How to Code Open-Ended Feedback in Market Research Using AI
-
Bella Williams
- 10 min read
AI Feedback Analysis is transforming the landscape of market research by enabling companies to delve into open-ended customer feedback with unprecedented efficiency. In today's fast-paced business environment, gathering insights is crucial, as organizations grapple with mountains of qualitative data. By harnessing AI technologies, companies can streamline the analysis process and extract actionable insights that drive strategy and enhance customer experiences.
The importance of understanding customer sentiment cannot be overstated. Effective AI Feedback Analysis not only identifies emerging patterns in feedback but also allows businesses to make data-driven decisions. This section will explore innovative techniques and tools that empower organizations to convert open-ended responses into clear, interpretable information, ultimately enhancing their decision-making capabilities.
Discover how AI Feedback Analysis is revolutionizing the way we handle open-ended feedback in market research. Well explore key techniques and tools that empower businesses to gain deep insights from customer responses.
AI Feedback Analysis is changing the game in market research, particularly in how we process open-ended feedback. Traditionally, sifting through qualitative feedback has been time-consuming and challenging, often leading to overlooked insights. However, AI-powered tools are now empowering businesses to extract meaningful patterns from customer responses efficiently. By leveraging advanced algorithms, AI can categorize feedback into themes, highlighting key customer sentiments and suggestions.
Using specific techniques such as sentiment analysis and topic modeling, companies can identify what resonates most with their audience. Tools such as Insight7 and MonkeyLearn are leading the way, offering capabilities that not only streamline data analysis but also enhance accuracy. As businesses adopt these techniques, they open the door to deeper understanding and more powerful decision-making based on customer insights. This level of analysis ensures companies remain agile in responding to market demands, ultimately fostering improved customer experiences.
Analyze & Evaluate Calls. At Scale.
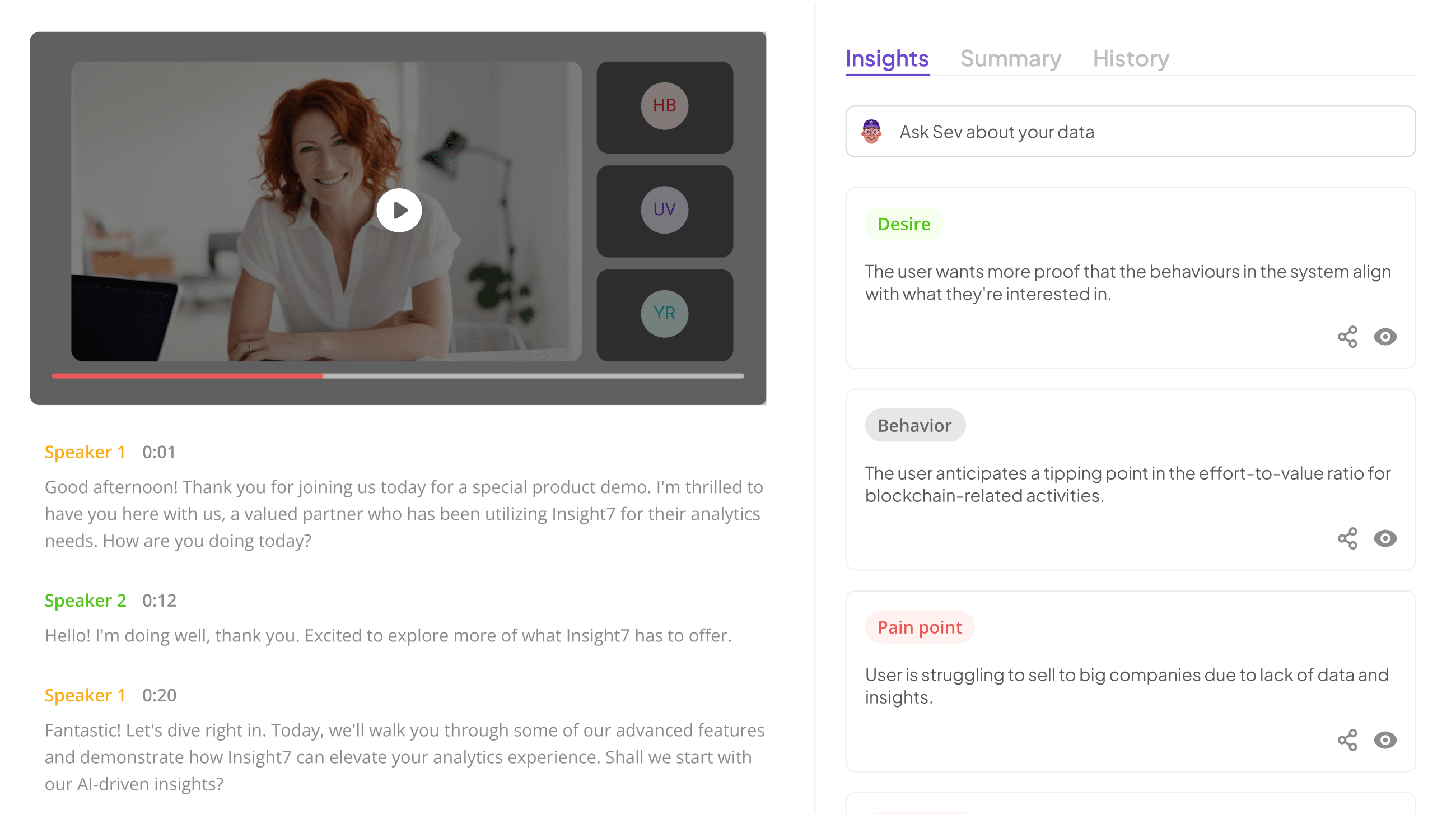
Leveraging AI Feedback Analysis for Market Research
AI Feedback Analysis offers a transformative approach to handling open-ended feedback in market research. By utilizing this technology, companies can streamline the tedious process of analyzing qualitative data. Traditional methods of manual coding are often inconsistent and time-consuming, leading to delays in generating actionable insights. Instead, AI automates the coding process, enabling researchers to focus on interpreting results rather than getting bogged down in data handling.
