How to Build QA Benchmarks From Historical Performance Data
-
Hello Insight
- 10 min read
Performance Data Benchmarking serves as a cornerstone for effective Quality Assurance (QA) processes. Understanding historical performance data allows organizations to evaluate their success and identify areas for improvement. By analyzing this data, teams can develop insights that not only enhance current practices but also shape future QA initiatives.
Establishing a solid foundation in Performance Data Benchmarking is essential. It equips QA teams with the necessary insights to measure their work against industry standards. Furthermore, it fosters a culture of continuous improvement, enabling organizations to refine their processes and outcomes effectively. By committing to this approach, businesses can drive exceptional quality and performance across their services.
Analyze & Evaluate Calls. At Scale.
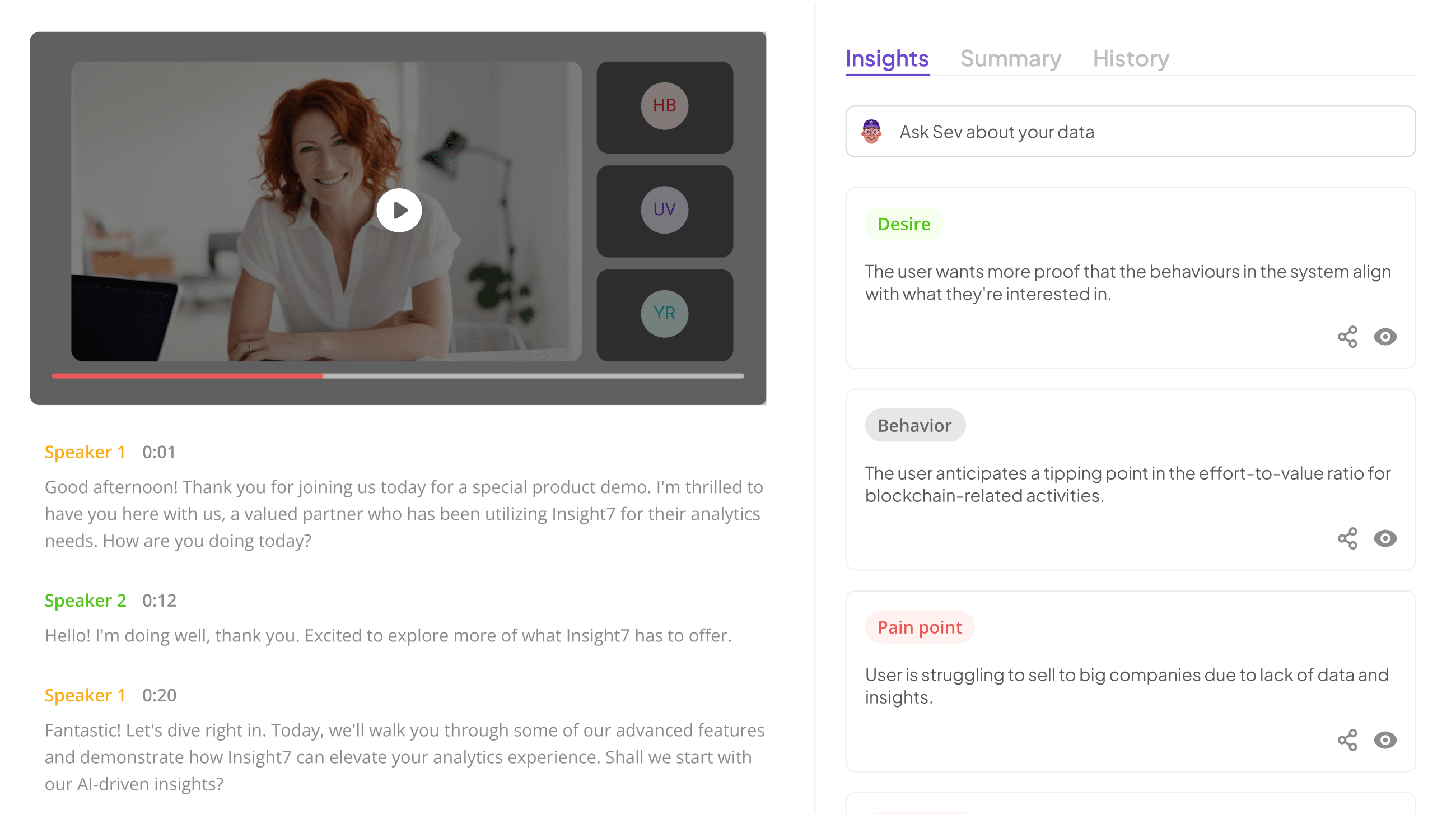
In this opening section, provide an overview of what Performance Data Benchmarking entails and why its crucial for Quality Assurance (QA) processes within organizations.
