How to Extract CX Signals From QA Data Without Bias
-
Hello Insight
- 10 min read
Unbiased CX Extraction in QA Data is essential for deriving accurate insights that foster positive customer experiences. When analyzing quality assurance data, any innate bias can skew results, leading to misguided decisions. Understanding the nuances that contribute to bias is crucial for organizations aiming to harness authentic customer signals. This introduction sets the stage for strategies that effectively counteract bias, ensuring the signals extracted truly reflect user sentiments.
As we delve deeper into the process of unbiased CX extraction, it’s vital to embrace methodologies that promote clarity and trustworthiness in data interpretation. By recognizing and addressing potential biases, teams can elevate the integrity of their findings. This section aims to provide a pathway to achieve reliable insights and optimize your QA processes effectively, thus driving improved customer satisfaction and loyalty.
Analyze & Evaluate Calls. At Scale.
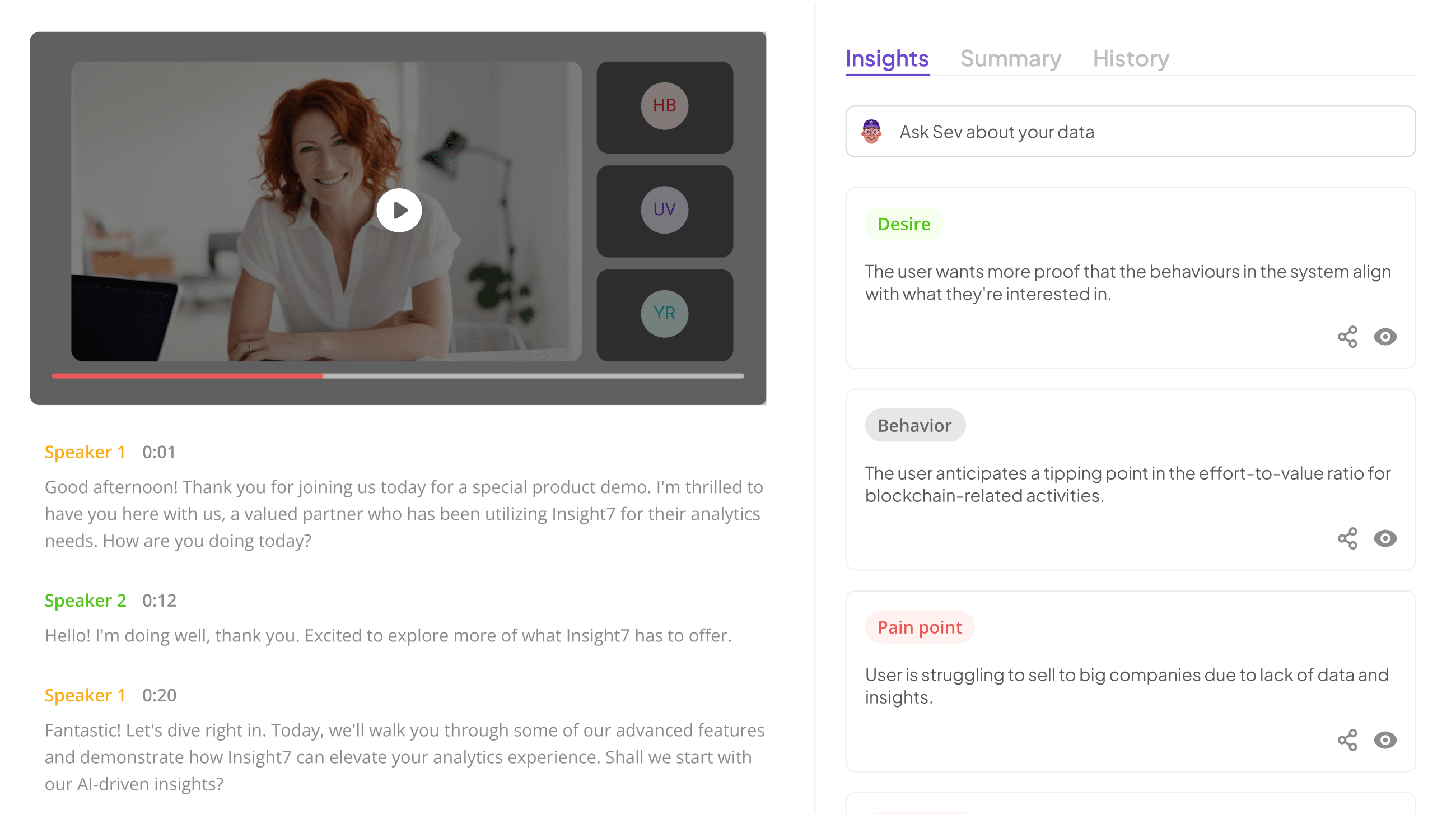
The Importance of Unbiased CX Extraction
Unbiased CX extraction is crucial for accurately interpreting customer experiences from quality assurance (QA) data. When biases infiltrate the extraction process, they distort the insights gained and lead to misguided conclusions. This can result in flawed strategies that fail to address genuine customer needs. Thus, maintaining objectivity ensures that the data accurately reflects the voice of the customer, which is essential for effective decision-making.
