In today’s dynamic call center environment, Predictive CX Forecasting acts as a beacon of insight, guiding organizations towards enhanced customer experience. By harnessing the power of data and analytics, businesses can anticipate customer needs, improve service quality, and optimize resource allocation. This forecasting approach not only informs strategies but also empowers call center teams to deliver personalized support, thereby fostering strong customer relationships.
As we delve deeper into the principles of Predictive CX Forecasting, it becomes clear that understanding customer behavior is crucial. This understanding aids in the development of effective predictive models, which can significantly influence decision-making processes. By analyzing historical data, organizations can identify trends and patterns, ensuring they remain proactive rather than reactive in their customer service efforts.
Analyze qualitative data. At Scale.
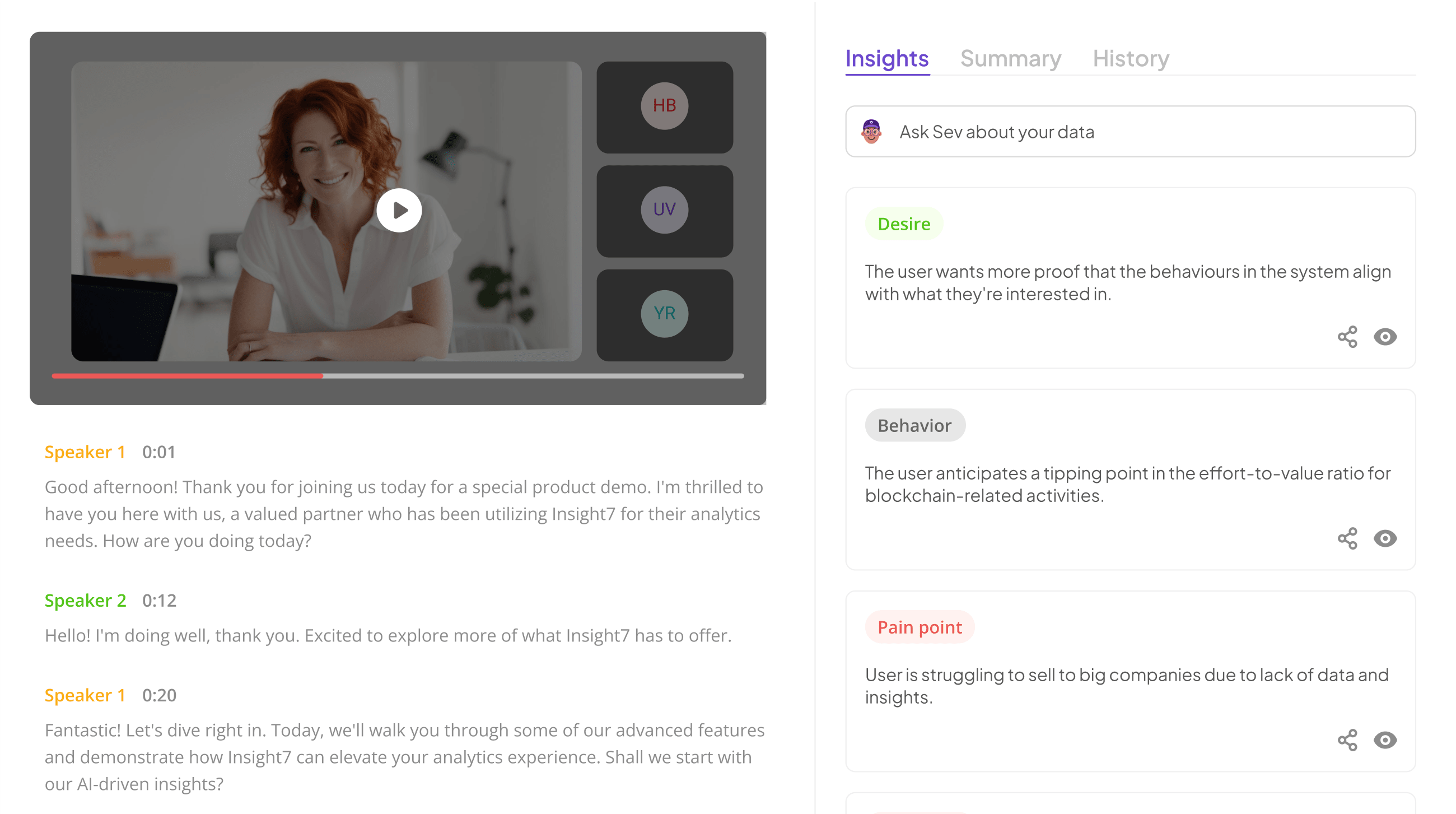
Building the Foundation for Call Center Predictive CX Forecasting
Building a strong foundation for Call Center Predictive CX Forecasting involves several critical steps that ensure accurate and actionable insights. First, understanding the fundamentals of predictive models is essential. These models analyze historical data to forecast future customer behaviors, preferences, and needs. By comprehending these elements, organizations can tailor their strategies effectively within the call center environment.
