How to Implement AI Agents for Predictive Business Forecasting
-
Bella Williams
- 10 min read
Predictive AI forecasting is reshaping how businesses anticipate trends, allowing for informed decision-making. In an era where data is abundant, organizations face the challenge of converting raw information into actionable insights. By harnessing advanced algorithms and machine learning techniques, businesses can analyze historical data and predict future outcomes with remarkable accuracy. This approach not only enhances operational efficiency but also empowers companies to adapt to market shifts proactively.
As you explore predictive AI forecasting, it becomes essential to understand its significance in the business landscape. Companies equipped with predictive insights can allocate resources effectively, minimize risks, and seize opportunities. Implementing AI agents for this purpose may initially seem daunting, yet the right strategies can simplify the process, leading to sustained growth and competitive advantage.
Analyze & Evaluate Calls. At Scale.
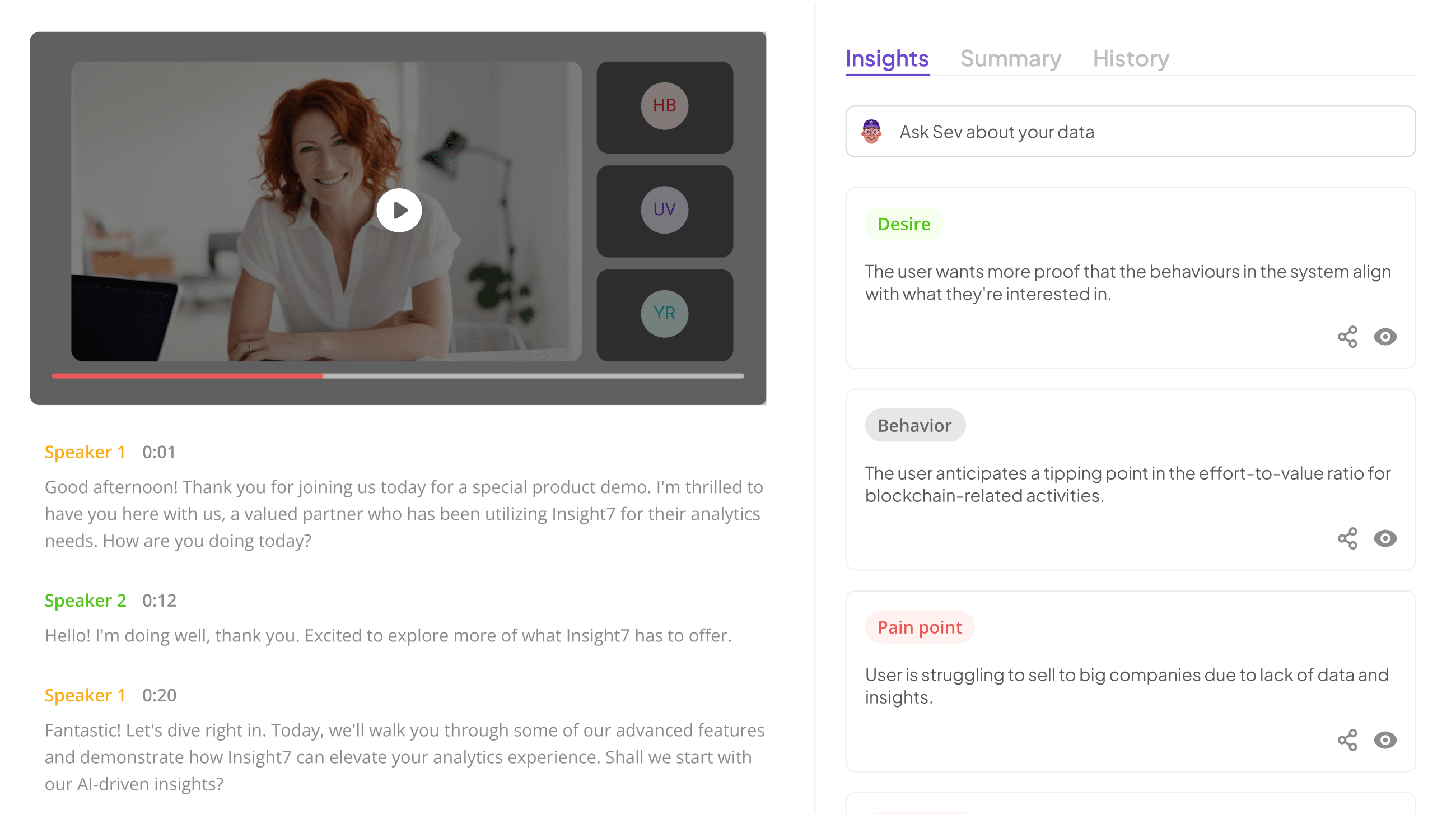
Understanding the Basics of Predictive AI Forecasting
Predictive AI forecasting utilizes advanced algorithms and historical data to make informed predictions about future business trends. By analyzing patterns and variables, businesses can anticipate market fluctuations and adjust their strategies accordingly. This process involves integrating vast amounts of data from various sources, which enhances decision-making and operational efficiency.