To achieve unbiased CX extraction, one must first recognize the common sources of bias in QA processes. These biases can stem from human judgment, inadequate training, or even flawed evaluation criteria. Identifying and addressing these biases allows organizations to extract meaningful insights that can drive improvement. This leads to better customer satisfaction and loyalty, ultimately enhancing organizational performance. Prioritizing unbiased CX extraction not only refines analytics but also fosters a culture of transparency and trust within teams.
Why Bias in QA Data Matters
Bias in QA data can significantly distort customer experience signals, leading to misguided business decisions. When quality assurance processes reflect systematic biases, the resultant insights may not accurately represent customer sentiments. This misalignment can cause teams to overlook critical feedback, ultimately damaging customer relationships and impairing strategic initiatives.
Identifying common sources of bias, such as subjective evaluations or skewed sample selections, is crucial to mitigating their impact. For example, if a QA team unconsciously favors certain demographics, they might neglect valuable signals from underrepresented groups. Such biases can hinder the effectiveness of decision-making and limit the true potential of Unbiased CX Extraction. By embracing unbiased practices in data evaluation, organizations can better reflect consumer needs and expectations, leading to more reliable insights that drive innovation and improve overall customer satisfaction.
- Understanding Bias and Its Impact
Bias in data analysis can significantly distort customer experience (CX) insights. Understanding bias and its impact is crucial for companies seeking to extract unbiased CX insights from QA data. Bias can arise from numerous sources, including subjective interpretations of customer feedback or skewed data sampling. Even slight biases can lead to misleading conclusions, affecting decision-making and ultimately the customer experience.
To mitigate bias, organizations should adopt strategies that promote objectivity. It is essential to recognize the different types of bias that may infiltrate analyses, such as confirmation bias or selection bias. Utilizing diverse teams in data analysis and employing standardized methods can also help ensure a broader perspective. Continuous monitoring for bias during QA processes will further enhance efforts towards unbiased CX extraction, promoting accurate, actionable insights that drive improvement.
- Common Sources of Bias in QA Processes
In quality assurance processes, recognizing common sources of bias is crucial for achieving unbiased CX extraction. One significant source is the selection bias, which occurs when the sample of data reviewed does not represent the entire dataset accurately. This can lead to skewed insights, which misguide decision-making and ultimately impact customer experience negatively. Another source of bias arises from confirmation bias, where evaluators prioritize input that confirms their pre-existing beliefs about the information being evaluated. This can further distort the analysis, preventing a clear understanding of genuine customer sentiments.
Additionally, evaluation criteria can introduce bias if they are not comprehensive or flexible enough to accommodate diverse interactions. Rigid frameworks tend to overlook nuances in customer experiences. Furthermore, evaluator bias, stemming from the individual beliefs and experiences of the QA team, can also distort evaluations. Addressing these sources of bias is key to refining QA processes and ensuring unbiased CX extraction, fostering a more accurate understanding of customer experiences and needs.