Next, quality data collection is paramount. Robust datasets enable the accurate application of analytical techniques and algorithms. This means gathering comprehensive information from various sources, such as customer interactions and feedback. Once the data is in place, employing various analytical methods will help uncover patterns that drive customer experience outcomes. Establishing a systematic approach to testing and refining these models will enhance forecasting accuracy and improve decision-making processes, ultimately leading to better customer satisfaction.
Understanding the Basics of Predictive CX Forecasting
Predictive CX Forecasting refers to the use of data analysis techniques to predict customer experience outcomes in call centers. By examining historical interaction data, businesses can spot patterns that shed light on customer behaviors and preferences. This forecasting tool enables call centers to anticipate customer needs, enhancing service delivery and satisfaction.
The importance of Predictive CX Forecasting in the call center environment cannot be overstated. It helps businesses allocate resources more effectively, ensuring that representatives are prepared for expected call volumes and types. Moreover, by identifying potential customer pain points before they arise, organizations can implement proactive measures to improve service quality. This not only leads to higher customer satisfaction but also fosters customer loyalty, resulting in long-term business success. Understanding these fundamentals is crucial for organizations aiming to harness the full power of data-driven insights in their customer interactions.
- Explanation of Predictive CX Forecasting
Predictive CX Forecasting fundamentally changes how organizations interpret data to enhance customer experiences. By analyzing historical interaction patterns and trends, businesses can anticipate future customer behaviors. This process relies on sophisticated statistical models and machine learning techniques, transforming raw data into actionable insights. These insights allow businesses to make informed decisions that improve customer satisfaction and loyalty.
In call centers, Predictive CX Forecasting is crucial for optimizing operations and enhancing service delivery. It enables organizations to align resources according to anticipated demand trends, reducing wait times and improving service levels. Additionally, it helps to identify potential issues before they escalate, allowing businesses to proactively address flaws in the customer journey. By understanding and applying these forecasting models, companies can significantly elevate their customer experience and drive meaningful engagement.
- Importance in the Call Center Environment
Predictive CX forecasting holds significant importance in the call center environment, where customer interactions can greatly influence overall business success. By accurately predicting customer behavior and needs, call centers can refine their operations, ensuring that agents are equipped with the right tools and knowledge. This proactive approach improves customer satisfaction and enhances loyalty, which is crucial in today’s competitive landscape.
In practical terms, effective predictive models allow call centers to identify patterns in customer calls and tailor their responses accordingly. This results in quicker resolutions for customers, leading to improved experience and efficiency. Additionally, by understanding which customer issues arise most frequently, management can focus training efforts on key areas, thus continually evolving the customer service experience. Therefore, integrating predictive CX forecasting into daily operations not only aids in managing current customer expectations but also prepares the call center for future challenges.
