AI-Driven Qualitative Analysis is revolutionizing how researchers approach multi-site studies. With the growing complexity of gathering and interpreting qualitative data, traditional manual methods often fall short, leading to inconsistencies and delays. By integrating AI tools, researchers can streamline their processes, allowing for quicker insights and more reliable data synthesis from diverse locations.
In this section, we will explore the powerful advantages of utilizing AI in qualitative analysis. We will discuss how these technologies enhance data processing and improve consistency across teams. Embracing AI-Driven Qualitative Analysis not only addresses the challenges researchers face, but also provides a framework for more comprehensive and effective multi-site research projects.
Analyze qualitative data. At Scale.
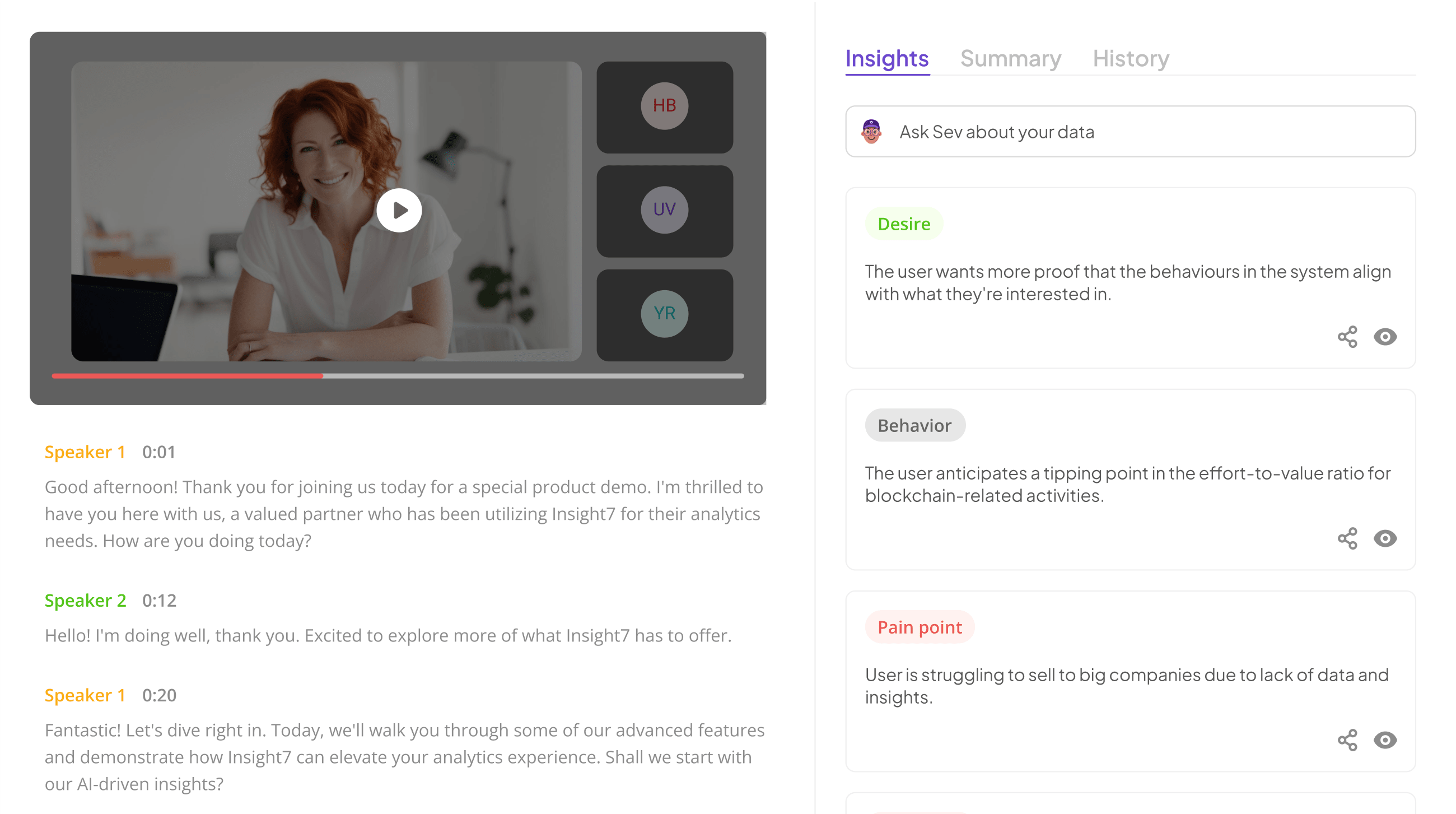
Key Benefits of AI-Driven Qualitative Analysis for Multi-Site Studies
AI-Driven Qualitative Analysis brings significant advantages to multi-site studies, primarily in enhancing data processing efficiency. Traditional methods of analyzing qualitative data can be slow and labor-intensive, often leading to delays in obtaining actionable insights. By employing AI tools, researchers can swiftly process large volumes of interview transcripts across multiple locations. This efficiency not only saves time but also enables teams to generate insights more rapidly, meeting the growing demands of clients for timely reporting.