Steps to Achieve Unbiased CX Extraction
Achieving unbiased CX extraction begins with identifying potential biases inherent in your QA data. Start by closely examining the criteria utilized for evaluation, ensuring they are comprehensive and not leaning towards specific outcomes. Techniques such as random sampling can help broaden your perspective and uncover hidden biases that may skew results. Analyzing trends within the data can also reveal triggers, allowing for adjustments to prevent bias from influencing performance assessments.
The next step involves implementing bias-reduction techniques to maintain objectivity. Strategies might include training evaluators to recognize their own biases or using software tools that provide standardized feedback. Continuous monitoring is essential; regularly assess the data to pinpoint any emergent biases and adjust your approach as necessary. By following these steps diligently, organizations can work towards truly unbiased CX extraction, encouraging a culture of fairness and transparency in evaluating customer experiences.
Step 1: Identifying Bias in Your Data
To ensure unbiased CX extraction, the first step is identifying bias in your data. Bias can manifest in various forms, subtly affecting how customer feedback is interpreted. For example, preconceived notions or framing effects can alter perceptions about what constitutes valuable feedback. Understanding these biases is crucial, as they can skew insights derived from QA data, leading to misguided conclusions.
Next, examine your QA processes for potential bias triggers. Analyze the selection criteria for feedback samples, as non-representative data can lead to inaccurate insights. Consider both qualitative and quantitative biases—such as demographic skews or particular keyword prevalence in customer comments. Employing diverse analytical techniques can reveal hidden biases, fostering a more reliable foundation for extracting customer experience (CX) signals. By actively identifying and addressing these biases, you lay the groundwork for a more accurate and trustworthy CX extraction process.
- Techniques for Detecting Bias
Detecting bias in QA data is crucial for ensuring unbiased CX extraction. To effectively identify bias, one can employ various techniques that scrutinize data sources and analysis methods. Start by examining data collection processes, ensuring diverse representation. This involves assessing demographic trends in your dataset to spot any skewed patterns. For instance, if feedback predominantly comes from a specific customer segment, the insights derived could be misleading.
Next, consider the language used in the data. Using sentiment analysis tools can help reveal biased language, which might distort perceptions of customer experience. Regular audits of the quality assurance team’s subjective interpretations are also vital. These evaluations can highlight inconsistencies and unconscious biases. Lastly, implementing controls, such as standardized scoring rubrics, can further aid in maintaining impartiality. By remaining vigilant in detecting these biases, you contribute to a more accurate and reliable understanding of customer feedback, ultimately supporting unbiased CX extraction.
- Analyzing QA Data for Bias Triggers
Analyzing QA Data for Bias Triggers is crucial to achieving Unbiased CX Extraction. The first step involves examining the data set for any signs of bias, which can significantly distort customer experience insights. Review recordings and transcriptions critically, looking for patterns that may reflect biased language or interpretative tendencies. Analyzing comments and sentiments expressed can help identify both positive and negative feedback, which is vital for an impartial understanding of customer needs.
Next, focus on triggers that may influence responses or shape outcomes, such as leading questions or biased sampling methods. Cross-reference findings against broader datasets to determine if specific biases impact overall customer experience. Establishing a robust framework for regularly analyzing these triggers will not only aid in achieving unbiased insights but also enhance the reliability of your QA process. By continually refining your methods, you can ensure that customer experience signals remain clear and actionable.
Step 2: Implementing Bias-Reduction Techniques
Implementing bias-reduction techniques is crucial for ensuring the accuracy of CX signals in QA data. To effectively achieve unbiased CX extraction, you can adopt several strategies tailored to mitigate the influence of bias on your findings. One effective technique involves employing a diverse team to analyze the data. This diversity can bring varying perspectives that help identify potential biases in interpretation.
Next, utilize standardized metrics that everyone on the team can follow. Consistency in evaluating data helps to minimize subjective interpretations and ensures that insights drawn are based on objective criteria rather than personal opinions. Additionally, it is essential to continuously monitor how data is collected and analyzed, adjusting methods as needed. By regularly assessing your techniques, you can proactively address any emerging biases, paving the way for more reliable insights that truly reflect customer experiences.
