How to Implement Predictive Analytics in Contact Centers
-
Bella Williams
- 10 min read
Predictive analytics implementation in contact centers represents a transformative approach to enhancing customer interactions. Through the effective analysis of historical data, contact centers can anticipate customer needs, streamline processes, and improve decision-making. This level of data-driven insight can significantly elevate the customer experience and operational efficiency.
Understanding the underlying principles of predictive analytics is crucial for successful implementation. By systematically gathering and analyzing customer data, organizations can identify trends and patterns that inform strategic initiatives. Adopting predictive analytics not only helps in addressing immediate concerns but also positions contact centers for long-term growth by continually adapting to changing customer expectations.
Analyze & Evaluate Calls. At Scale.
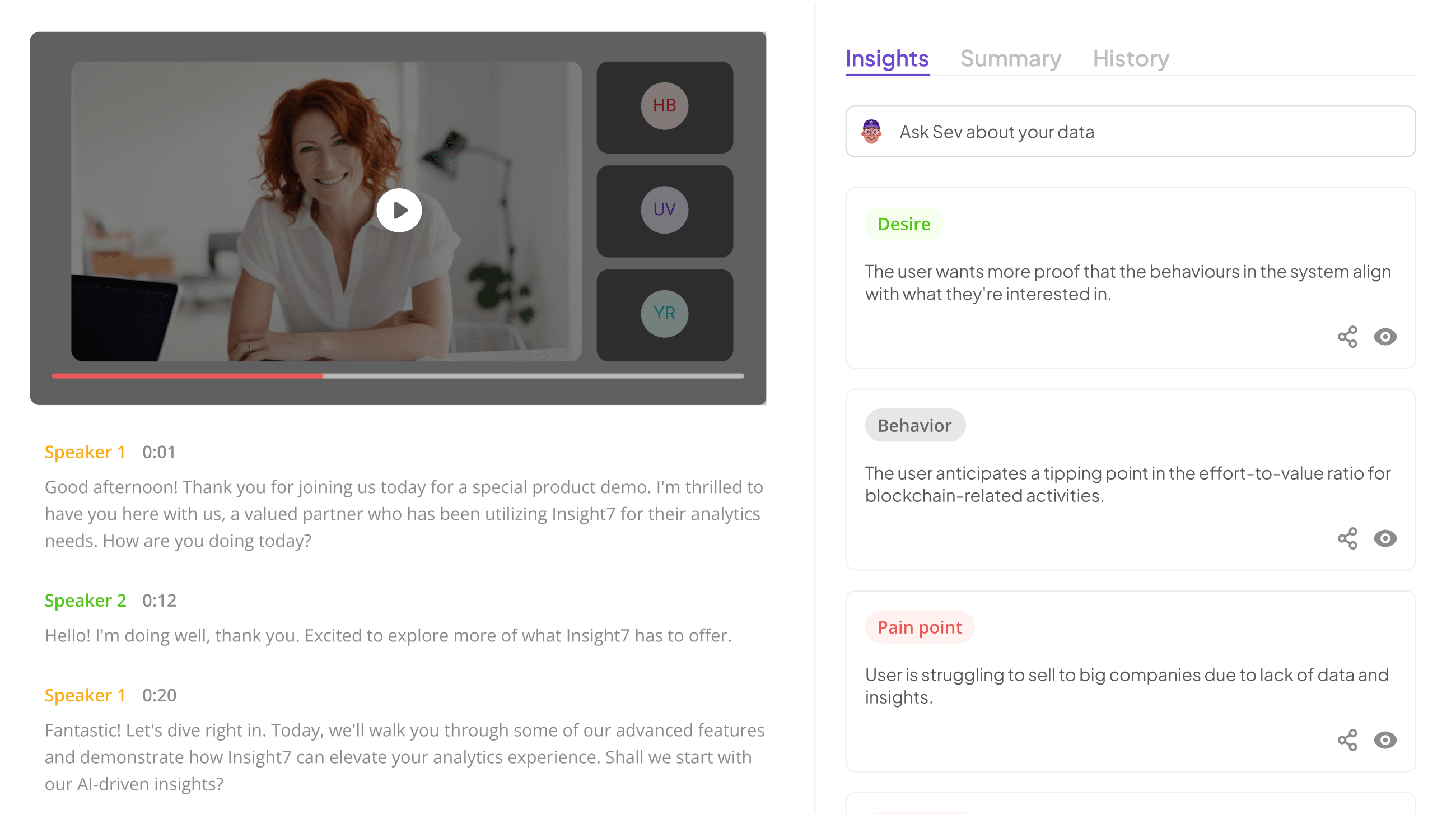
Understanding the Essentials of Predictive Analytics Implementation
Predictive Analytics Implementation requires a clear understanding of its fundamental principles and methodology. To start, understanding your business objectives is essential; this will define what you aim to achieve through your analytics efforts. Clearly articulated goals help in aligning data strategies with desired outcomes, ensuring that the implementation focuses on relevant metrics.
