How to Conduct Large-Scale Interview Analysis with AI
-
Bella Williams
- 10 min read
“`markdown
In the realm of AI-powered interview analysis, structured data presentation is essential. By employing effective markdown techniques, analysts can clearly communicate findings and insights derived from extensive interview data. This formatting helps ensure that crucial themes and patterns within the data are highlighted and easily accessible to stakeholders.
Markdown allows users to create visually organized reports that can include headings, lists, and links. For instance, it can be beneficial to break down data into categories, such as key themes, findings, and recommendations. Utilizing lists or bullet points enhances readability, making it simpler for teams to identify core areas of interest. Moreover, incorporating visual elements like charts within markdown can effectively showcase trends gleaned from the data, thus enhancing the overall impact of the AI-powered interview analysis outcomes.
Introduction to AI-powered Interview Analysis
AI-powered Interview Analysis has emerged as a transformative solution for organizations seeking to manage extensive qualitative data efficiently. In today's fast-paced environment, the ability to quickly capture and interpret interview insights is crucial for informed decision-making. Traditional methods of manual analysis can be cumbersome and prone to biases, leading to inconsistent findings across research teams.
With the integration of AI into the interview analysis process, organizations can streamline their workflows. By automating the extraction of themes and trends from interview transcripts, AI technology not only accelerates report generation but also enhances the overall quality of insights. Embracing this innovative approach allows teams to focus on strategic interpretation rather than getting bogged down in repetitive tasks, paving the way for more impactful outcomes.
Analyze & Evaluate Calls. At Scale.
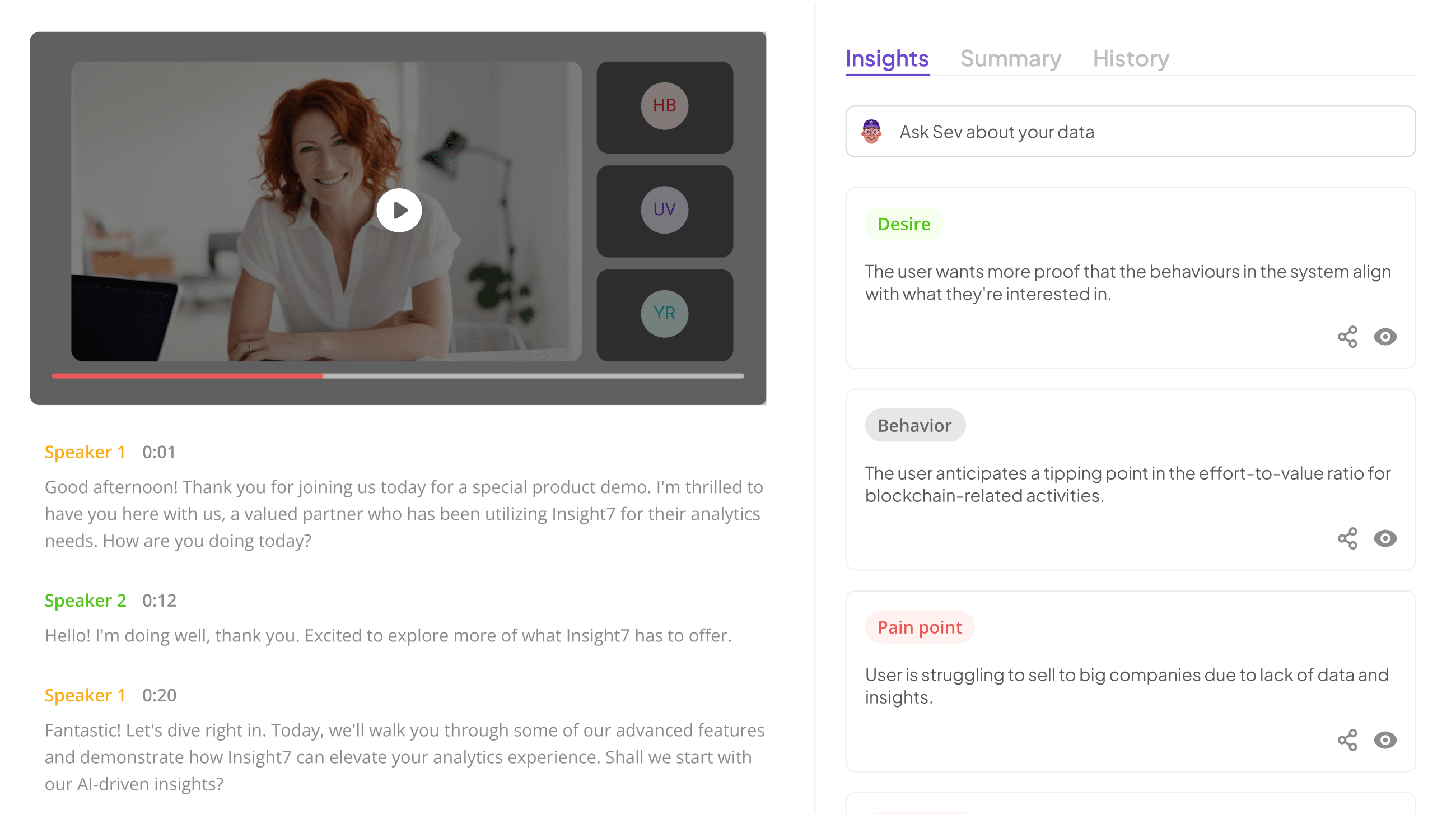
Leveraging AI-powered Interview Analysis Tools
AI-powered Interview Analysis tools offer innovative solutions to streamline the daunting task of analyzing qualitative data. Traditional methods often involve time-consuming manual processes and can lead to inconsistencies among team members. By incorporating these advanced tools, organizations can automate large-scale analysis, thus enhancing speed and accuracy. This shift not only accelerates report generation but also reduces biases that may arise from different interpretations.
Utilizing such tools typically involves a few key strategies. First, teams can leverage AI algorithms to analyze transcripts, enabling them to identify themes and insights swiftly. Secondly, the integration of natural language processing techniques aids in understanding the nuances of interview responses. Lastly, these tools can generate comprehensive reports and visualizations, facilitating better decision-making based on reliable insights. Embracing AI-powered Interview Analysis tools ultimately enhances efficiency and fosters data-driven strategies within organizations.
The Role of insight7 and Other Key Tools
