How to Optimize AI Agents for Predictive Customer Behavior Analysis
-
Bella Williams
- 10 min read
Predictive AI Optimization has emerged as a crucial tool for businesses seeking to enhance their understanding of customer behavior. By efficiently analyzing vast amounts of data, organizations can uncover patterns and trends that inform strategic decision-making. The result is a more personalized customer experience, driving engagement and loyalty among consumers.
In this section, we will explore the importance of optimizing AI agents for predictive analytics. We'll discuss various tools and strategies that help improve accuracy in predictions, ensuring businesses can respond effectively to changing consumer needs. Understanding these aspects will empower organizations to harness the full potential of Predictive AI Optimization, paving the way for data-driven success in a competitive market.
Analyze & Evaluate Calls. At Scale.
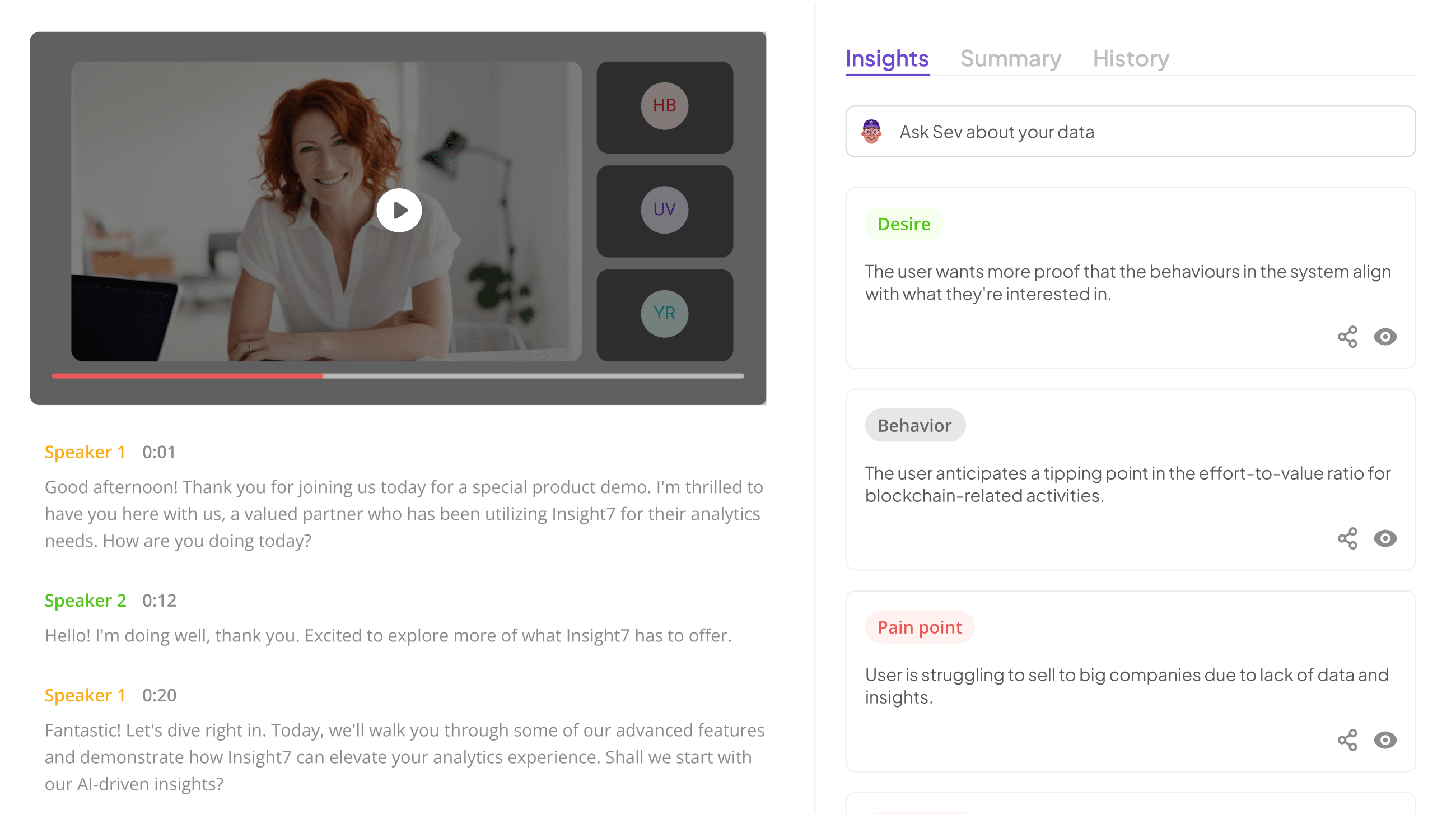
Understanding Predictive AI Optimization Tools
Predictive AI optimization tools are essential for analyzing customer behavior and enhancing decision-making. These tools rely on data-driven insights to forecast future customer actions, helping organizations adjust their strategies effectively. The crux of predictive AI optimization lies in the ability to analyze vast amounts of data, identifying patterns that inform marketing tactics and customer engagement strategies.
