How to Implement AI-Based Call Center Workforce Demand Forecasting
-
Bella Williams
- 10 min read
AI Workforce Forecasting is revolutionizing how call centers anticipate and manage demand. Traditionally, staffing decisions relied on historical data and intuition, often leading to inefficiencies. By integrating AI into workforce forecasting, call centers can analyze vast datasets, identifying patterns and trends that human oversight may overlook. This advancement enables businesses to predict call volume fluctuations accurately and deploy resources more effectively.
Understanding the intricacies of AI Workforce Forecasting is essential for call center managers aiming to optimize their operations. This innovative approach not only enhances service quality but also reduces costs associated with overstaffing or understaffing. As the industry evolves, adopting AI tools will become increasingly critical for maintaining a competitive edge and ensuring customer satisfaction.
Analyze & Evaluate Calls. At Scale.
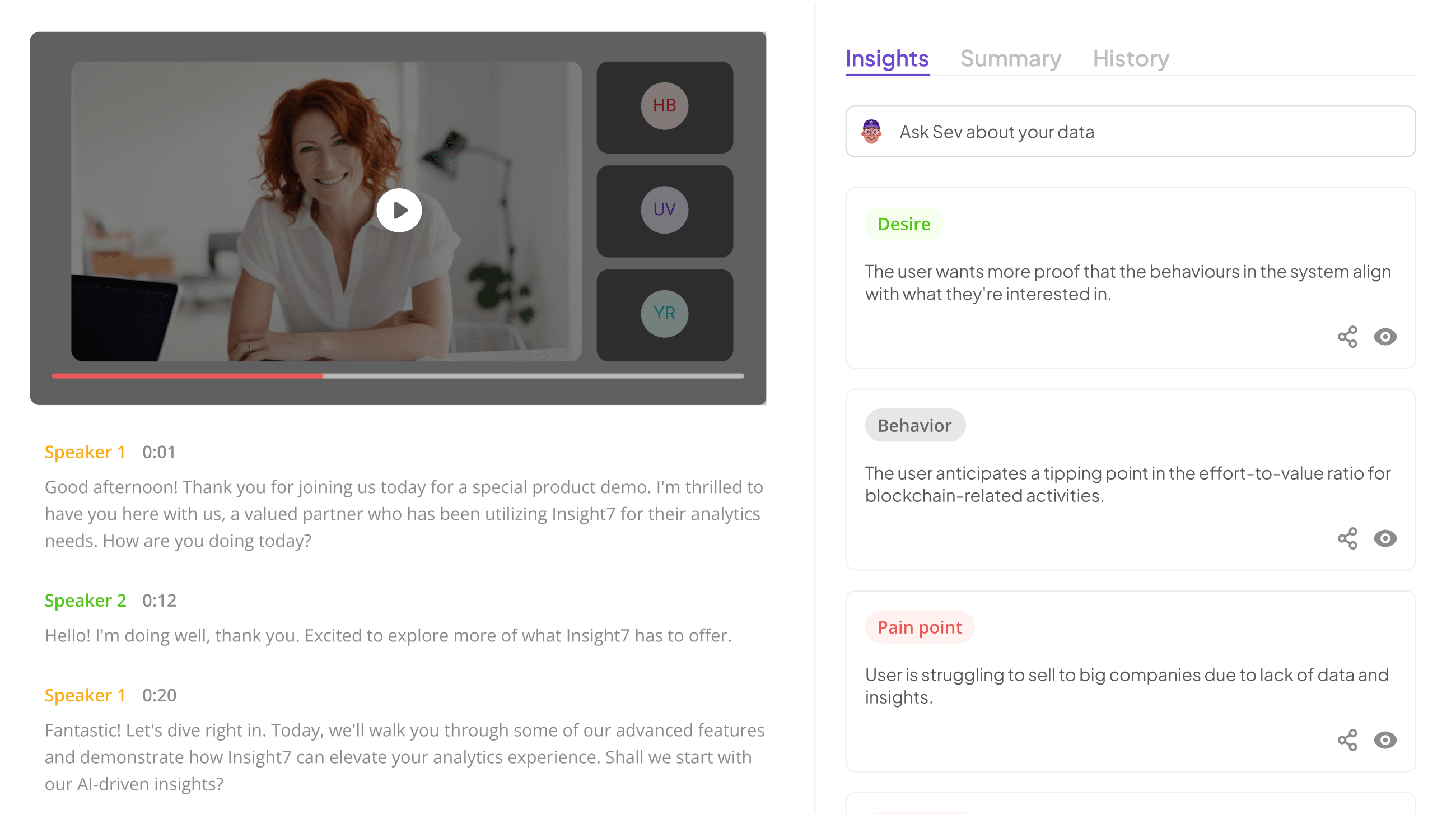
Understanding AI Workforce Forecasting Benefits
AI Workforce Forecasting offers significant advantages that can transform call center operations. By predicting workforce demand more accurately, businesses can ensure they have the right number of agents available at peak times. This not only enhances customer satisfaction by reducing wait times but also minimizes agent burnout and stress, leading to a more engaged workforce.
