Speech Burnout Detection is becoming an essential tool in understanding emotional well-being through vocal indicators. In various settings, stress and fatigue manifest not only in behavior but also in the subtle nuances of how individuals communicate. By recognizing shifts in tone, pitch, and pace, we can identify signs of burnout that might otherwise go unnoticed.
The approach combines advanced acoustic analysis with artificial intelligence, allowing for an in-depth examination of speech patterns. This synergy aids in early detection, empowering organizations to provide timely support to individuals in need. Understanding these acoustic signals can significantly enhance workplace environments and lead to healthier, more productive interactions.
Analyze qualitative data. At Scale.
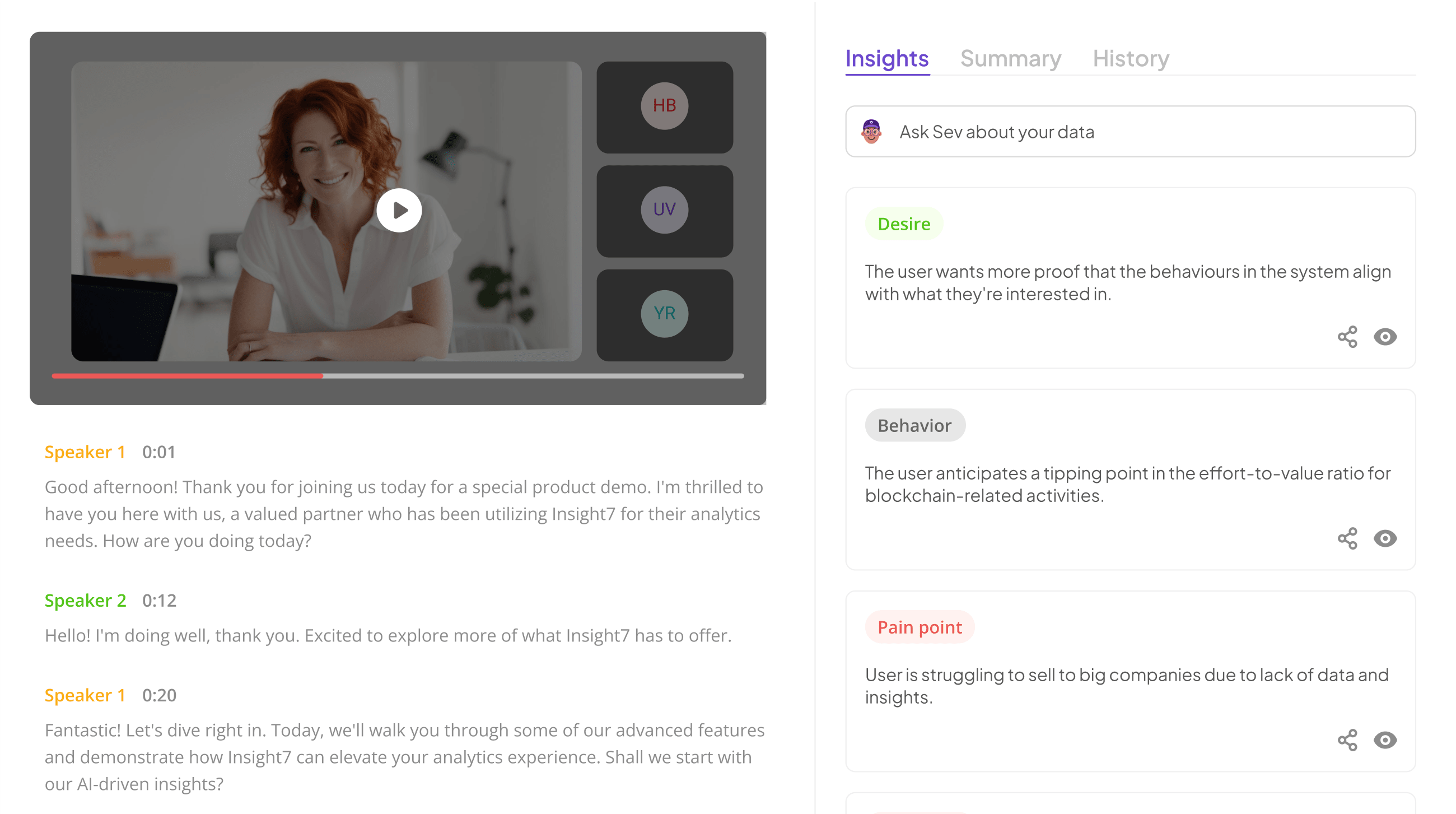
Understanding Speech Burnout Detection
Understanding Speech Burnout Detection involves recognizing how vocal characteristics can indicate a person's mental and emotional state. Burnout can manifest through changes in pitch, tone, and speech patterns, making acoustic analysis a vital tool in identifying it. The focus here is on the various acoustic signals that practitioners can monitor, as each signal reveals different aspects of potential burnout.