To utilize these tools effectively, businesses must focus on several key aspects. First, data quality is paramount; accurate, clean data leads to more reliable predictions. Secondly, algorithm selection plays a crucial role in the accuracy of forecasting models. It is important to choose algorithms that fit the specific needs of your business and customer demographics. Lastly, ongoing evaluation and adjustment of models ensure continued alignment with customer behavior trends, thus maximizing the value of predictive AI optimization tools.
Leveraging insight7 for Enhanced Predictions
To enhance predictions effectively, organizations must harness advanced analytical capabilities. The platform provides a user-friendly interface that facilitates comprehensive data analysis from customer interactions. By streamlining the process, companies can better utilize the large volume of customer signals generated today.
Successful predictive AI optimization relies on timely insights to stay competitive. By consolidating scattered information into a centralized platform, teams can collaborate more efficiently. The faster insights are translated into actionable strategies, the more effectively businesses can adapt to customer preferences and market shifts. This proactive approach not only improves service delivery but also cultivates a deeper understanding of customer needs, ensuring long-term success in customer-centric operations.
Exploring Other Key Tool Options
The efficiency of predictive customer behavior analysis often depends on the tools used for optimization. Exploring other key tool options is essential for organizations seeking to enhance their analytical capabilities. Many emerging platforms offer unique features that can improve data interpretation and customer insights significantly. For instance, IBM Watson Customer Experience Analytics provides advanced data visualization tools that help businesses detect patterns in consumer behavior effectively.
Another viable option includes Salesforce Einstein Analytics, which integrates seamlessly with existing CRM systems, enabling seamless data flow. H2O.ai and SAS Customer Intelligence 360 also present powerful capabilities for predictive AI optimization. Each tool offers distinct approaches to data analysis and customer insights, catering to different business needs. These tools not only facilitate deeper understanding of customer behaviors but also ensure that AI agents operate at their full potential. Evaluating these options will allow businesses to choose the best fit for their predictive analytics goals.
- IBM Watson Customer Experience Analytics
The role of IBM Watson Customer Experience Analytics is foundational in the realm of understanding and enhancing customer behavior. This advanced analytics platform synthesizes vast amounts of customer data, translating it into actionable insights that drive decision-making. By utilizing machine learning algorithms, businesses can better predict customer preferences and behaviors, allowing them to tailor marketing strategies effectively. The platform enables organizations to combine data sources, offering a comprehensive view of customer interactions and preferences.
Implementing predictive AI optimization through this analytics platform involves several critical steps. First, organizations must prepare their data meticulously, ensuring it is relevant and free of inaccuracies. Next, the algorithm selection process comes into play, where businesses choose and train machine learning models to analyze customer behavior accurately. Ultimately, these processes enhance the customer experience, fostering loyalty and driving growth. By continually refining these analytics capabilities, companies can stay aligned with shifting market demands and improve engagement.
- Salesforce Einstein Analytics
Salesforce Einstein Analytics serves as a powerful platform for enhancing predictive AI optimization in customer behavior analysis. It provides businesses with the ability to derive actionable insights from their customer data. By leveraging advanced analytics and machine learning, organizations can analyze trends and make informed decisions that enhance customer experiences.
