Text analytics has evolved into a vital tool for extracting meaningful insights from vast amounts of textual data. The advent of technology has made it easier to analyze conversations at scale, allowing businesses to harness customer feedback and refine their strategies effectively. In this Text Analytics Guide, we aim to illuminate the key processes involved in transforming raw text into actionable insights.
Understanding how to leverage text analytics can enhance decision-making and provide a competitive edge. The following sections will detail the systematic approach needed to navigate the complexities of text analysis. As we explore each step, you'll learn how to collect data, preprocess it, extract significant information, and ultimately apply these insights to improve your business outcomes.
Generate visualizations from your qualitative data. At Scale.
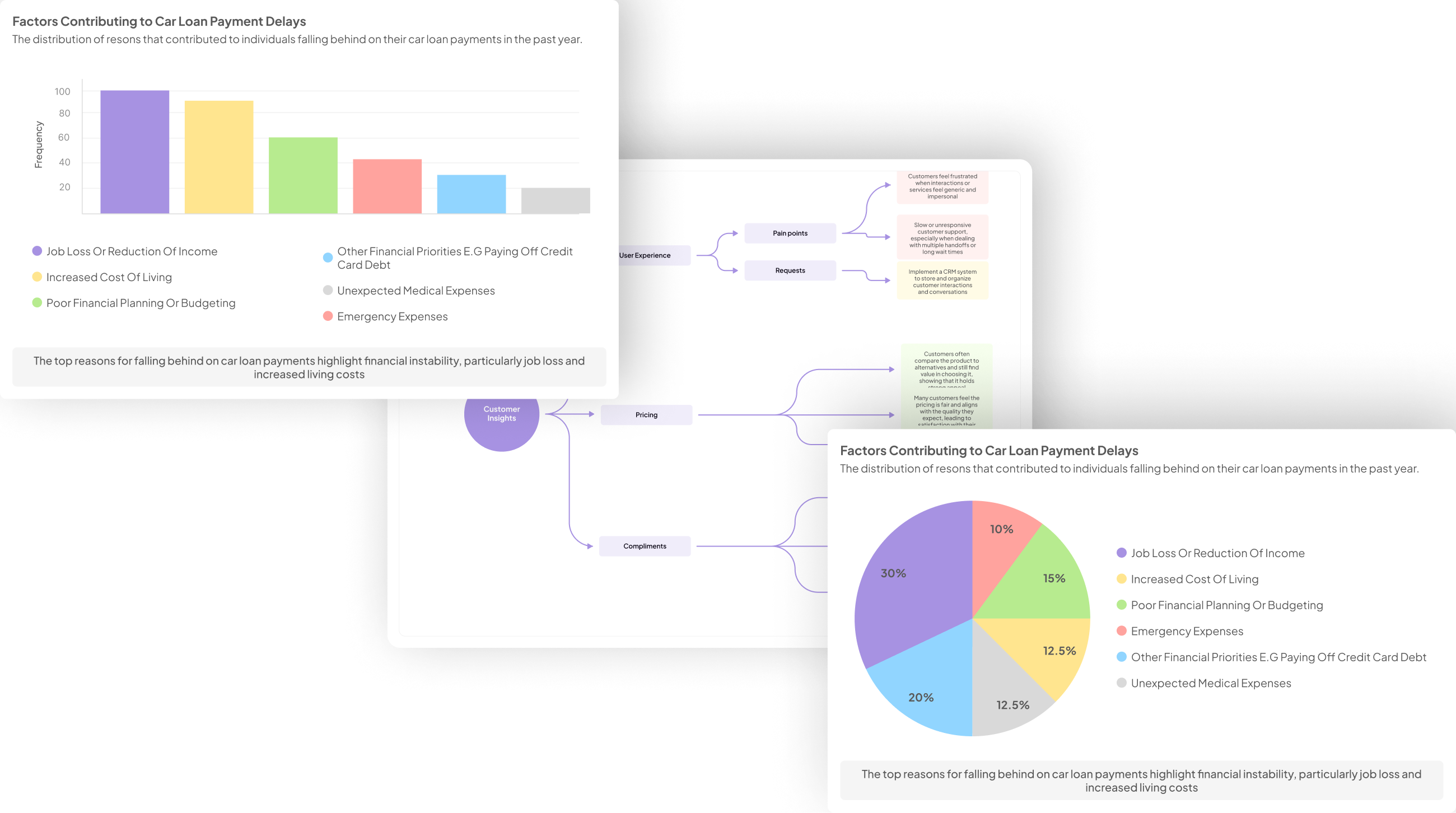
Understanding the Basics of Text Analytics Guide
Text analytics is a powerful method that transforms unstructured text into valuable insights. Understanding the basics of a Text Analytics Guide helps demystify this fascinating field, ensuring you can effectively harness its potential. To get started, it's essential to grasp fundamental concepts such as tokenization, stop words, and stemming. Each of these components plays a critical role in preparing raw text for analysis, making information more manageable and meaningful.
