How to Create Model-Based Agents for Qualitative Research Success
-
Bella Williams
- 10 min read
Qualitative Agent Modeling plays a pivotal role in shaping qualitative research outcomes. By employing modeled agents, researchers can simulate complex interactions, enabling a deeper understanding of nuanced human behavior and responses. This approach offers a compelling method to analyze qualitative data, allowing for enriched insights that traditional methods might overlook.
In this introduction, we will explore how these models enhance the research process. From defining specific research objectives to designing meticulous agent frameworks, the pathway to effective qualitative research success hinges on thoughtful implementation of these strategies. Understanding how to harness the power of Qualitative Agent Modeling will empower researchers to elevate their studies and gain transformative insights.
Analyze & Evaluate Calls. At Scale.
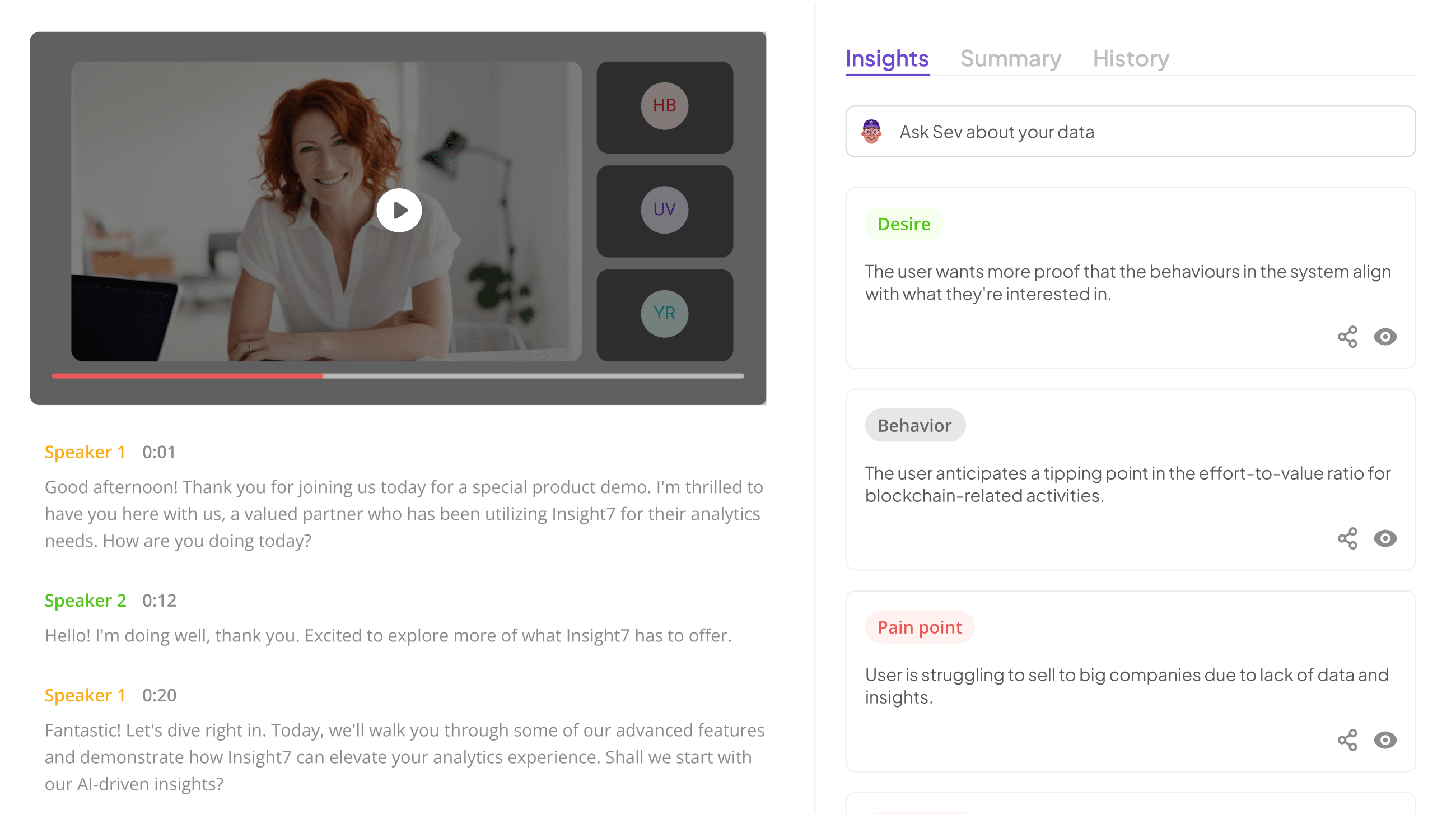
The Importance of Qualitative Agent Modeling
Qualitative Agent Modeling is an essential component of modern qualitative research, enhancing the analytical capacity of researchers. These models provide a structured way to synthesize information gathered from complex data sources, bridging gaps between raw data and actionable insights. By simulating the behaviors and interactions of various agents, researchers can gain nuanced perspectives that traditional methods may overlook.
The growing volume of data generated in research necessitates innovative approaches. Qualitative Agent Modeling allows researchers to efficiently analyze large datasets, transforming scattered insights into coherent narratives. Successful implementation of these models can lead to significant breakthroughs across fields, including social sciences, marketing, and user experience research. As research demands evolve, adopting qualitative agent modeling will enable researchers to stay ahead, ensuring their analyses remain relevant and impactful.
Understanding the Role of Agents
In qualitative research, understanding the role of agents is crucial for successful data handling and insights generation. Model-based agents serve as intermediaries that analyze and interpret qualitative data, streamlining the research process. These agents leverage algorithms and predefined parameters to distill vast amounts of information into manageable insights, making them invaluable in complex research environments.