To effectively employ Speech Burnout Detection, it is essential to consider key components such as prosody, speech rate, and clarity. These factors can change due to stress, fatigue, and emotional exhaustion. Analyzing these elements allows one to detect early signs of burnout through speech. Additionally, utilizing AI algorithms enhances detection accuracy, transforming raw speech data into actionable insights. This advanced approach empowers organizations to support their teams proactively and mitigate burnout, creating a healthier work environment.
Identifying Acoustic Signals
In the realm of Speech Burnout Detection, identifying acoustic signals is crucial for understanding emotional states in speech. Acoustic signals encompass various vocal characteristics, such as pitch, tone, and rhythm. By analyzing these features, we can gain insights into an individual's mental exhaustion or stress levels.
To effectively identify these signals, focus on the following aspects:
Pitch Variation: Changes in pitch may indicate stress or fatigue. A flat or monotonous tone can signal burnout.
Speech Rate: Slower speech often correlates with cognitive overload. Conversely, rapid speech might suggest anxiety, linked to burnout.
Volume Fluctuation: A decrease in volume can show diminished energy or interest, while erratic volume levels may indicate emotional distress.
Pauses and Filler Words: Increased pauses or the use of filler words can reflect hesitation or a lack of clarity, common signs of burnout.
Identifying these acoustic signals enables better support for individuals experiencing burnout, ultimately leading to more effective interventions and healthier work environments.
The Role of AI in Detecting Burnout
AI plays a transformative role in identifying burnout through advanced analytics of speech patterns and acoustic signals. By analyzing nuances such as pitch, tone, and rhythm, AI can uncover subtle indicators of emotional distress. This system empowers organizations to proactively address employee well-being, fostering a healthier work environment. Utilizing speech burnout detection technology, companies can identify individuals who may be experiencing burnout earlier than traditional methods would allow.
Furthermore, AI enhances the efficiency of burnout detection by processing vast amounts of conversational data quickly and accurately. This capability can lead to insights that are not easily visible through human observation alone. Consequently, implementing AI in speech burnout detection not only streamlines the process but also improves the precision of identifying individuals at risk. In effect, this technological synergy serves as a powerful tool in combating workplace burnout and enhancing overall employee productivity and satisfaction.
Extract insights from interviews, calls, surveys and reviews for insights in minutes
Steps to Implement Speech Burnout Detection Systems
Implementing Speech Burnout Detection systems involves several critical steps to ensure accuracy and reliability. First, data collection and annotation are paramount. Gather significant audio samples representing various states of employee interactions. It's essential to annotate these samples based on their emotional tone and intensity, which aids in training the detection models effectively.
Next, feature extraction from acoustic signals plays a crucial role. This step involves analyzing aspects like pitch, tone, and speech rate, which are indicative of burnout levels. By identifying these key features, developers can create robust algorithms that recognize burnout signals within speech.
