companies can ensure their quality assurance efforts are not only reactive but also strategic. This shift not only builds product reliability but also fosters customer trust and satisfaction, pivotal in maintaining a competitive edge in todayโs market environment. Embracing this optimization approach leads to considerably improved quality assurance programs and overall business performance.
The Role of Data in Predictive QA Optimization
Data serves as the backbone for predictive QA optimization, enabling organizations to refine their quality assurance programs. By analyzing historical data trends, teams can uncover patterns that may indicate underlying quality issues. This analytical approach helps identify critical areas for improvement by pinpointing weaknesses early in the development process.
Moreover, effective data collection and analysis allow teams to anticipate potential failures before they occur. For instance, by using customer feedback and testing results, organizations can implement proactive measures rather than reactive fixes. This predictive capability not only enhances product quality but also fosters a culture of continuous improvement within QA teams. Consequently, leveraging data effectively transforms quality assurance from a reactive task into a strategic advantage, positioning companies for sustained success in a competitive landscape.
Benefits of Implementing Predictive QA Optimization
Implementing predictive QA optimization brings significant advantages that can enhance your quality assurance processes. First and foremost, it enables more accurate forecasting by analyzing historical data trends and identifying potential quality issues before they arise. This proactive approach not only reduces the time spent on reactive measures but also fosters a culture of continuous improvement.
Furthermore, predictive QA optimization enhances resource allocation. Organizations can focus their efforts where they're needed most, streamlining their processes and increasing efficiency. By leveraging data-driven insights, teams are better equipped to prioritize tasks and allocate resources wisely. Overall, adopting predictive analytics transforms quality assurance from a reactive function into a proactive strategy that drives operational excellence.
This shift towards a data-centric mindset empowers decision-makers with the tools they need to improve overall quality and customer satisfaction, making predictive QA optimization a vital component of modern QA programs.
Extract insights from interviews, calls, surveys and reviews for insights in minutes
Key Steps to Leverage Predictive Analytics for QA Optimization
To optimize your quality assurance program through predictive analytics, it's crucial to follow a structured approach. First, identify the key metrics and performance indicators that define quality within your organization. These metrics serve as a foundation for understanding quality trends and areas needing improvement. Clearly defined KPIs allow teams to focus on critical aspects, ensuring that efforts align with organizational goals.
Next, gather and analyze relevant data to draw insights about current quality processes. Employ advanced analytics tools to assess historical data, looking for patterns that indicate potential issues or improvements. Data analysis can reveal underlying problems that subtle quality evaluations might miss. By understanding these patterns, organizations can implement proactive measures that enhance overall QA effectiveness. This systematic approach not only streamlines processes but also positions your QA program for sustainable growth through predictive QA optimization.
Step 1: Identify Quality Metrics and KPIs
Identifying quality metrics and key performance indicators (KPIs) is crucial for effective Predictive QA Optimization. The first step involves determining which metrics will accurately reflect the quality of your product or service. Focus on both quantitative metrics, such as the number of defects per release, and qualitative measures, like customer satisfaction scores. Understanding these metrics allows teams to align their goals with organizational objectives and spot trends over time.
After identifying the right metrics, choose KPIs that will help track performance against those metrics. KPIs should be specific, measurable, achievable, relevant, and time-bound. For instance, tracking the defect rate during a specific development phase can provide insights into areas needing improvement. By establishing these metrics and KPIs, organizations can create a foundation for leveraging predictive analytics, ultimately driving better decisions and continuous quality enhancement.
Step 2: Collect and Analyze Data
Gathering and examining data is crucial in the predictive QA optimization process. Begin by compiling relevant data from various sources, such as customer feedback, product performance metrics, and historical quality records. This data lays the groundwork for gaining actionable insights. Understanding the different facets of your collected data enables you to pinpoint both strengths and weaknesses within your current QA programs.
Once the data is gathered, employ analytical tools to assess trends and patterns that may not be readily apparent. For example, comparing feedback across different locations can help unveil regional quality issues. Applying predictive analytics to these insights allows for informed decision-making about potential improvements and interventions. Ultimately, the goal is to transform raw data into valuable information that supports continuous enhancement within your QA processes.
