How to Implement AI-Based Predictive Call Handling Optimization
-
Bella Williams
- 10 min read
Predictive Call Optimization is revolutionizing how organizations handle customer interactions. Imagine a call center where AI anticipates customer needs before they even express them. With real-time data analysis, agents are empowered to provide tailored solutions that enhance the customer experience and streamline operations.
Understanding the intricacies of predictive call optimization is crucial for those looking to implement AI-based call handling systems effectively. By leveraging historical data and AI algorithms, organizations can predict call volumes and optimize agent performance. This proactive approach not only improves efficiency but also fosters stronger connections with customers by ensuring their needs are met promptly and accurately.
Analyze & Evaluate Calls. At Scale.
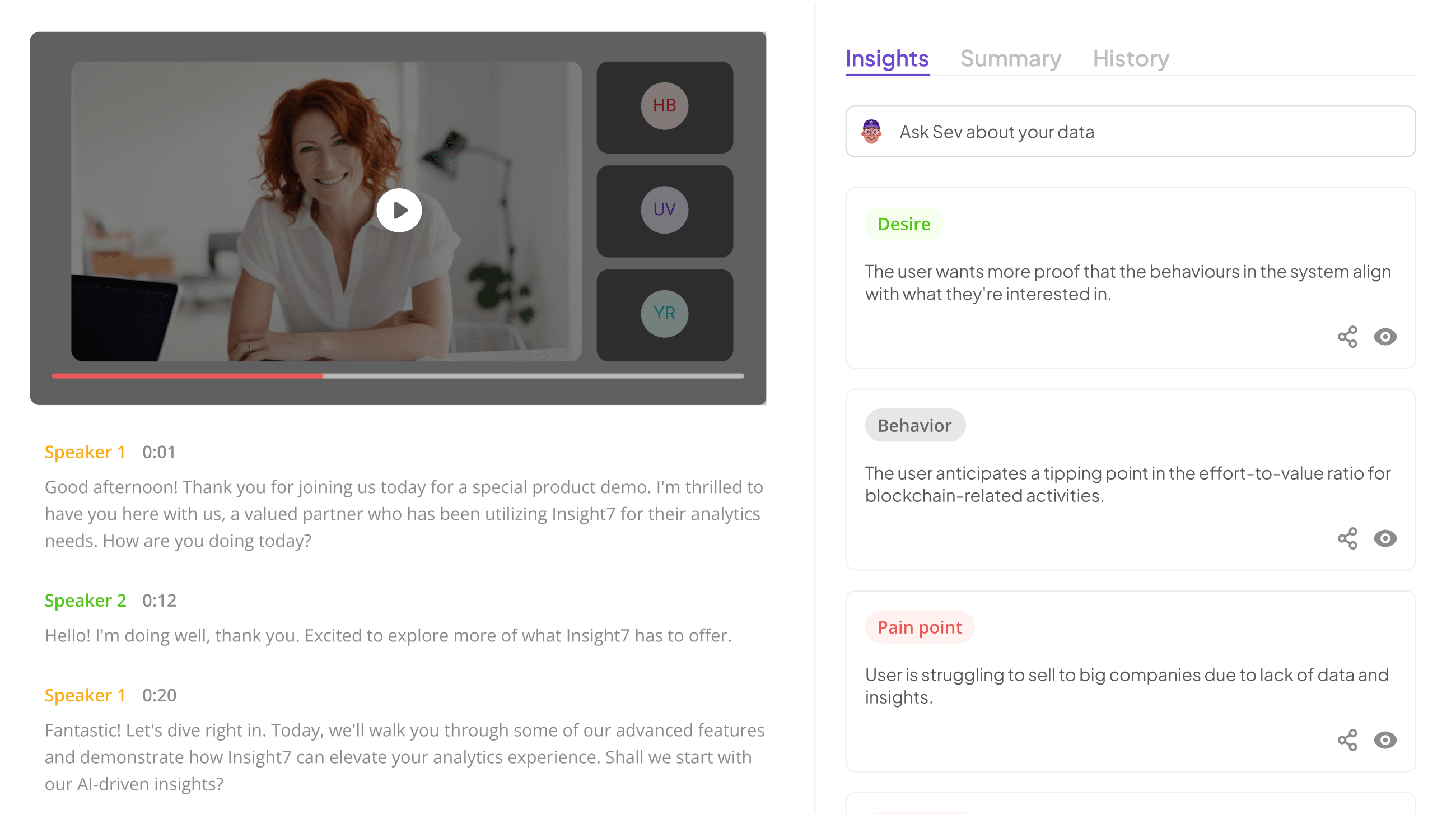
How Predictive Call Optimization Transforms Customer Experience
Predictive Call Optimization plays a pivotal role in reshaping the customer experience across various industries. By utilizing advanced algorithms to anticipate customer needs, businesses can significantly enhance their service efficiency. This proactive approach ensures that customers are connected to the right agents who possess the appropriate skills and knowledge to address their concerns effectively.