Finally, developing AI models for detection is necessary. Using machine learning techniques, these models should be trained on the previously annotated data. Continuous evaluation and refinement of these models will enhance their accuracy, enabling effective detection of burnout via speech analysis. Each of these steps is vital for creating a reliable Speech Burnout Detection system.
Step 1: Data Collection and Annotation
In the initial phase of Speech Burnout Detection, data collection and annotation are crucial steps that lay the foundation for effective analysis. This process involves gathering acoustic signals from speech samples, which may relate to various contexts such as workplace environments or personal experiences. A diverse dataset is essential to capture the nuances of speech that may reflect burnout, such as tone, pitch, and rhythm.
Annotation involves labeling these collected samples to identify specific characteristics or emotions associated with burnout. Various methods can be employed, such as manual tagging or using semi-automated tools that enhance accuracy. By carefully collecting and annotating data, we ensure that the subsequent analysis is robust and reliable. Ultimately, this groundwork facilitates the detection of burnout through acoustic signals, paving the way for more sophisticated models and solutions in the future.
Step 2: Feature Extraction from Acoustic Signals
Feature extraction from acoustic signals is a crucial step in the process of detecting burnout through speech analysis. This involves analyzing various characteristics of the audio recordings to identify patterns indicative of fatigue. Key features such as pitch, tone, and speech rate provide insights into an individualโs emotional and cognitive state, helping to assess burnout. For optimal results, itโs important to standardize the raw audio data, allowing for consistent feature extraction across different samples.
Once the features are extracted, they serve as input for machine learning models that recognize burnout patterns. Analyzing these features can highlight subtle cues in speech that traditional methods might overlook. By focusing on acoustic parameters, we can enhance the accuracy of speech burnout detection, paving the way for more effective interventions. Understanding this step is essential for leveraging technology in recognizing the signs of burnout early and effectively.
Step 3: Developing AI Models for Detection
Developing AI models for detecting burnout through speech involves several critical steps. Initially, the acoustic features extracted in the previous phase serve as the foundational elements for the model. Utilizing these features, various algorithms can be trained to recognize patterns indicative of stress, fatigue, or disengagement. It is essential to select the right machine learning techniques, such as support vector machines or deep learning, to enhance accuracy in Speech Burnout Detection.
Once the model is trained, it undergoes rigorous testing and validation. Continuous evaluation against diverse datasets ensures the model can recognize signs of burnout in different contexts, making it robust and reliable. Finally, the integration of feedback loops allows for the model to improve over time, adapting to new patterns in speech data. This iterative process is crucial for maintaining the effectiveness of Speech Burnout Detection solutions in real-world applications.
Tools for Speech Burnout Detection
The identification of speech burnout requires reliable tools that can analyze acoustic signals effectively. Various platforms offer specialized functionalities to monitor speech patterns, which can highlight signs of burnout. For instance, software like Praat provides in-depth analysis of pitch, tone, and duration, enabling users to discern subtle vocal changes associated with stress and fatigue. Similarly, OpenSMILE excels in feature extraction, focusing on a wide array of acoustic features that can be critical for detecting burnout.
In addition to these, TensorFlow and Kaldi are popular frameworks used to develop custom AI models. These tools empower researchers to create tailored machine learning algorithms that can learn from specific datasets, thus enhancing the accuracy of burnout detection. Each of these tools plays a vital role in harnessing the power of acoustic signals, making them indispensable for effective speech burnout detection. By leveraging these technologies, practitioners can obtain actionable insights into individuals' mental states, ultimately paving the way for timely interventions.
insight7
Detecting burnout through Speech Burnout Detection offers significant insights into emotional and mental health states. By analyzing various acoustic signals in speech, AI can recognize patterns indicative of stress or fatigue. This technology allows for early intervention, making it easier to address employee well-being in real-time.
The intersection of AI and acoustic analysis facilitates the identification of key vocal indicators, such as changes in pitch, tone, and speech rate. As organizations increasingly value mental health, understanding these signals can enhance workplace culture. Tools like advanced speech analysis platforms can provide valuable feedback, enabling proactive measures against burnout. Overall, the integration of Speech Burnout Detection transforms traditional approaches to mental well-being in professional environments. Hence, cultivating a supportive atmosphere can lead to increased productivity and job satisfaction for all.
