How to Identify Patterns in Research Debriefs Using AI
-
Hello Insight
- 10 min read
AI-Driven Pattern Recognition in research debriefs represents a transformative shift in how we analyze data. Traditionally, identifying trends from interviews and surveys required extensive manual effort, often leading to biases and overlooked insights. However, with advancements in AI, we can now process vast amounts of information efficiently, revealing significant patterns that inform decision-making.
This integration of technology enhances our ability to derive actionable insights swiftly and accurately. By automating this process, researchers can focus more on interpreting data, ultimately improving the quality and speed of their findings. Understanding how to harness AI-Driven Pattern Recognition equips professionals with the tools needed to excel in a data-driven environment.
Analyze & Evaluate Calls. At Scale.
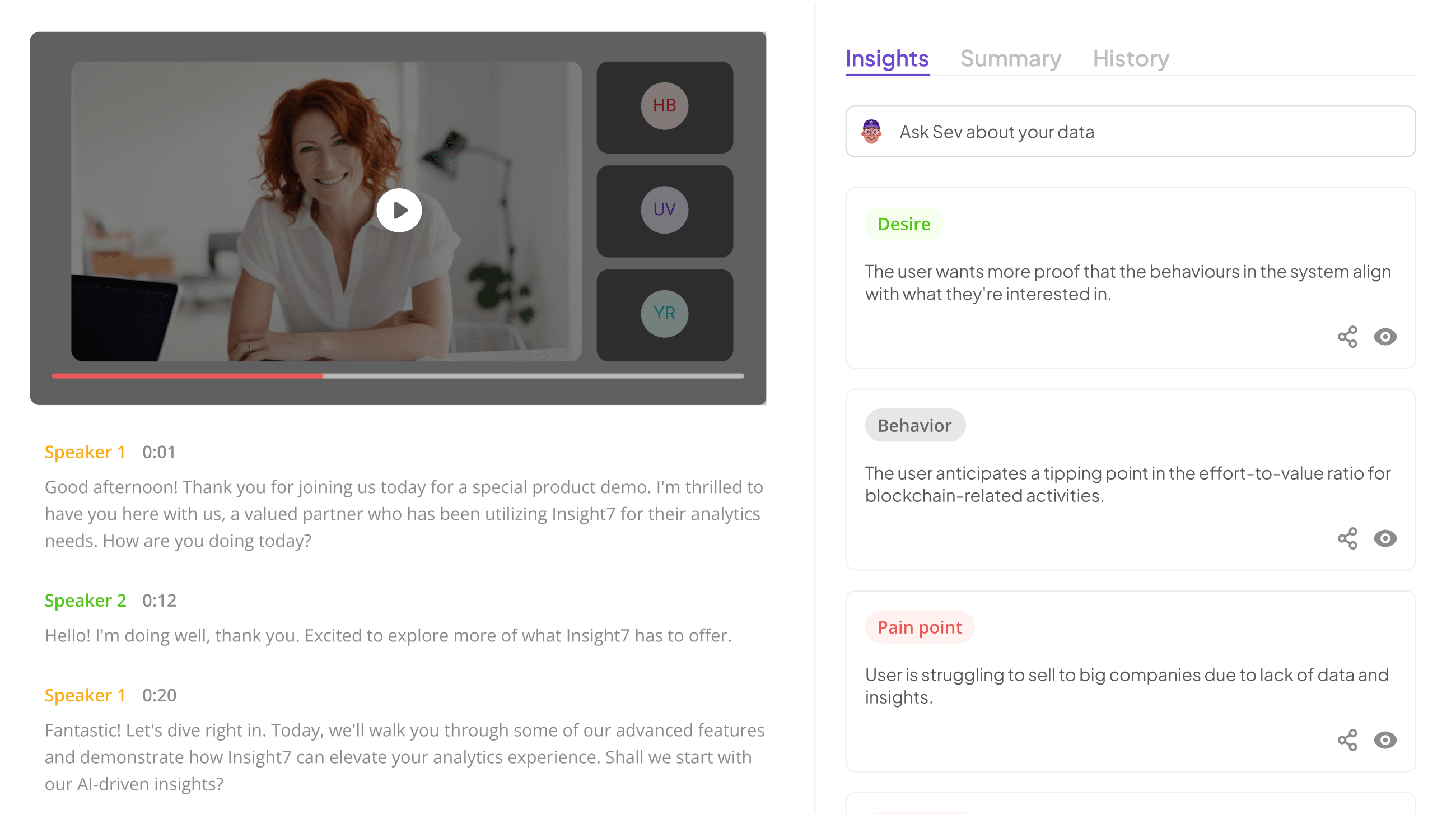
The Role of AI-Driven Pattern Recognition in Research Debriefs
AI-Driven Pattern Recognition plays a pivotal role in the analysis of research debriefs, helping teams streamline processes and focus on significant insights. By automating the identification of patterns within qualitative data, researchers can save time that would otherwise be spent on labor-intensive manual analysis. This technology not only increases efficiency but also reduces biases typically introduced by varied interpretative styles among team members.