Moreover, the transformation of customer experience through Predictive Call Optimization is not merely about technology; it's about understanding customer behavior through data analysis. Businesses that invest in historical data analysis can uncover insights that enable them to forecast call volumes and anticipate common inquiries. This added layer of preparedness allows agents to manage their time better and focus on providing exceptional customer interactions, ultimately leading to increased satisfaction and loyalty.
Enhancing Efficiency with Predictive Call Optimization
Enhancing efficiency with predictive call optimization is crucial for improving customer service operations. By utilizing AI algorithms for predictive analysis, organizations can anticipate customer inquiries and optimize staff allocation, ensuring that the right agents handle the most relevant cases. This process not only reduces wait times but also enhances the overall customer experience by connecting callers with knowledgeable representatives promptly.
Incorporating optimal call routing further boosts agent performance. When calls are directed based on predictive models, agents spend less time addressing unrelated queries. Instead, they can focus on complex issues that require their expertise. This targeted approach promotes higher satisfaction rates among customers and allows for more effective use of resources. Overall, predictive call optimization fosters a more responsive, efficient, and ultimately successful call center environment.
- Leveraging AI Algorithms for Predictive Analysis
AI algorithms serve as a cornerstone for implementing effective predictive analysis in call handling. By leveraging these algorithms, organizations can analyze historical call data to uncover trends that inform future operations. This process begins with gathering and segmenting past call records, which allows for the identification of key patterns that signal peak call times and common customer inquiries. These insights lead to more informed decision-making, enhancing overall predictive call optimization.
As AI continues to evolve, the potential for refining call handling processes increases. Utilizing these algorithms not only streamlines call routing but also equips agents with preemptive guidance based on customer behavior predictions. The result is a more efficient system that meets customer needs promptly. By closely monitoring interactions and outcomes, organizations can continuously refine their approaches. Ultimately, this integration of AI enhances customer experience, paving the way for innovative strategies in predictive call optimization.
- Boosting Agent Performance through Optimal Call Routing
Optimal call routing is vital for enhancing agent performance and overall operational efficiency. When calls are directed based on real-time data and customer profiles, agents can engage more effectively, addressing customer needs faster. This approach significantly reduces the average handling time and increases customer satisfaction levels. By utilizing AI-driven insights, you can streamline the routing process, allowing representatives to focus on more complex inquiries that require their expertise.
Moreover, predictive call optimization plays a crucial role in identifying patterns in call volume and types, enabling better workforce management. This anticipatory approach helps in allocating resources efficiently, ensuring that the right agents are available during peak times. Implementing such robust systems ultimately empowers agents, enhancing their skills and performance while also improving the overall customer experience. Thus, optimal call routing is not merely a technical solution but a strategic move for sustainable success.
Predictive Call Optimization Best Practices
To ensure effective Predictive Call Optimization, businesses should adhere to a set of best practices that enhance performance and improve customer interactions. First, employing historical data is vital for better forecasting. Analyzing past call patterns allows organizations to anticipate peak times and customer needs, enabling proactive resource allocation.
Second, continuous monitoring and feedback loops play a crucial role. Regularly assessing performance metrics and agent feedback helps identify areas for improvement and training opportunities. This iterative process fosters a culture of growth and adaptation, ensuring that predictive models remain relevant and effective. By implementing these practices, companies can create a robust framework that not only enhances efficiency but also maximizes the overall call experience for both agents and customers. Thus, mastering Predictive Call Optimization ultimately leads to heightened satisfaction and increased loyalty.
- Utilizing Historical Data for Better Forecasting
Understanding the patterns in historical data is crucial for predictive call optimization. By analyzing past call information, businesses can identify trends and behaviors that inform future interactions. For instance, examining the frequency of specific queries during peak times enables organizations to allocate resources more effectively. This proactive approach enhances overall customer satisfaction, as agents are better prepared to handle anticipated requests.