In summary, implementing bias-reduction techniques involves creating diverse teams, standardizing metrics, and regularly monitoring processes. These measures collectively enhance your capability for unbiased CX extraction, enriching your understanding of customer insights.
- Strategies for Neutralizing Bias
To neutralize bias in the extraction of customer experience (CX) signals from QA data, it is crucial to adopt systematic strategies. First, establish standardized criteria for evaluating feedback to ensure consistency. Utilizing tools that standardize responses will help minimize subjective interpretations. Additionally, implement diverse teams when analyzing data as varied perspectives can identify biases that may otherwise go unnoticed.
Another effective strategy involves continuous monitoring and iteration of your analysis processes. Collect feedback from analysts to assess if biases persist and adjust methods accordingly. Creating an open environment for discussing biases can facilitate deeper insights and foster accountability. Finally, consider employing AI-driven solutions that help in analyzing large datasets objectively. These advanced technologies can filter out emotional responses and focus on actionable data, greatly contributing to unbiased CX extraction.
- Continuous Monitoring and Adjustment
Continuous monitoring and adjustment play a pivotal role in achieving unbiased CX extraction from QA data. By consistently evaluating performance, teams can identify shifts in patterns, ensuring that customer experience signals remain accurate and relevant. This ongoing process allows organizations to adapt their strategies as necessary, emphasizing a commitment to quality and transparency.
To ensure effectiveness, consider three key actions:
Regular Data Reviews: Schedule consistent reviews to analyze QA data, highlighting trends and anomalies. This step helps in recognizing any emerging biases promptly.
Adjust Criteria Dynamically: As customer expectations evolve, adjust your assessment criteria to reflect current realities. This flexibility keeps the evaluation process aligned with the actual customer experience.
Training and Feedback: Establish continuous training sessions based on the insights gathered. Regular feedback loops will empower teams to enhance their skills and understand the nuances of unbiased CX extraction.
By fostering an adaptable environment, businesses can significantly improve their ability to extract accurate CX signals and respond effectively to customer needs.
Tools for Unbiased CX Extraction
Unbiased CX extraction relies on the right tools to ensure the fair interpretation of customer experience signals from QA data. First, leveraging robust analytics platforms, like Qualtrics or SurveyMonkey, can streamline the identification of insights while minimizing bias. These tools enable organizations to accumulate feedback in a structured manner, ensuring a more accurate reflection of customer sentiments.
Additionally, incorporating advanced features like weight adjustments in scoring can mitigate subjective influence. This method allows for the prioritization of criteria, helping evaluators focus on the most impactful aspects of customer interactions. Regularly updating and refining these criteria based on ongoing learnings keeps the analysis relevant and precise. By utilizing these tools effectively, organizations can foster a culture of unbiased CX extraction, providing reliable insights that drive decision-making and enhance customer experience.
Extract insights from interviews, calls, surveys and reviews for insights in minutes
Insight7
The process of Unbiased CX Extraction involves transforming raw QA data into actionable insights without the influence of preconceived notions or biases. In today's fast-paced environment, companies are flooded with customer signals from various sources, but efficiently analyzing this data proves to be a challenge. By focusing on extracting what truly matters from customer interactions, organizations can better understand their clients' needs and preferences.
To achieve unbiased outcomes, begin by recognizing potential biases present in the data. Techniques such as comparative analysis and feedback triangulation can help identify these biases. Once they are pinpointed, implementing effective strategies becomes crucial. This includes the use of standardized metrics and tools designed to minimize subjectivity during data interpretation. Finally, continuous monitoring ensures that insights remain relevant and actionable, allowing businesses to adapt swiftly as customer needs evolve. Ultimately, a commitment to unbiased CX extraction will not only enhance customer satisfaction but also drive long-term success.
Additional QA Analytics Tools
Additional QA analytics tools play a crucial role in achieving unbiased CX extraction from your data. These tools enhance your ability to gather, analyze, and interpret customer insights more effectively. By leveraging specialized software, organizations can identify patterns and trends within customer feedback without the interference of personal biases. This is essential for maintaining objectivity throughout your analysis processes.