To effectively implement predictive AI forecasting, businesses must grasp its foundational concepts. First, understand how historical data can serve as a reliable indicator of future performance. Second, recognize the significance of data quality; clean and relevant data leads to more accurate forecasts. Lastly, familiarize yourself with different AI models and their applications in forecasting. These foundational components will empower organizations to harness predictive AI effectively, ultimately leading to improved operational strategies and enhanced competitive advantage.
What is Predictive AI Forecasting?
Predictive AI forecasting refers to the use of artificial intelligence techniques to analyze historical data and predict future outcomes. By leveraging advanced algorithms and machine learning models, businesses can forecast trends and behaviors with greater accuracy. This predictive capability enables organizations to make well-informed decisions based on anticipated market changes, customer preferences, and potential risks.
To effectively implement predictive AI forecasting, businesses must first identify relevant data sources, including sales history, market conditions, and customer feedback. Subsequently, AI models can be trained to recognize patterns and draw insights from this data. By continually evaluating the predictions against actual outcomes, companies can refine their methodologies over time, thus enhancing their forecasting accuracy. Overall, predictive AI forecasting empowers businesses to operate more efficiently, minimize risks, and capitalize on emerging opportunities in their respective markets.
The Importance of Predictive AI Forecasting in Business
Predictive AI forecasting plays a vital role in modern business strategies by enabling companies to anticipate market trends and consumer behavior. Implementing this technology allows organizations to make informed decisions backed by data-driven insights, reducing risks associated with uncertainty. Accurate forecasting can optimize inventory management, enhance customer satisfaction, and ultimately drive profitability.
Moreover, businesses that employ predictive AI forecasting can identify potential opportunities and challenges in advance. They can tailor marketing strategies and product offerings to meet evolving customer needs, thus gaining a competitive edge in their industry. By understanding the importance of predictive analytics, companies can not only streamline operations but also foster innovation through better anticipation of market dynamics. Adopting these forecasting methods is no longer an option; it is essential for sustaining growth in todayโs fast-paced business environment.
Implementing AI Agents for Predictive Business Forecasting
Implementing AI agents for predictive business forecasting involves a structured approach that aligns technology with organizational goals. First, identify specific business objectives, such as sales growth or inventory management. Clear objectives help establish a baseline for data requirements and desired outcomes. Next, gather and prepare your data meticulously. Data quality directly impacts the performance of AI models, so ensure it is accurate and relevant.
After data preparation, selecting the right AI models is crucial. Consider factors such as the complexity of your data and the desired forecasting accuracy. Once models are chosen, training and validating them is essential to ensure they operate effectively. This process requires continuous monitoring and optimization to adapt to changing business environments. By thoughtfully implementing these steps, organizations can foster robust frameworks for predictive AI forecasting, ultimately enhancing decision-making and driving success.
Extract insights from interviews, calls, surveys and reviews for insights in minutes
Key Steps in Setting Up Predictive AI Agents
Setting up predictive AI agents involves several crucial steps that pave the way for effective forecasting. First, itโs essential to identify your business goals and objectives, as these will dictate the framework for your predictive AI solutions. Clear objectives help shape the questions your AI agents will answer and guide your data collection efforts.
Next, collecting and preparing your data becomes vital. Ensure that the data is relevant, high-quality, and structured properly to facilitate accurate predictive modeling. With your data in place, selecting the right AI models is the next step, which can significantly impact the effectiveness of predictive AI forecasting. After choosing your models, training and validating them before full implementation is crucial, as it ensures their reliability and accuracy. Finally, continuous monitoring and optimization will help refine the AI agentsโ performance, adapting to new data and changing business environments. Following these steps will ensure a strong foundation for your predictive AI initiatives.
Step 1: Identify Business Goals and Objectives
To initiate the process of implementing Predictive AI Forecasting, it is essential to identify clearly defined business goals and objectives. Start by asking fundamental questions about what you aim to achieve with this technology. Consider objectives such as improving forecasting accuracy, enhancing inventory management, or optimizing customer engagement. Each of these goals will shape your strategy for utilizing predictive AI agents.
Next, ensure alignment between these goals and your overall business strategy. This alignment helps in articulating specific, measurable objectives, like reducing forecasting errors by a significant percentage or increasing sales conversions through targeted marketing. A clear understanding of your objectives will guide subsequent steps in the implementation process, from data collection to model selection, ensuring that your business harnesses predictive AI effectively and efficiently.