Moreover, AI-driven analysis fosters improved consistency and reliability in findings. In multi-site research, differing interpretations from various researchers can introduce bias and inconsistencies in the results. Utilizing AI minimizes these discrepancies by providing standardized frameworks for analysis. As a result, organizations can ensure that insights derived from different sites are coherent and aligned. This not only strengthens the validity of the study but also enhances the decision-making process based on those insights. Integrating AI in qualitative analysis offers an innovative approach to overcoming traditional challenges associated with multi-site research.
Enhanced Data Processing Efficiency
Efficient data processing serves as the backbone of any successful AI-driven qualitative analysis. When conducting multi-site research, the ability to streamline the handling of diverse datasets is crucial. Different locations may generate unique insights, but compiling and interpreting vast volumes of qualitative data can be overwhelming. By employing AI tools, researchers can automate initial data parsing and categorization, significantly reducing the time required for preliminary analysis.
Moreover, this enhanced data processing efficiency safeguards consistency in results. AI algorithms can detect patterns and trends across various interviews seamlessly, ensuring that no critical insights are overlooked. This not only aids in maintaining the reliability of the analysis but also frees up valuable time for researchers to engage in deeper, more nuanced interpretations. With AI-driven qualitative analysis, teams can focus on drawing meaningful conclusions from their findings, thereby driving informed decision-making in a multi-site context.
Improved Consistency and Reliability
Achieving improved consistency and reliability through AI-driven qualitative analysis can significantly enhance research outcomes across multiple sites. By integrating AI tools, researchers can process vast amounts of qualitative data more uniformly, mitigating the risks of human bias. This approach not only streamlines the analysis process but also ensures that insights remain coherent across varied locations and contexts.
AI tools facilitate the systematic examination of qualitative data, providing standardized coding and theme identification. This level of automation reduces discrepancies that often arise when analyzing data manually by different teams. Additionally, researchers can quickly aggregate insights from multiple projects, allowing for a comprehensive view that strengthens decision-making. As researchers increasingly adopt AI technology, the overall reliability of qualitative findings improves, leading to more informed strategies and solutions across diverse sites.
Extract insights from interviews, calls, surveys and reviews for insights in minutes
Tools for Conducting AI-Driven Qualitative Analysis Across Multiple Sites
In today’s multi-site qualitative research landscape, utilizing AI-driven qualitative analysis tools is imperative for effective data handling. Researchers can benefit from several leading platforms that streamline and enhance the analysis process. Popular tools such as Insight7, NVivo, Dedoose, MAXQDA, and Atlas.ti provide distinctive features tailored for diverse research needs. These tools enable comprehensive coding, text analysis, and visualization, significantly reducing time and effort in gathering insights.
When employing these tools, researchers can expect improved accuracy and consistency in their findings. For instance, Insight7 integrates AI capabilities to automate coding, thereby minimizing bias that can arise from manual processes. Similarly, NVivo's strong analytical framework allows for complex inquiries, facilitating richer interpretations across different sites. By engaging these platforms, research teams can efficiently manage qualitative data while ensuring that insights generated reflect a well-rounded perspective across the geographical spectrum involved in the study.