Key Components for Effective Predictive CX Forecasting
Successful Predictive CX Forecasting hinges on several key components, each intricately connected to delivering exceptional customer experiences. First, focus on data collection and quality, as the foundation of accurate forecasting starts with reliable data sources. Effective data gathering ensures that insights reflect true customer sentiments and behaviors. It is essential to eliminate noise and inaccuracies to enhance the reliability of forecasts.
Next, analytical techniques and algorithms play a vital role in understanding customer trends and predicting future outcomes. By utilizing advanced modeling methods, businesses can transform raw data into meaningful insights that drive decisions. Careful application of these analytics helps in identifying potential issues before they escalate and enables companies to tailor their services effectively. Ultimately, blending high-quality data with robust analytical frameworks empowers organizations to enhance customer experience in a meaningful, data-driven way.
- Data Collection and Quality
Gathering the right data is crucial for effective Predictive CX Forecasting. To start, focus on ensuring that data collected from multiple sources, like customer calls and surveys, is both accurate and relevant. A variety of data points can provide insights into customer behavior, preferences, and pain points. This depth of information enables better analysis and ultimately more accurate forecasting.
Quality is just as important as quantity when it comes to data collection. High-quality data means it’s clean, consistent, and free from biases. Regular audits must be conducted to validate the integrity of the data, ensuring it aligns with the evolving needs of the business. By prioritizing data collection and quality, organizations can build a solid foundation for Predictive CX Forecasting, enabling them to anticipate customer needs and improve overall satisfaction.
- Analytical Techniques and Algorithms
Analytical techniques and algorithms play a crucial role in effectively forecasting customer experience (CX) outcomes. By harnessing various methodologies, organizations can transform raw call data into actionable insights that drive improvement in service quality. Techniques such as regression analysis, decision trees, and machine learning algorithms facilitate the identification of patterns and correlations within vast datasets. These analytical tools enable teams to predict customer behavior, allowing for proactive responses to emerging trends.
Furthermore, employing algorithms that incorporate customer sentiment analysis can enhance the quality of predictions. By understanding vocal tone and word choice in customer interactions, organizations gain deeper insights into customer satisfaction levels. This approach not only assists in addressing current issues but also aids in strategic planning for future enhancements. Ultimately, the integration of sophisticated analytical techniques is key to successful predictive CX forecasting, fostering a more responsive and adaptive call center environment.
Extract insights from interviews, calls, surveys and reviews for insights in minutes
Steps for Implementing Call Center Predictive Models for CX Outcomes
Implementing call center predictive models for CX outcomes involves a structured approach to ensure accuracy and relevance. The first crucial step is setting clear objectives for predictive CX forecasting, which entails identifying key customer experience goals and aligning them with the broader business objectives. This alignment guarantees that the models developed will directly impact your desired outcomes, thereby providing greater value.
Next, selecting and utilizing the right tools is essential. This may include platforms like IBM Watson Analytics or NICE Nexidia, which can facilitate effective data analysis. Afterward, focus on analyzing data and training models. This step requires segmenting and preparing your data to enhance the model's accuracy during training and validation. Finally, continuous performance monitoring and iterative refinements are vital for maintaining and improving model efficacy. This comprehensive approach ensures that predictive CX forecasting remains a valuable asset in enhancing customer experiences in call centers.
Step 1: Setting Clear Objectives for Predictive CX Forecasting
To embark on Predictive CX Forecasting, it is essential first to set clear objectives. This involves identifying the key customer experience goals that align with your organization’s overarching business outcomes. By defining these objectives, you create a focused direction that guides the entire forecasting process. It is important to consider what aspects of customer experience you want to enhance, whether it's response times, resolution rates, or customer satisfaction levels.
Next, ensure that these goals are measurable and achievable. This means establishing key performance indicators that will help evaluate success. By aligning these objectives with specific business outcomes, such as increased retention or sales growth, you establish a clear pathway for utilizing predictive models effectively. Clarity in objectives not only streamlines efforts but also helps in ensuring that everyone involved understands the end goals. Setting these foundational elements is crucial for advanced forecasting strategies to thrive.