Another critical element is data collection. High-quality data forms the backbone of any predictive analytics project. Itโs important to manage and organize this data properly for effective analysis. Subsequently, the model-building phase involves selecting appropriate algorithms to interpret the data and predict future trends. Continuous monitoring post-deployment is equally vital, allowing for real-time adjustments and improvements based on actual performance. Each of these steps creates a solid foundation for effective predictive analytics, positioning contact centers to enhance decision-making and customer experience.
The Core Technologies Behind Predictive Analytics Implementation
To effectively execute predictive analytics implementation in contact centers, several core technologies are essential. First, robust data management systems play a vital role in collecting and organizing large volumes of customer interaction data. This data serves as the foundation for analysis, ensuring accurate insights into customer behavior and preferences.
Another critical technology is machine learning algorithms, which process the data to identify patterns and predict future outcomes. These algorithms enhance decision-making by allowing contact centers to anticipate customer needs, ultimately improving service quality. Additionally, data visualization tools provide an intuitive way to interpret the results, making it easier for teams to derive actionable insights from complex data sets.
Lastly, cloud computing technologies ensure scalability and flexibility in data analysis, enabling real-time predictions and streamlining operations. By integrating these technologies, contact centers can harness predictive analytics for more personalized customer experiences and optimized workflows.
Steps to Start Predictive Analytics Implementation in Contact Centers
To begin your predictive analytics implementation in contact centers, it's essential first to identify your business objectives. Clearly defined goals help shape the direction of your project and ensure alignment with overall business strategies. Next, focus on data collection and management by gathering relevant customer interaction data. This data should be accurate, comprehensive, and well-organized to facilitate further analysis.
Once your data is in place, model building and testing come next. This step involves selecting appropriate algorithms and creating predictive models based on the data analysis. Evaluate the performance of these models to ensure they meet your accuracy requirements before moving to the final stage. Finally, deployment and monitoring are crucial to maintain the effectiveness of your predictive analytics. Regularly review the models, assess their impact on customer interactions, and make necessary adjustments for continuous improvement. Following these steps ensures a structured and effective approach to predictive analytics implementation in your contact center.
- Identifying Business Objectives
Identifying business objectives is a crucial step in the predictive analytics implementation process. First, organizations need to clarify their goals by asking fundamental questions about what they hope to achieve. This could include improving customer satisfaction, reducing operational costs, or increasing sales. Each objective should be specific, measurable, attainable, relevant, and time-bound to ensure clarity and focus throughout the implementation.
Next, consider the metrics that will gauge success. By understanding key performance indicators, businesses can align their predictive analytics strategies effectively. A practical approach includes defining how predictive analytics will help meet these objectives. For example, utilizing data insights can enhance agent performance and improve customer interactions. Overall, clearly identifying business objectives lays the foundation for effective predictive analytics implementation, driving impactful outcomes for contact centers.
- Data Collection and Management
Successful predictive analytics implementation relies heavily on effective data collection and management strategies. Begin by identifying the various data sources available, including call transcripts, customer feedback, and interaction history. Properly organizing this data is essential to facilitate insightful analysis later on. All data points should be aggregated into a centralized system that allows for easy access and manipulation as needed.
Next, implement standardized processes for managing and refining the incoming data. This includes regularly updating the data repositories and ensuring that data quality meets the required standards. By maintaining clean, structured data, contact centers can uncover valuable patterns and trends that drive decision-making. Ultimately, having an organized data collection and management system lays the groundwork for successful predictive analytics implementation, allowing organizations to respond proactively to customer needs and preferences in real time.