Implementing AI Feedback Analysis allows businesses to uncover patterns and sentiments that may otherwise go unnoticed. For instance, through advanced natural language processing, AI can identify key themes in customer responses more efficiently. This not only accelerates the timeline for generating reports but also enhances the accuracy and reliability of insights, making AI an essential tool for modern market research. Embracing these technologies can significantly improve decision-making processes and keep organizations competitive in their respective industries.
Learn how leveraging AI Feedback Analysis can streamline the processing of open-ended feedback in market research.
AI Feedback Analysis significantly enhances the efficiency of processing open-ended feedback in market research. Traditional methods often involve manual coding which can be labor-intensive and subject to biases. By integrating AI, businesses can automate this process, allowing teams to focus on deriving actionable insights rather than getting bogged down in data entry and interpretation.
One key benefit of AI Feedback Analysis is its ability to quickly identify themes and sentiments within large volumes of text. This rapid analysis reduces the time required to generate reports, meeting the increasing demand for timely insights from clients. Furthermore, the consistency and accuracy of AI models help to mitigate human errors, ensuring a reliable output that empowers organizations to make data-driven decisions. Embracing this technology not only streamlines operations but also enhances the overall quality of market research.
Understanding the Role of AI in Feedback Analysis
AI Feedback Analysis is fundamentally transforming how businesses uncover and interpret open-ended feedback. At its core, AI enables a more efficient and nuanced examination of customer sentiments, allowing researchers to process large volumes of qualitative data quickly. This capability is essential in modern market research, where real-time insights can dictate strategy and improve customer experiences.
One of the primary roles of AI in feedback analysis is its ability to identify patterns and themes within unstructured data. By utilizing natural language processing (NLP), AI systems can distill complex feedback into actionable insights. This allows businesses to respond swiftly to customer needs and adapt their offerings based on the collected feedback. Furthermore, AI minimizes human bias in interpreting responses, ensuring that insights reflect authentic customer experiences rather than subjective interpretations. As market research evolves, AI Feedback Analysis will remain a crucial element for businesses aiming to stay competitive and responsive to their customers.
Explore the fundamental role AI plays in analyzing open-ended feedback, and why it is essential for modern market research.
AI Feedback Analysis plays a pivotal role in interpreting open-ended feedback, making it indispensable for contemporary market research. As businesses increasingly rely on qualitative data, traditional manual analysis can be time-consuming and prone to bias. AI streamlines this process by automating the extraction of themes and sentiments from various responses, ensuring quick and accurate insights. This capability is particularly valuable for organizations with limited resources, allowing them to quickly generate reports without sacrificing quality.
