How to Detect Call Pattern Anomalies in High-Volume Centers
-
Bella Williams
- 10 min read
Call anomaly detection serves as a crucial component for maintaining operational efficiency in high-volume centers. As call traffic surges, identifying deviations from established patterns becomes vital for ensuring quality control. By scrutinizing call metrics, organizations can uncover discrepancies that may indicate underlying issues, such as poor customer service or inefficient processes.
In this introduction, we will explore the importance of call anomaly detection in high-volume centers. Understanding what constitutes a call anomaly will provide a solid foundation for establishing effective monitoring strategies. By leveraging data-driven insights, organizations can enhance their service quality and ultimately foster customer satisfaction in a competitive landscape.
Analyze & Evaluate Calls. At Scale.
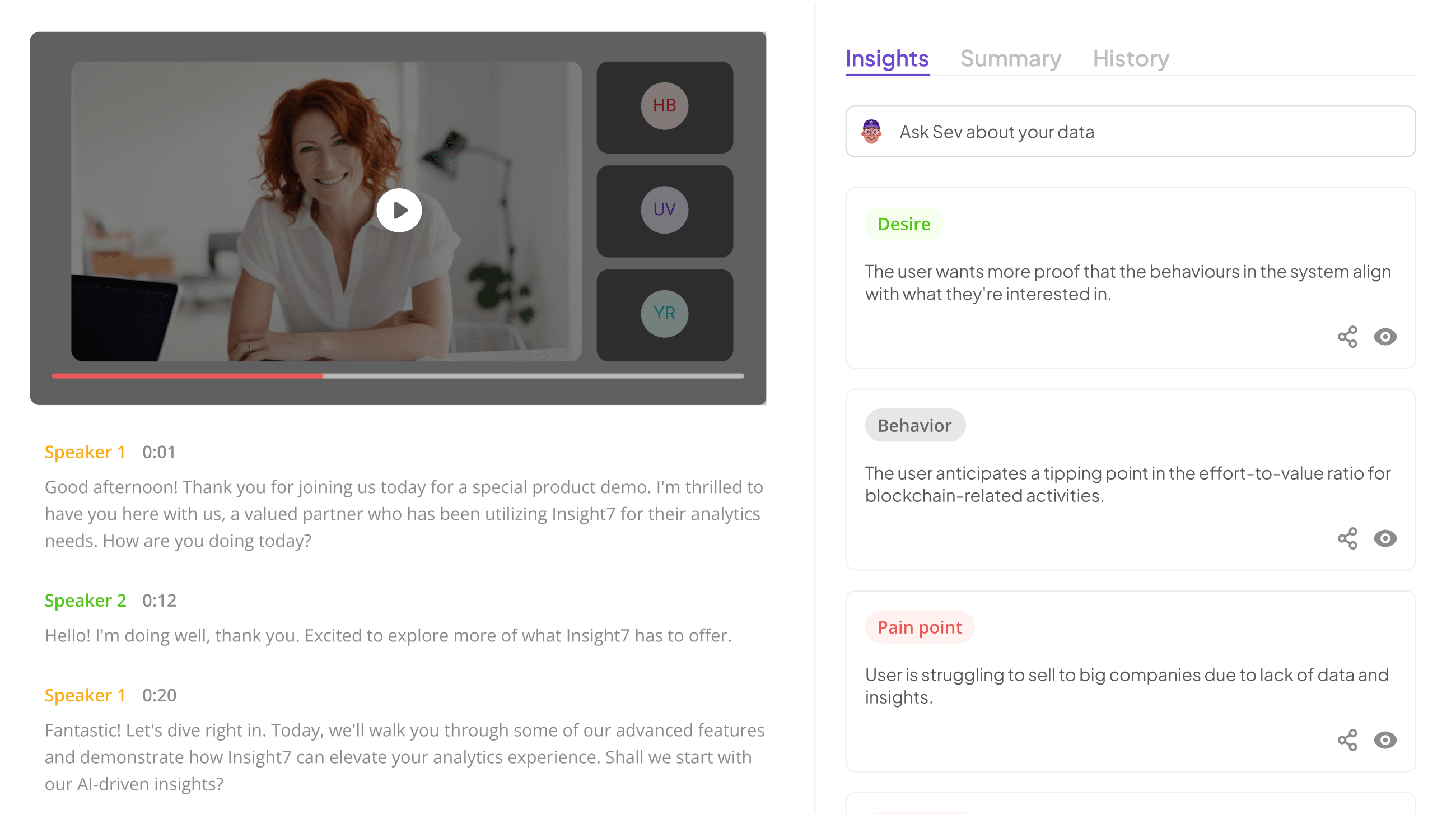
Understanding Call Anomaly Detection
Understanding Call Anomaly Detection involves recognizing deviations in call patterns that may indicate issues such as compliance failures or operational inefficiencies. In high-volume call centers, where vast amounts of data are generated daily, accurately identifying these anomalies becomes crucial. Call anomaly detection systems analyze conversations to pick up on unusual patterns, comparing them against established benchmarks. This ongoing monitoring allows teams to react swiftly to potential problems.
To effectively implement call anomaly detection, one must consider several key factors. First, clearly define what constitutes a 'normal' call pattern to establish a reliable baseline. Next, utilize advanced analytics and machine learning algorithms to sift through large volumes of data. By flagging outlier calls for further review, organizations can ensure quality and compliance. Ultimately, a robust understanding of call anomaly detection empowers teams to enhance performance, training, and customer satisfaction.
What Constitutes a Call Anomaly?
Call anomalies refer to irregular patterns within call data that deviate from expected behaviors. These deviations can manifest in various ways, including abnormal call volumes, unexpected spikes or drops in call duration, and shifts in call timing. Identifying these anomalies is crucial for centers handling a high volume of calls, as they can signify underlying issues ranging from staffing problems to customer dissatisfaction.