Furthermore, employing AI algorithms to process historical data uncovers insights that may not be immediately apparent. These insights can guide strategic decisions, such as optimizing call routing to match agents' strengths with customer needs. The result is a more efficient call handling process that minimizes wait times while improving the quality of service provided. Ultimately, leveraging historical data not only refines operational strategies but also creates a more responsive customer experience, solidifying the foundation for effective predictive call optimization.
- Implementing Continuous Monitoring and Feedback Loops
Continuous monitoring and feedback loops are essential for achieving effective predictive call optimization. By systematically tracking call performance and outcomes, organizations can gain insights into customer-agent interactions. This consistent evaluation helps identify trends and areas for improvement in real-time, enabling businesses to respond proactively to customer needs.
Establishing a feedback culture among agents is crucial. Regularly sharing insights from call data fosters an environment of growth and adjustment. Incorporating tools that automatically analyze calls allows teams to refine their approaches based on actual interactions. Additionally, integrating customer feedback into training programs enhances overall service quality. Ultimately, a focus on continuous monitoring creates a cycle where insights lead to actionable improvements, driving superior customer experiences and operational efficiency.
Extract insights from interviews, calls, surveys and reviews for insights in minutes
Steps to Implement AI-Based Predictive Call Handling Optimization
To implement AI-based predictive call handling optimization, start by thoroughly analyzing your call center data. This involves gathering relevant data and key performance metrics, such as call volumes and duration. Next, look for patterns and predictive indicators that can highlight recurring customer issues. By understanding the data, you can tailor your predictive models more effectively.
Once you have a solid data foundation, the next step is to set up the appropriate AI models. Select the right AI tools and frameworks suited to your needs and train the models based on the analyzed data to ensure accurate predictions. Subsequently, integrating these models with your existing systems is crucial for streamlining processes. Ensure system compatibility and facilitate seamless data flow across platforms. Finally, evaluate and regularly adjust your system. Conduct performance assessments and iterate based on feedback and outcomes to refine the optimization process. This strategic approach will significantly enhance your predictive call optimization efforts.
Step 1: Analyzing Call Center Data
To effectively implement predictive call optimization, the first step is analyzing call center data. This involves gathering relevant metrics that can shed light on customer interactions and service agent performance. Start by compiling data from various sources, such as call transcripts, customer feedback, and performance reports. This comprehensive data collection serves as the foundation for understanding how factors like call duration and question frequency influence customer satisfaction and employee efficiency.
Next, identify patterns and predictive indicators within the analyzed data. Look for trends in the types of inquiries received and potential bottlenecks in response times. For example, you might discover that specific regions have unique questions that require tailored agent training or resources. Evaluating this data not only enhances workflows but also maximizes the effectiveness of your customer service representatives, setting the stage for more informed strategies in implementing AI-based systems for predictive call optimization.
- Gathering Relevant Data and Metrics
Gathering relevant data and metrics is essential for successful predictive call optimization. Start by identifying the key data points that contribute to understanding customer interactions. Metrics such as call volume, duration, customer demographics, and agent performance provide invaluable insights that shape the optimization process.
Next, it’s important to utilize historical data effectively. Analyzing past call records reveals patterns and trends that inform predictive models. This allows for the identification of predictive indicators—elements that forecast customer behavior and guide call routing strategies. By collecting and analyzing these metrics continuously, businesses can remain agile and adjust their approach based on real-time insights. This data-driven framework not only enhances the customer experience but also improves overall operational efficiency.
- Identifying Patterns and Predictive Indicators
Identifying patterns and predictive indicators is essential in achieving effective predictive call optimization. Begin by meticulously analyzing historical call data to uncover trends. This data can reveal frequent pain points, customer preferences, and common inquiries. By aggregating this information, you can highlight significant themes, tailoring your approach to address customer needs proactively. Utilizing AI models to sift through these patterns allows for swift identification of key issues, streamlining call handling processes.
Another critical aspect is the establishment of predictive indicators. These indicators are measurable variables, such as call volume or average resolution time, that signal forthcoming trends. By monitoring these metrics over time, you can forecast demand and optimize resource allocation. Ultimately, this proactive approach not only enhances operational efficiency but also elevates the overall customer experience. Fostering such insights enhances your organization's ability to adapt, ensuring that customer interactions are smooth and effective.
Step 2: Setting Up AI Models
To establish effective AI models for Predictive Call Optimization, it’s essential to choose the right tools and frameworks. Begin by selecting AI platforms that cater specifically to call handling needs. Research various offerings to find solutions that support customizable analytics and performance metrics tailored to your organization’s goals. A robust AI tool will not only streamline the data processing but also enhance the algorithm’s efficiency.