Moreover, AI enhances consistency across research teams. Human researchers may interpret data differently, leading to varying insights. However, AI systems provide a uniform approach, resulting in more reliable findings. By leveraging advanced algorithms, businesses can dissect complex feedback and discover actionable insights confidently. As market demands rapidly evolve, integrating AI into feedback analysis ensures that organizations remain competitive and responsive to customer needs, ultimately driving strategic decisions based on precise data.
Case Study: AI Feedback Analysis in Action
AI Feedback Analysis is increasingly becoming a vital part of market research processes. In this case study, one organization faced common challenges when analyzing open-ended feedback from surveys and interviews. Their team was struggling with manual data processing, which slowed down report generation and introduced bias into their findings. To tackle this, they began using AI tools to automate the analysis of qualitative data.
The results were significant. By integrating AI feedback analysis, the organization could swiftly identify common themes and extract valuable insights from customer responses. This not only reduced the time spent on manual coding but also ensured more consistent interpretations across their team. As a result, the organization was able to deliver quicker reports, keeping clients satisfied and engaged. This case highlights how implementing AI in feedback analysis not only streamlines processes but also enhances the quality of insights derived from customer feedback.
Examine a real-world example of how businesses implement AI Feedback Analysis to unlock customer insights and drive strategic decisions.
Many businesses are now utilizing AI Feedback Analysis to turn customer feedback into actionable insights. For instance, by interviewing customers who chose not to continue using a service, businesses can tap into open-ended responses to uncover valuable insights. AI algorithms can process these qualitative responses, extracting themes and patterns that help identify key issues driving customer decisions.
In practice, AI Feedback Analysis allows companies to distill qualitative data into strategic directions, fostering a deeper understanding of customer sentiment. This analysis not only highlights areas needing improvement but also empowers businesses to make data-driven decisions. As customer expectations evolve, implementing AI-powered strategies becomes paramount for staying competitive and responsive to market demands. By harnessing AI insights, organizations can truly elevate their understanding of consumer behavior and streamline their strategic planning processes.
Extract insights from interviews, calls, surveys and reviews for insights in minutes
Tools and Techniques for Effective AI Feedback Analysis
Tools and techniques for effective AI feedback analysis play a critical role in enhancing the understanding of open-ended responses in market research. To maximize the potential of AI in this context, organizations should utilize a combination of advanced analytical tools and systematic methodologies. By embracing AI-powered platforms, researchers can efficiently process vast amounts of feedback, allowing for quicker and more reliable insights.
One significant technique involves selecting appropriate AI tools tailored for feedback analysis. For instance, platforms like insight7 and MonkeyLearn are excellent choices for qualitative data analysis and sentiment interpretation. Additionally, implementing a step-by-step coding approach can further refine the analysis process. First, ensure data collection and preparation are thorough. Next, select tools that align with the specific needs of the project. After applying AI models, interpreting insights accurately becomes paramount for making informed decisions. By employing these tools and techniques, organizations can enhance their AI feedback analysis, leading to actionable insights in market research.
Dive into the top tools and methodologies that facilitate the coding of open-ended feedback using AI.
The effective coding of open-ended feedback using AI hinges on several key tools and methodologies. Start by exploring notable AI-driven platforms that can simplify the analysis process. Among these, tools such as Insight7 and MonkeyLearn stand out for their user-friendly interfaces and robust features. Insight7 excels at qualitative data analysis, pulling actionable insights from open-ended responses. Similarly, MonkeyLearn specializes in sentiment analysis, helping researchers interpret customer feedback effortlessly.
In addition to these platforms, employing methodologies such as natural language processing and machine learning can enhance the accuracy of feedback analysis. Techniques like data preparation and iterative model training are essential for maximizing results. By understanding and harnessing these top tools and methodologies, businesses can streamline their open-ended feedback analysis effectively. Ultimately, utilizing AI Feedback Analysis not only accelerates the coding process but also provides deeper insights into customer sentiments, enabling informed decision-making.
Top AI Feedback Analysis Tools
AI Feedback Analysis is becoming increasingly vital in todayโs data-driven landscape. Organizations are turning to sophisticated tools designed to streamline the coding and interpretation of open-ended feedback. With these powerful solutions, teams can enhance the efficiency and accuracy of their feedback analysis processes. This creates opportunities for more insightful interpretations and quicker report generation, vital to meeting client demands.