Moreover, leveraging AI Workforce Forecasting allows organizations to make data-driven staffing decisions, tailored to historical call patterns, seasonal trends, and real-time events. This precision in workforce management reduces operational costs, as fewer agents may be needed during quieter periods. Ultimately, the use of AI in forecasting enables call centers to operate more efficiently, leading to increased profitability and improved service delivery.
Enhancing Operational Efficiency
Effective operational efficiency is paramount in any call center environment. By harnessing AI workforce forecasting, organizations can optimize resource allocation and align staffing levels with anticipated demand. This predictive approach allows for a dynamic response to call volume fluctuations, ensuring that customer service representatives are adequately supported, while also avoiding overstaffing.
When implementing this strategy, there are key factors to consider. First, accurate data collection is crucial. Gathering historical call data, customer trends, and seasonal fluctuations establishes a robust foundation for forecasts. Second, selecting appropriate AI tools tailored to the unique needs of the call center can greatly enhance forecasting accuracy. Regular model testing and monitoring further allow continuous refinement, ensuring that the forecast adapts to changing patterns in customer interactions. It is this blend of precision and adaptability that drives operational excellence through AI workforce forecasting.
Reducing Operational Costs
Implementing AI Workforce Forecasting can significantly reduce operational costs within a call center. By accurately predicting demand, organizations can optimize staffing levels and resources. This approach minimizes expenses associated with overstaffing and understaffing, ensuring that agents are available when needed without unnecessary overhead.
For achieving financial efficiency, consider focusing on key strategies:
Data-Driven Scheduling: Use historical data to create optimal schedules that align work shifts with anticipated call volume.
Dynamic Resource Allocation: Adjust agent availability based on real-time forecasts to adapt quickly to changes in demand.
Performance Monitoring: Continuously analyze agent performance metrics to identify areas for improvement and efficient training.
Cost-Benefit Analysis: Regularly evaluate the financial impacts of workforce choices to maximize the value of every dollar spent.
By integrating these strategies, organizations not only reduce costs but also improve service quality and operational efficiency. Embracing AI Workforce Forecasting can lead to sustainable savings in the long run.
Extract insights from interviews, calls, surveys and reviews for insights in minutes
Implementing AI Workforce Forecasting in Call Centers
Implementing AI Workforce Forecasting in call centers begins with data collection and preparation. This initial step is crucial, as the accuracy of forecasting depends heavily on the quality and comprehensiveness of the data gathered. Collect historical call data, including factors such as call volume, service times, and peak periods. This information will serve as the foundation for subsequent analysis.
Next, selecting the right AI tools for forecasting is essential. Popular options include platforms like XANT, Verint Monet, and NICE inContact, among others. Each tool has unique features, so itโs vital to choose one that aligns with your specific needs. After selecting a tool, you will enter the model training and testing phase. This involves feeding the system with the prepared data and adjusting parameters to improve accuracy. Once the model is trained, deploy the AI solution and continuously monitor its performance. Adjust the process as needed to refine forecasting accuracy over time.
