How to Measure QA Reviewer Accuracy With AI Confidence Scores
-
Hello Insight
- 10 min read
In the dynamic realm of quality assurance (QA), understanding AI QA accuracy through confidence scores is paramount. As organizations increasingly rely on AI technologies, evaluating the precision of their QA reviewers becomes essential. By utilizing these confidence scores, stakeholders can make informed decisions about reviewer performance, identify areas for improvement, and enhance the overall QA process.
AI QA accuracy is not solely about delivering data; it's about contextualizing it for actionable insights. Confidence scores provide quantifiable measures, offering a clear perspective on how effectively reviewers are performing. This section will explore the interplay between AI and QA, shedding light on the transformative capabilities of AI confidence scores in fostering accuracy and reliability in quality assurance endeavors.
In the ever-evolving field of quality assurance (QA), the integration of artificial intelligence (AI) offers innovative approaches to measure reviewer accuracy. This blog post will delve into how AI confidence scores can accurately assess the effectiveness and accuracy of QA reviewers.
In the dynamic realm of quality assurance (QA), organizations are increasingly turning to artificial intelligence (AI) to enhance reviewer accuracy. AI confidence scores have emerged as a game-changing tool that provides quantifiable measures of a reviewer's performance. By leveraging these scores, companies can gain insights into where reviewers excel and where further training may be necessary.
Understanding the function of AI confidence scores is essential for organizations aiming to improve QA accuracy. These scores evaluate a reviewer's decisions against established benchmarks, offering a clearer picture of effectiveness. As companies integrate these advanced metrics into their processes, they can enhance not only the precision of their reviews but also the overall quality of their outcomes. This innovative approach heralds a future where AI QA accuracy becomes a central pillar of effective quality management.
Analyze & Evaluate Calls. At Scale.
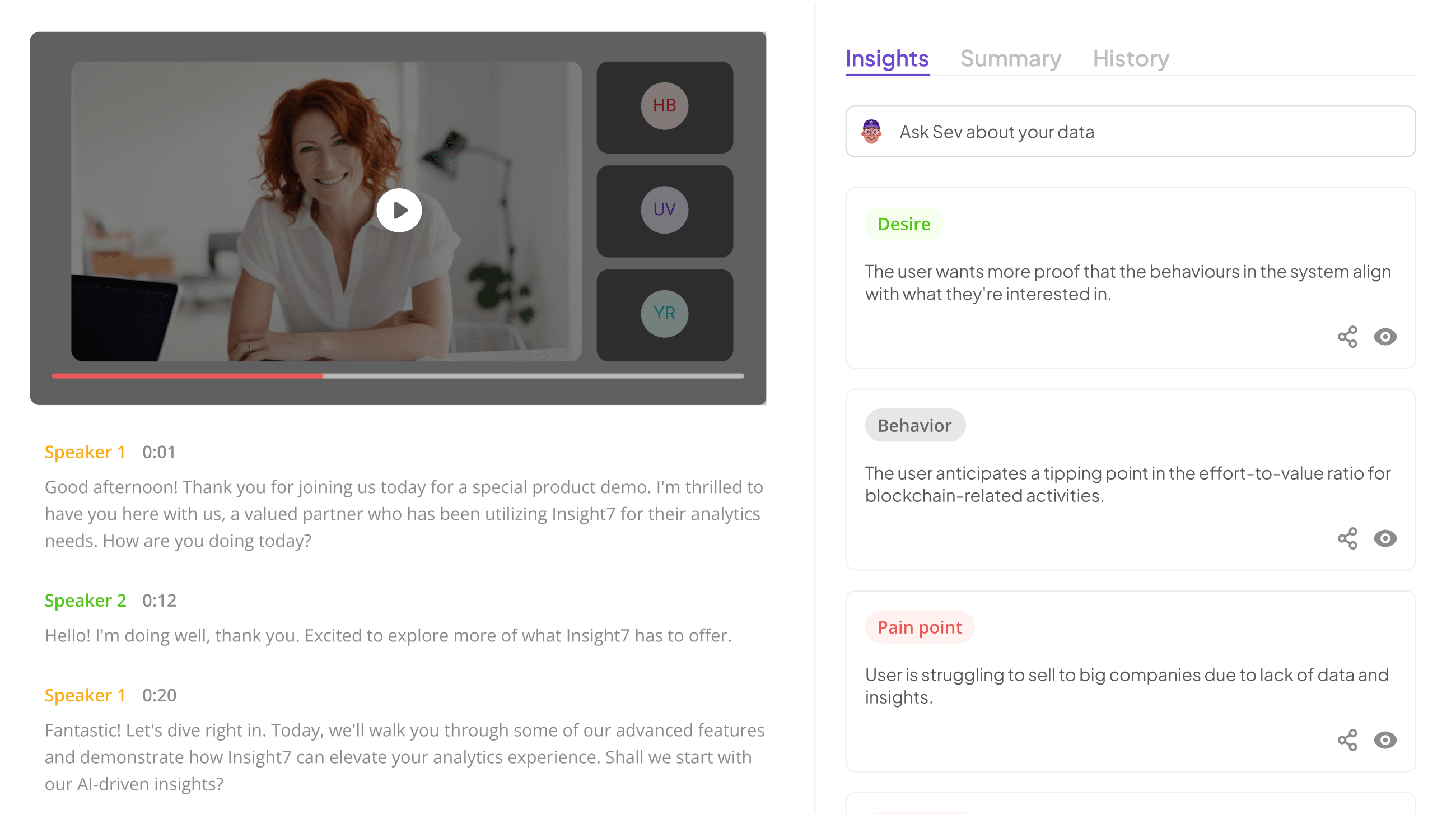
How AI QA Accuracy is Enhanced with Confidence Scores
AI-driven tools are revolutionizing the quality assurance (QA) landscape by integrating confidence scores into their evaluation processes. These scores serve as objective metrics, revealing the levels of certainty AI has regarding a QA reviewer's accuracy. By assigning a numerical value to predictions, confidence scores guide teams in identifying both strengths and weaknesses within their workflows. This results in enhanced AI QA accuracy, as reviewers can focus on areas needing improvement based on these insights.