Among the top AI Feedback Analysis tools, several stand out. Insight7 facilitates coding qualitative responses, providing actionable insights from open-ended feedback. MonkeyLearn offers intuitive AI-driven solutions for both sentiment analysis and the interpretation of nuanced feedback. Lexalytics specializes in text analytics and natural language processing, allowing users to decode complex customer responses effectively. RapidMiner provides a comprehensive platform tailored for data science teams to operationalize their AI models. Lastly, IBM Watson harnesses advanced machine learning capabilities to unlock valuable trends from intricate feedback data. By utilizing these tools, organizations can transform open-ended feedback into strategic insights, driving impactful decisions.
Discover the leading tools that enhance the efficiency and accuracy of feedback analysis, starting with insight7.
In the dynamic field of market research, feedback analysis is vital for understanding customer sentiment. Discovering the right tools can significantly enhance efficiency and accuracy, starting with advanced AI capabilities. One standout tool offers robust qualitative data analysis, allowing businesses to translate open-ended feedback into actionable insights swiftly. As organizations amass vast amounts of customer responses, efficient data processing becomes essential.
Other notable tools include platforms that excel in sentiment analysis and natural language processing. These tools automate the initial reading of transcripts, saving valuable time for researchers who juggle multiple priorities. By harnessing AI Feedback Analysis, businesses can transform cluttered qualitative data into clear, strategic decisions. Embracing these technologies will not only streamline feedback analysis but also empower organizations to make informed, data-driven choices that resonate with their customer base.
- insight7: Offers robust capabilities for qualitative data analysis, leveraging AI to draw actionable insights from open-ended responses.
In the realm of qualitative data analysis, insight7 stands out by harnessing the power of AI to transform open-ended feedback into actionable insights. It addresses common challenges faced by researchers, such as lengthy analysis times and inconsistencies among team members. By automating the coding process, insight7 not only reduces the manual workload but also enhances the accuracy of the insights drawn from interviews and surveys.
With its sophisticated algorithms, insight7 allows teams to quickly identify themes and trends in open-ended responses. This capability leads to faster reporting and timely decision-making, critical in todayโs fast-paced market environment. The platform excels in summarizing complex data, helping researchers make sense of vast amounts of feedback without the bias and variation inherent in manual coding. Ultimately, leveraging AI Feedback Analysis with insight7 empowers organizations to drive effective strategies based on robust consumer insights.
- MonkeyLearn: Provides user-friendly AI-driven solutions for sentiment analysis and feedback interpretation.
AI Feedback Analysis is at the forefront of modern market research, allowing businesses to effectively harness customer insights from open-ended feedback. Among the user-friendly AI-driven solutions available, one notable option excels in providing intuitive tools for sentiment analysis and feedback interpretation. Organizations can easily process intricate data, converting qualitative comments into actionable insights. This technology simplifies understanding customer emotions, enabling teams to respond strategically to consumer needs.
Utilizing these AI tools, businesses can streamline their analysis process. They can automatically categorize sentiments, identifying key themes and trends within customer feedback. By facilitating detailed reports, teams can visualize patterns, ultimately leading to informed decision-making. This method not only saves time but also enhances the quality of insights. In an era where data is abundant and often overwhelming, employing AI feedback analysis allows companies to transform raw data into meaningful suggestions that drive growth and innovation.
- Lexalytics: Specializes in text analytics and natural language processing to decode customer feedback effectively.
AI Feedback Analysis plays a pivotal role in modernizing how businesses understand customer sentiments. Utilizing sophisticated text analytics and natural language processing, organizations can effectively decode the nuances of open-ended feedback. This technology allows companies to quickly process large volumes of qualitative data, uncovering hidden themes and insights that inform decision-making.
With this analytical approach, businesses can streamline their feedback collection process. It starts with capturing customer responses, followed by the application of AI models to extract key themes and sentiments. As a result, companies can gain actionable insights, swiftly transforming raw feedback into strategic initiatives. As the landscape of market research evolves, embracing advanced technologies for AI Feedback Analysis empowers organizations to cultivate deeper customer relationships and enhance their market positioning.
- RapidMiner: Delivers a comprehensive platform for data science teams to operationalize AI feedback models.