A call anomaly may also involve unusual discussions or topics that arise within conversations, which could indicate either emerging customer concerns or risks of non-compliance with established guidelines. Effective call anomaly detection systems utilize both historical data and real-time analytics to flag these irregularities. By pinpointing these anomalies, organizations can improve training programs, enhance customer engagement, and ensure compliance, ultimately leading to better operational efficiency.
Common Call Patterns in High-Volume Centers
In high-volume centers, understanding common call patterns is essential for effective communication and service delivery. Call dynamics often follow predictable trends, allowing teams to anticipate customer needs and streamline processes. Analyzing call duration and frequency reveals much about customer interactions. For instance, short calls may indicate simple inquiries, while longer conversations often signify more complex issues that require resolution.
Identifying these patterns also aids in detecting anomalies that may indicate problems, such as unusual spikes in call volumes or changes in call duration. By monitoring these trends, organizations can implement timely interventions to enhance customer experience. Additionally, tracking average resolution times can pinpoint areas where staff might need further training or support. Understanding these common patterns is pivotal in efficiently managing resources and ensuring satisfaction, ultimately contributing to improved performance in call anomaly detection efforts.
Steps to Implement Effective Call Anomaly Detection
To effectively implement call anomaly detection in high-volume centers, itโs essential to start with thorough data collection and preprocessing. This involves gathering call data over a significant period to assess typical patterns and identify deviations. Clean and organize this data to ensure itโs suitable for analysis, removing any irrelevant or duplicate information. A well-structured dataset provides a strong foundation for the next steps.
Next, utilize machine learning algorithms tailored for anomaly detection. These algorithms can automatically analyze the data and flag calls that exhibit unusual patterns or behaviors. Techniques such as clustering and classification can help identify underlying trends, allowing you to gain insights into agent performance and customer interactions. By continuously refining the algorithms based on real-time data, you enhance the system's ability to detect anomalies.