Performance Data Benchmarking refers to the systematic process of comparing an organization's performance metrics against industry standards or historical data. This practice involves collecting, analyzing, and interpreting various performance indicators to gauge the effectiveness and efficiency of operational processes. By establishing benchmarks, organizations create reference points that guide decision-making and facilitate continuous improvement.
In Quality Assurance (QA) processes, Performance Data Benchmarking serves as a crucial tool for ensuring product and service quality. It enables organizations to identify areas of underperformance and implement targeted strategies for enhancement. Furthermore, assessing historical performance data helps establish realistic expectations, fostering accountability within teams. As a result, maintaining high-quality standards not only boosts customer satisfaction but also strengthens the overall organizational reputation.
Steps to Initiate Performance Data Benchmarking in QA
To initiate Performance Data Benchmarking in QA, begin by identifying relevant historical data. It’s crucial to select data sources that provide accurate insights into your organization's QA processes. Consider qualitative metrics, such as customer feedback, alongside quantitative data like error rates and resolution times. This multifaceted approach will offer a comprehensive view, allowing for effective comparative analysis.
Next, analyze past performance trends. Look for patterns or anomalies in your historical data. Identify periods of significant improvements or declines and correlate these with specific QA practices or changes in procedures. This analysis will not only reveal the strengths and weaknesses of your current QA methods but will also help in formulating strategic benchmarks that align with your organization's goals. By understanding these trends, you can create actionable insights for enhancing overall quality assurance processes.
Identify Relevant Historical Data
To build effective QA benchmarks, it is crucial to identify relevant historical data that will inform your analysis. Start by gathering performance data from previous projects, focusing on metrics that directly correlate with success indicators relevant to your organization’s objectives. Historical performance data can provide invaluable insights into past achievements, thus serving as a foundation for setting future benchmarks.
When analyzing this data, consider key factors such as the project scope, duration, and results. Identify the types of performance metrics that have previously driven improvements or flagged issues. By concentrating on this specific data, you create a clearer picture of performance trends and areas that require focus. This method not only enhances the accuracy of performance data benchmarking but also ensures that future benchmarks are grounded in reality and aligned with organizational objectives.
Discuss the importance of selecting the right data and the criteria to consider for meaningful analysis.
Selecting the right data is crucial for effective performance data benchmarking. The right data ensures that analysis drives meaningful insights, helping organizations make informed decisions for quality assurance. When choosing data, it's essential to consider its relevance, accuracy, and comprehensiveness. Data that reflects the specific objectives and scenarios faced by your QA processes will yield more applicable insights.
Moreover, data selection criteria should include timeliness—ensuring the data captures current trends and customer experiences. Additionally, consider the diversity of data sources to create a well-rounded perspective. Integrating qualitative insights alongside quantitative metrics can enhance the overall understanding of performance. By prioritizing these factors in data selection, organizations can foster a culture of continuous improvement in their QA practices, ultimately resulting in better alignment with customer needs and expectations.
Analyze Past Performance Trends
Analyzing past performance trends is vital for identifying consistent patterns in data. By examining historical performance, organizations can uncover significant insights that reveal areas of improvement or decline. This analysis allows teams to understand fluctuations in quality and efficiency over time, fostering informed decision-making for future enhancements.
The first step in analyzing these trends is to collect relevant historical data, ensuring it is accurate and comprehensive. Then, utilize tools to visualize this data, making it easier to spot anomalies and trends. Organizations can categorize performance metrics by time frames, which aids in recognizing seasonal patterns and shifts in user feedback. This systematic approach to performance data benchmarking helps teams establish precise and relevant benchmarks that align with their quality assurance goals. Through thorough analysis, organizations can weave a narrative from their historical data, transforming insights into actionable strategies for improved performance.