RapidMiner offers a dynamic platform designed to enhance the capabilities of data science teams in operationalizing AI feedback models. This platform integrates diverse tools and functionalities, empowering users to efficiently manage and analyze open-ended survey responses. When feedback data is coded with AI, it transforms raw insights into actionable strategies.
Moreover, RapidMiner simplifies complex processes by providing user-friendly interfaces and robust analytics capabilities. It allows teams to visualize data, identify trends, and derive meaningful conclusions from customer responses. By utilizing such a platform, organizations can significantly improve their market research efforts through strategic decision-making based on real data insights. The ability to operationalize AI feedback models leads to improved efficiency and faster insights, enabling teams to focus more on strategy rather than tedious data management. The shift toward automated feedback analysis is essential for staying competitive in today's fast-paced market environment.
- IBM Watson: Utilizes advanced machine learning to parse complex feedback data and reveal underlying trends.
Advanced machine learning has significantly transformed how businesses interpret complex feedback data, driving more effective marketing strategies. This particular technology excels in extracting nuanced insights from open-ended responses, allowing organizations to pinpoint underlying trends that could go unnoticed through traditional methods. By employing algorithms that analyze the text, it's possible to reveal patterns and sentiments that inform product development, customer service improvements, and overall brand messaging.
The AI Feedback Analysis process ultimately enables companies to decipher vast amounts of qualitative data swiftly and accurately. As a result, businesses gain clarity on customer pain points, preferences, and expectations. Moreover, the capacity to generate structured reports or visual presentations enhances collaboration among teams, allowing them to act decisively based on reliable insights. Such capabilities not only streamline operations but also foster a deeper understanding of target markets, ensuring that businesses remain competitive and responsive to consumer needs.
Step-by-Step Approach to Coding Open-Ended Feedback
To effectively code open-ended feedback, itโs crucial to follow a structured step-by-step approach. Start by collecting and preparing your data, ensuring all responses are organized and ready for analysis. Preparation can significantly influence the effectiveness of your coding process, as clean and well-structured data leads to more accurate insights.
Next, select the right AI tools tailored for feedback analysis. Tools like insight7 or MonkeyLearn can improve the efficiency of the coding process, automating many of the tedious aspects associated with qualitative data. After choosing your tools, implement your chosen AI models to begin analyzing the feedback systematically. Remember, this is where AI Feedback Analysis truly shines, parsing through large volumes of data to uncover valuable patterns. Lastly, carefully interpret the insights gained from your analysis. Analyzing these findings can yield actionable steps to enhance your strategy, transforming raw feedback into strategic market advantages.
Uncover a structured approach to effectively code and interpret open-ended feedback using AI technologies.
In the journey of coding open-ended feedback, a structured approach is crucial for unlocking deeper insights. Begin with Data Collection and Preparation, where raw feedback is gathered, ensuring that it's well-organized and formatted for analysis. This initial stage sets the groundwork for effective AI Feedback Analysis, as clean data is essential for accurate interpretation.
Next, move to Selecting the Right AI Tools. The right tools can streamline your process, enabling efficient coding and interpretation of the data. Tools like insight7, MonkeyLearn, and IBM Watson are pivotal in transforming qualitative feedback into actionable insights. Following tool selection, Implementing AI Models for Feedback Analysis becomes the focal point. Utilizing advanced algorithms, AI can identify themes, sentiments, and patterns within responses, offering clarity to complex data sets. Finally, in the Interpreting Insights and Taking Action step, businesses can harness AI-driven findings to inform strategic decision-making, ultimately leading to enhanced customer satisfaction and engagement.
- Step 1: Data Collection and Preparation
Effective data collection and preparation are crucial initial steps in AI Feedback Analysis. Begin by gathering open-ended feedback from various sources, such as surveys, interviews, or social media. This ensures a diverse and comprehensive dataset that captures varying perspectives. It's essential to standardize the collected data, as inconsistencies can hinder the analysis process. Consider organizing responses into manageable formats for further processing, such as spreadsheets or databases.
Once the data is collected, the preparation phase includes transcription and cleaning. Accurate transcription of audio or written responses is vital, ensuring that the subsequent AI analysis is based on reliable information. After transcription, remove any extraneous details, such as typos or irrelevant comments, to provide a clear dataset. This meticulous preparation establishes a firm foundation for deeper insights, ultimately allowing AI to reveal valuable themes and trends in customer feedback. Thus, a well-planned data collection and preparation stage is indispensable for leveraging AI Feedback Analysis effectively.