As you venture deeper into text analytics, recognizing its various applications becomes crucial. Text analytics can uncover customer sentiments, identify emerging trends, and enhance decision-making processes. Moreover, having a solid foundation allows you to efficiently navigate more advanced techniques like sentiment analysis and topic modeling. By following a well-structured Text Analytics Guide, you'll not only gain knowledge but also empower yourself to make data-driven decisions enriched by actionable insights.
Key Concepts in Text Analytics Guide
Key concepts in text analytics form the foundation for conducting effective analysis and deriving valuable insights from large volumes of text data. Understanding these fundamental aspects empowers analysts to navigate the complexities of text analytics more efficiently. Key principles include data preprocessing, which ensures the quality of the raw text before analysis, and feature extraction, which identifies relevant attributes for modeling.
Additionally, understanding natural language processing (NLP) allows for sophisticated text analysis techniques, such as sentiment analysis and topic modeling. These tools enable users to discern patterns, themes, and emotions expressed in the data. Furthermore, the role of visualization cannot be overlooked, as visual representations help in communicating insights clearly. By grasping these concepts, users can better engage with their text analytics guide, transforming raw data into actionable intelligence that can drive informed decision-making.
Importance and Applications of Text Analytics Guide
Text analytics plays a crucial role in today’s data-driven environment. The importance of a comprehensive Text Analytics Guide cannot be overstated, especially for organizations seeking actionable insights. By analyzing large volumes of text data, businesses can uncover trends and patterns that inform decision-making. This process enables companies to better understand customer sentiment, improve product offerings, and enhance overall user experience.
There are several applications of text analytics that showcase its versatility. Firstly, it aids in market research by sifting through customer feedback and reviews. Secondly, it can optimize customer support by identifying frequently asked questions and resolving issues swiftly. Finally, text analytics supports content strategy by revealing which topics resonate best with audiences. By utilizing the guidance offered in a Text Analytics Guide, organizations can harness the power of their text data for practical benefits, ultimately driving growth and innovation.
Generate Journey maps, Mind maps, Bar charts and more from your data in Minutes
Step-by-Step Text Analytics Guide
A thorough Step-by-Step Text Analytics Guide can transform how organizations interpret and utilize textual data. First, begin with data collection and preprocessing, as these foundational steps ensure accuracy and relevance. Gather disparate forms of text, such as transcriptions or survey responses, and clean the data for analysis. This process reduces noise and enhances the quality of insights derived later.
Next, focus on text extraction and analysis. Utilize software tools to extract meaningful patterns or themes from the text. Employ techniques like sentiment analysis to understand underlying emotions or trends. By systematically following these steps, anyone can efficiently derive actionable insights from textual data. This structured approach not only aids in decision-making but also enhances customer engagement and satisfaction through informed strategies.
Step 1: Data Collection and Preprocessing
Data collection and preprocessing are crucial first steps in any text analytics project. This stage involves gathering the raw text data from various sources, like social media, customer feedback, or surveys. It’s essential to ensure that the collected data is both relevant and representative of the subject matter you wish to analyze. Preprocessing then refines this raw data, which can include normalizing text, removing stop words, and correcting typos. Effective preprocessing enhances the quality of data, ensuring that the resulting insights are accurate and meaningful.
Moreover, during this step, it is important to address any biases in data collection. Using diverse sources can help mitigate this risk and foster comprehensive analysis later on. Additionally, organizing the data into a structured format is necessary for subsequent analytical processes. Following these steps prepares a solid foundation for deeper exploration and understanding in your text analytics journey.
Step 2: Text Extraction and Analysis
Text extraction is the gateway to uncovering valuable insights from unstructured data. During this phase, it’s crucial to convert raw textual information, such as transcripts from audio files, into structured data. This often involves using transcription software to convert spoken words into text format. Once you have the transcribed data, the focus shifts to analysis, where you can identify patterns, sentiments, and key themes. The goal is to derive actionable insights that can inform business decisions.
In-depth analysis comes next, allowing you to categorize and quantify insights effectively. You may choose to highlight pain points, extract critical keywords, or summarize key conversations. Using templates for specific analytical purposes can streamline this process and enable you to focus on what matters most. Overall, this step is crucial in the Text Analytics Guide as it sets the foundation for making informed decisions based on the data collected.
Conclusion of Text Analytics Guide
In conclusion, the Text Analytics Guide equips you with the necessary knowledge to derive valuable insights from vast amounts of text data. By following each step outlined in this guide, you can confidently analyze conversations and other textual information to make data-driven decisions.
This journey through text analytics is about understanding and simplifying complex data. As you implement these methods, remember that thorough analysis transforms raw data into actionable insights, ultimately enhancing your decision-making processes and contributing to business success. Embrace these techniques, and you'll unlock new opportunities for growth and understanding.