Explain how to examine historical trends to gain insights into performance improvements or declines.
To examine historical trends effectively, it is essential to first gather relevant performance data that spans a significant timeframe. Start by organizing data points in a way that highlights fluctuations in performance metrics over time. This could include system response times, error rates, or customer satisfaction scores. By analyzing this information, one can pinpoint specific periods of improvement or decline and understand the factors influencing these changes.
Next, utilize visualizations such as graphs or charts to represent the data. This visual representation aids in identifying patterns, such as recurring issues or peak performance periods. Additionally, comparing data trends against external benchmarks can provide valuable context. Recognizing seasonal variations or the impact of significant events on performance metrics allows teams to derive actionable insights and improve service quality. Ultimately, a robust analysis of historical trends in performance data benchmarking helps in strategizing future improvements in QA processes.
Extract insights from interviews, calls, surveys and reviews for insights in minutes
Building QA Benchmarks from Historical Performance Data
Building QA benchmarks from historical performance data involves systematically analyzing past performance to establish realistic and achievable standards. This process begins with defining benchmark goals that directly tie into organizational objectives, ensuring the benchmarks serve a meaningful purpose. Key considerations include specific areas for improvement and historical contexts that provide a framework for goal-setting. Establishing these clear goals is fundamental, as they lay the groundwork for comprehensive performance data benchmarking.
Next, implementing a robust benchmarking framework is essential for applying these standards effectively. This framework should incorporate methodologies tailored to your organization's needs, focusing on consistency and reliability in measurement. Utilizing various tools is critical in this phase, as they provide necessary insights and enable ongoing adjustments. By leveraging historical performance data, organizations can not only track progress but also create a culture of continuous improvement in their QA processes.
Defining Benchmark Goals
Defining benchmark goals is a pivotal step in the performance data benchmarking process. It begins with establishing clear, realistic objectives that align with your organization’s strategic vision. This process requires an understanding of current performance metrics, as well as insight into the desired outcomes. When you have a solid grasp of where you currently stand, you can then determine where you aim to be, ensuring that your benchmarks are both challenging and attainable.
To effectively set these goals, consider factors such as past performance data, market standards, and internal capabilities. Establishing a timeline for reevaluation is also crucial, as this allows you to adapt your benchmarks based on evolving data and objectives. This iterative approach ensures that your benchmarks remain relevant and can foster continuous improvement within your QA processes. By defining these goals, you create a structured pathway for measuring success and achieving operational excellence.
Describe how to set realistic and attainable benchmarks that align with organizational objectives.
Setting realistic and attainable benchmarks that align with organizational objectives is essential for effective performance data benchmarking in Quality Assurance (QA). First, it’s crucial to clearly define what success looks like. Engage stakeholders to understand their perspectives and requirements, ensuring that benchmarks reflect both current capabilities and aspirational goals. This collaboration promotes a sense of ownership, enhancing commitment toward achieving these benchmarks.
Next, assess historical performance data to establish achievable targets. Look for trends and patterns that reveal historical highs and lows, which can guide the setting of new expectations. Choose metrics that are specific, measurable, attainable, relevant, and time-bound (SMART). Incorporating these elements helps maintain alignment with organizational objectives while driving continual improvement. Finally, revisit these benchmarks periodically to adapt them as necessary, ensuring they remain both realistic and aligned with evolving business needs.
Implementing Benchmarking Frameworks
Implementing Benchmarking Frameworks requires a holistic approach to understand how your Quality Assurance (QA) initiatives can benefit from historical performance data. The first step is defining clear and measurable benchmarks that reflect your organization’s objectives. Establishing these benchmarks enables teams to set expectations and creates a foundation for continuous improvement.
Once benchmarking goals are defined, various methodologies can be employed to implement these frameworks effectively. You can utilize quantitative analysis to compare current performance against established benchmarks. Additionally, integrating feedback loops—gathering insights from customer interactions and service performance—serves as a crucial mechanism for ongoing refinement. Implementing these frameworks ensures that your QA processes align with both operational efficiency and customer satisfaction, ultimately driving better performance outcomes.
Detail the framework or methodologies that can be used to apply these benchmarks effectively.