With its intuitive dashboards and customizable reports, Einstein Analytics allows users to visualize data effectively. This means that business leaders can quickly interpret and react to customer trends, driving better engagement strategies. Additionally, the platform enables real-time data processing, allowing companies to adapt their marketing and service strategies on the fly, which is crucial for staying ahead in a competitive market. Ultimately, harnessing Salesforce Einstein Analytics can significantly elevate an organization's capacity to predict customer behavior and refine its overall operational strategy.
- H2O.ai
Predictive AI Optimization involves harnessing advanced tools that allow businesses to analyze customer behavior in real time. One such platform excels in delivering robust analytical capabilities tailored for understanding customer interactions. This tool enables businesses to predict trends and behaviors by utilizing machine learning algorithms that process and interpret vast amounts of data.
To fully leverage this platform, organizations should focus on maximizing its features. Firstly, understanding the unique functionalities it offers can significantly enhance data-driven decision-making. Secondly, optimizing the integration process with existing systems ensures seamless data flow, maximizing the utility of customer insights. Lastly, regular evaluations of predictive outcomes play a critical role in continuously adjusting strategies for improved customer engagement. By utilizing these strategies, businesses can transform raw data into actionable insights, paving the way for informed marketing and sales tactics.
- SAS Customer Intelligence 360
SAS Customer Intelligence 360 presents a comprehensive suite of tools that enhance Predictive AI Optimization. By capturing detailed customer interactions, it provides invaluable insights that drive data-driven decision-making. As a platform, it not only allows seamless data integration but also efficiently transcribes customer calls into actionable reports. This functionality enables companies to adapt their strategies based on real-time feedback.
Moreover, the platform emphasizes the importance of transforming traditional sales tactics into a consultative approach. This shift fosters a deeper connection with customers and encourages meaningful conversations. Utilizing advanced analytics, businesses can identify patterns in customer behavior and tailor their marketing efforts accordingly. Incorporating SAS Customer Intelligence 360 into your strategy will significantly enhance the effectiveness of customer engagement initiatives, paving the way for more informed and responsive business practices.
💬 Questions about How to Optimize AI Agents for Predictive Customer Behavior Analysis?
Our team typically responds within minutes
Extract insights from interviews, calls, surveys and reviews for insights in minutes
Implementing Predictive AI Optimization: Key Strategies
Implementing Predictive AI Optimization requires a structured approach to ensure effective customer behavior analysis. Begin with data preparation and management, focusing on collecting relevant customer data. This involves recognizing what specific information aligns with your goals. Ensuring data quality and cleanliness is equally vital; reliable outcomes depend on accurate datasets free from errors.
Next is algorithm selection and training. Identify suitable machine learning algorithms tailored for your predictive modeling needs. Understanding the nuances of various algorithms helps in making informed decisions. Subsequently, invest time in training models that accurately represent customer behavior patterns. By refining these models continuously, you can enhance their predictive capabilities. This structured strategy can significantly improve the effectiveness of AI agents, ultimately leading to better insights and informed decision-making.
Data Preparation and Management
Data preparation is crucial for optimizing AI agents in predictive customer behavior analysis. The first step involves collecting relevant customer data from various sources. This might include customer surveys, social media interactions, and transaction histories. Ensuring a comprehensive data set will allow for more accurate and targeted predictive modeling.
Following data collection, the focus must shift to ensuring data quality and cleanliness. It’s essential to identify and address any inconsistencies or missing values within the data to foster reliability. When data is clean, the algorithms used for predictive modeling can function more effectively, leading to improved insights and forecasts. Regular audits of the data management process can help in maintaining the integrity needed for successful predictive AI optimization.
Step 1: Collecting Relevant Customer Data
Collecting relevant customer data forms the foundation for effective predictive AI optimization. Businesses must first identify which customer information is most pertinent to their objectives, such as demographics, preferences, and behaviors. Valuable data sources can include customer interactions, surveys, and feedback collected through various touchpoints. Analyzing these data points offers insights that enhance understanding of customer needs and improve service delivery.
Once the right data has been identified and collected, it is essential to structure it for optimal analysis. This structure can aid in predicting future behavior and tailoring marketing strategies accordingly. By establishing a strong dataset, organizations can leverage AI tools to forecast customer trends more accurately. Effective data collection not only drives predictive modeling but also serves as a vital element in creating personalized customer experiences that foster loyalty and engagement.