The significance of these agents extends beyond mere data processing. They enhance contextual understanding by adapting analyses based on specific research objectives. For instance, qualitative agent modeling helps in identifying key themes and patterns that might otherwise remain obscured. Additionally, these agents can offer a more nuanced understanding of participant perspectives, which is essential for informing study conclusions and driving future inquiries. Recognizing their importance can significantly elevate the quality and robustness of qualitative research outcomes.
- Definition and significance of model-based agents in qualitative research
Model-based agents in qualitative research, often referred to as qualitative agent modeling, serve as vital tools for generating and analyzing qualitative data. These agents are designed to simulate human-like behavior, allowing researchers to create nuanced profiles that reflect diverse viewpoints. By employing such agents, researchers can gather richer insights that enhance the understanding of complex social phenomena.
The significance of model-based agents lies in their ability to facilitate deeper analysis and synthesis of qualitative data. They can efficiently process extensive datasets and identify patterns that might not be immediately visible. Moreover, these agents can adapt to various contexts, making them versatile across different research disciplines. Their deployment can lead to more robust findings and foster innovative approaches to problem-solving, ultimately driving qualitative research success.
- How these agents enhance data analysis and synthesis
Model-based agents play a crucial role in enhancing data analysis and synthesis in qualitative research. They enable researchers to effectively organize and interpret large datasets, allowing for deeper insights. By employing these agents, researchers can streamline the analysis process, identifying patterns and trends with greater efficiency. This efficiency not only saves time but also improves the quality of the insights derived from the data.
Qualitative agent modeling facilitates the integration of diverse data sources, providing a comprehensive view of the subject matter. This approach allows researchers to conduct comparative analyses and explore nuanced perspectives across various datasets. As a result, these agents contribute significantly to the synthesis of information, leading to informed decisions and actionable strategies. Ultimately, effective data analysis and synthesis through qualitative agent modeling enhance the overall impact of qualitative research, driving meaningful outcomes.