Insight7
AI-Driven Qualitative Analysis offers a transformative approach for conducting multi-site qualitative research. To effectively harness this technology, organizations must understand key components that enhance the analytical process. Initially, identify the research objectives and the specific qualitative data you aim to analyze. This includes interviews, focus groups, or open-ended survey responses, which can be collected across different sites.
Next, choose the right AI tools that facilitate data synthesis and help in identifying patterns across sizable datasets. Utilizing AI can significantly improve the speed and reliability of qualitative analysis. Once the data is processed, interpreting the insights collaboratively with all stakeholders provides critical context and drives actionable outcomes.
By following these steps, organizations can create a robust framework for conducting AI-driven qualitative analysis, enabling a more informed and strategic approach to decision-making across multiple sites. The integration of sophisticated AI tools simplifies the complexities of qualitative analysis, ensuring no critical insights are overlooked.
NVivo
NVivo is a powerful tool designed to support AI-Driven Qualitative Analysis in multi-site research projects. It simplifies the process of managing and analyzing qualitative data, enabling researchers to identify patterns and themes more efficiently. With features tailored for collaboration, NVivo allows teams across different locations to work together seamlessly. This capability is particularly beneficial in multi-site studies where data consistency and reliability are paramount.
One key advantage of using NVivo is its ability to handle various data types, such as interviews, focus groups, and surveys. Additionally, it offers tools for coding and categorizing data, ensuring a thorough analysis process. Through advanced visualization options, NVivo helps users present their findings in an engaging manner. By integrating these features, researchers can transform complex qualitative data into actionable insights, ultimately enhancing the overall research outcome. Using NVivo effectively can result in significant improvements in both the analysis and interpretation of data collected from multiple sites.
Dedoose
Dedoose stands out as an efficient tool for teams conducting AI-driven qualitative analysis in multi-site research. This platform enables researchers to upload and organize data from various sources effortlessly. Whether working with interview transcripts or survey responses, the application’s versatility facilitates a seamless analysis process. Users can extract meaningful themes, generate visualizations, and create comprehensive reports, enhancing the overall research experience.
One notable feature of Dedoose is its ability to handle data privacy responsibly while maintaining a high level of accuracy. With a native transcription service available in over 60 languages, it allows researchers to focus on their insights rather than spending excessive time on manual data entry. By simplifying the analysis of qualitative data, Dedoose empowers users to draw actionable conclusions faster, ultimately fostering more efficient collaboration across multi-site studies.
MAXQDA
MAXQDA serves as a powerful tool for conducting AI-driven qualitative analysis, especially in multi-site research settings. It offers intuitive features designed to help researchers analyze vast amounts of qualitative data more effectively. Through its user-friendly interface, researchers can seamlessly organize interviews, surveys, and focus groups, which are critical for obtaining comprehensive insights across different locations.
To maximize the potential of MAXQDA, researchers should focus on the following aspects:
Data Organization: This enables efficient storage and retrieval of qualitative data, ensuring that key themes and insights are easily accessible.
AI Integration: By utilizing machine learning capabilities, MAXQDA can perform initial analyses on large datasets, allowing researchers to identify trends and patterns quickly.
Visualization Tools: The software provides various visualization options, helping to transform complex data into comprehensible formats.
By effectively leveraging these features, researchers can enhance their workflows significantly, paving the way for more profound, AI-driven insights across diverse sites.
Atlas.ti
In the realm of AI-driven qualitative analysis, utilizing advanced tools is essential for managing data from multiple sites. This is where Atlas.ti excels, offering a comprehensive platform that simplifies the analysis process while enhancing collaboration. The tool’s robust capabilities enable researchers to efficiently code, annotate, and visualize qualitative data, which is vital for drawing meaningful insights. Its intuitive interface also allows teams to work seamlessly, regardless of geographic location, ensuring that all voices and perspectives are represented in the analysis.
Moreover, Atlas.ti harnesses the power of AI to streamline data processing. By automating routine tasks, researchers can focus on interpretation and understanding, rather than getting bogged down by manual coding. This results in improved analysis quality and consistency. Embracing such dynamic tools is not just about efficiency; it’s about elevating the quality of insights derived from diverse data sources. Utilizing Atlas.ti in AI-driven qualitative analysis can transform how multi-site studies are conducted, ensuring richer, more actionable outcomes.