When applying benchmarks effectively, organizations should consider a structured approach that incorporates various methodologies. First, establish a clear understanding of the objectives behind the benchmarking effort. It is essential to define what metrics are most relevant to your organization and how they align with overall goals. By focusing on specific performance indicators, teams can tailor their analysis, making it more effective and actionable.
Next, employing methodologies such as continuous improvement and root cause analysis can enhance the benchmarking process. Continuous improvement encourages regular evaluation of performance data, allowing organizations to adapt their benchmarks as needed. Root cause analysis helps identify underlying issues that may be affecting performance metrics. Incorporating these methodologies ensures that performance data benchmarking not only tracks past outcomes but also guides future enhancements, fostering a culture of ongoing improvement.
Tools for Performance Data Benchmarking
Selecting appropriate tools is essential for effective Performance Data Benchmarking. Various tools can assist in analyzing and comparing past performance to set reliable benchmarks in Quality Assurance. Understanding the capabilities of these tools allows organizations to make data-driven decisions that enhance their processes.
1. insight7: This platform stands out with its user-friendly interface and comprehensive reporting features. It simplifies the benchmarking process, integrating historical data to provide actionable insights which can significantly improve QA practices.
2. Apache JMeter: Known for its versatility, JMeter excels at measuring performance across different applications. Its broad functionality allows users to simulate various scenarios, providing valuable insights into load testing and performance evaluation.
3. HP LoadRunner: Ideal for large enterprises, LoadRunner facilitates extensive testing and benchmarking for complex applications. It enables detailed analysis that supports high-stakes decisions in performance management.
4. Gatling: This tool is developer-centric, focusing on ease of use for building performance tests quickly. Gatling’s advanced scripting capabilities foster efficient testing environments, delivering swift and continuous feedback.
5. Neoload: Effective for intricate application scenarios, Neoload supports extensive testing options while ensuring seamless integration with CI/CD pipelines. This ability fosters consistency in performance testing, critical for modern development practices.
Using these tools effectively can transform the way organizations approach Performance Data Benchmarking, ensuring precise measurements and continuous improvement in QA processes.
insight7
Performance Data Benchmarking is an essential process that helps teams assess their quality assurance efforts against historical performance data. When developing a benchmarking strategy, begin by clearly defining your goals. These benchmarks should not only reflect past performance but also align with current organizational objectives. Tailoring these benchmarks enables your team to set realistic targets that drive quality improvements.
Next, consider implementing a structured framework to evaluate performance data more effectively. One popular approach is to categorize historical data according to relevant metrics, allowing for a streamlined comparison. By employing analytical tools, teams can identify patterns and trends that indicate areas for improvement. It's vital to ensure that the historical data considered is comprehensive and relevant, providing a solid foundation for informed decision-making. This data-driven approach ultimately leads to actionable insights, which enhance QA processes and foster a culture of continuous improvement.
Start with a description of insight7, its features, and how it excels in benchmarking tasks.
Insight7 is an intuitive platform designed for effective Performance Data Benchmarking, enabling organizations to glean valuable insights from their historical performance data effortlessly. Central to its functionality is an easy-to-navigate interface, which allows users of any skill level to access the platform and utilize it without extensive training. Users can seamlessly manage various tasks—from transcribing calls to compiling comprehensive reports for business reviews—enhancing overall efficiency in their benchmarking efforts.
The platform excels in its ability to analyze multiple calls concurrently, extracting key insights that inform decision-making processes. Through features like automated reports and call analysis capabilities, Insight7 identifies pain points and customer sentiments, providing critical evidence to back up findings. This data-driven approach not only simplifies the benchmarking process, but also empowers teams to make informed, strategic decisions based on historical performance trends, facilitating continuous improvement in quality assurance processes.
Additional Tools
To enhance your Performance Data Benchmarking efforts, various additional tools can streamline your analysis and improve outcomes. The choice of tools often depends on the specific requirements of your projects and the nature of the data you are working with. By integrating the right tools, teams can efficiently gauge performance metrics and adjust strategies accordingly.
1. Apache JMeter: This robust tool specializes in measuring and analyzing application performance. It allows users to create and execute performance tests, providing critical insights into how applications behave under various load conditions.
2. HP LoadRunner: A leading choice for enterprises, LoadRunner offers comprehensive solutions for load testing and performance benchmarking. Its powerful analytics help teams understand system behavior and can highlight areas for improvement.