Top Tools for Predictive QA Optimization
Choosing the right tools is essential to execute effective predictive QA optimization. Various platforms can assist in collecting, analyzing, and evaluating data at scale. These tools are designed to simplify the entire process, allowing teams to focus more on insights and less on manual tasks. For instance, tools like IBM Watson Analytics and SAS Predictive Analytics offer sophisticated algorithms that can uncover trends and forecast potential issues, thus enhancing the quality assurance process.
In addition to these options, RapidMiner provides an intuitive interface for both novice and experienced users, allowing for easy data manipulation and visualization. With these tools, teams can streamline evaluations against predefined criteria and ensure compliance with quality standards. Ultimately, adopting advanced technologies in predictive QA optimization leads to more reliable outcomes, increased efficiency, and a greater understanding of client needs.
insight7
Effective Predictive QA Optimization requires a comprehensive understanding of the data generated through quality assurance efforts. By identifying key quality metrics and employing advanced analytics, businesses can uncover actionable insights. This approach fosters a proactive stance toward quality issues, encouraging organizations to address potential problems before they escalate, ultimately improving customer satisfaction.
Transitioning to Predictive QA Optimization can significantly enhance collaboration within teams. With streamlined access to insights, decision-makers can quickly respond to quality signals and craft strategies grounded in real-time data. This shift not only minimizes inefficiencies but also empowers teams to focus on innovation and providing top-quality services. The results speak for themselves: organizations embracing predictive strategies witness a marked improvement in both operational efficiency and overall quality outcomes.
IBM Watson Analytics
Utilizing advanced technology can significantly enhance your quality assurance programs. IBM Watson Analytics offers robust capabilities for achieving this goal through predictive analytics. By harnessing its data visualization and querying features, teams can gain transformative insights related to product performance. This serves as a significant asset for implementing predictive QA optimization.
The platform empowers users to analyze trends and patterns in vast datasets with ease. You can compare results from different locations or product iterations, allowing for nuanced analysis and informed decision-making. By identifying both positive and negative customer feedback, teams can anticipate potential issues before they escalate. Ultimately, leveraging tools like these facilitates a proactive approach to QA, leading to improved customer satisfaction and product quality. Integrating such technologies into your QA processes ensures that you're not only reacting to issues but proactively optimizing your offerings.
SAS Predictive Analytics
SAS Predictive Analytics offers sophisticated capabilities designed to enhance the efficiency of quality assurance programs. By utilizing advanced algorithms and statistical techniques, organizations can analyze historical data and identify patterns critical for predictive QA optimization. Employing this powerful tool allows businesses to foresee potential quality issues before they become costly problems, ultimately streamlining processes and elevating product standards.
Through its intuitive interface, SAS enables users to seamlessly navigate complex datasets, facilitating informed decision-making. With the ability to visualize data trends, teams can proactively adjust their strategies to maintain high quality. Implementing SAS Predictive Analytics helps organizations shift from reactive QA measures to a more predictive approach, ensuring a robust quality framework that supports continuous improvement. This proactive stance not only mitigates risks but also fosters a culture of accountability and excellence in quality assurance practices.
RapidMiner
RapidMiner serves as an instrumental platform for organizations looking to implement Predictive QA Optimization effectively. Its capabilities allow teams to gather, process, and analyze vast amounts of data, transforming it into actionable insights. By utilizing its data mining and machine learning features, businesses can identify patterns that enhance product quality and customer satisfaction. This data-driven approach fosters a more precise evaluation of quality metrics and performance indicators.
Moreover, RapidMiner facilitates the visualization of insights, enabling teams to discern positive and negative trends within their QA processes. This clarity helps in making informed decisions that directly impact product development and user experience. By incorporating this tool into a QA program, organizations can optimize operations, reduce risks, and ultimately enhance the overall quality assurance process through predictive analytics. The integration of RapidMiner into the QA optimization framework underscores its importance in navigating today's competitive landscape.
Conclusion: Embracing Predictive QA Optimization for Future Growth
The future of quality assurance is undergoing a transformative shift with predictive QA optimization. Companies are realizing the immense value of harnessing data to proactively identify potential issues, rather than merely reacting to problems as they arise. By integrating predictive analytics into QA processes, organizations can anticipate customer needs and enhance product quality, driving sustainable growth.
Embracing this approach not only streamlines operations but also fosters a culture of continuous improvement. As businesses adapt to a more data-driven landscape, predictive QA optimization will become essential for staying ahead of competitors. Ultimately, organizations that prioritize this innovative strategy will better align with shifting market demands and position themselves for lasting success.