Step 1: Data Collection and Preparation
Data collection and preparation is a foundational step in AI workforce forecasting, crucial for predictive accuracy. Begin by gathering various data types, including historical call volume, staffing levels, and customer interaction patterns. This information lays the groundwork for understanding trends and peak operational times. Ensure data quality by validating and cleaning the datasets. Poor data can lead to inaccurate forecasts, impacting overall performance.
Next, structure the data appropriately for analysis. Organize it into relevant categories, such as time intervals and service types. This organization allows the AI models to recognize patterns more efficiently. Donโt forget to consider external factors like holidays or marketing campaigns that could influence call volumes. Once your data is ready, you can confidently move to the next phase of implementing AI workforce forecasting. By investing time in data preparation, you set the stage for more accurate predictions and enhanced decision-making capabilities.
Step 2: Choosing the Right AI Tools for Forecasting
Selecting the appropriate AI tools is crucial for effective workforce forecasting in call centers. First, consider functionality. Look for solutions that provide data analysis, call transcription, and reporting capabilities, as these features will enhance the insights derived from customer interactions. Effective AI tools should also support real-time decision-making, enabling managers to respond swiftly to changing call demand.
๐ฌ Questions about How to Implement AI-Based Call Center Workforce Demand Forecasting?
Our team typically responds within minutes
Next, assess the integration capabilities of potential AI tools. Seamless integration with existing systems is essential for maximizing data utility and minimizing operational disruptions. Additionally, prioritize tools that offer user-friendly interfaces to ensure your team can effectively utilize them. Lastly, ensure that the chosen AI workforce forecasting solutions comply with data security regulations to protect customer information. By taking these factors into account, you can choose the right AI tools that align with your organizationโs forecasting goals.
- insight7
The journey of AI Workforce Forecasting begins with understanding its fundamental role in optimizing call center operations. By harnessing data analytics and machine learning algorithms, organizations can anticipate shifts in call volume and workforce requirements. This predictive capability empowers managers to schedule staff more effectively and maintain service quality during peak times.
To fully realize the benefits of AI-based workforce forecasting, organizations must focus on several key areas. First, invest in robust data collection processes to gather relevant historical call data. Next, select cutting-edge AI tools tailored to your operational needs, such as XANT, Verint Monet, or NICE inContact. After implementation, itโs crucial to continually train and refine AI models based on live data, ensuring accuracy and reliability. This iterative approach allows businesses to stay ahead of demand fluctuations while also enhancing customer satisfaction.
In summary, a systematic adoption of AI Workforce Forecasting not only streamlines operations but also enhances service delivery, laying the foundation for long-term success.
- XANT
Integrating AI into workforce forecasting systems significantly enhances the efficiency of call centers. XANT plays a pivotal role in this process by providing advanced solutions that streamline demand forecasting, ensuring that workforce management aligns with customer needs. This system uses historical data and predictive analytics, enabling call centers to anticipate call volumes and adjust staffing accordingly. As a result, teams can maintain optimal service levels and improve customer satisfaction.
Moreover, XANT encourages real-time insights that empower managers to make informed decisions. By analyzing trends, identifying peak times, and adjusting resource allocation proactively, call centers can achieve a competitive edge. Key features like automated reporting and performance tracking enable better understanding of agent productivity. Consequently, integrating AI-powered tools like XANT within call centers transforms traditional forecasting methods and drives operational excellence in AI workforce forecasting.
- Verint Monet
When considering AI workforce forecasting in call centers, itโs essential to focus on the significance of advanced analytics tools. One prominent solution is an innovative platform that streamlines workforce management through predictive analytics. This platform can analyze historical data, including call volumes and agent performance, to provide actionable insights. By leveraging AI technologies, it enhances operational efficiency and helps meet fluctuating demand seamlessly.