Moreover, the infusion of confidence scores into QA processes enables organizations to make data-driven decisions for training efforts and resource allocation. For example, if a reviewer consistently receives low confidence scores, it may signal the need for targeted training or mentorship. Thus, confidence scores not only promote accountability but also foster a culture of continuous improvement. As AI's capabilities evolve, integrating these scores will play a pivotal role in refining QA accuracy and elevating overall performance.
AI-driven tools are revolutionizing the way QA processes are conducted. By utilizing confidence scores, AI can provide objective metrics that highlight areas of accuracy and improvement for QA reviewers.
AI-driven tools are reshaping the quality assurance (QA) landscape by streamlining the review process. These innovative technologies deploy confidence scores, which serve as objective metrics that clearly indicate the performance of QA reviewers. This data-driven approach spots areas of strength and highlights opportunities for improvement, leading to more effective quality assessments.
Utilizing AI confidence scores can drastically enhance QA accuracy. By offering quantifiable insights, these scores enable organizations to set precise benchmarks for reviewer performance. This not only motivates QA reviewers to improve their accuracy but also fosters an environment of continuous feedback and learning. As confidence scores reveal patterns in reviewer behavior, teams can strategically allocate resources and training efforts, ultimately fostering an approach rooted in improvement and excellence in QA processes. The integration of these AI tools ensures that QA dynamics are more transparent, accountable, and geared toward achieving optimum results.
The Role of AI Confidence Scores in Quality Assurance
AI confidence scores play a critical role in enhancing quality assurance (QA) processes by providing measurable insights into reviewer accuracy. These scores, generated from data-driven analysis, indicate how confident AI is about the assessments made by QA reviewers. This information helps to identify strengths and weaknesses in the reviews, allowing organizations to refine their QA methodologies. By leveraging AI confidence scores, companies can ensure that their QA processes not only uphold high standards but also constantly improve.