- Identifying Key Customer Experience Goals
Identifying key customer experience (CX) goals is essential for successful predictive CX forecasting. To achieve meaningful outcomes, organizations must clearly define what they aim to enhance. Common goals include improving customer satisfaction, increasing sales conversion rates, and shortening the onboarding processes for new employees. Each of these objectives must align with broader business outcomes to create a cohesive strategy that drives results.
To enhance the efficacy of predictive CX forecasting, it is crucial to consider both quantitative and qualitative metrics. For instance, tracking average ticket prices and customer satisfaction scores are tangible indicators of success. Furthermore, analyzing training effectiveness for customer service representatives can reveal areas for improvement, directly impacting customer interactions. By setting these clear goals, organizations can tailor their strategies, ensuring that data-driven insights lead to actionable results and a more satisfying customer journey.
- Aligning with Business Outcomes
Aligning customer experience outcomes with overarching business goals is crucial for any call center. To achieve this alignment, organizations must clearly define their objectives and understand how predictive CX forecasting can facilitate their strategic vision. Identifying key performance indicators enables teams to track customer satisfaction while ensuring that their efforts resonate with broader business outcomes.
Successful alignment requires a structured approach. First, organizations should assess their current performance metrics and understand how these relate to customer experience goals. Next, they can utilize predictive analytics to forecast outcomes and visualize customer trends. Finally, continuous feedback loops from both customers and agents inform refinement processes, ensuring that initiatives are persistently aligned with evolving business objectives. By integrating predictive CX forecasting into strategic planning, call centers can enhance customer experiences and drive greater business success.
Step 2: Selecting and Utilizing the Right Tools for Predictive Modeling
Selecting the right tools for predictive modeling is crucial in forecasting CX outcomes effectively. Start by evaluating options that best fit your organizational needs and capabilities. Tools such as IBM Watson Analytics and Avaya Predictive Analytics come highly recommended for their expansive functionalities. Consider features like ease of use, data integration capabilities, and the ability to generate insights from historical call data.
Once you choose the appropriate tools, utilize them to analyze customer interactions and gather deep insights. For example, NICE Nexidia allows teams to identify patterns in customer behavior, while Google AI can enhance those insights with advanced analytical capabilities. Implementing these tools not only streamlines the forecasting process but also democratizes access to valuable data across your organization. Prioritize the engagement of all team members with these tools to enhance predictive CX forecasting efforts significantly and drive better business decisions moving forward.
- insight7
To truly harness the power of predictive models, organizations must grasp the essence of Predictive CX Forecasting. This approach uses historical data to anticipate customer interactions and experiences in a call center environment. By understanding patterns in customer behavior, organizations can proactively cater to needs before they arise. This not only enhances customer satisfaction but also streamlines operations, leading to improved overall performance.
The journey to effective Predictive CX Forecasting requires the alignment of several components. First, organizations need to ensure robust data collection processes, focusing on quality and relevance. Next, employing the right analytical techniques and algorithms is crucial for translating raw data into meaningful insights. As organizations refine their models, they can predict outcomes with increasing accuracy, ensuring that every customer interaction is a step towards stronger engagement and loyalty. The future of call centers depends on these predictive capabilities to stay ahead in a competitive landscape.
- IBM Watson Analytics
To enhance predictive CX forecasting, one pivotal tool is commonly utilized to transform customer data into actionable insights. It can efficiently analyze large datasets, uncovering patterns and trends in consumer behavior that ultimately inform strategies for improving customer experience outcomes. With its advanced capabilities, businesses can pinpoint positive and negative feedback, enabling targeted improvements in service delivery.
Utilizing this tool streamlines the process of querying data, allowing users to perform a variety of analyses. For instance, organizations can compare insights across locations or timeframes to identify discrepancies in customer experiences. This level of analysis is critical for businesses aiming to refine their call center operations effectively. By systematically extracting and interpreting these insights, companies can anticipate customer needs and enhance satisfaction continuously, paving the way for more informed decision-making in customer experience management.
