AI Decision Agents have emerged as pivotal tools in today’s data-driven world, redefining how organizations make strategic choices. As businesses face an overwhelming influx of data, these intelligent systems can analyze vast amounts of information, identify trends, and provide actionable insights, ultimately enhancing decision-making processes. The integration of AI Decision Agents enables organizations to respond swiftly to changing market dynamics, ensuring they stay competitive and relevant.
Understanding and implementing AI Decision Agents requires thoughtful consideration of your specific goals and operational needs. By effectively leveraging these advanced technologies, organizations can transform uncertainty into informed strategies, leading to improved outcomes. This section will guide you through the fundamentals needed to harness AI Decision Agents for strategic decision-making, ensuring you maximize their potential in your operations.
Analyze & Evaluate Calls. At Scale.
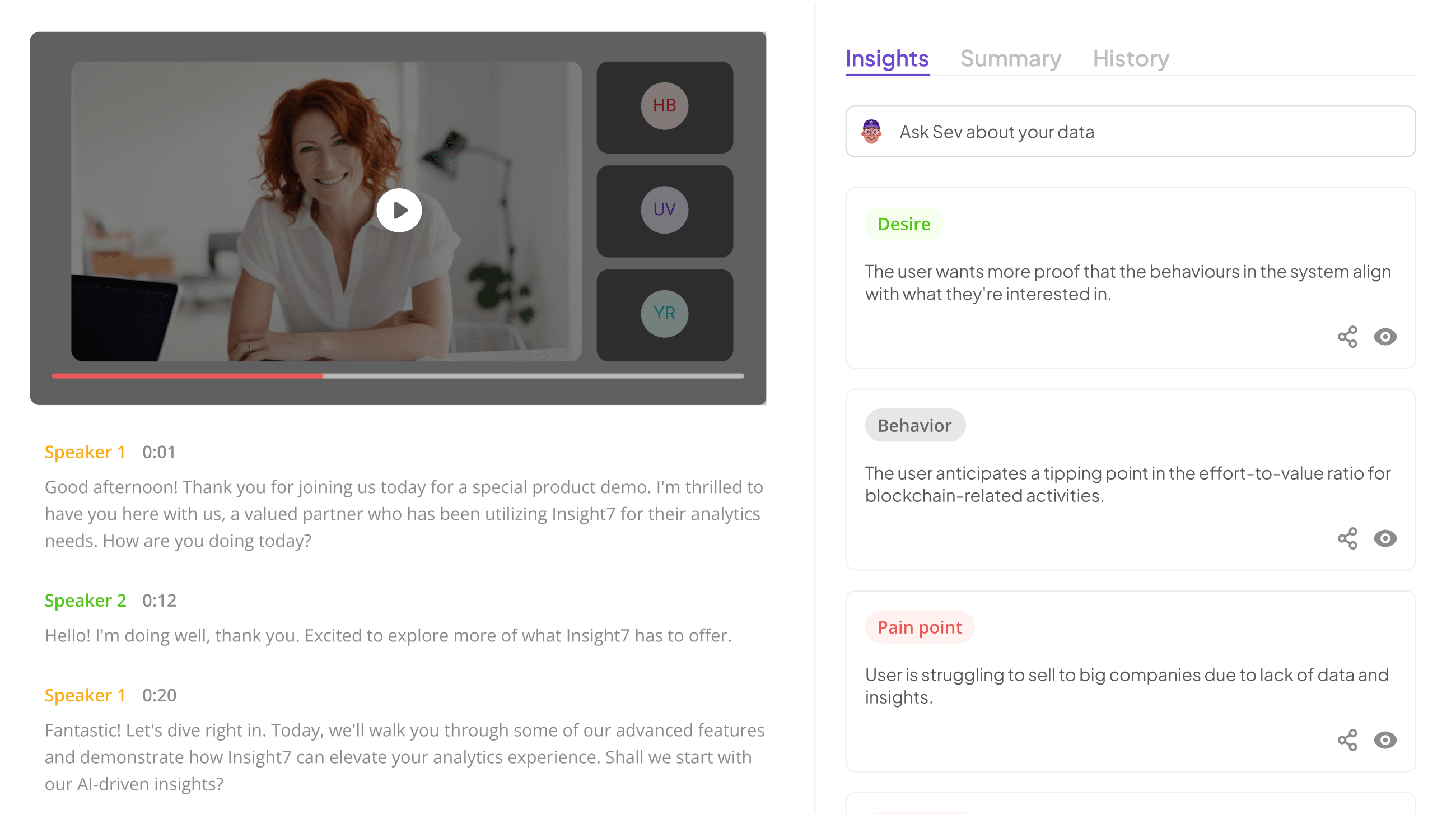
Understanding AI Decision Agents
AI Decision Agents represent a transformative approach to problem-solving in various contexts. These agents utilize algorithms and data to analyze complex situations, providing insights that inform strategic decision-making. They function by processing vast amounts of information rapidly, identifying patterns, and generating recommendations based on established parameters. This capability enables organizations to react swiftly to changing circumstances, ensuring that decisions are both timely and data-driven.