Praat
Praat is a powerful software tool widely used for analyzing speech and acoustic signals. Its capabilities allow researchers and developers to dive deep into the nuances of speech patterns that might indicate issues like burnout. By examining parameters such as pitch, intensity, and speech rate, Praat can serve as a vital resource in the realm of Speech Burnout Detection.
Using Praat isn't just about observing raw data; it requires understanding how these acoustic features relate to emotional states. For instance, a notable drop in pitch or a significant change in speaking speed can be red flags. Such insights can inform organizations of potential burnout within their teams, enabling proactive measures to enhance employee well-being and productivity. The combination of acoustic analysis and AI makes Praat indispensable for pioneering studies in this field.
OpenSMILE
OpenSMILE is a powerful toolkit specifically designed for analyzing acoustic signals in speech. It plays a pivotal role in the realm of Speech Burnout Detection by facilitating the extraction of relevant audio features that can signal signs of burnout. The toolkit processes speech recordings to identify parameters like pitch, tone, and emotional stress, enabling researchers and practitioners to gauge a speaker's mental state accurately.
Key functions of OpenSMILE include its ability to analyze large datasets efficiently, providing comprehensive insight into speech patterns. Users can customize the toolkit to focus on features that are particularly relevant for burnout detection. This adaptability allows for rigorous acoustic analysis, opening the door for predictive modeling of burnout indicators. By utilizing OpenSMILE, organizations can create targeted interventions while enhancing overall mental health support through data-driven insights.
TensorFlow
TensorFlow serves as an essential tool in the realm of Speech Burnout Detection. It is an open-source machine learning library that provides flexibility and comprehensive resources for developing AI models. Its extensive functionality allows users to construct, train, and deploy complex neural networks effectively, making it ideal for processing acoustic signals related to human speech.
With TensorFlow, developers can implement various algorithms to analyze speech patterns and detect potential burnout indicators. The library's capacity to handle large datasets is particularly valuable, enabling practitioners to train models on vast amounts of acoustic data. Furthermore, TensorFlow's community support and extensive documentation facilitate learning and troubleshooting, making it accessible for both beginners and experienced data scientists. By utilizing TensorFlow, researchers can enhance their ability to recognize critical burnout signals, thereby improving overall mental health awareness through innovative technology.
Kaldi
Kaldi is a powerful tool used in the field of speech technologies, particularly for processing acoustic signals. It offers a framework for building speech recognition systems, enabling researchers and companies to analyze and detect various speech patterns effectively. Utilizing Kaldi can significantly enhance Speech Burnout Detection capabilities by enabling the extraction of relevant features from audio recordings.
Using Kaldi, developers can create models that specifically target the acoustic signals associated with burnout. Firstly, it allows users to preprocess audio data thoroughly, ensuring high-quality input for analysis. Secondly, it offers deep learning techniques that enhance the model's accuracy in recognizing vocal attributes indicative of emotional states. Lastly, leveraging Kaldi's extensive library of resources supports ongoing improvements and refinements in detecting the subtle cues of burnout in speech. This empowers organizations to respond proactively to employee well-being, fostering a healthier workplace environment.
Conclusion: The Future of Speech Burnout Detection
As we look ahead, the future of Speech Burnout Detection holds immense promise. With advancements in artificial intelligence and acoustic analysis, we can expect more accurate and faster identification of burnout indicators in speech. These technological improvements will enable individuals and organizations to detect burnout early, allowing for timely interventions and support.
Moreover, integrating Speech Burnout Detection into workplace environments can foster better mental health awareness. By using acoustic signals as a tool for detection, we can create more responsive systems that prioritize well-being. In essence, the journey towards improving burnout detection through speech technology is just beginning, and its potential impact cannot be overstated.