Applications in Research Contexts
In research contexts, qualitative agent modeling serves as a powerful tool for data analysis and interpretation. Different fields, including social sciences, healthcare, and market research, benefit significantly from these agent-based models. By simulating human interactions and experiences, researchers can gain deeper insights into complex social dynamics and consumer behaviors.
Successful applications of qualitative agent modeling include market evaluations and user experience studies. For instance, in product development, agents can analyze customer feedback and preferences, helping to tailor services to meet specific needs. Similarly, in healthcare, these models can simulate patient interactions to improve service delivery and patient outcomes. Such diverse applications illustrate the adaptability and effectiveness of qualitative agent modeling in enhancing research outcomes.
- Insights into various fields benefiting from agent-based models
Agent-based models have proven to be transformative across numerous fields, enriching qualitative research through their unique capabilities. From social sciences to healthcare, the benefits of these models are diverse and significant. In sociology, agent-based models enable researchers to simulate social interactions, shedding light on complex societal behaviors and phenomena. In economics, they help in understanding market dynamics by mimicking decision-making processes among consumers and businesses alike.
Moreover, the educational sector leverages these models to analyze student behavior, enhancing learning outcomes through targeted interventions. Environmental studies also benefit, as agent-based models can simulate ecosystem dynamics, illustrating the impact of human behavior on natural resources. By employing qualitative agent modeling, researchers can capture intricate relationships and patterns that standard methodologies may overlook, facilitating deeper insights into their respective fields. Whether in understanding consumer trends or social networks, the applications are as varied as they are compelling.
- Examples of successful implementations in qualitative research
Successful implementations of qualitative research often illustrate how qualitative agent modeling can transform data analysis and interpretation. One prominent example is the use of agent-based models in educational research, where agents simulate student interactions in virtual learning environments. This allows researchers to uncover nuanced insights about learning behaviors, thus improving educational strategies.
Another effective application can be seen in healthcare research, where qualitative agents represent patient experiences. By analyzing these agents' interactions, researchers gain an in-depth understanding of patient needs and preferences. These examples demonstrate how qualitative agent modeling enhances data richness, revealing patterns that traditional methods may overlook. Ultimately, the integration of such models into various research settings underscores their potential to drive more successful outcomes in qualitative research endeavors.
Steps to Create Effective Qualitative Agent Models
Creating effective qualitative agent models begins with clearly defining your research objectives. This foundational step ensures that your models address relevant questions, providing meaningful insights. Start by outlining what you aim to discover through your research. Techniques such as stakeholder interviews and situational analysis can help clarify these objectives.
Next, design your agent model with precision. Selecting appropriate data sources and parameters is critical; ensure these align with your objectives. Employing robust methodologies and tools will enhance the reliability of your model. For instance, you can utilize software like NVivo or ATLAS.ti to assist in the design process. Remember, specificity is vital, as it reduces the risk of ambiguity and enhances the overall quality of your qualitative agent modeling. Regularly evaluate and refine the model based on initial findings, promoting a cycle of continuous improvement.
Extract insights from interviews, calls, surveys and reviews for insights in minutes
Step 1: Define Your Research Objectives
Clearly defining your research objectives is crucial to the success of qualitative agent modeling. Start by identifying your specific goals, as these will guide the entire research process. Consider what insights you aim to uncover, which stakeholders you want to engage, and how these insights will be utilized. Each objective should be clear, focused, and aligned with the broader context of your research to ensure that all efforts contribute meaningfully.
Next, utilize techniques such as brainstorming sessions, stakeholder interviews, and problem-framing workshops to solidify your objectives. It is important to engage all team members in this process for a more comprehensive understanding of the research question. By defining your objectives precisely, you set the stage for effective agent modeling that directly supports your research goals, making your findings more impactful in the qualitative research arena.