- Model Building and Testing
Model building and testing is a pivotal phase in the implementation of predictive analytics within contact centers. During this phase, data scientists utilize historical data to create models that predict customer interactions and behaviors. These models are shaped by identifying variables that significantly influence outcomes, such as call volume, customer satisfaction, or issue resolution rates. By understanding these variables, organizations can fine-tune their service strategies effectively.
Once models are built, thorough testing is essential to ensure their accuracy and reliability. This involves running simulations and comparing predicted results against actual outcomes. If discrepancies arise, further adjustments to the model may be necessary until it meets predefined performance standards. Incorporating continuous feedback loops enhances the modelโs predictive power, allowing for incremental improvements based on real-world data. Proper model building and testing ultimately support more informed decision-making and improved operational efficiency in contact centers.
- Deployment and Monitoring
Effective deployment and monitoring are critical components of successful predictive analytics implementation in contact centers. First, ensure that the deployment process is seamless and aligns with the specific objectives of your analytics strategy. This involves determining whether you prefer a self-service model, allowing users to interact with data independently, or a more automated approach that generates periodic reports based on predefined parameters. Engaging stakeholders in this decision can streamline the implementation process.
Once deployed, continuous monitoring is essential to maintain the accuracy and relevance of your predictive models. Establish clear metrics to evaluate the success of your analytics solution and adapt it based on feedback and performance. Regular evaluations will help you identify areas for improvement and ensure that the insights you derive continue to meet your evolving business needs. By focusing on robust deployment and ongoing monitoring, you can enhance the effectiveness of predictive analytics in your contact center operations.
Extract insights from interviews, calls, surveys and reviews for insights in minutes
Top Tools for Predictive Analytics Implementation in Contact Centers
Implementing predictive analytics in contact centers requires selecting the right tools to leverage data effectively. Various tools empower organizations to analyze vast sets of customer data, transforming insights into actionable strategies. A number of leading platforms have emerged as essential for predictive analytics implementation, each offering unique features to cater to diverse needs.
insight7: This platform focuses on self-service data analysis, enabling teams to manage customer conversations effortlessly and gather valuable insights. Its ease of use allows for quick responses to customer signals.
IBM SPSS: Renowned for statistical analysis, IBM SPSS excels in predictive analytics, offering robust functions for data mining and predictive modeling. Organizations can rely on SPSS to derive actionable insights from complex datasets.
SAS Advanced Analytics: This powerful tool provides advanced analytics, machine learning, and artificial intelligence features. SAS facilitates deep exploration of data, empowering contact centers to make informed decisions.
RapidMiner: Known for its user-friendly interface, RapidMiner offers a comprehensive suite for data preparation, machine learning, and model deployment, significantly streamlining the predictive modeling process.
Microsoft Azure Machine Learning: This cloud-based platform supports end-to-end machine learning lifecycle management. It integrates well with existing systems, making it a versatile option for predictive analytics implementation.
Tableau: While primarily a data visualization tool, Tableau also offers analytical capabilities that help teams easily interpret data and uncover trends, enhancing decision-making in contact centers.
Choosing the right tools is critical for successful predictive analytics implementation, ensuring that contact centers can respond proactively to customer needs and drive business success.
insight7
Understanding the intricacies of predictive analytics implementation can significantly improve operational efficiency in contact centers. The journey starts by identifying key business objectives that the analytics can address. For instance, companies may aim to reduce customer wait times or enhance user satisfaction levels. Having clear goals paves the way for effective data collection and management, ensuring the right information is gathered to drive insights.
Once the data is in hand, model building and testing are crucial components. This phase involves creating algorithms that can predict customer behaviors or patterns based on the collected data. Following successful testing, deploying these models allows contact centers to proactively address customer needs. Continuous monitoring and adjusting of the predictive models are essential to maintain their relevance and effectiveness in a rapidly changing environment. By following these structured steps, organizations can leverage predictive analytics to foster a more responsive and efficient customer service experience.