Step 2: Ensuring Data Quality and Cleanliness
Maintaining data quality and cleanliness is a vital aspect of predictive AI optimization. The efficacy of AI agents in analyzing customer behavior hinges on accurate, timely, and reliable data. First, it is essential to establish robust data governance, which includes standardizing data sources and ensuring uniformity. Effective data cleansing involves identifying and rectifying errors, inconsistencies, or redundancies that may distort insights. This step can dramatically improve the reliability of predictive models.
Second, continuous monitoring of data inputs is crucial. Regular audits and checks help maintain the integrity of the datasets, ensuring they reflect customer dynamics accurately. Engaging data specialists for oversight can bolster this process. When organizations commit to high standards in data management, they empower predictive AI systems to deliver more precise forecasts and actionable insights. Ultimately, this diligence enhances the overall customer experience by enabling businesses to respond to changing behaviors effectively.
Algorithm Selection and Training
Selecting the right algorithms and training them meticulously is crucial in optimizing predictive AI for customer behavior analysis. With numerous machine learning models available, the challenge lies in identifying which algorithms best suit your specific goals. Some popular choices include decision trees, neural networks, and ensemble methods. These models can significantly enhance predictive accuracy when tailored to your unique data set and business requirements.
Once the algorithms are chosen, training them becomes the next significant step. This involves feeding the models with high-quality customer data, ensuring they learn from accurate patterns and trends. Regularly updating these models with fresh data is vital to maintain their effectiveness. Balancing the complexity of the model with performance efficiency will help drive meaningful insights. Emphasizing both algorithm selection and model training establishes a strong foundation for predictive AI optimization, empowering your strategies in customer behavior analysis.
Step 1: Choosing Appropriate Machine Learning Algorithms
Choosing the right machine learning algorithms is crucial for effective predictive AI optimization in customer behavior analysis. Start by identifying the specific goals of your predictive model. Are you looking to improve customer engagement, predict churn, or personalize experiences? Different algorithms excel in various scenarios, influencing the accuracy and effectiveness of your insights.
Next, consider data characteristics such as volume, variety, and velocity. For instance, supervised learning algorithms, like decision trees and linear regression, are ideal when labeled data is available. Alternatively, unsupervised methods like clustering can uncover patterns within unlabelled data. It’s essential to evaluate performance metrics, including accuracy, precision, and recall, to select the best-fitting algorithm for your needs. By thoughtfully selecting appropriate algorithms, you can significantly enhance your AI-driven analysis, paving the way for informed business decisions.
Step 2: Training Models for Customer Behavior Prediction
Training models for customer behavior prediction involves a detailed process that integrates various data sources and advanced algorithms. This step is crucial for predictive AI optimization because it sets the foundation for effective customer insights. Begin by gathering a broad spectrum of customer data, including demographic information and purchasing history. This data will inform the algorithms you select, enabling them to identify patterns indicative of customer behavior.
Once the data is collected, the next phase involves choosing the right machine learning algorithms that align with your goals. Algorithms like decision trees, logistic regression, or neural networks can be employed depending on the complexity of the behavior you aim to predict. As the models are trained, continuous evaluation of their accuracy is essential to refine and improve predictions. This iterative process not only enhances customer understanding but also drives timely strategic decisions. By meticulously training your models, you lay the groundwork for more meaningful interactions with customers and a competitive edge in the market.
Conclusion: The Future of Predictive AI Optimization in Customer Analytics
Predictive AI Optimization in customer analytics is poised to revolutionize how organizations understand consumer behavior. By harnessing advanced algorithms and data-driven insights, businesses can anticipate customer needs with unprecedented accuracy. This proactive approach leads to more meaningful interactions, enhancing customer loyalty and satisfaction.
Looking ahead, the integration of predictive AI will likely become a cornerstone for strategic decision-making. Companies embracing these technologies will differentiate themselves by not just responding to trends but predicting them. As data collection and analysis tools evolve, organizations must remain agile, leveraging innovations to continuously refine their predictive capabilities. Embracing this future ensures businesses stay relevant in an ever-changing marketplace.
💬 Questions about How to Optimize AI Agents for Predictive Customer Behavior Analysis?
Our team typically responds within minutes