Step 2: Collect and Prepare Your Data
Effective data collection and preparation is crucial for successful predictive AI forecasting. Begin by gathering relevant datasets from multiple sources, including historical sales data, market trends, and customer feedback. This diverse array of data will help create a well-rounded model, enabling the AI agent to understand various influencing factors. It is essential to ensure data accuracy and completeness, as any gaps can lead to misleading predictions.
Next, clean and preprocess the data by removing duplicates and filling in missing values. This step enhances the quality of the data, ensuring it's ready for analysis. Also, consider normalizing or transforming data to create consistency in the dataset. By investing time in meticulous data preparation, you create a strong foundation for your predictive AI models, ultimately improving their performance and reliability in forecasting business outcomes.
Step 3: Choose the Right AI Models
To ensure effective predictive AI forecasting, choosing the right models is crucial. Different types of AI models, including regression algorithms, time-series analyses, and neural networks, cater to various forecasting needs. Itโs essential to understand your specific business requirements and data characteristics before making a selection. Consider the volume, velocity, and variety of your data, as these factors will influence model performance.
When evaluating models, think about accuracy, interpretability, and scalability. For instance, if you need quick insights and easy explanations, simpler models like linear regression may suffice. Conversely, complex scenarios might necessitate advanced neural networks. Explore options like decision trees or ensemble methods, which can enhance accuracy while managing large datasets. Finally, always test models using historical data to validate their forecasting capabilities before full implementation.
Step 4: Train and Validate Your Models
Training and validating your models is essential to achieving accurate predictive AI forecasting outcomes. Start by splitting your dataset into training, validation, and test sets, which enables you to train your model effectively while preventing overfitting. The training set is where the model learns patterns from the data, while the validation set assesses its performance in a controlled manner. Finally, the test set provides an unbiased evaluation of the modelโs predictive capabilities.
๐ฌ Questions about How to Implement AI Agents for Predictive Business Forecasting?
Our team typically responds within minutes
Once the training process is complete, carefully evaluate the model's performance using various metrics such as accuracy, precision, and recall. Continuous validation not only fine-tunes the model but also helps determine if adjustments are necessary. Iteratively refining your models will ensure they remain robust and reliable. Remember, the goal is to create predictive AI forecasting models that consistently deliver valuable insights for your business, leading to informed decision-making and strategic success.
Step 5: Implement Continuous Monitoring and Optimization
To ensure the success of your predictive AI forecasting efforts, continuous monitoring and optimization are essential. Regularly tracking the performance of your AI models allows for timely identification of areas needing adjustment. Monitoring key performance indicators (KPIs) such as accuracy, precision, and recall is crucial in this process. These metrics help gauge the effectiveness of your predictions and provide insight into how well your models align with actual business outcomes.
Optimization involves refining your AI models based on feedback and changing business conditions. Iteratively updating your algorithms enhances their predictive capabilities, enabling your organization to adapt to market trends. Embracing customer feedback further empowers your forecasting efforts, creating a cycle of improvement and responsiveness. By consistently evaluating and optimizing your predictive AI forecasting system, you position your business for sustained growth and agility in an ever-evolving marketplace.
Top Tools for Predictive AI Forecasting
Predictive AI forecasting relies on powerful tools that empower businesses to make data-driven decisions. Selecting the right tools is crucial for effective implementation. Start with insight7, which provides a user-friendly platform for turning raw data into actionable insights. Its capabilities allow teams to easily analyze customer interactions and extract key themes and pain points through automated workflows.
Next, consider IBM Watson, recognized for its advanced analytics and machine learning capabilities. It offers businesses the ability to build and train custom models tailored to their unique forecasting needs. DataRobot is another strong contender, providing an automated approach to model training and deployment, significantly reducing the time and expertise needed to generate accurate forecasts.
Google AI Platform and Microsoft Azure Machine Learning are also excellent options, both providing extensive resources and tools for creating and managing AI models at scale. These platforms integrate seamlessly with existing data infrastructures, allowing for a streamlined predictive forecasting process. By choosing the right combination of these tools, businesses can enhance their predictive accuracy and ultimately achieve better outcomes.
insight7
To excel in predictive AI forecasting, organizations must recognize the substantial role of continual learning. Insights gleaned from historical data shape future predictions and help refine decision-making processes. Therefore, having a robust system to seamlessly gather and analyze customer signals is crucial. Engaging with customers opens avenues to discover invaluable knowledge that can improve forecasting accuracy.