AI-powered interview analysis relies on sophisticated tools designed to process and derive insights from large volumes of qualitative data. A key player in this domain is a robust platform that streamlines the journey from raw interviews to actionable insights. This particular tool helps organizations save time and resources by automating much of the initial analysis. It enables teams to focus on deeper insights rather than getting bogged down in transcribing and basic sorting.
In addition to this leading tool, several other platforms enhance AI-powered interview analysis. These include alternative solutions that may offer unique features, such as advanced sentiment analysis and customizable reporting capabilities. Each tool serves a specific need, ensuring users can find the right fit for their interview analysis requirements. Choosing the appropriate combination of these tools can significantly enhance the efficiency and effectiveness of analyzing qualitative data for informed decision-making.
- insight7: A leading tool for large-scale interview analysis.
insight7 stands out as a leading tool for large-scale interview analysis, particularly for organizations looking to employ AI-powered strategies. Its innovative approach simplifies the process of analyzing vast amounts of data derived from interviews, making it accessible for teams of all sizes. By utilizing this tool, researchers can efficiently decipher qualitative data, transforming it into actionable insights that inform strategic decisions.
Users often face challenges like time consumption and data biases while analyzing interviews. However, this tool mitigates those obstacles by automating transcription and analysis processes, significantly speeding up report generation. With built-in consistency checks, teams can trust the accuracy of results, ultimately enhancing their decision-making capabilities. Embracing AI-powered interview analysis allows businesses to stay competitive in fast-paced environments, where understanding customer insights is crucial.
- Tool 2: Overview and features.
AI-powered Interview Analysis provides users with innovative tools designed to analyze and derive insights from large-scale interview data effectively. In this section, we will explore the features of Tool 2, emphasizing its functionality and user-friendly design. This tool streamlines the research process, enabling teams to identify themes and insights with just a few clicks. It allows users to define project goals, whether they aim to improve engagement or drive sales, ensuring that the analysis aligns with specific objectives.
One of the standout features of Tool 2 is its ease of navigating themes and insights. Users can quickly delve into specific areas to uncover pain points, supported by contextual quotes from interview transcripts. The ability to publish structured reports with findings, insights, and recommendations enhances the overall efficiency of the analysis process. With customizable templates for exporting reports, this tool not only helps visualize data but also ensures that users can present their findings in an engaging manner. Overall, Tool 2 exemplifies the capabilities of AI-powered Interview Analysis in transforming raw qualitative data into actionable insights.