To effectively incorporate AI confidence scores into QA practices, several key steps are necessary. First, organizations should focus on thorough data collection and preprocessing to ensure high-quality input. Second, training and calibrating AI models is crucial for accurate predictions. Third, seamless integration with existing QA processes allows for a smooth transition and operation. Finally, a robust analysis and interpretation of the confidence scores provide actionable insights, directly influencing AI QA accuracy. By following these steps, companies can substantially enhance their QA processes and outcomes.
Understanding how AI confidence scores function is crucial to implementing them in QA processes. Well explore the mechanics behind these scores and their impact on evaluating reviewer accuracy.
AI confidence scores represent a pivotal advancement in evaluating QA reviewer accuracy. Understanding how these scores function is essential for effective implementation in quality assurance processes. At the core, confidence scores quantify the certainty an AI system has regarding the quality of a review, providing a numerical value that indicates potential error rates. This measurement can guide managers in pinpointing areas needing improvement while assessing the overall effectiveness of their QA teams.
The mechanics behind these scores involve various algorithms that analyze reviewer performance against established benchmarks. By leveraging historical data and patterns, AI can produce confidence scores that reflect reviewer strengths and weaknesses. This data-driven approach not only streamlines decision-making but also fosters a culture of accountability within QA teams. Ultimately, a thorough comprehension of AI QA accuracy through confidence scores enhances the ability to maintain high standards in quality assurance efforts.
Implementing Steps to Measure QA Accuracy
To measure QA accuracy effectively, organizations must undertake a systematic approach that prioritizes data integrity and analysis. The first step involves data collection and preprocessing. Gathering high-quality transcripts from various channels ensures that the AI system has a solid foundation for measuring QA reviewer performance. Once the data is structured, the next phase is AI model training and calibration. This process fine-tunes the AI algorithms to understand nuances in the QA criteria, enhancing the overall accuracy of confidence scoring.
Following this, integration with existing QA processes becomes crucial. By embedding AI confidence scores into daily QA evaluations, organizations can create a seamless workflow that leverages technology without disrupting established practices. Finally, analysis and interpretation of these confidence scores provide actionable insights into reviewer performance. This comprehensive approach guarantees that AI QA accuracy isn't just a theoretical measurement but a practical tool for continuous improvement in quality assurance efforts.
To effectively utilize AI confidence scores, organizations need to follow specific steps to ensure proper implementation and analysis. Below are the main steps involved:
To effectively utilize AI confidence scores in measuring QA reviewer accuracy, it is essential to approach the implementation strategically. First, organizations should focus on data collection and preprocessing. Gathering quality data that represents various scenarios ensures that the AI model can learn effectively. This step also involves cleaning the data to eliminate any noise that could interfere with model performance.
Next, organizations must engage in AI model training and calibration. This involves training the AI tools to recognize patterns and make predictions regarding reviewer accuracy. Regular calibration of the models will help maintain their accuracy and adjust for any changes in the QA process.
Following model training, the integration of these tools with existing QA processes should take place. It is crucial that AI confidence scores are seamlessly incorporated into the workflow to provide insights without disrupting the current systems. Finally, analysis and interpretation of confidence scores allow for targeted improvements. By understanding these scores, teams can identify strengths and weaknesses within their QA efforts, paving the way for continual enhancement of AI QA accuracy.
- Data Collection and Preprocessing
Data collection and preprocessing are foundational steps in evaluating AI QA accuracy. The initial phase involves gathering relevant data that accurately reflects the QA activities under review. This data should include various QA reviews, reviewer comments, and the corresponding outputs produced by the system. Diverse datasets will enhance the validity of the AI confidence scores used later in the analysis.
Following data collection, preprocessing is essential for ensuring the dataset's quality. This involves cleaning the data to remove any inconsistencies or irrelevant information. Properly prepared data allows AI models to learn effectively and provides reliable inputs for generating accuracy assessments. This detailed attention during preprocessing allows QA teams to derive meaningful insights from AI confidence scores, ultimately fostering improved reviewer performance and quality assurance processes.