Understanding AI Decision Agents involves recognizing their potential applications within business strategy. They aid in optimizing processes, enhancing customer interactions, and improving overall operational efficiency. Furthermore, they empower teams to focus on high-stakes decisions rather than routine tasks, driving innovation and growth. As organizations progressively integrate these agents into their workflows, they not only improve decision accuracy but also cultivate a culture centered around data-informed choices, ultimately positioning themselves for sustainable success in today's competitive landscape.
What are AI Decision Agents?
AI Decision Agents are advanced systems designed to assist businesses in making strategic, data-driven decisions. They utilize complex algorithms to analyze vast amounts of data, providing insights that might be overlooked by human analysis alone. Essentially, these agents operate by mimicking human decision-making processes, allowing organizations to enhance their efficiency and effectiveness.
The core functions of AI Decision Agents include data interpretation, predictive analysis, and recommendation generation. By processing patterns and trends in historical data, they can forecast potential outcomes and suggest the best courses of action. This not only improves decision quality but also accelerates the decision-making process. With their ability to constantly learn from new data, AI Decision Agents evolve and adapt, ensuring that businesses remain agile in competitive markets. By integrating these agents into their operations, companies can drive innovation, respond quickly to market changes, and ultimately, achieve better financial performance.
The Role of AI Decision Agents in Business Strategy
AI Decision Agents play a pivotal role in shaping business strategy by providing insights that drive data-driven decisions. These intelligent systems analyze vast amounts of data, uncovering trends and predicting outcomes that can influence strategic initiatives. By integrating AI Decision Agents into decision-making processes, businesses can enhance their capability to respond promptly to market changes while minimizing human error.
Moreover, AI Decision Agents facilitate a more consultative approach by asking targeted questions and generating specific insights tailored to organizational needs. This personalized interaction not only increases accountability in decision-making but also helps establish measurable performance standards for strategic initiatives. As businesses continue to navigate a technology-driven environment, leveraging the capabilities of AI Decision Agents will be essential for maintaining competitive advantages and achieving strategic goals effectively.
Extract insights from interviews, calls, surveys and reviews for insights in minutes
Implementing AI Decision Agents: A Step-by-Step Guide
Implementing AI Decision Agents begins with clearly defining your strategic goals. By establishing these key objectives, you create a focused foundation that guides the entire implementation process. Once your goals are set, the next step is to choose the right tools for constructing your AI Decision Agents. Options such as DataRobot, H2O.ai, and IBM Watson are robust platforms that facilitate seamless integration of AI into decision-making processes.
Data collection and preparation are crucial for the success of AI Decision Agents. This phase involves gathering relevant data and cleaning it for analysis, ensuring your AI has the best information available. Following this, the design of your AI Decision Agents should align with the defined strategic goals, prioritizing clarity and specificity in its functionality. Finally, comprehensive testing and deployment ensure that the agents perform effectively in real-world scenarios, paving the way for informed, data-driven decisions that enhance your strategy.
Step 1: Identifying Strategic Goals
Identifying strategic goals is the foundational step in implementing AI Decision Agents for data-driven decisions. This process begins by defining clear, measurable objectives that align with your organization's vision and mission. Engaging stakeholders across departments ensures that the goals reflect diverse perspectives, fostering collaboration and enhancing the overall effectiveness of the AI agents.