Analyze qualitative data. At Scale.
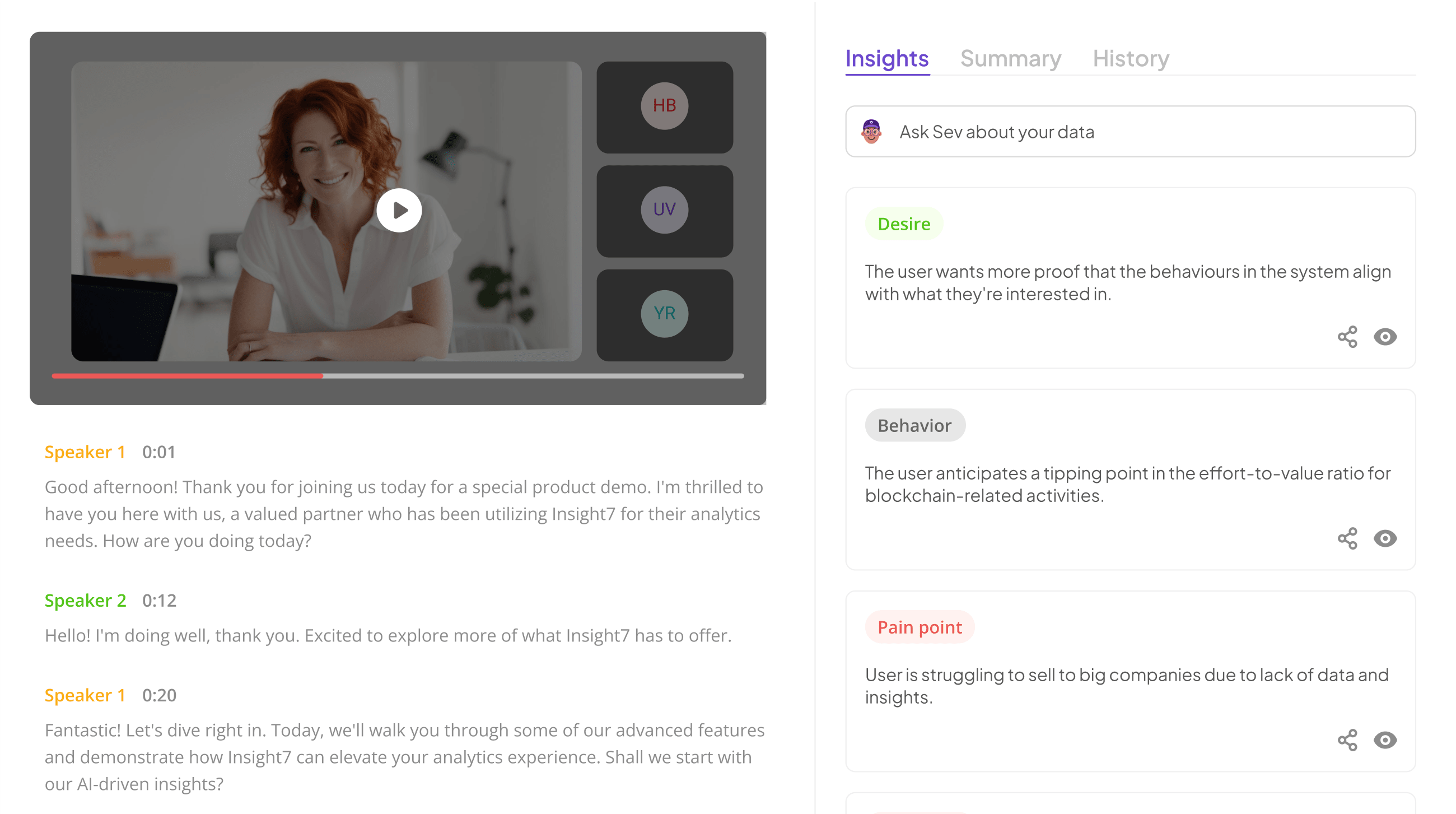