A few notable tools include Qualtrics, SurveyMonkey, UserTesting, and Medallia. Each of these platforms offers unique features designed to streamline data collection and provide actionable insights. For example, Qualtrics stands out with its ability to create detailed surveys tailored to specific needs, while SurveyMonkey simplifies the feedback process for both consumers and organizations. UserTesting delivers invaluable qualitative insights through real customer interactions, and Medallia allows for real-time data analysis, fostering timely decision-making. Utilizing these additional QA analytics tools empowers teams to perform unbiased CX extraction, ultimately leading to a more accurate understanding of customer experiences.
- Qualtrics
Qualtrics serves as a powerful tool in extracting customer experience signals from QA data without bias. This platform facilitates rigorous data analysis by offering robust features that transcribe and assess interaction calls. By generating insights from these conversations, organizations can move from a transactional approach to a more consultative engagement with customers.
To ensure unbiased CX extraction, Qualtrics provides valuable analytics that helps identify patterns and trends. With various capabilities, the tool assists in comprehensively analyzing both individual and grouped interactions. This not only improves understanding of customer sentiments but also refines training for personnel. Organizations can thus remain adaptable and aligned with real-time market demands, ultimately driving better outcomes. Adopting such a data-driven approach enables more accurate insights into customer experiences and fosters a continual cycle of improvement.
- SurveyMonkey
Survey tools play a pivotal role in the process of unbiased CX extraction from Quality Assurance (QA) data. When it comes to collecting insights, utilizing the right survey platform can significantly enhance data quality. By implementing structured surveys, organizations can gather feedback directly from customers, ensuring that a wide range of perspectives is included. This diversity in feedback minimizes the risk of bias, providing a more holistic view of customer experiences.
Survey tools enable organizations to analyze both qualitative and quantitative data efficiently. They help highlight trends and issues within QA processes, allowing for meaningful adjustments that cater to customer needs. To further enhance unbiased CX extraction, continuous evaluation of survey designs and question formats is essential. By refining survey methodologies over time, organizations can foster a culture of transparency and responsiveness, ultimately leading to improved customer experiences.
- UserTesting
UserTesting serves as a vital tool in the quest for unbiased CX extraction. It provides actionable insights by simulating real user experiences, which helps organizations grasp how customers interact with products or services. This platform allows teams to gather feedback directly from users, ensuring that the data collected reflects genuine customer sentiments.
To achieve unbiased CX extraction, it’s important to focus on the integrity of test conditions. Keeping variables consistent across sessions allows for clearer comparisons and more reliable feedback. Moreover, UserTesting facilitates the identification of trends and patterns in user behavior, enabling teams to prioritize improvements based on actual user experiences. By emphasizing diverse perspectives in testing sessions, organizations can avoid skewed results and better understand their customer base. Engaging with real customers not only enriches data quality but also lays the foundation for continuous, customer-driven enhancements.
- Medallia
Incorporating effective tools is crucial for achieving unbiased CX extraction, especially when dealing with QA data. One prominent option is designed to help organizations analyze customer interactions and feedback systematically. By providing advanced analytics and reporting features, it allows businesses to uncover valuable insights from their customer communications without the interference of bias.
Effective utilization of such a tool enables organizations to focus on key aspects of customer experiences. This encompasses assessing the tone, responsiveness, and overall sentiment captured in quality assurance processes. Furthermore, it can facilitate the identification of areas that require improvement while maintaining a neutral perspective, ensuring that data-driven decisions are based on objective findings rather than subjective interpretations. This approach contributes significantly to fostering a customer-centric culture and enhancing overall satisfaction.
Conclusion: Ensuring Unbiased CX Extraction in Your Organization
Ensuring unbiased CX extraction within your organization requires a commitment to transparency and awareness of potential biases. By implementing structured processes, teams can identify and address bias in their QA data. This approach not only enhances the reliability of customer insights but also promotes a culture of inclusivity that values diverse perspectives.
To achieve this goal, organizations should adopt clear protocols for data analysis. By regularly reviewing methods and outcomes, teams can continually refine their practices, ensuring they remain objective. Prioritizing unbiased CX extraction will empower your team to derive accurate insights that truly reflect customer experiences, ultimately leading to better decision-making and improved outcomes.