Next, prioritize these goals based on their potential impact and feasibility. This may involve categorizing them into short-term and long-term objectives. For instance, immediate goals could focus on improving customer feedback analysis, while long-term ones might aim to optimize overall business processes. By solidifying these strategic goals, you create a roadmap that guides the development and deployment of AI Decision Agents, ultimately positioning your organization for success in a competitive landscape. With well-defined goals in place, teams can confidently move forward with the subsequent steps in the implementation process.
Step 2: Choosing the Right Tools for AI Decision Agents
Choosing the right tools for AI Decision Agents is crucial in optimizing their performance and effectiveness. Start by evaluating your specific needs and objectives. Different tools provide varied functionalities, from machine learning capabilities to data analysis and visualization. Assessing these features helps ensure that the chosen tools align with your strategic goals.
Next, consider factors such as ease of use and integration. User-friendly platforms allow quicker adaptation among team members, fostering a smoother implementation process. Furthermore, ensure that these tools can easily integrate with your existing data systems. To further streamline decision-making, tools like IBM Watson and TensorFlow enable advanced analytics and predictive capabilities. Tailoring your selection of tools to specific business requirements enhances the overall efficiency of AI Decision Agents, ultimately leading to more informed, data-driven decisions.
- insight7
In the context of implementing AI Decision Agents, organizations must navigate various complexities to ensure effective integration. This requires a clear understanding of strategic goals and thorough preparation of data. AI Decision Agents are powerful tools that can transform how businesses interpret customer insights and respond to market trends. They drive data-driven decisions, enabling companies to stay ahead of the competition by providing timely, actionable insights.
To effectively implement AI Decision Agents, organizations should consider several critical aspects: identifying their strategic goals is paramount, as it sets the direction for the AI integration. Next, choosing the right tools is essential, allowing businesses to capitalize on the strengths of various platforms. Following this, careful attention must be given to data collection and preparation, ensuring high-quality inputs for the AI agents. By focusing on these elements, organizations can leverage AI Decision Agents to streamline operations and foster a data-driven culture.
💬 Ask About This Article
Have questions? Get instant answers about this article.
- DataRobot
AI Decision Agents play a crucial role in enhancing data-driven strategies by streamlining the data analysis process. Utilizing an intuitive interface, businesses can engage with complex datasets more efficiently. This approach allows organizations to obtain actionable insights through automated reports, making strategic decision-making more accessible and effective.
There are key features essential for implementing AI Decision Agents smoothly. First, setting clear strategic goals helps align the AI's capabilities with company objectives. Next, selecting the right tools is vital; an advanced AI platform can further enhance data interpretation and visualization. Additionally, preparing and structuring data effectively ensures that the AI can generate accurate insights. Finally, testing the AI agents prior to deployment will help identify potential roadblocks, facilitating a seamless integration into existing workflows. By following these steps, organizations can harness the power of AI Decision Agents for strategic decision-making that is both informed and timely.
- H2O.ai
H2O.ai is a significant player in the realm of AI decision agents. These tools are designed to streamline decision-making through automated data analysis, thereby powerful insights. Remarkably, AI decision agents utilize advanced algorithms to identify patterns and correlations within large datasets, improving overall strategic planning. As organizations strive to make data-driven decisions, integrating these agents can offer substantial advantages.
When implementing such agents, organizations must first define their strategic goals. This clarity helps in selecting the most suitable algorithms and models for their specific needs. Once the goals are established, businesses can utilize H2O.ai’s tools for data preparation and automated machine learning processes, ensuring accuracy and efficiency. Testing these models before deployment guarantees that the insights generated align with the organization's objectives. In the ever-evolving landscape of data management, choosing the right AI decision agents is crucial for fostering informed decision-making.
- IBM Watson
The effectiveness of AI decision agents relies significantly on the advanced capabilities offered by various tools available in the market. One such tool provides robust frameworks for data analysis, natural language processing, and machine learning. Implementing these agents necessitates understanding how they interpret and process vast amounts of data to derive meaningful insights.