Once you have selected your AI tools, the next step involves training the models. Historical call data serves as the foundation for building accurate predictive capabilities. This data should encompass variables such as call duration, customer interactions, and agent performance. The training process requires continuous refinement to ensure that the models accurately predict call outcomes, resulting in optimized handling and improved customer satisfaction. Investing time in this stage is crucial, as it lays the groundwork for successful predictive analysis in your operation.
- Selecting the Right AI Tools and Frameworks
Choosing the right AI tools and frameworks is essential for successful predictive call optimization. Begin by identifying the specific needs of your organization to determine the most suitable options. Consider factors such as user-friendliness, integration capabilities, and support options. Selecting tools that provide robust analytics and allow customization will streamline your implementation process.
Next, evaluate various frameworks suited for your operational structure. Ensure that they can adapt to your evolving goals focused on predictive call optimization. Comprehensive training for your team on these tools is crucial, as mastery enhances their effectiveness. Lastly, maintain an open line of communication with tool providers to resolve any potential issues and gain insights on best practices, ultimately improving your call handling strategies. Make informed decisions and continuously refine your approach to stay ahead in a competitive landscape.
- Training Models for Accurate Predictions
Training models effectively is pivotal for achieving accurate predictions in the domain of predictive call optimization. This begins with data preparation, where historical call data is gathered and cleaned. Data quality directly influences model performance, so it’s essential to remove any inconsistencies or irrelevant information. Once cleaned, the data can be split into training and testing sets to ensure that the model generalizes well to new, unseen data.
Next, various machine learning algorithms—such as decision trees or neural networks—can be applied to train the model. Each algorithm has strengths suited for different types of data. It's crucial to evaluate model performance using metrics like accuracy and recall to find the best fit for the specific call-handling context. Continuous monitoring and adjustment of the model ensure it remains effective over time, adapting to new patterns in customer behavior and call trends. Through these methods, organizations can refine their predictive capabilities and enhance overall customer service.
💬 Questions about How to Implement AI-Based Predictive Call Handling Optimization?
Our team typically responds within minutes
Step 3: Integrating with Existing Systems
Integration with existing systems is a crucial step in implementing AI-based predictive call optimization. The goal is to ensure compatibility with your current infrastructure, which may involve various databases, communication tools, and customer relationship management (CRM) systems. Begin by assessing your existing technologies to determine how the new AI solution can fit within the established framework. This helps avoid interruptions in service and maintains data continuity across platforms.
Next, facilitating seamless data flow across systems is essential for maximizing the efficacy of predictive call optimization. This can be achieved through APIs and connectors that allow data sharing and synchronization. Enhanced integration allows real-time access to valuable insights, aiding in decision-making. By ensuring these systems work together harmoniously, businesses can better meet customer needs and drive operational efficiency. Ultimately, a well-integrated system enhances the effectiveness of AI solutions and elevates the overall customer experience.
- Ensuring Compatibility with Current Infrastructure
When integrating AI-based predictive call handling optimization, ensuring compatibility with current infrastructure is essential. The existing systems must be able to support new technologies without causing disruption. To achieve this, organizations should assess their current technology setup, identifying gaps that may hinder seamless integration. This includes evaluating hardware capabilities, software applications, and communication tools in use.
Next, consider compatibility protocols for data transfer. Predictive call optimization relies heavily on data analytics, so having a robust data flow mechanism is crucial. Workflows should be designed to ensure that both historical and real-time data can be accessed easily. Additionally, collaboration among IT departments and stakeholders will facilitate the fine-tuning of processes. Prioritizing the alignment of AI solutions with existing systems can significantly enhance operational efficiency and customer satisfaction.
- Facilitating Seamless Data Flow Across Platforms
To facilitate seamless data flow across platforms, it is crucial to ensure that different systems can share information efficiently. By optimizing predictive call handling, organizations can create an integrated experience that allows for smooth communication between various tools and databases. This integration helps eliminate data silos, enabling call centers to leverage valuable insights derived from multiple data sources.
Moreover, implementing solutions for seamless data sharing can significantly enhance operational efficiency. For example, using Application Programming Interfaces (APIs) allows disparate systems to exchange data in real time. This connectivity is essential for predictive call optimization, as it empowers agents with immediate access to relevant customer information, thereby improving response times and overall service quality. A holistic approach ensures that insights generated from AI analytics inform every aspect of the call-handling process, leading to better customer interactions and streamlined operations.