IBM SPSS
In the realm of predictive analytics implementation, utilizing robust tools can significantly enhance outcomes. One such tool is a powerful statistical software suite that enables users to perform advanced analytics, including predictive modeling. This software specializes in transforming raw data into actionable insights, pivotal for contact centers aiming to improve customer interactions and operational efficiency.
To effectively employ this tool, the initial step involves data preparation, ensuring that the input data is clean and structured for analysis. Following that, users can build various statistical models to predict customer behavior and trends. These insights can guide strategic decision-making and enhance service delivery, ultimately leading to increased customer satisfaction. Understanding how to utilize this software is essential for any contact center seeking to harness the full potential of predictive analytics in their operations.
SAS Advanced Analytics
SAS Advanced Analytics offers a robust framework for implementing predictive analytics in contact centers. It combines advanced statistical analysis with machine learning to glean insights from historical data. By using these tools, organizations can identify trends and patterns that may impact customer interactions, leading to improved service and efficiency.
To effectively implement predictive analytics, start by ensuring your data is organized and relevant. This involves collecting diverse data points from customer interactions, like call transcripts and feedback. Next, SAS helps in building predictive models that can forecast future behaviors or outcomes. Continuous monitoring of these models is vital; this allows for adjustments based on real-time metrics and performance analysis. By leveraging SAS Advanced Analytics, contact centers can make informed decisions that enhance both customer satisfaction and operational effectiveness.
RapidMiner
RapidMiner stands out as a robust tool for predictive analytics implementation in contact centers. This versatile platform allows users to create, evaluate, and deploy predictive models without needing extensive programming knowledge. User-friendly interfaces guide analysts through the model-building process, enabling them to transform raw data into actionable insights effectively.
One of the standout features of RapidMiner is its ability to handle various data forms seamlessly. Analyzing customer interactions, feedback, and call logs becomes effortless, as users can visualize trends and patterns within datasets. This capability aids in identifying areas for service improvement and enhancing customer satisfaction. Furthermore, RapidMiner supports collaborative efforts, making it easier for teams to share insights and align on strategies for optimizing contact center operations, thus ensuring businesses derive the maximum value from their data analytics initiatives.
Microsoft Azure Machine Learning
Microsoft Azure Machine Learning offers a comprehensive platform designed to enhance predictive analytics implementation in contact centers. This tool empowers organizations to build, deploy, and manage machine learning models efficiently. With its user-friendly interface and powerful capabilities, data scientists and business analysts can easily collaborate, accelerating the decision-making process.
In the context of contact centers, predictive analytics can significantly improve customer service quality. For instance, organizations can analyze call data, identify customer sentiment, and anticipate potential issues before they escalate. By integrating Microsoft Azure Machine Learning into their workflows, businesses can harness actionable insights, leading to optimized operations and improved customer experiences. This aligns with the overall goal of predictive analytics implementation, allowing contact centers to be proactive rather than reactive.
Tableau
Tableau is a powerful visualization tool that plays a crucial role in the predictive analytics implementation process within contact centers. By transforming complex data sets into accessible and visually engaging dashboards, it empowers teams to uncover actionable insights quickly. This tool supports decision-makers in identifying trends, patterns, and areas of improvement far more efficiently than traditional reporting methods.
Utilizing Tableau in predictive analytics allows teams to merge diverse data sources, enabling a holistic view of operations. Users can easily create custom visualizations that highlight key performance indicators, making it simpler to recognize customer behavior and needs. As insights are drawn from data visualizations, organizations can optimize their strategies and enhance customer experience. In summary, Tableau is invaluable for turning predictive analytics concepts into tangible outcomes that drive success in contact center operations.
Conclusion on Mastering Predictive Analytics Implementation in Contact Centers
Mastering predictive analytics implementation in contact centers is not just a technical endeavor; it involves a cultural shift towards data-driven decision-making. Organizations must prioritize aligning their business objectives with specific analytic strategies to ensure impactful outcomes. By doing so, they can enhance customer experience, reduce operational costs, and improve overall efficiency.
Furthermore, ongoing monitoring and iteration are essential to refine predictive models based on real-time data and insights. Engaging teams across different departments fosters a collaborative mindset that empowers them to utilize these analytics effectively. Ultimately, a commitment to continual improvement will facilitate successful predictive analytics implementation, driving a competitive edge in the market.