In practice, applying AI-driven methods allows for quick synthesis of findings from interviews and open-ended survey responses. As a result, research teams gain access to consistent insights, facilitating faster decision-making. By utilizing AI tools, they can effectively capture key themes across large datasets, ultimately enhancing the quality and depth of their analysis. Implementing these technological advancements in research debriefs empowers organizations to harness valuable insights and stay competitive in their respective fields.
Identifying Key Insights with AI-Driven Pattern Recognition
AI-Driven Pattern Recognition can transform the way researchers extract valuable insights from their data. By utilizing advanced algorithms, this technology enables the identification of hidden trends that might otherwise go unnoticed. The automatic detection of patterns allows researchers to focus more on high-level insights rather than getting bogged down by manual data analysis processes.
The benefits of adopting AI in pattern recognition extend beyond speed. It enhances accuracy, reduces human error, and brings greater consistency in identifying critical themes within research debriefs. Researchers can cultivate a more nuanced understanding of their data, leading to informed decisions rooted in empirical evidence. Consequently, AI-Driven Pattern Recognition fosters a more efficient workflow, allowing professionals to generate meaningful reports swiftly and effectively, using focused keywords to hone in on the most relevant insights. This approach not only streamlines analysis but also empowers organizations to act on data-driven decisions with confidence.
- Explanation of how AI can detect and highlight essential patterns and insights.
AI can effectively identify and highlight essential patterns and insights by analyzing vast amounts of data far beyond human capability. This process involves sifting through complex datasets to detect trends that may be overlooked in manual analyses. By employing sophisticated algorithms, AI can reveal patterns that bathe hidden opportunities and highlight critical areas for focus in research debriefs.
The key advantage of this AI-driven pattern recognition lies in its speed and accuracy. It can instantly process multiple variables and dimensions, presenting insights that are crucial for informed decision-making. Furthermore, AI enhances the visibility of insights by integrating natural language processing, allowing it to interpret both context and sentiment within the data. This multifaceted understanding ensures researchers receive comprehensive and actionable insights tailored to specific themes, enabling them to craft more precise reports and recommendations.
- Benefits of automated pattern recognition over manual analysis.
Automated pattern recognition provides several distinct advantages over manual analysis, most notably in speed and accuracy. With the help of AI-Driven Pattern Recognition, research teams can process vast amounts of data much quicker than human analysts. While manual analysis often leads to inconsistencies and biases due to subjective interpretations, AI tools can deliver objective results consistently. This reduction in human error ensures that insights are based solely on data rather than individual perspectives.
Moreover, the efficiency of automated systems allows researchers to focus on more strategic tasks. Instead of spending days analyzing qualitative data, teams can utilize AI technologies to identify trends and key insights more swiftly. This not only streamlines the research process but also enhances collaboration among team members. By utilizing AI-Driven Pattern Recognition, businesses can respond to client needs more effectively, ultimately increasing their competitive advantage in the market.