Step 4: Evaluating and Adjusting the System
Evaluating and adjusting the system is crucial to ensure effective predictive call optimization. Start by conducting regular performance assessments to identify areas of improvement. This may involve analyzing the model's accuracy, reviewing call data, and comparing outcomes against set benchmarks. Use metrics that matter most to your operations, such as average handling time and customer satisfaction scores, to gauge success.
Next, iterate based on feedback and outcomes. Gather insights from team members about real-world system functionality. Pay attention to the nuances of call patterns and customer interactions, as they can reveal unexpected demands. Make necessary adjustments to optimize call routing and enhance agent performance. Ultimately, the key lies in maintaining a dynamic approach, ensuring that your predictive systems evolve alongside changing business needs and customer expectations. This continuous evaluation and adjustment process will help you refine strategies for successful customer engagement in a competitive landscape.
- Conducting Regular Performance Assessments
Conducting regular performance assessments is essential for maximizing the benefits of predictive call optimization. By systematically reviewing agent performance, organizations can identify strengths and weaknesses, allowing for targeted training and development. Assessments should focus on various aspects of call handling, including greetings, engagement, and issue resolution, ensuring that each representative aligns with best practices.
To conduct effective assessments, consider following these key steps:
- Set Clear Evaluation Criteria: Develop specific metrics based on customer interaction goals.
- Utilize Call Transcripts: Analyze transcripts to measure adherence to the evaluation criteria.
- Provide Constructive Feedback: Offer actionable insights to agents after each assessment, fostering continuous improvement.
- Review Results Regularly: Schedule monthly or quarterly evaluations to track progress over time.
- Adjust Strategies as Needed: Be flexible and modify criteria based on emerging trends in customer interactions and evolving organizational goals.
Implementing these steps ensures an adaptive and responsive approach to performance, ultimately enhancing the effectiveness of predictive call optimization processes.
- Iterating Based on Feedback and Outcomes
To successfully implement AI-based predictive call optimization, iterating based on feedback and outcomes is essential. Continuously evaluating the effectiveness of the system paves the way for improved call handling processes. Engaging with customer feedback allows organizations to refine their strategies, ensuring that the AI algorithms used remain relevant to the current environment of customer interactions. This proactive approach helps identify both strengths and weaknesses, enabling a cycle of constant improvement.
The outcomes of implemented changes should also be measured against set benchmarks. Gathering metrics related to call performance, customer satisfaction, and agent efficiency will provide a clear picture of progress. By analyzing these metrics regularly, necessary adjustments can be made to enhance predictive call optimization continually. In this way, organizations can foster a culture of responsiveness, remaining adaptable to evolving customer needs and expectations.
Top Tools for Predictive Call Optimization Implementation
Implementing predictive call optimization requires the right set of tools to facilitate seamless integration and accurate analysis. First on the list, the insight7 platform streamlines data collection and analysis, allowing teams to evaluate call center performance effectively. It enables organizations to pinpoint areas for improvement through actionable insights derived from customer interactions.
Next, Google AI Platform provides robust machine learning capabilities, offering predictive analytics to forecast call volumes and customer inquiries. Following closely, IBM Watson utilizes natural language processing to analyze conversations, extracting valuable information that informs training and operational adjustments. The Amazon Connect service offers a flexible, cloud-based environment that integrates with AI tools, streamlining the call handling process. Also noteworthy is Five9, which enhances agent productivity through intelligent routing, while Genesys provides a unified communication platform that facilitates real-time data usage for optimal caller experiences. Using these tools effectively can significantly enhance predictive call optimization strategies.
insight7
To achieve effective predictive call optimization, organizations must focus on understanding customer insights and behavioral patterns. By analyzing historical call data, companies can identify key indicators that predict customer needs and preferences. This foundational step enables the development of AI models that enhance interactions and streamline call handling processes.
The integration of AI tools can significantly improve efficiency. Companies should prioritize seamless integration with existing systems to ensure a smooth flow of information, which allows for real-time adjustments and refined predictions. Regular performance evaluations are essential to adapt the system based on received feedback and outcomes. Embracing these strategies can lead to optimized call routing, resulting in heightened customer satisfaction and loyalty. Ultimately, organizations that invest in predictive call optimization will be well-positioned to thrive in a competitive market.