- NICE Nexidia
NICE Nexidia offers a powerful toolset for organizations aiming to enhance their customer experience (CX) forecasting. By transforming vast amounts of conversational data into actionable insights, it supports businesses in understanding customer needs and expectations. This capability is crucial for predictive CX forecasting, enabling call centers to effectively analyze trends and patterns in customer interactions.
The platform facilitates seamless data transcription and analysis, allowing users to process multiple audio files at once. Through its intuitive interface, users can visualize outcomes and extract relevant insights in just a few clicks. These insights reveal pain points and highlight key moments in customer conversations, empowering organizations to align their strategies with customer sentiment. When integrated into predictive models, NICE Nexidia enhances the accuracy of CX forecasting, ultimately leading to improved customer satisfaction and loyalty.
- Avaya Predictive Analytics
Avaya Predictive Analytics is a powerful solution that empowers organizations to enhance customer experience through data-driven insights. It utilizes sophisticated algorithms to analyze call center data, helping businesses anticipate customer needs and preferences. Predictive CX forecasting enables teams to make informed decisions, streamline operations, and ultimately improve customer satisfaction.
The benefits of using Avaya Predictive Analytics are significant. First, it allows for the identification of patterns in customer interactions, which can inform strategies for personalized engagement. Second, it provides insights into potential risks, enabling proactive measures to address customer concerns before they escalate. Finally, utilizing this technology fosters a culture of continuous improvement, as organizations refine their processes based on actionable data. By leveraging predictive insights, businesses can navigate the complexities of customer interactions and drive positive outcomes in their service delivery.
- Google AI
In the realm of predictive CX forecasting, advanced technologies empower call centers to optimize customer experiences effectively. A significant player in this domain provides powerful AI tools designed to analyze vast amounts of data for insightful predictions. By implementing these tools, organizations can better understand customer behavior and preferences, driving improved outcomes and satisfaction rates.
One notable feature of this AI platform is its ability to process and analyze customer interactions swiftly. Utilizing machine learning algorithms, it helps in identifying trends and patterns in customer data, allowing businesses to tailor their approaches accordingly. Furthermore, the platform fosters continuous improvement by enabling regular updates and refinements based on real-time performance, thus enhancing the accuracy of predictive models and ensuring that insights remain relevant. Through these capabilities, organizations can leverage AI to improve overall decision-making in customer experience management.
Step 3: Analyzing Data and Training Models for Accurate CX Predictions
In Step 3, the focus turns to analyzing data and training models for accurate CX predictions. Data segmentation and preparation are crucial first steps. This involves categorizing datasets to better identify trends and extract meaningful insights. By thoroughly understanding customer feedback and behavior, organizations can highlight patterns that significantly impact customer experience.
Next, training models is essential for predictive CX forecasting. This process involves using historical data to create algorithms that learn and adapt over time. Employing various analytical techniques ensures that these models can accurately anticipate customer needs and preferences. Continuous validation of these models against new data further refines accuracy, allowing businesses to stay ahead in a competitive landscape. By effectively analyzing data and training models, organizations can develop strong predictive capabilities that drive improved customer experiences.
- Data Segmentation and Preparation
Data segmentation and preparation play a pivotal role in effective predictive CX forecasting. It involves organizing call center data into meaningful groups to enhance insights and drive better forecasting outcomes. Segmenting data by key variables—such as location, customer demographics, and call types—allows for a more nuanced analysis of customer interactions. This process helps identify trends and informs strategic decisions for targeted improvements.
To prepare data effectively, the following steps should be considered:
- Identify Key Segments: Begin by determining the most relevant segments, such as geographical areas or customer profiles.
- Data Collection: Gather data across various touchpoints, ensuring comprehensive coverage of customer interactions.
- Clean and Organize Data: Remove inaccuracies and inconsistencies to maintain data integrity.
- Analyze for Insights: Use analytical tools to derive actionable insights from the segmented data.