- Importance of clarity in research objectives
Clarity in research objectives is fundamental when creating model-based agents for qualitative research success. Clearly defined research objectives provide a solid framework for qualitative agent modeling. When researchers outline specific goals, they are better equipped to design agents that target pertinent data and insights. This not only facilitates the research process but also enhances the quality of the outcomes derived from qualitative studies.
To achieve clarity in research objectives, consider the following key points:
Specificity: Define what you aim to explore or understand within your study.
Measurability: Ensure your objectives can be assessed through qualitative metrics.
Achievability: Set practical goals that can be realistically accomplished within your research's timeframe and scope.
Relevance: Align your objectives closely with the core themes and questions central to your research interest.
Time-Bound: Incorporate a timeline for achieving your objectives, which can guide your research pace and focus.
These elements collectively contribute to developing effective qualitative agent models that yield valuable insights. Thus, taking careful consideration in defining objectives streamlines the process toward successful qualitative research outcomes.
💬 Questions about How to Create Model-Based Agents for Qualitative Research Success?
Our team typically responds within minutes
- Techniques for setting precise and achievable goals
Setting precise and achievable goals is crucial for success in qualitative agent modeling. First, outline specific objectives that reflect what you want to achieve in your research. This clarity helps in maintaining focus throughout the project. When goals are vague, they lead to confusion and a lack of direction, which can impede progress.
Next, employ SMART criteria to refine these objectives. Ensure your goals are Specific, Measurable, Achievable, Relevant, and Time-bound. For instance, instead of stating a general aim like "understand user behavior," a more focused goal would be "analyze user feedback from 100 interviews within two months." This method not only enhances goal clarity but also provides a framework for evaluating your qualitative agent modeling efforts over time. Employing these techniques ensures your goals align with the overall objectives of your research project, paving the way for meaningful insights and contributions to the field.
Step 2: Design Your Agent Model
Designing your agent model is a crucial step in qualitative agent modeling. To effectively create a model-based agent, begin by carefully selecting the data sources and parameters that will inform your research. Consider the specific information you want to gather and analyze, as this will shape your agent's functionality. Your choices here determine how well the agent can adapt to the qualitative nuances of the data you are exploring.
Next, leverage appropriate tools and methodologies for designing a robust model. This involves outlining the algorithms and frameworks that will guide the agent's decision-making and actions during research. Focusing on these core elements ensures that your model not only meets current research needs but is also adaptable to future inquiries. By constructing a well-defined agent model, you pave the way for effective qualitative insights and research success.
- Selecting appropriate data sources and parameters
Selecting appropriate data sources and parameters is a critical step in the creation of effective qualitative agent models. First, identify diverse data sources that can capture subtle nuances in human behavior and attitudes. This can include interviews, surveys, focus groups, or observational data. Additionally, ensure that your parameters focus on specific variables that are relevant to your research objectives. Tailoring your approach to the distinctive context of your qualitative research will enhance the richness and depth of the insights generated.
Next, consider the relevance and credibility of the chosen data sources. It is essential to select sources that truly reflect the perspectives of the target demographic. Understanding how to define the parameters will guide the modeling process effectively. By refining your focus, you increase the accuracy of your qualitative agent modeling, ultimately leading to more meaningful outcomes in your research efforts. This strategic selection process must align with your overall research goals, ensuring that the data collected is fit for purpose.
- Tools and methodologies for designing robust models
Designing robust models for qualitative agent modeling requires a thoughtful approach that encompasses both the tools employed and the methodologies adopted. Various frameworks can guide researchers in their quest to create effective agents that yield meaningful qualitative insights. Firstly, familiarity with diverse modeling tools is essential, as each offers distinct functionalities suited to different research needs. Software like NVivo, ATLAS.ti, and MAXQDA can facilitate data management and analysis, enhancing the overall efficiency of the qualitative research process.