- AI Model Training and Calibration
In the context of AI QA accuracy, model training and calibration are critical processes that directly influence reviewer performance. Initially, a robust dataset must be gathered, ensuring it includes a diverse array of scenarios and outcomes relevant to quality assurance. This data serves as the foundation upon which the AI model learns and develops its predictive abilities. Subsequently, training the model involves iteratively adjusting its parameters based on this data to enhance its accuracy in assessing QA reviewer performance.
Calibration follows training and is essential for fine-tuning the AI's predictions. This process aligns the output confidence scores with actual performance metrics. For instance, if an AI model consistently rates reviewer accuracy too high, calibration will help adjust its scoring to better reflect true outcomes. By effectively training and calibrating AI models, organizations can achieve a clear understanding of QA reviewer accuracy, enabling data-driven insights that foster continuous improvement in quality assurance processes.
- Integration with Existing QA Processes
Integrating AI confidence scores into existing QA processes is essential for enhancing accuracy and efficiency. Organizations must first assess their current QA methods and determine compatibility with AI solutions. This evaluation ensures that any new tools introduced will seamlessly align with established workflows.
Next, teams should prioritize training on the AI systems to foster familiarity among QA reviewers. By understanding how AI confidence scores are calculated and applied, reviewers can leverage this technology to refine their assessments. Continuous feedback loops, incorporating regular reviews of AI outputs, can further enhance reviewer skills and confidence. By making AI QA accuracy an integral part of the QA culture, organizations can significantly improve their measurement of reviewer performance while maintaining high standards in quality assurance.
Ultimately, successful integration hinges on a thoughtful strategy that emphasizes collaboration between technology and human expertise, creating a harmonious balance in their QA processes.
- Analysis and Interpretation of Confidence Scores
Analyzing and interpreting confidence scores is essential in understanding AI QA accuracy. Confidence scores provide insights into how well AI models predict the effectiveness of QA reviewers. These scores help organizations identify both strengths and weaknesses in their evaluation processes, fostering continuous improvement. By examining these metrics, teams can gain clarity on which aspects of the QA process yield reliable results and which require attention.
When interpreting these scores, it is important to focus on three key areas. First, contextual awareness determines how confidence scores are influenced by various factors, like the quality of input data. Second, establishing benchmark thresholds aids in comparing performance over time and against industry standards. Lastly, involving human reviewers in the assessment ensures a balanced evaluation, adding a layer of qualitative insight to the quantitative data provided by AI systems. This comprehensive approach enhances overall QA accuracy, helping teams achieve their operational goals effectively.
Extract insights from interviews, calls, surveys and reviews for insights in minutes
AI QA Accuracy Tools and Technologies
AI QA Accuracy tools and technologies are transforming the way organizations evaluate the effectiveness of their QA reviewers. These innovative solutions leverage artificial intelligence to assess and enhance reviewer performance through objective metrics, paving the way for better quality assurance processes. A central element of these tools is the generation of AI confidence scores, which indicate the level of certainty AI has regarding a reviewer’s performance.
The diverse landscape of AI technologies includes various powerful solutions designed to support these confidence scoring methods. Prominent examples, such as insight7, CheckMate, DeepQA, ReviewMint, and QualiTrack, offer unique features that help organizations analyze data effectively. By utilizing these AI-driven tools, QA teams can pinpoint areas for improvement, streamline their workflows, and ultimately improve the overall accuracy of their quality assurance efforts. This technological advancement is key to fostering a robust QA environment that harnesses data-driven insights for continuous enhancement.
The landscape of AI tools for measuring QA accuracy is diverse. Here, we will highlight some of the top technologies that support confidence scoring in QA reviews.
In the ever-expanding realm of AI tools for evaluating QA accuracy, several standout technologies are reshaping how reviewers are assessed. These tools employ AI confidence scoring, which offers a systematic approach to gauge the effectiveness of quality assurance. By leveraging both structured data and predictive analytics, these technologies can identify patterns and highlight areas needing improvement. This capability translates into real-time insights that help organizations refine their QA processes.