By following these steps, organizations can enhance their predictive modeling efforts, leading to more accurate Customer Experience forecasts. This holistic approach ultimately empowers businesses to improve customer interactions and outcomes.
- Model Training and Validation
In the process of Model Training and Validation for Predictive CX Forecasting, establishing a robust framework is crucial. First, data collection is of paramount importance. Quality data serves as the foundation for accurate predictions, allowing for meaningful insights into customer behaviors and preferences. Preprocessing this data involves cleaning, normalizing, and segmenting it into relevant categories, facilitating effective training.
Next, model training is focused on using various algorithms to enhance prediction accuracy. This involves running multiple iterations to identify the best performing models. Validation is equally important, as it ensures that the model can generalize to unseen data. This step typically includes techniques like cross-validation to measure how well your model performs across different subsets of the data. Proper training and validation processes ultimately lead to reliable outcomes that support improved decision-making in call centers.
Step 4: Monitoring, Evaluating, and Refining Predictive Models
Monitoring, evaluating, and refining predictive models is crucial for achieving accurate outcomes in predictive CX forecasting. This process ensures that the models consistently align with evolving customer behaviors and preferences. Continuous performance monitoring involves regularly assessing the model’s predictions against actual customer experiences. By examining discrepancies, organizations can identify areas for improvement and respond to emerging trends swiftly.
Iterative model refinement is equally important in this process. As data evolves, models must adapt to maintain accuracy. This may involve updating the data sets or adjusting the algorithms used for forecasting. Engaging in feedback loops with customer service representatives can yield insights to enhance model performance. Ultimately, committing to these practices allows organizations to deliver exceptional customer experiences and make data-driven decisions that benefit the entire call center operation.
- Continuous Performance Monitoring
Continuous performance monitoring is critical for organizations aiming to improve customer experience outcomes through predictive models. Regularly assessing call center performance enables teams to gain valuable insights into customer interactions. This process involves tracking key performance indicators, such as call resolution rates and customer satisfaction scores, to fine-tune modeling strategies effectively.
Establishing a framework for continuous performance monitoring is vital for timely feedback and improvement. Begin by defining specific metrics that align with customer experience goals; regularly collect and analyze call data. Use this information to identify trends or recurring issues. Additionally, create a culture of feedback among customer service representatives, as their insights can unveil hidden challenges. Incorporating feedback loops helps ensure that predictive models remain relevant and effective, leading to enhanced customer satisfaction and loyalty. By prioritizing these practices, organizations can elevate their predictive CX forecasting efforts in call centers.
- Iterative Model Refinement and Improvement
Iterative model refinement and improvement are essential steps in the process of predictive CX forecasting. Once the initial model is built, it must undergo continuous evaluations to ensure its accuracy and relevance. This process starts by consistently monitoring performance metrics, gathering user feedback, and identifying discrepancies between predicted and actual outcomes. By understanding these differences, analysts can pinpoint areas for enhancement, paving the way for more precise customer experience predictions.
There are several key strategies for effective model improvement. First, analyze customer behavior patterns and trends to refine the model; this will provide more context for decision-making. Second, update the data used for training the model regularly. Fresh and relevant data significantly enhances predictive accuracy. Lastly, incorporating machine learning algorithms allows for adaptive learning, enabling models to evolve with changing customer dynamics. This iterative approach fosters a robust predictive framework, ultimately resulting in better customer engagement and satisfaction.
Conclusion: The Future of Predictive CX Forecasting in Call Centers
The future of predictive CX forecasting in call centers promises to transform customer interactions and enhance satisfaction. As more companies embrace data-driven models, the ability to predict customer needs and preferences will become increasingly sophisticated. With advancements in AI and machine learning, organizations can expect to receive actionable insights from customer interactions, allowing for more tailored responses.
This evolution not only streamlines operations but also empowers customer service representatives with crucial information. By anticipating customer questions and issues, call centers can better train their teams and improve service quality. Ultimately, predictive CX forecasting will redefine the customer experience, creating more meaningful connections between businesses and their clients.