Tools for AI-Driven Pattern Recognition
AI-driven pattern recognition tools play a crucial role in enhancing the efficiency and accuracy of research debriefs. Various platforms cater to different needs, enabling researchers to extract valuable insights quickly and systematically. The market is vibrant with tools specifically designed to assist in recognizing patterns, streamlining the research process significantly.
Prominent tools include Insight7, known for its robust capabilities in identifying research patterns, and MonkeyLearn, a versatile platform that excels in text analysis. Additionally, RapidMiner offers comprehensive data science functionalities that include pattern recognition. For visualizing insights, Tableau is popular among researchers for its intuitive interface. Lastly, IBM Watson Studio leads in providing AI-driven insights, making it a reliable option for in-depth research analysis. Leveraging these tools can transform how researchers interpret data, turning complex information into actionable insights.
- insight7: Leading tool for identifying research patterns.
insight7 stands out as an exceptional tool designed to enhance the identification of research patterns. With its advanced AI-driven pattern recognition features, this tool enables users to analyze complex datasets effortlessly. By utilizing machine learning algorithms, it identifies recurring themes and insights, thus streamlining the research process. Researchers can quickly derive relevant findings tailored to specific objectives, saving time and resources in the analysis phase.
Moreover, insight7 allows users to define goals and customize their tags, facilitating a more refined approach to data exploration. By clustering insights based on defined parameters, it becomes easier to extract meaningful information and sentiments. The combination of intuitive design and powerful analytical capabilities positions this tool as a valuable asset for any researcher looking to harness data effectively. Ultimately, the accuracy and efficiency of AI-driven pattern recognition can significantly elevate the quality of research outcomes.
- MonkeyLearn: A versatile platform for text analysis.
The versatility of a comprehensive text analysis platform allows researchers to efficiently extract valuable insights from their data. By utilizing powerful AI-driven pattern recognition capabilities, users can automate the identification of themes and relationships within vast amounts of text. This process not only streamlines data analysis but also enhances accuracy, reducing the risk of human oversight that commonly occurs with manual evaluations.
Key features of this platform include native transcription services that accommodate diverse data formats, allowing researchers to input audio and video files seamlessly. Once the data is transcribed, users can analyze it collectively or individually. The focus remains on extracting pertinent quotes and themes, empowering researchers to pose specific questions that lead to actionable insights. Through this integration of technology, AI-driven pattern recognition transforms the way insights are harvested and utilized, reinforcing the importance of recognizing patterns in research debriefs effectively.
- RapidMiner: Comprehensive data science software with pattern recognition capabilities.
AI-Driven Pattern Recognition serves as a cornerstone in modern data analysis, particularly in research debriefs. This powerful software enables users to sift through large volumes of data effortlessly, identifying patterns and trends that might otherwise go unnoticed. By employing sophisticated algorithms, it can mark essential themes and insights that emerge from the data, ultimately enhancing the quality of research outcomes.
Additionally, using such a tool streamlines the analysis process significantly. For example, it allows users to categorize data according to predefined goals, such as retention or user experience. This structured approach not only saves time but also facilitates a more nuanced understanding of insights, enabling researchers to trace findings back to their sources. In this way, comprehensive data science software can transform raw information into actionable strategies, underscoring the profound impact of AI-driven pattern recognition on research efficacy.
- Tableau: A popular tool for visual pattern analysis.
Tableau has established itself as a leading software for visual pattern analysis, particularly beneficial for researchers seeking to uncover meaningful insights in their data. By offering an intuitive interface and powerful visualization capabilities, Tableau enables users to transform complex data sets into easily interpretable visual formats. This visual representation is crucial for identifying trends and patterns, making it an essential tool in the realm of AI-driven pattern recognition.
Utilizing Tableau, researchers can explore various dimensions of their data through interactive dashboards and visualizations. This not only aids in recognizing emerging patterns but also facilitates collaborative discussions around research findings. When integrated with AI tools, Tableau enhances the ability to automatically highlight significant patterns, ensuring researchers can focus on the insights that matter the most. By visualizing AI-generated results, Tableau empowers users to make informed decisions and strategize effectively based on clear data interpretations.