First, your team should identify the strategic goals for utilizing AI decision agents. This will guide the selection of the appropriate technology. The next step is to ensure quality data collection and preparation, as the accuracy of the AI-driven insights directly correlates with the data's integrity. Once the design of the agents is complete, rigorous testing is crucial before deployment to ensure efficiency and reliability in real-world applications. By systematically following these steps, organizations can effectively integrate AI decision agents, unlocking new levels of decision-making capability.
- TensorFlow
TensorFlow serves as a powerful open-source framework for developing machine learning models that can enhance AI Decision Agents. By facilitating the design, training, and deployment of various neural network architectures, TensorFlow empowers organizations to build robust systems capable of analyzing vast data sets. This capability allows AI Decision Agents to derive insights, predict outcomes, and ultimately drive strategic decisions based on real-time information.
The appeal of TensorFlow lies in its flexibility and scalability, supporting both simple and complex models. Users can easily define computations and continuously optimize their models through extensive libraries and tools. Moreover, TensorFlow's supportive community and comprehensive documentation provide valuable resources for developers, ensuring that implementing AI Decision Agents becomes a feasible task for businesses aiming to make informed decisions in today’s data-driven landscape. By harnessing TensorFlow, organizations can transform their approach to decision-making, making it more data-centric and efficient.
Step 3: Data Collection and Preparation
To effectively implement AI Decision Agents, data collection and preparation are critical steps in the process. Start by identifying the necessary data sources that align with your strategic goals. This might involve gathering internal data, such as sales figures or customer interactions, as well as external data such as market trends and competitor insights. Each type of data plays a pivotal role in ensuring your AI Decision Agents can analyze and provide valuable recommendations.
Once you have identified your data sources, the next step is to clean and organize the data. Data cleaning involves removing inaccuracies and inconsistencies, while organization ensures that it is structured for easy accessibility. Proper data preparation allows AI Decision Agents to efficiently process information, leading to more accurate and insightful outputs. By investing time in data collection and preparation, you lay a solid foundation for your AI agents, enhancing their capability to drive informed, strategic decisions.
Step 4: Designing the AI Decision Agents
Designing the AI Decision Agents involves crafting systems that can effectively interpret data and support strategic decision-making. The first step is defining the core objectives these agents should fulfill, which provides a foundation for their behavior and functionality. Specificity is vital in this stage; unclear requirements can lead to ineffective decision agents that fail to meet business needs.
Next, establish guidelines that clarify how these AI Decision Agents should operate. This includes setting accountability measures, performance standards, and evaluation criteria. By ensuring clarity, organizations can create agents that not only execute tasks but also provide valuable insights and feedback. Ultimately, well-designed AI Decision Agents can transform data into actionable strategies, enhancing overall decision-making processes.
Step 5: Testing and Deployment
The deployment of AI Decision Agents is a crucial step that ensures these systems are viable in a real-world context. Initially, you’ll want to conduct rigorous testing to validate their functionality. This includes assessing their performance against predefined benchmarks and ensuring that they consistently produce accurate, reliable results. Attention to detail is vital here; any errors or inaccuracies can lead to flawed decision-making.
Once testing is complete and the AI Decision Agents meet all criteria, it’s time to move to deployment. This involves integrating the agents within existing systems and workflows. Ensure that all stakeholders are informed and trained, allowing for a smooth transition. Continuous monitoring and feedback loops should also be established, enabling adjustments as necessary to improve performance over time. This structured approach to testing and deployment paves the way for effective and strategic data-driven decisions.
Conclusion: The Future of AI Decision Agents in Strategic Decision Making
As companies increasingly adopt AI decision agents, their role in strategic decision-making will become more pronounced and transformative. These agents will not only analyze vast amounts of data but also provide insights that can enhance business strategies, personalizing recommendations based on specific needs and preferences. The evolution of AI decision agents is crucial for organizations seeking efficiency and adaptability in a fast-paced market.
In the future, AI decision agents will likely operate in partnership with human decision-makers, improving outcomes through real-time analytics and predictive modeling. This collaborative approach ensures that strategies are not only data-driven but also contextually relevant. Businesses that embrace this technology can anticipate market trends and respond proactively, solidifying their competitive edge in a dynamic environment. The ability to harness AI for strategic decision-making will define successful organizations in the years ahead.
💬 Ask About This Article
Have questions? Get instant answers about this article.