Google AI Platform
The Google AI Platform serves as an invaluable tool for organizations aiming to enhance their Predictive Call Optimization strategies. By providing a user-friendly interface, even those without technical expertise can harness its capabilities. Users can easily access functionalities like transcribing calls and generating reports that highlight customer experiences, making optimization efforts streamlined and efficient.
This platform also offers robust analytics, allowing users to examine patterns from multiple calls simultaneously. Insights can be extracted to identify pain points and desires, giving businesses a clearer understanding of customer needs. Beyond simple analysis, the platform's ability to generate evidence-backed recommendations enables teams to make informed decisions promptly. By utilizing these features effectively, companies can greatly improve their call handling processes, ultimately leading to a more satisfying customer journey.
IBM Watson
Artificial Intelligence plays a crucial role in predictive call optimization, offering solutions that streamline call handling. Utilizing advanced algorithms and sophisticated data analysis, users can harness these capabilities to improve operational efficiency significantly. By analyzing historical data, organizations can forecast call volumes and patterns, leading to more informed decision-making.
One of the standout features is its ability to enhance agent performance through optimal call routing. By understanding each agent’s strengths and weaknesses, the system can allocate calls strategically. Furthermore, the use of continuous monitoring enables organizations to adapt swiftly and refine their strategies based on real-time insights. Such advancements not only improve customer experiences but also drive higher satisfaction rates among agents, leading to a more effective workforce overall.
Amazon Connect
Amazon Connect serves as a powerful tool for businesses seeking to enhance their customer interactions through predictive call optimization. This cloud-based contact center solution allows organizations to streamline their call workflows, leveraging advanced AI capabilities to offer tailored customer experiences. By automating routine tasks and directing calls to the right agents, it decreases wait times and increases customer satisfaction.
Implementing predictive call optimization using Amazon Connect enables businesses to analyze historical call data, thus identifying trends and patterns that inform future interactions. This data-driven approach helps in making informed decisions that ultimately enhance agent performance and efficiency. With its flexible integration capabilities, Amazon Connect ensures that organizations can align it with their existing systems, making the transition to a more optimized call handling process seamless. Adopting such technology allows businesses to not only respond to customer needs effectively but also build lasting relationships through efficient service delivery.
Five9
In the realm of AI-driven customer service, harnessing advanced technologies for efficient call management is pivotal. A key player in this innovation journey streamlines communication processes through predictive call optimization. This approach employs sophisticated algorithms to analyze historical data, enhancing agents' abilities to respond effectively to customer inquiries. By pinpointing call patterns, it allows organizations to anticipate needs, improving overall service delivery.
Furthermore, the integration of this optimization method significantly boosts productivity and operational efficiency. Agents can focus on high-priority calls, ensuring that customers receive timely and relevant support. Continuous evaluation and feedback loops refine these systems, making them adaptable to changing customer behaviors. The result is a harmonious balance between technology and human interaction, leading to better customer experiences and agent performance. Embracing predictive call optimization is not just a trend; it is crucial for enhancing the quality of service in any customer-centric environment.
Genesys
In the realm of AI-based predictive call handling optimization, an advanced platform stands out for its capability to enhance call management. Its features streamline processes, bringing efficiency to the forefront. The system utilizes intricate algorithms that analyze vast amounts of data, transforming how interactions are managed. This predictive call optimization enables organizations to anticipate customer needs better, leading to improved conversational dynamics.
Furthermore, this technology ensures agents are aligned with the right calls at the right time. By optimizing call routing based on historical data, customer profiles, and current trends, businesses can deliver a more tailored customer experience. Agents become better equipped to engage meaningfully, asking targeted questions that elicit valuable insights. This results in not only satisfied customers but also a motivated team that feels empowered in their roles, allowing the organization to thrive in a competitive environment.
Conclusion: The Future of Predictive Call Optimization in AI-Driven Call Centers
The future of predictive call optimization in AI-driven call centers looks promising, as organizations increasingly realize its potential to enhance customer interactions. By harnessing advanced AI algorithms, these systems enable more accurate predictions, which ultimately leads to better call handling and improved customer satisfaction. As such technologies become more refined, call centers can expect to see a significant transformation in operational efficiency.
Moreover, ongoing advancements in machine learning and data analytics will continue to shape predictive call optimization strategies. This evolution will not only streamline processes, but also allow for a more personalized customer experience. As businesses embrace these innovations, the ability to analyze and respond to customer needs in real-time will become a critical differentiator in an increasingly competitive landscape.
💬 Questions about How to Implement AI-Based Predictive Call Handling Optimization?
Our team typically responds within minutes