Step-by-Step Guide to Implement AI-Driven Qualitative Analysis in Multi-Site Projects
To implement AI-Driven Qualitative Analysis in multi-site projects, start with thorough data collection and integration. Collect qualitative data from all sites through interviews, focus groups, or surveys, ensuring consistency in the questions asked. Once your data is gathered, integrate it into a suitable format compatible with your AI tools. This important step allows for comprehensive data analysis across sites.
Next, utilize AI tools for data analysis, choosing ones that best suit your project's needs. These tools can quickly identify themes and patterns, reducing the time and effort required for manual analysis. After the AI generates insights, it is essential to interpret these findings rigorously. Cross-reference the insights with data from all sites to ensure accuracy and depth in your interpretation. This systematic approach enhances the reliability of your findings, providing valuable and consistent insights across your multi-site project.
Step 1: Data Collection and Integration
Effective data collection and integration are fundamental to conducting AI-Driven Qualitative Analysis. The first step involves gathering qualitative data from multiple sources, including interviews, focus groups, and surveys. It’s crucial to ensure this data is diverse and represents the various perspectives inherent in multi-site research. By doing this, researchers can create a richer context for their analysis, which can significantly enhance the quality of the insights generated.
Once the data is collected, integration comes into play. This process involves consolidating information from different sites and formats, making it accessible for AI analysis. Utilizing suitable AI tools, researchers can upload and organize datasets efficiently. This stage not only simplifies the workflow but also ensures compliance with data privacy requirements. Therefore, a well-executed data collection and integration phase sets a solid foundation for meaningful analysis, driving insights that can lead to informed decision-making across multiple contexts.
Step 2: Utilizing AI Tools for Data Analysis
AI-Driven Qualitative Analysis plays a pivotal role in streamlining data analysis during multi-site research projects. First, it efficiently categorizes and synthesizes large volumes of qualitative data. Utilizing AI tools can help researchers swiftly analyze participant insights and identify recurring themes. This accelerates the process of gaining actionable insights from diverse sources.
Next, researchers should focus on defining specific themes relevant to their study objectives. By doing so, the AI can group insights into meaningful categories, allowing for easier interpretation. Tools can also provide sentiment analysis, which elevates understanding of participant emotions around key topics. Ultimately, leveraging these technologies not only saves valuable time but also enhances the accuracy and depth of the analysis. Therefore, employing AI tools is essential for achieving high-quality results in multi-site qualitative research.
Step 3: Interpreting AI-Generated Insights Across Sites
Interpreting AI-generated insights across multiple sites is essential for a cohesive understanding of qualitative data. This process involves considering various perspectives and contextual factors that may arise from different locations. Begin by examining the generated insights closely. Look for common themes or contrasts that might highlight unique cultural or operational nuances across sites. Such insights can provide depth to findings, driving more informed decision-making.
Furthermore, it's crucial to validate the AI-generated insights through cross-referencing with original data sources, such as interviews or focus groups. This verification ensures the reliability of the findings. Additionally, engage stakeholders in discussions around these insights to draw out diverse interpretations. By fostering collaboration, you can enhance the quality and applicability of AI-driven qualitative analysis, leading to actionable outcomes that resonate across all participating sites.
Conclusion: Embracing AI-Driven Qualitative Analysis for Comprehensive Multi-Site Studies
As we conclude our exploration of AI-Driven Qualitative Analysis for multi-site studies, it’s essential to recognize the transformative potential these tools bring to qualitative research. Embracing AI not only enhances the accuracy of insights but also streamlines the analysis process, making it more efficient and less susceptible to human bias. With growing demands for quick and reliable results, integrating AI in qualitative methodology becomes crucial for researchers striving to maintain quality across multiple sites.
Utilizing AI-driven tools fosters collaboration among diverse research teams, allowing for a unified approach to data interpretation. By addressing inconsistencies and expediting reporting, researchers can focus on enriching their understanding of complex data. Ultimately, those who adopt AI-driven qualitative analysis will be better equipped to navigate the challenges of multi-site studies and deliver comprehensive insights that truly reflect the multifaceted nature of human experience.