The benefits of employing such a tool extend beyond predictive analytics. It also aids in workforce planning and scheduling by accurately estimating the number of agents needed during peak periods. This ensures optimal resource allocation and improved service levels for customers. Implementing this robust forecasting solution allows organizations to respond proactively to changing patterns, ultimately enhancing customer satisfaction and operational resilience.
- NICE inContact
AI Workforce Forecasting plays a crucial role in optimizing call center operations by accurately predicting staffing requirements. The focus here is on leveraging advanced tools that streamline workforce management activities. Various platforms, such as NICE inContact, offer robust features that enable call centers to effectively analyze and project demand. This ensures that the right number of agents are scheduled at peak times, enhancing service quality.
Incorporating AI into workforce management allows for data-driven insights that dynamically adjust to fluctuating call volumes. The use of such technologies facilitates real-time monitoring and improves the accuracy of forecasts. Furthermore, it provides valuable analytics on historical performance, leading to informed decision-making and a more agile workforce. Ultimately, integrating AI-powered solutions enhances operational efficiency and contributes significantly to the overall success of call center operations.
- Genesys
The implementation of AI workforce forecasting in call centers can greatly enhance service levels. This technology streamlines the allocation of resources by predicting customer demand fluctuations. By effectively anticipating call volumes, it helps maintain optimal staffing levels, which leads to reduced wait times and improved customer satisfaction.
When considering a suitable framework for AI workforce forecasting, several tools are available. These tools capture and analyze data, allowing organizations to identify patterns in customer interactions. Importantly, this data-driven approach enables proactive adjustments to workforce management strategies, ensuring that agents are available when demand peaks. Ultimately, adopting AI workforce forecasting improves overall operational efficiency, allowing call centers to respond dynamically to changing customer needs.
Step 3: Model Training and Testing
Training and testing your AI model is a critical phase in AI workforce forecasting. In this step, you refine your algorithm by feeding it historical data, enabling it to learn from patterns in call volume, customer behavior, and workforce availability. This process involves selecting the right training dataset and splitting it into training and validation sets, which helps in effectively evaluating the model's performance.
Once the model is trained, rigorous testing is crucial. This involves running simulations using unseen data to assess the model's accuracy in predicting future workforce needs. The results of this testing provide valuable insights into adjustments required for optimal performance. By successfully training and testing the model, organizations can enhance their ability to anticipate call center activity, improve staffing efficiency, and ultimately provide better customer service. This systematic approach lays the foundation for deploying a reliable AI workforce forecasting system.
Step 4: Deployment and Monitoring
After successful model training and testing, the next phase is deployment and monitoring. This process involves integrating the AI workforce forecasting model into your existing call center systems. During deployment, you'll want to define how the predictions are delivered, whether through automated reports or a user-friendly dashboard. This flexibility allows managers to access real-time data or explore metrics at their convenience.
Post-deployment, monitoring the AI forecasting system is crucial. Regular assessments will help identify how well the model performs in predicting workforce demand. Consider tracking key performance indicators, such as forecast accuracy and user engagement with the insights provided. By continuously refining the model based on performance feedback, you can ensure that AI workforce forecasting remains relevant and effective, helping your call center optimize staffing levels to meet customer demands efficiently.
Conclusion: Maximizing Success with AI Workforce Forecasting
To maximize success with AI Workforce Forecasting, organizations must embrace a structured approach. A well-defined strategy begins with understanding specific metrics that drive forecasting accuracy. By analyzing historical data patterns, businesses can fine-tune their AI models, ensuring they reflect current and future demand.
Moreover, continuous evaluation of these models is crucial. Regular monitoring allows teams to make timely adjustments based on shifting trends. Fostering collaboration between technology and frontline staff also enhances the effectiveness of AI-driven insights, leading to well-informed operational decisions and improved customer experiences. Ultimately, comprehensive and proactive strategies are key to achieving optimal outcomes in workforce demand forecasting.
๐ฌ Questions about How to Implement AI-Based Call Center Workforce Demand Forecasting?
Our team typically responds within minutes