Establishing regular reviews and updates of the detection system is crucial for maintaining its effectiveness. Implementing these steps will lead to a more efficient call management system, ultimately improving compliance and customer satisfaction.
Step 1: Data Collection and Preprocessing
In the initial stage of Call Anomaly Detection, data collection and preprocessing are vital for effective analysis. First, gather a comprehensive dataset that includes call records, customer interactions, and agent performance metrics. This data serves as the foundation for identifying unusual patterns that may indicate anomalies. It's crucial to ensure that the dataset is not only extensive but also relevant to the specific patterns you aim to detect.
Next, the preprocessing step involves cleaning and organizing this raw data. This might include removing duplicates, handling missing values, and standardizing data formats. By structuring the data effectively, anomalies can be identified more accurately. Additionally, segmenting the data based on timeframes or call types can provide valuable insights during further analysis. Thus, this step lays the groundwork for successfully detecting call patterns that diverge from the norm in high-volume centers.
Step 2: Utilizing Machine Learning Algorithms
Machine learning algorithms play a crucial role in enhancing call anomaly detection. They allow for the analysis of vast amounts of call data, revealing patterns that might indicate irregularities. By utilizing supervised and unsupervised learning techniques, one can train models to identify call behaviors that diverge from the norm. This process involves not only detecting anomalies but also understanding the context of these anomalies within high-volume centers.
To achieve effective call anomaly detection, follow these steps:
Data Preparation: First, ensure that your call data is clean and structured. This involves removing irrelevant information and handling missing values.
Feature Engineering: Next, derive features from the raw call data that are indicative of potential anomalies. This could include call duration, frequency, agent performance metrics, and more.
๐ฌ Questions about How to Detect Call Pattern Anomalies in High-Volume Centers?
Our team typically responds within minutes
Model Selection: Choose appropriate machine learning algorithms, such as clustering methods for unsupervised learning or decision trees for supervised learning, to detect anomalies effectively.
Model Training and Evaluation: Train your model on historical call data and evaluate its performance using appropriate metrics like precision, recall, and F1 score.
Continuous Monitoring and Improvement: Finally, regularly update the model with new data to ensure it adapts to evolving call patterns, thereby maintaining the accuracy of anomaly detection.
Extract insights from interviews, calls, surveys and reviews for insights in minutes
Tools for Call Anomaly Detection
To successfully implement call anomaly detection, it is vital to leverage effective tools designed to analyze high volumes of call data. These tools empower users to sift through extensive call recordings and identify patterns that deviate from the norm. For instance, Insight7 offers a powerful platform with capabilities to analyze call trends, facilitating compliance and quality assurance. Users can utilize advanced filtering features to focus on significant calls, ensuring resources are directed where they matter most.
Another noteworthy tool in this domain is CallMiner. This software specializes in deep learning and natural language processing, which helps detect compliance issues and training gaps. Tools like Nexidia and NICE inContact offer detailed analytics dashboards, providing real-time insights and reporting features. By integrating such tools, high-volume centers can enhance their ability to detect call anomalies, ultimately leading to improved operational efficiency and regulatory compliance.
insight7
In the realm of call anomaly detection, identifying unusual patterns is crucial for maintaining efficiency in high-volume centers. Understanding the various aspects of call activities enables teams to pinpoint anomalies effectively. First, itโs important to establish a clear baseline of typical call patterns, which helps in recognizing deviations. These deviations may manifest as an unexpected surge in call volume or abnormal call durations, prompting a deeper investigation.
Next, data analysis plays a pivotal role in elucidating the reasons behind these anomalies. By employing algorithms that can analyze large datasets, centers can uncover underlying issues that may contribute to these abnormalities. The implementation of machine learning models enhances the detection process, ensuring that high-volume centers can respond swiftly to anomalies, leading to improved outcomes. Thus, proactive call anomaly detection not only streamlines operations but also provides the insights necessary to enhance customer service and operational efficiency.