Analyze & Evaluate Calls. At Scale.
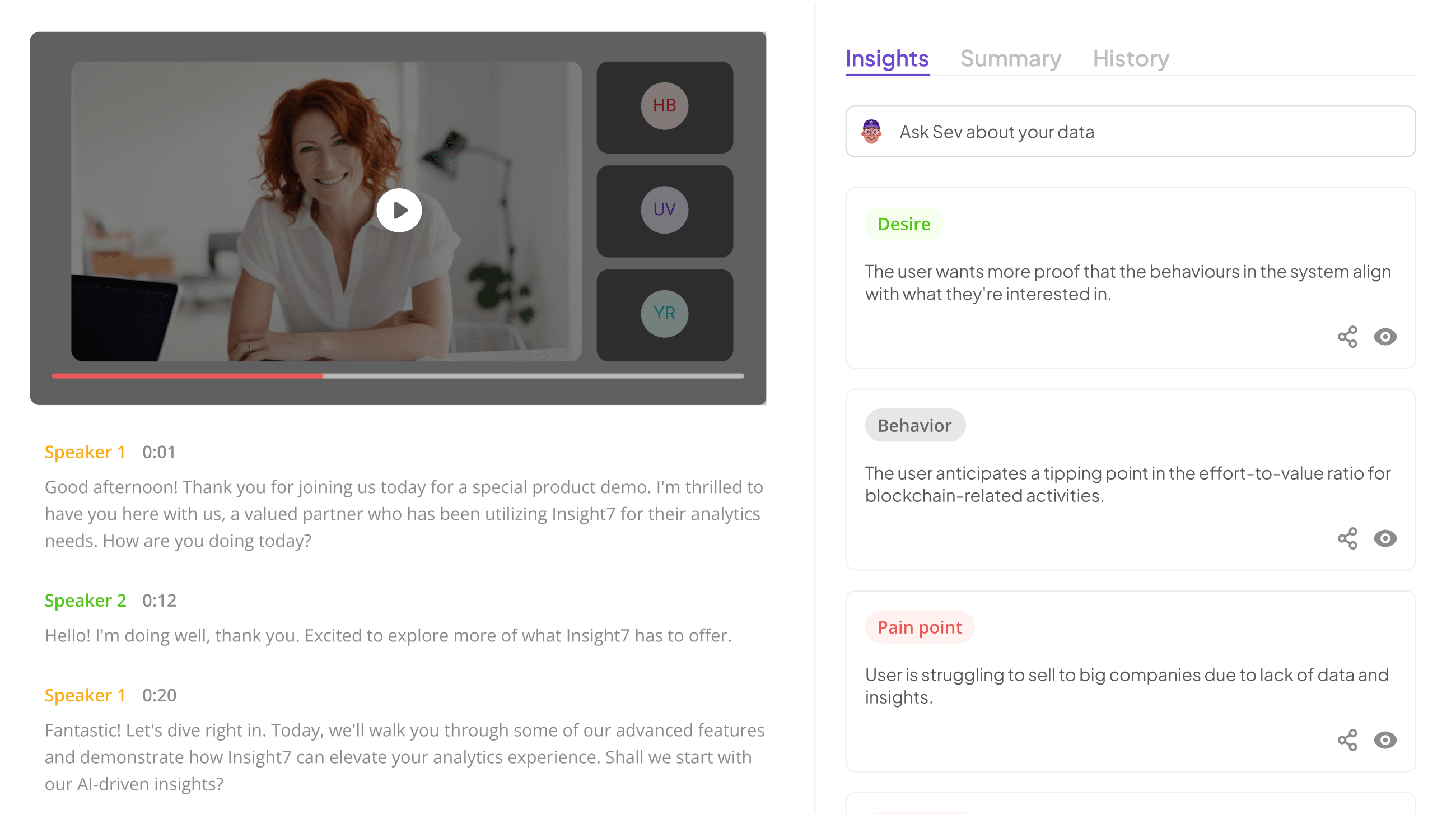