- Tool 3: Overview and features.
Tool 3 offers a comprehensive approach to AI-powered interview analysis, designed to streamline the process and enhance accuracy. This tool enables analysts to set project goals efficiently, allowing for targeted insights based on user-defined parameters. It supports a variety of objectives, such as improving engagement, driving sales, or refining customer-centric strategies, making it adaptable across different research needs.
Key features of this tool include the ability to uncover themes from interviews seamlessly, providing actionable insights backed by direct quotes from transcripts. Users can easily navigate through themes, edit insights, and publish findings into structured reports. These reports summarize key conclusions, align insights with project goals, and even allow for template customization when exporting. By integrating these functionalities, Tool 3 not only simplifies analysis but also enhances the reliability of insights drawn from extensive interview data.
- Tool 4: Overview and features.
AI-powered Interview Analysis tools provide a comprehensive solution for analyzing large volumes of interview data efficiently. Tool 4 offers a versatile interface designed to streamline the entire analysis process. Users can start by defining specific project goals, such as enhancing engagement or driving sales. This enables the tool to pull out relevant themes automatically, highlighting key pain points and insights derived from the interviews.
Moreover, Tool 4 enables users to access detailed context around insights, ensuring that the findings are not just data points but stories that drive actionable conclusions. Notably, the ability to export findings into structured reports or presentations provides a seamless way to communicate results to stakeholders. This inclusive approach ensures that teams can trust the data, fostering confidence in the findings and supporting informed decision-making in their projects.
Steps to Conduct AI-powered Interview Analysis
To effectively conduct AI-powered interview analysis, several key steps need to be followed, ensuring streamlined processes and accurate insights. First, begin with data collection and preparation. This involves gathering necessary interview data from various sources and ensuring it is formatted consistently for easier processing. Data preprocessing is vital, as cleaning and structuring the information lay the groundwork for successful analysis.
๐ฌ Questions about How to Conduct Large-Scale Interview Analysis with AI?
Our team typically responds within minutes
Next, implement AI algorithms tailored to your research objectives. Select appropriate algorithms based on the type of analysis you wish to conduct, ensuring they suit your data characteristics. This may involve training and testing AI models on your dataset to evaluate their effectiveness before full-scale application. By following these steps, teams can enhance the consistency and speed of their interview analysis, smoothening the path toward actionable insights. The integration of AI tools can effectively combat manual biases and reduce bottlenecks in data interpretation.
Extract insights from interviews, calls, surveys and reviews for insights in minutes
Step 1: Data Collection and Preparation
Data collection and preparation are essential first steps in conducting AI-powered interview analysis. Begin by gathering data from various sources, including interviews, surveys, and user feedback. Organizing this data efficiently ensures a seamless transition to the analysis phase, allowing for better insights to be drawn from participant responses. Properly labeled datasets simplify the process of training AI algorithms later on.
Once the data is collected, the next task is preprocessing. This step may involve transcribing interviews, cleaning up text, and removing any irrelevant information. Error correction is crucial to improve the accuracy of AI interpretations. This foundational work sets the stage for the AI-powered analysis, ensuring that insights can be extracted effectively and meaningfully from the information provided. By prioritizing data quality and organization, you will enhance the overall effectiveness of your large-scale interview analysis.
- Techniques for gathering interview data.
Gathering interview data is fundamental for any qualitative analysis, especially within an AI-powered framework. First, define your research objectives to ensure that the questions asked during interviews are targeted and relevant. Develop a structured set of questions, considering both open-ended and closed-ended formats to capture diverse responses. Recording interviews, whether through audio or video, enhances accuracy and allows for deeper analysis in the future.
Second, ensure a varied selection of interview participants. This diversity can provide richer insights and minimize biases. Transcribing interviews accurately is crucial, as it serves as the primary dataset for AI-powered interview analysis. Once transcribed, code the data diligently by identifying recurring themes or keywords. Using quantitative methods, such as frequency analysis, can bring consistency and clarity to the interpretation of qualitative data. This systematic approach will help you harness AI more effectively for uncovering valuable insights.
- Preprocessing data for AI insights.
To extract valuable insights from interview data, preprocessing is vital. This step ensures that the information is clean, structured, and ready for analysis. Initially, raw interview data often contains noise, such as filler words and background chatter, which can mislead AI analysis. By employing techniques like transcription cleaning, one can eliminate irrelevant segments, helping to focus on meaningful dialogue.