Tool 2: CallMiner
CallMiner serves as a powerful tool in the realm of call anomaly detection, offering various features designed for high-volume centers. By analyzing vast amounts of call data, it helps organizations identify unusual patterns that might indicate compliance issues or sales inconsistencies. Users can sift through extensive recordings quickly and effectively, focusing on calls that exceed a certain duration or contain specific keywords essential for regulatory standards.
One key feature is its ability to segment calls based on various parameters, enabling tailored analysis to meet specific compliance requirements. Additionally, it offers insightful dashboards that display trends and performance metrics to highlight discrepancies efficiently. By utilizing CallMiner, organizations can better understand their call patterns, ensuring both operational efficiency and adherence to compliance rules. This ultimately fosters a culture of continuous improvement in high-volume environments, allowing teams to address areas of concern swiftly and maintain regulatory compliance.
Tool 3: Nexidia
With the increasing complexity of customer interactions in high-volume centers, effective call anomaly detection is essential. Nexidia serves as a vital tool for analyzing conversations at scale, making it easier for teams to gain actionable insights. Users can effortlessly upload call recordings, and the tool automates the transcription process, allowing for the efficient handling of multiple files simultaneously.
Once the calls are transcribed, the platform provides a structured library for sorting and analyzing the data. Users can extract insights by utilizing predefined templates designed for specific use cases, such as understanding customer sentiment or identifying pain points. The intuitive interface facilitates quick queries, summarizing key aspects of calls and highlighting critical information. By leveraging Nexidia, organizations enhance their ability to detect anomalies in call patterns, ultimately improving customer experience and operational efficiency.
Tool 4: NICE inContact
NICE inContact serves as a robust solution for identifying unexpected trends and discrepancies in call data. By utilizing advanced analytics, this tool helps teams monitor and review high-volume call patterns effectively. The platform not only enables users to pinpoint anomalies but also assists in understanding the underlying reasons for these changes, thereby transforming raw data into actionable insights for business improvement.
One of the key features of NICE inContact is its ability to automate the analysis process, allowing teams to focus more on strategic actions rather than getting bogged down with manual reviews. This adaptive approach to Call Anomaly Detection enhances operational efficiency, enabling customer service teams to react promptly to emerging issues. Furthermore, by offering a centralized view of call conversations, it simplifies collaboration and ensures that insights are readily accessible to all relevant stakeholders.
Tool 5: Avaya
In high-volume centers, effectively monitoring call activity is crucial for ensuring compliance and improving operational efficiency. Tool 5: Avaya provides comprehensive solutions for Call Anomaly Detection. By analyzing recorded conversations, this system allows for the identification of patterns indicative of anomalies, such as unusual call durations or fluctuating call volumes. This analytical power aids businesses in maintaining quality standards and compliance with relevant regulations.
To fully utilize Avayaโs capabilities, consider the following approaches. First, ensure all calls are recorded consistently for a comprehensive dataset. Next, implement analytics that sift through these recordings to extract actionable insights. This enables you to highlight areas needing improvement without wading through hours of mundane dialogue. Lastly, use these insights to train employees effectively, enhancing overall service quality while ensuring adherence to compliance standards. By proactively monitoring call patterns, you can achieve greater clarity in performance management.
Conclusion: Emphasizing the Importance of Call Anomaly Detection
Call anomaly detection plays a crucial role in maintaining the quality and efficiency of interactions in high-volume centers. By identifying unexpected variations in call patterns, organizations can quickly address issues that may compromise customer satisfaction and service quality. Proactive monitoring of these anomalies not only highlights potential compliance failures but also points to areas for improving training and support for staff.
In conclusion, investing in effective call anomaly detection systems is essential for any high-volume center. These systems empower organizations to maintain consistent performance, ensure regulatory compliance, and enhance overall customer experience. As customer expectations rise, the ability to swiftly recognize and act on anomalies will ultimately set leading organizations apart from their competitors.
๐ฌ Questions about How to Detect Call Pattern Anomalies in High-Volume Centers?
Our team typically responds within minutes