Among the notable tools in this space are systems like CheckMate and DeepQA, which specialize in scoring reviewer performance based on customized evaluation criteria. These platforms enable organizations to define parameters crucial for their specific QA processes, ensuring a tailored review mechanism. With capabilities to automate feedback and suggest corrective actions, these tools not only enhance QA efficiency but also help in cultivating a culture of continuous improvement within teams. As businesses increasingly rely on AI for QA assessments, adopting these technologies is key to fostering greater accuracy and reliability in quality reviews.
Leading Tools for Enhancing QA Accuracy
To enhance QA accuracy, organizations increasingly turn to sophisticated AI-driven tools designed for better evaluation. Leading tools like insight7, CheckMate, DeepQA, ReviewMint, and QualiTrack offer features that integrate AI confidence scores into the QA review process. These tools not only automate assessments but also provide insight into reviewer performance and areas needing improvement.
Among them, insight7 stands out for its user-friendly interface, allowing easy data input and analysis. Its customization options ensure that companies can tailor evaluations to their specific needs. CheckMate employs advanced algorithms to analyze calls against predetermined criteria, offering concrete metrics for compliance and accuracy. Each tool has unique capabilities, but collectively, they represent a significant step toward achieving higher AI QA accuracy. Incorporating such tools into QA workflows ensures that organizations can maintain rigorous standards and continuously improve their review processes.
The following tools offer robust capabilities to utilize AI confidence scores effectively:
The integration of advanced tools enhances the effectiveness of AI confidence scores in assessing QA reviewer accuracy. Each tool has unique features that streamline the measurement process and provide insightful data. For instance, tools like CheckMate and DeepQA focus on analyzing communication patterns and outcomes to derive meaningful scores. These tailored features help users pinpoint specific strengths and weaknesses within their QA teams.
Moreover, the significance of these tools lies not just in their ability to generate confidence scores but also in how they present this data. Solutions such as ReviewMint and QualiTrack offer customizable dashboards and report formats, allowing organizations to edit and distribute findings efficiently. This flexibility enables businesses to align AI QA accuracy with their operational needs and establish a more reliable feedback loop for continuous improvement. Ultimately, the thoughtful application of these tools fosters enhanced accuracy and quality within review processes.
- insight7
In the realm of quality assurance, understanding how AI QA accuracy can be measured is essential for success. Insight7 emerges as a powerful platform that simplifies the evaluation of reviewer performance through the use of AI confidence scores. Implementing this innovative approach helps teams pinpoint areas requiring improvement while fostering a more efficient review process.
To achieve optimal results, organizations should focus on specific strategies. First, establish a foundation with robust data collection and preprocessing practices. Next, invest in AI model training and calibration to deliver accurate confidence scoring. Subsequently, ensure seamless integration with existing QA processes. Finally, continuously analyze and interpret these confidence scores to facilitate informed decision-making. Embracing AI confidence scores not only enhances reviewer accuracy but also aligns your quality assurance efforts with modern technology, positioning your organization for ongoing success.
- CheckMate
In the journey of understanding AI QA accuracy, CheckMate emerges as a pivotal player in refining the evaluation process. This tool harnesses advanced algorithms to measure not just the performance of QA reviewers, but also the reliability of their assessments through AI confidence scores. By analyzing conversation patterns and outcomes, CheckMate provides vital insights into reviewer efficiency and areas for potential enhancement, thereby promoting a cycle of continuous improvement.
To ensure optimal utility of CheckMate, users should focus on three fundamental aspects:
- Data Integrity: Secure high-quality, relevant data inputs to maximize the accuracy of assessments.
- Benchmark Establishment: Develop clear performance criteria that align with organizational goals and standards.
- Iterative Feedback: Utilize the insights generated to create a feedback loop that continuously enhances reviewer training and practices.
By adopting CheckMate, organizations can enhance their understanding of QA reviewer capabilities and foster a culture focused on growth and precision.