- Step 2: Selecting the Right AI Tools
Choosing the right AI tools for feedback analysis can significantly enhance the quality and depth of your market research. Begin by evaluating your specific needs, as different AI platforms offer unique functionalities tailored to various aspects of data analysis. Not all tools provide the same capabilities, so understanding what you require is critical for effective outcomes.
Once you've outlined your needs, explore the following categories of AI tools. First, consider solutions specializing in text analytics, such as Lexalytics, which can effectively decipher customer sentiments. Next, look into platforms like MonkeyLearn for user-friendly interfaces that simplify sentiment analysis and feedback interpretation. Finally, explore comprehensive data science platforms like RapidMiner, which allows for operationalizing AI models to derive actionable insights from your data.
Selecting the right AI tools is essential for capturing valuable insights from open-ended feedback, thus driving informed decision-making in your market research strategies.
- Step 3: Implementing AI Models for Feedback Analysis
Implementing AI models for feedback analysis begins with selecting the right algorithms tailored for open-ended responses. This step leverages AI feedback analysis to unlock valuable insights from qualitative data. Begin by preprocessing your data, which involves cleaning, tokenizing, and organizing feedback into manageable formats. This foundational work is crucial for the algorithms to process the information accurately and effectively.
Next, choose a model that aligns with your specific analytical needs. Various models, such as natural language processing and machine learning algorithms, can discern themes within the data. Train these models using annotated datasets to improve their accuracy in identifying sentiments and trends. After training, implement the models to analyze actual feedback responses and generate reports. Regularly evaluate the model's performance and refine it as new data comes in. This iterative process ensures your AI feedback analysis remains relevant and effective, ultimately enhancing your market research outcomes.
- Step 4: Interpreting Insights and Taking Action
To effectively interpret insights derived from AI Feedback Analysis, itโs crucial to synthesize the data into actionable strategies. Begin by identifying key themes and sentiments that emerge from the qualitative data. These themes, clustered around core customer experiences, help you pinpoint opportunities and challenges. For example, if a recurring theme is the desire for better user collaboration tools, this indicates a significant avenue for product enhancement or innovation.
Once themes are established, prioritize them based on their relevance to your overall business goals. This prioritization ensures that resources are allocated efficiently towards areas that promise the highest impact. Implementing changes based on these insights not only demonstrates responsiveness to customer feedback but also fosters loyalty and engagement. Regularly revisiting and analyzing newly collected feedback ensures continuous improvement and adaptation to evolving customer needs. By combining effective coding with clear strategies, organizations can translate AI-generated insights into meaningful actions.
Conclusion: Transforming Market Research with AI Feedback Analysis
The integration of AI Feedback Analysis marks a turning point in market research methodologies. This transformative approach enables researchers to quickly process open-ended feedback, significantly reducing analysis time and improving accuracy. As traditional methods often introduce bias and inconsistency, AI offers a more reliable alternative that can streamline the reporting process. By employing AI, businesses can gain actionable insights faster, thus meeting the demands of clients who require timely and relevant data.
Moreover, AI Feedback Analysis equips researchers with the tools necessary to tackle complex qualitative data. By shifting from manual coding to automated analysis, organizations will be able to focus more on interpreting the results and implementing strategic initiatives. Embracing these capabilities not only enhances efficiency but also positions companies to better understand and respond to consumer needs in a rapidly changing market.
In conclusion, AI Feedback Analysis is transforming market research by providing unprecedented insights into consumer sentiment. Embrace these advanced tools and techniques to stay ahead in a competitive marketplace.
AI Feedback Analysis is changing the game in market research by uncovering valuable insights into consumer sentiment. With the vast amount of open-ended feedback received today, traditional methods may fall short. However, by utilizing advanced AI techniques, businesses can efficiently process and interpret this data, leading to a deeper understanding of customer emotions and preferences.
Embracing AI Feedback Analysis not only enhances research accuracy but also cultivates a competitive edge. As market dynamics evolve, leveraging these advanced tools is essential for businesses looking to adapt and thrive. By integrating AI-driven insights, organizations can make informed decisions that resonate with their target audience, ultimately positioning themselves as industry leaders.