Next, categorizing the data into themes is essential for AI-powered interview analysis. This involves identifying key topics and sentiments within the conversations. By using advanced tag or keyword systems, you can create a rich database that reflects the nuances of each interviewee's viewpoint. Once categorized, export the refined data into a suitable format for your AI algorithms. This thorough preparation enhances the accuracy of the insights generated, ultimately driving more informed decision-making processes.
Step 2: Implementing AI Algorithms
Implementing AI algorithms for interview analysis can significantly enhance the accuracy and speed of data interpretation. Start by selecting suitable algorithms that align with your objectives. Such algorithms can include natural language processing (NLP) models for understanding text and sentiment analysis tools to gauge emotional responses. This selection is crucial as it lays the groundwork for effective AI-powered interview analysis.
Once the algorithms are selected, the next step involves training and testing AI models. Utilize your prepared interview data to train these models, ensuring they learn to identify patterns and themes effectively. Testing the models on unseen data guarantees their reliability and accuracy. Over time, these models will refine their capabilities, leading to more precise insights and faster turnaround for your analysis. By following these steps, organizations can leverage AI to transform raw interview data into actionable insights, streamlining their decision-making processes.
- Selecting suitable algorithms for analysis.
When selecting suitable algorithms for AI-powered interview analysis, itโs crucial to align the algorithmโs capabilities with your research objectives. Start by assessing the characteristics of your data, such as size and complexity, to determine what method works best. For instance, natural language processing (NLP) algorithms excel in extracting themes and sentiments, making them ideal for textual interview data. These algorithms can process large volumes of unstructured text quickly, revealing insights that would be difficult to analyze manually.
Next, consider the specific outcomes you aim to achieve with the analysis. Different algorithms have unique strengths. For descriptive insights, clustering algorithms can group similar responses, while predictive algorithms can help identify trends over time. Itโs often beneficial to employ a combination of algorithms to enhance the depth of analysis. Begin with a trial-and-error approach, testing different models, and iterating based on the insights generated. This way, you can refine your approach to ensure that the AI-powered interview analysis yields actionable insights relevant to your goals.
- Training and testing AI models.
Training and testing AI models is a vital part of conducting AI-powered interview analysis. Initially, the right algorithms must be selected based on the specific objectives of the interview analysis. These algorithms can vary widely, from natural language processing techniques to deep learning models, each serving different analytical purposes.
Once the algorithms are selected, the actual training process begins. This involves feeding the AI model a plethora of interview data, allowing it to learn patterns, tones, and sentiments present in human conversations. After training, the next phase involves rigorous testing to ensure the model's accuracy and effectiveness. Testing helps identify potential biases and areas for improvement, ensuring that the AI effectively analyzes interviews with minimal errors.
In summary, a well-structured approach to training and testing AI models is essential for maximizing the benefits of AI-powered interview analysis. This process not only enhances model performance but also strengthens the reliability of insights drawn from interviews.
Conclusion on AI-powered Interview Analysis
AI-powered Interview Analysis transforms the way organizations conduct qualitative research by enhancing efficiency and accuracy. Traditional methods often involve cumbersome manual processes that are time-intensive, leading to inconsistencies in insights. With AI intervention, teams can quickly analyze large volumes of interview data, allowing for faster decision-making and more reliable results.
Moreover, these technologies reduce bias by standardizing the analysis process across diverse research teams. By adopting AI-powered Interview Analysis, organizations not only streamline their workflows but also gain a competitive edge through faster, more actionable insights. Embracing these tools is essential for adapting to the increasing demand for timely and trustworthy data interpretations in todayโs business landscape.
### Leveraging AI-powered Interview Analysis Tools
Choosing the right tools is crucial for an effective AI-powered interview analysis. First, one of the most prominent tools available is a platform designed specifically for large-scale interview analysis. This tool streamlines data collection, offering functionalities such as transcription and analysis visualization. Its user-friendly interface allows teams to easily navigate insights derived from interviews.
Next, there are additional tools worth considering. These can include those that focus on sentiment analysis or theme identification, which enhance the depth of qualitative analysis. Each tool should be selected based on specific project goals and desired outputs. Finally, combining these tools increases efficiency and provides a more comprehensive understanding of interview data, leading to actionable insights and better decision-making.
๐ฌ Questions about How to Conduct Large-Scale Interview Analysis with AI?
Our team typically responds within minutes