Moreover, employing structured methodologies can streamline model construction. These may include defining the research objectives clearly, selecting relevant data sources, and applying triangulation techniques to validate findings. Using iterative development allows for ongoing improvements based on feedback and data insights. By integrating these tools and methodologies, researchers can design robust qualitative agent models that not only capture nuanced human behavior but also translate those insights into actionable knowledge for various applications.
Top Tools for Qualitative Agent Modeling
Creating effective qualitative agent models relies heavily on the right tools. When delving into qualitative agent modeling, selecting the appropriate software can enhance both data collection and analysis. Insight7 stands out for its user-friendly interface, enabling users to analyze large volumes of text from interviews effortlessly. This tool facilitates the extraction of valuable insights from customer conversations, bridging gaps in traditional analysis methods.
Apart from Insight7, several other tools contribute significantly to qualitative agent modeling. NVivo, for example, excels in managing and examining various data formats, allowing researchers to visualize trends effectively. ATLAS.ti offers robust qualitative data analysis features, making it easier to interpret complex datasets. MAXQDA aids in coding and organizing qualitative data, while Dedoose provides a web-based option that enhances collaborative efforts among research teams. Each of these tools plays a critical role, addressing different needs within qualitative research, ultimately driving success.
Insight7
In Insight7, the exploration of qualitative agent modeling reveals its profound impact on enhancing research efforts. Model-based agents serve as crucial tools for navigating complex qualitative data, transforming how researchers analyze and synthesize information. These agents are particularly beneficial in contexts where traditional analysis methods struggle to keep pace with the volume of data generated by dynamic customer interactions.
To harness the full potential of qualitative agent modeling, researchers should focus on several key elements. First, it’s imperative to clearly define research objectives that guide the development of agent models. Next, designers must choose appropriate data sources and parameters that align with the targeted outcomes. Lastly, leveraging advanced tools specifically tailored for qualitative agent modeling can streamline the analysis process and enable efficient extraction of actionable insights. By embracing these principles, researchers can significantly enhance their qualitative research success.
- Overview and unique features
The overview of qualitative agent modeling highlights its central role in enhancing qualitative research outcomes. This innovative approach empowers researchers to create model-based agents that process and analyze data efficiently. The unique features of qualitative agent modeling include its ability to automate data synthesis, identify patterns, and generate insights from complex datasets. As a result, researchers can focus on strategic analysis rather than labor-intensive data manipulation.
Moreover, qualitative agent modeling democratizes access to research insights, making it easier for any team member to contribute to the process. This encourages broader participation and fosters an inclusive research environment. The technology's user-friendly interface streamlines workflows, enabling quick transformations from raw data to actionable reports. By integrating tools that visualize conversations and key sentiments, qualitative agent modeling not only enhances efficiency but also enriches the depth of qualitative research.
- Applications in qualitative research
Qualitative Agent Modeling has profound applications in qualitative research, extending its relevance across various fields. From healthcare to marketing, these models serve as a bridge that connects complex data with insightful interpretations. They enable researchers to examine human behaviors and social interactions by simulating scenarios and analyzing responses. This approach not only streamlines data analysis but also helps in uncovering underlying trends and patterns that inform decision-making.
In practice, qualitative agent models find utility in different areas such as user experience design, where they enhance our understanding of customer interactions. Additionally, they can be used to evaluate the effectiveness of communication strategies in organizational contexts. By capturing and analyzing qualitative data from various sources, these models empower researchers to generate actionable insights. Overall, integrating qualitative agent modeling into research processes fosters a deeper understanding of qualitative phenomena, ultimately leading to more informed and impactful outcomes.
Other Essential Tools
Choosing the right tools is essential for successful qualitative agent modeling. Various software options can enhance your research efforts by providing advanced data analysis capabilities. NVivo offers a user-friendly interface for organizing and analyzing qualitative data, ensuring researchers can easily code and retrieve complex insights. ATLAS.ti is another essential tool that excels in visualizing data relationships, empowering users to derive meaningful patterns.