- IBM Watson Studio: Engineered for AI-driven insights.
The application of advanced analytical tools can significantly enhance the understanding of research debriefs. At the forefront of this innovation is a platform specifically designed to yield AI-driven insights. By using state-of-the-art algorithms, users can effortlessly identify and visualize intricate patterns hidden within vast datasets. This capability allows researchers to filter findings based on specific themes and topics, resulting in actionable insights tailored to their needs.
Moreover, the integration of AI-driven pattern recognition facilitates a more efficient workflow. Users can generate reports in minutes, selecting key insights and observations to include. This process not only accelerates the analysis but also improves the quality and accuracy of the findings. The ability to quickly summarize essential data enables organizations to pivot strategies and make informed decisions. As AI technology continues to evolve, embracing these tools will be crucial for researchers aiming to harness the full potential of their data.
Extract insights from interviews, calls, surveys and reviews for insights in minutes
Steps to Implement AI-Driven Pattern Recognition in Research
Implementing AI-Driven Pattern Recognition in research requires a systematic approach to ensure optimal outcomes. First, it is essential to prepare your data meticulously for AI analysis. This involves cleaning, structuring, and organizing the research data to ensure its quality and relevance. Properly formatted data allows AI algorithms to function effectively and extract valuable insights accurately.
Next, selecting the right AI tools is critical. Assess your specific needs and identify tools that align with your research objectives. Consider factors such as ease of use, functionality, and integration with existing systems to make an informed decision. After obtaining results from your chosen tools, interpreting the AI-generated patterns comes into play. Familiarize yourself with techniques that enable you to understand these insights in the context of your research objectives. This will ensure that your findings are actionable and can inform future research decisions.
Step 1: Preparing Data for AI Analysis
To harness AI-driven pattern recognition effectively, the first step is preparing your data for analysis. This involves carefully structuring and cleaning your research data to ensure accuracy and meaningful insights. Begin with defining the scope of your analysis and determining what data is relevant. Collecting qualitative and quantitative data in a consistent format helps streamline the analysis process.
Next, meticulously clean the data by removing duplicates, correcting errors, and standardizing terminology. Filtering out irrelevant or noisy information enhances the quality of insights generated by AI tools. Once your data is organized, uploading it to the selected AI platform is seamless. The combination of well-prepared data and advanced AI capabilities significantly boosts your ability to identify patterns and derive impactful insights from research debriefs. Proper preparation establishes a solid foundation for successful AI-driven analysis.
- How to structure and clean research data before AI analysis.
Before diving into AI-Driven Pattern Recognition, it’s essential to structure and clean research data effectively. The initial phase involves organizing your raw data, categorizing it into relevant themes such as participant demographics, interview responses, and open-ended survey feedback. This organization not only aids clarity but also enhances the accuracy of subsequent AI analyses.
Next, cleaning the data is crucial for reliable insights. This step includes removing duplicates, correcting inconsistencies, and addressing missing values. Inconsistencies can lead to flawed interpretations, skewing the AI's ability to identify genuine patterns. Applying qualitative coding techniques can further help in establishing uniformity across the data. By investing time in these foundational steps, you'll set the stage for a successful AI analysis that yields meaningful insights and identifies authentic patterns in your research debriefs.
Identifying Key Insights with AI-Driven Pattern Recognition
AI-Driven Pattern Recognition is a revolutionary approach that enables researchers to uncover critical insights from vast amounts of data. By utilizing advanced algorithms, AI can effectively analyze research debriefs and recognize patterns that might be invisible to the human eye. This automated analysis saves time, enhances accuracy, and allows researchers to focus on interpreting the results instead of sifting through data manually.
Automated pattern recognition offers various benefits. It reduces the risk of human error, enables the identification of recurring themes, and can provide real-time insights, enhancing decision-making processes. Additionally, AI tools can handle complex datasets, improving the overall efficiency of research efforts. By integrating this technology, teams can make data-driven decisions faster, leading to more effective strategies and solutions. Embracing AI in research debriefs is not just a trend but a crucial step towards intelligent and insightful decision-making.