- DeepQA
DeepQA is a cutting-edge tool dedicated to enhancing AI QA accuracy by utilizing advanced algorithms to evaluate reviewer performance. By leveraging extensive data analysis, DeepQA offers precise insights into the decision-making processes of QA reviewers. This ensures that the effectiveness of reviews can be quantified, allowing organizations to make informed adjustments in their quality assurance strategies.
The integration of DeepQA into existing QA frameworks enhances real-time feedback on reviewer accuracy, which is critical for maintaining high standards. Additionally, this tool assists in identifying patterns of common errors, enabling targeted training for QA teams. By focusing on AI QA accuracy, DeepQA not only streamlines the review process but also promotes continuous improvement. Ultimately, organizations can expect improved outcomes and a more effective QA system by implementing such advanced technologies.
- ReviewMint
In the realm of quality assurance, tools that enhance AI QA accuracy play a pivotal role in refining processes. Among these tools, ReviewMint stands out for its user-friendly interface, designed to democratize insights across the organization. By simplifying access to data, it allows team members—regardless of expertise—to analyze and extract valuable insights seamlessly.
The core functionality of ReviewMint revolves around capturing and visualizing conversations, enabling teams to pinpoint customer pain points, behaviors, and desires. The platform excels in summarizing insights through evidence-backed narratives, making it easier for users to derive actionable conclusions. By aggregating multiple files into projects, ReviewMint allows teams to evaluate hundreds of calls efficiently, revealing trends and overall performance metrics. This approach enhances AI QA accuracy, providing organizations with a robust framework for ongoing quality enhancement.
- QualiTrack
QualiTrack stands out as a powerful solution for assessing AI QA accuracy. By systematically tracking the performance of QA reviewers, it enables organizations to identify strengths and weaknesses in their review processes. This tracking not only highlights areas needing improvement but also builds confidence in the accuracy and reliability of the QA results.
The functionality of QualiTrack is rooted in its robust data analysis capabilities. When integrating AI confidence scores with traditional evaluation methods, QA teams gain insights that are both quantitative and qualitative. This combination allows for a more nuanced understanding of reviewer performance. By implementing QualiTrack, organizations can foster a culture of continuous improvement, ensuring that QA processes evolve in alignment with the company's standards and goals. As a result, the overall quality assurance outcomes are enhanced, leading to better customer satisfaction and operational efficiency.
Conclusion: The Future of AI QA Accuracy
The future of AI QA accuracy looks promising as technology continues to evolve. By harnessing AI confidence scores, organizations can expect a significant improvement in measuring and enhancing the accuracy of quality assurance reviewers. This advancement shifts the focus towards data-driven insights, empowering businesses to make informed decisions about their QA processes.
Incorporating AI tools that calculate confidence scores not only streamlines existing workflows but also enhances compliance and performance evaluation. As businesses adapt to these innovations, the overall quality of output will improve, reinforcing consumer trust. Ultimately, the integration of AI will redefine the standards of accuracy in quality assurance practices.
As AI technology continues to progress, its role in enhancing QA accuracy and efficiency is only set to grow. Emphasizing AI confidence scores allows for more accurate, reliable, and scalable quality assurance processes. Integrating these advanced tools will enable businesses to maintain high standards in their QA efforts.
As AI technology advances, its contribution to improving QA accuracy and efficiency is undeniably significant. The use of AI confidence scores enables QA processes to become more precise and dependable, leading to enhanced overall effectiveness. When teams incorporate these scores into their operations, they move towards a model that not only identifies inaccuracies but also provides concrete metrics for improvement.
The process of integrating AI confidence scores is transformative for businesses. By establishing clear benchmarks through these scores, organizations can ensure that their QA efforts align with the highest standards. This integration fosters scalability and consistency, empowering teams to manage increasing volumes of quality assurance with confidence. Ultimately, the enhanced accuracy provided by AI will facilitate better products and services while enabling businesses to optimize their resources for sustained excellence in quality assurance.