MAXQDA stands out for its versatile support of both qualitative and mixed methods research. It allows for comprehensive data analysis, which facilitates multi-dimensional insights. Furthermore, Dedoose is beneficial for collaborative projects, enabling multiple users to work on data analysis simultaneously in a cloud-based environment. Using these essential tools effectively will streamline your research process and enhance the quality of the insights generated through qualitative agent modeling.
- NVivo
NVivo is a powerful tool that supports qualitative research through model-based agent methodologies. It provides an environment where researchers can efficiently gather, organize, and analyze qualitative data. By creating distinct projects, researchers can compile various data sources, such as interviews and focus groups, enabling comprehensive analysis and insight generation.
One of the key features of NVivo is its ability to facilitate the visualization of data patterns and trends. This is particularly beneficial for Qualitative Agent Modeling, as it allows researchers to easily identify themes and correlations within their data. The intuitive interface simplifies the process of importing files, transcribing audio, and even integrating with platforms like Google Drive. By utilizing NVivo, researchers can streamline their workflows, thus enhancing the overall quality and impact of their qualitative research outcomes.
- ATLAS.ti
The application of qualitative agent modeling is significantly enhanced by effective tools designed for data analysis. One such tool empowers researchers to import various data types easily, facilitating an organized workspace for qualitative research. With features that support file uploads from multiple sources, including audio and text, researchers can streamline their workflow and focus on extracting insights.
This tool offers rich functionalities, such as creating project-specific queries that allow users to visualize and analyze patterns across vast datasets. By setting up projects, researchers can manage large volumes of transcriptions and responses effectively. This organization fosters a clearer understanding of participant feedback and responses, leading to valuable insights that inform research findings and decision-making.
- MAXQDA
MAXQDA is a powerful tool that facilitates the process of qualitative agent modeling in research. It provides a robust framework for organizing and analyzing qualitative data, ultimately enhancing the depth of insights gathered. Users can easily create projects, upload various data types, and transcribe audio files directly within the platform. This versatility makes it an essential component for researchers aiming to build effective agent models.
A significant feature of MAXQDA is its ability to visualize connections and trends within data. Users can deploy various analytical techniques, such as coding responses and creating matrices to compare results. This feature allows researchers to quickly identify patterns, refining their understanding of qualitative data. MAXQDA not only simplifies data management but also boosts the overall effectiveness of qualitative agent modeling in various research contexts. As researchers navigate complex data landscapes, tools like MAXQDA serve as vital assets for achieving research success.
- Dedoose
Dedoose serves as an essential tool for those venturing into qualitative agent modeling in research. This platform enables researchers to organize, analyze, and visualize data effectively. Unlike other qualitative software, it emphasizes collaborative efforts, allowing teams to work together seamlessly, regardless of location. By integrating different forms of data, users can gain comprehensive insights that drive qualitative research forward.
One of the standout features of this platform is its user-friendly interface, which simplifies complex data analysis processes. Researchers can use it to code data, uncover patterns, and generate visual data representations. This capability not only enhances understanding but also aids in presenting findings convincingly. Additionally, the platform’s cloud-based system allows real-time updates, ensuring that all team members access the latest information. In this way, employing qualitative agent modeling becomes more efficient, promoting success in various research endeavors.
Conclusion: Achieving Success with Qualitative Agent Modeling
Success with qualitative agent modeling hinges on a clear understanding of research objectives and precise model design. By defining what you wish to achieve, you create a solid foundation for your agents to operate effectively. Additionally, specific guidelines on performance standards enhance accountability and ensure that your models yield reliable insights.
Implementing qualitative agent modeling enables researchers to derive deeper insights and make informed decisions. As agents accurately interact with data, they facilitate a more consultative approach, enriching the quality of research outcomes. In this evolving landscape, harnessing such models can significantly contribute to conducting impactful qualitative research.
💬 Questions about How to Create Model-Based Agents for Qualitative Research Success?
Our team typically responds within minutes