Step 2: Selecting the Right AI Tools
When selecting the right AI tools for pattern recognition in research debriefs, clarity on your objectives is essential. Start by identifying what specific insights you wish to extract from your data, as different tools offer varied capabilities. Some platforms excel at text analysis, while others provide advanced visualizations that can help you uncover meaningful trends. The right choice should align with your goals and the complexity of your data.
Next, consider the usability of the AI-driven tools. A user-friendly interface can save time during analysis and improve the efficiency of your workflow. Look for tools that facilitate customization and allow for easy integration with your existing data sources. Finally, assess the scalability of each tool, ensuring it can accommodate your analytical needs as your research scales. By choosing tools wisely, you can enhance the ability to uncover significant patterns and insights from your research debriefs.
- Criteria for choosing the best AI tools for your needs.
When selecting the appropriate AI tools for your needs, it’s essential to consider a few critical factors. The first step is to assess the specific requirements of your research objectives. Identify what patterns you aim to uncover and how AI can enhance that process. Streamlining your operations with AI-driven pattern recognition can save time and improve accuracy, but tools should align with your goals.
Next, evaluate the usability and integration capabilities of potential tools. The ideal AI solution should be user-friendly and seamlessly integrate with your existing systems. Additionally, consider the scalability of these tools; as your research needs evolve, your chosen AI should accommodate that growth. Lastly, examine the support and resources available for each tool. Comprehensive tutorials and responsive customer support can significantly ease the transition to AI-driven analyses. When you weigh these criteria, you empower your research with the most effective technology for your specific needs.
Step 3: Interpreting AI-Generated Patterns
To effectively interpret AI-generated patterns, it is essential to understand the insights provided through AI-driven pattern recognition. This technology analyzes large datasets, identifying meaningful connections and trends that could easily be overlooked through manual analysis. By clustering insights into specific themes, researchers can discern factors that impact their objectives, such as risk and member experience. Coding the data according to predefined tags allows for targeted analysis, making it easier to track sentiments and unique participant voices.
Moreover, once patterns are generated, researchers should actively engage with the data to draw conclusions. This means examining the insight clusters to determine their implications on decision-making. An interactive approach helps in validating findings and ensures that subsequent actions are rooted in robust analysis. By maintaining clarity on the research goals, you can efficiently relate AI insights to your strategies, maximizing the utility of AI-generated patterns in your research debriefs.
- Techniques for understanding and leveraging AI findings in research debriefs.
Understanding AI findings in research debriefs is instrumental for insights that drive decision-making. Adopting AI-Driven Pattern Recognition techniques can simplify the analysis process, making it quicker and bias-free. One effective approach is ensuring data preparation includes cleaning and structuring. This step maximizes AI's capacity to uncover patterns that may be missed during manual analysis.
Another vital technique involves selecting the right AI tools. Tools like IBM Watson Studio and Tableau can offer automated insights, allowing researchers to focus on interpretation. Furthermore, fostering a culture of collaboration within teams encourages diverse perspectives to interpret AI-generated patterns accurately. Finally, continuous learning and adaptation to AI's evolving capabilities can enhance the effectiveness of research debriefs. By integrating these strategies, teams can unlock valuable insights and streamline their analysis processes.
Conclusion: The Future of AI-Driven Pattern Recognition in Research
As we look towards the future, the role of AI-driven pattern recognition in research is poised for exponential growth. The demand for faster and more accurate insights will drive researchers to adopt AI technologies, transforming traditional methodologies. Continuous advancements in machine learning and data processing capabilities will enhance the effectiveness and reliability of these tools, making them essential for extracting meaningful patterns from vast data sets.
Additionally, the integration of AI-driven pattern recognition will pave the way for more innovative applications across various research fields. By automating processes and reducing human bias, researchers will be empowered to focus on strategic analysis rather than data collection. As AI evolves, the potential for groundbreaking discoveries using these tools will revolutionize how research is conducted and insights are derived, shaping the future of decision-making in numerous industries.