Moreover, integrating AI agents into the predictive forecasting model revolutionizes how businesses respond to market fluctuations. By harnessing real-time analytics, companies can anticipate shifts and react proactively rather than reactively. Cultivating a culture of data-driven insight leads not only to enhanced operational efficiency but also establishes a competitive edge in today's dynamic marketplace. As organizations prioritize predictive AI forecasting, they will discover that embracing innovation is key to sustainable growth and success.
IBM Watson
IBM Watson offers advanced capabilities for organizations looking to implement Predictive AI Forecasting. By harnessing its sophisticated algorithms and data processing power, businesses can identify trends and foresee potential market shifts with remarkable accuracy. This technology enables organizations to translate extensive data into actionable insights, enhancing decision-making processes and improving overall efficiency.
To effectively utilize this AI platform, companies must first integrate their existing data sources. Once the data is organized, the AI can be trained to recognize patterns that are relevant to the specific business context. Additionally, it allows continuous model refinement, ensuring that predictions remain accurate over time. By taking advantage of these features, organizations can gain a competitive edge, anticipate customer needs, and optimize their operational strategies in an ever-evolving market landscape.
DataRobot
DataRobot provides an intuitive platform designed to simplify Predictive AI Forecasting for businesses of all sizes. By automating essential processes, it enables organizations to harness powerful analytical tools without requiring extensive data science expertise. This means that even teams with limited resources can confidently build and deploy predictive models.
The platform offers a streamlined experience, helping users identify critical business objectives and align their data accordingly. Once the data is prepared, DataRobot employs advanced algorithms to generate accurate forecasts. Continuous monitoring is also a key feature, ensuring that models remain effective as underlying data changes. Overall, utilizing such a resource can significantly enhance decision-making, allowing businesses to anticipate market trends and adjust their strategies proactively.
Key Features of DataRobot
- Automated Machine Learning: Simplifies the modeling process by automating model selection and tuning.
- User-Friendly Interface: Ensures accessibility for users regardless of their technical background.
- Scalability: Supports projects from small scale to enterprise-level implementations.
- Integration Capabilities: Allows seamless connections with various data sources and business applications.
- Performance Monitoring: Offers tools for ongoing assessment and refinement of models for sustained accuracy.
These features collectively empower organizations to implement Predictive AI Forecasting strategies effectively, enhancing overall business performance.
Google AI Platform
The Google AI Platform serves as a powerful tool in the realm of Predictive AI Forecasting. It offers an accessible interface that allows businesses to create and implement machine learning models without requiring extensive expertise. This democratization of technology enables all team members, regardless of their technical background, to utilize the platform for data analysis and forecasting predictions. Users can seamlessly transform raw data into actionable insights, ensuring informed decision-making across various business contexts.
One of the platformโs standout features is its ability to analyze large datasets efficiently. It provides customizable solutions tailored to specific business needs, whether for identifying customer pain points or tracking market trends. By enabling collaborative workflows, the platform ensures that teams can work together to refine models and improve accuracy. Ultimately, the Google AI Platform positions organizations to make data-driven predictions, enhancing their strategic planning capabilities and fostering a competitive edge in the market.
Microsoft Azure Machine Learning
Microsoft Azure Machine Learning offers a robust environment for developing and deploying AI models tailored to predictive business forecasting. It provides an integrated platform where data scientists can build, test, and manage machine learning models seamlessly. This enables businesses to harness their data effectively, fostering predictive AI forecasting capabilities that enhance decision-making processes.
To implement AI agents using this platform, several important steps become essential. Firstly, businesses should define their specific forecasting goals, allowing the platform to cater to unique operational needs. Next, meticulous data collection and preparation are crucial, ensuring that all relevant datasets are clean and ready for analysis. After data preparation, selecting suitable AI models becomes vital, as different scenarios may require varied approaches tailored to historical data patterns and future predictions. Lastly, ongoing monitoring and optimization of the models guarantee that the forecasting remains accurate and relevant over time. By adopting these practices within Microsoft Azure Machine Learning, organizations can significantly improve their forecasting accuracy and yield significant business insights.
Conclusion: Leveraging Predictive AI Forecasting for Competitive Advantage
In today's competitive environment, harnessing the power of predictive AI forecasting can significantly impact business success. By accurately anticipating trends and customer behavior, organizations can make informed decisions that enhance efficiency and drive growth. Utilizing AI agents transforms data into actionable insights, allowing businesses to respond swiftly to market changes.
Furthermore, practical implementation of these technologies empowers teams at all levels to contribute to organizational strategy. When businesses prioritize predictive AI forecasting, they not only optimize their resources but also position themselves ahead of competitors. Embracing this approach is essential for those aiming to thrive in a rapidly evolving market.
๐ฌ Questions about How to Implement AI Agents for Predictive Business Forecasting?
Our team typically responds within minutes