3. Gatling: Designed with developers in mind, Gatling provides a lightweight performance testing framework. Its scripting capabilities allow for detailed and meticulous performance testing, making it easier to integrate into the development lifecycle.
4. Neoload: Focused on complex applications, Neoload effectively simulates user traffic to assess application performance. This tool is ideal for organizations looking to measure response times accurately and ensure quality under heavy loads.
Each of these tools contributes significantly to building reliable QA benchmarks from historical data, aiding in informed decision-making and strategic planning. By incorporating a mix of these resources, QA teams can enhance their performance data benchmarking initiatives.
- Apache JMeter: Highlight its capabilities in performance measurement.
Apache JMeter is a powerful tool that excels in performance measurement, making it invaluable for organizations focusing on performance data benchmarking. It enables testers to simulate a variety of scenarios, including incoming requests to web applications, by generating load on servers. This capability allows teams to assess how applications respond under stress and identify potential bottlenecks. Furthermore, JMeter supports various protocols, providing a comprehensive framework for testing beyond just web applications.
Additionally, JMeter offers easy-to-read reports featuring graphs and charts that help visualize performance metrics. This visualization is crucial for understanding historical data trends and evaluating the impact of changes on benchmark performance scores. With its ability to integrate seamlessly with other tools and frameworks, Apache JMeter stands as a critical asset in building quality assurance benchmarks from historical performance data. By effectively utilizing JMeter, organizations can ensure their applications deliver optimal performance, even in demanding conditions.
- HP LoadRunner: Discuss its utility in enterprise-level benchmarking.
HP LoadRunner serves as a vital tool in enterprise-level benchmarking, especially when it comes to evaluating performance data. It enables organizations to simulate user interactions, allowing them to understand application behavior under various load conditions. By generating realistic traffic patterns, it supports accurate performance testing, which is crucial for identifying bottlenecks in systems and ensuring smooth operational efficiency.
One of the key utilities of LoadRunner lies in its comprehensive reporting capabilities. It offers insights into system performance over time, which aids organizations in establishing meaningful benchmarks. By comparing current performance against historical data, teams can assess improvements and make data-driven decisions. Furthermore, integrating HP LoadRunner into the enterprise's testing framework enhances the overall quality assurance process, ensuring applications can handle expected demands efficiently. This focus on Performance Data Benchmarking ultimately drives better user experiences and organizational success.
- Gatling: Explain its focus on developer-centric performance testing.
Gatling stands out in the realm of performance testing by placing a strong emphasis on developer-centric approaches. This tool is designed to integrate seamlessly into the development process, making it easier for developers to implement performance tests alongside their coding tasks. By focusing on scenarios that mimic real user behaviors and providing easy-to-read reports, Gatling enables developers to identify performance bottlenecks early in the development cycle.
Moreover, Gatling's scripting capabilities ensure that performance data benchmarking is accessible to those familiar with frontend technologies. Developers can write tests using a domain-specific language that feels intuitive and aligns with their existing workflows. In doing so, it fosters a culture of performance awareness, encouraging teams to continuously monitor performance metrics and make informed decisions based on historical performance data. By integrating performance testing directly into the development pipeline, Gatling enables robust applications that are both efficient and user-friendly.
- Neoload: Describe its effectiveness in complex application performance testing.
Neoload proves highly effective for complex application performance testing, providing a robust solution tailored to handle the intricacies of various applications. By simulating real-world user traffic, it helps QA teams identify bottlenecks and optimize system performance. This capability is particularly beneficial for organizations managing diverse and intricate user interactions, as it enables precise measurement against historical performance data.
Moreover, Neoload enhances performance data benchmarking by integrating comprehensive analytics tools. These tools allow stakeholders to visualize performance trends over time, fostering informed decision-making. The platform's ability to support different protocols and environments ensures it can adapt to any testing scenario, offering granular insights into application responsiveness. By employing Neoload, teams not only validate their benchmarks but also establish a reliable framework for ongoing performance optimization efforts, ensuring their applications consistently meet user expectations.
Conclusion: Maximizing QA through Performance Data Benchmarking
Implementing Performance Data Benchmarking in Quality Assurance (QA) processes is essential for organizations aiming to enhance their overall service quality. By leveraging historical performance data, companies can identify trends, measure improvements, and set realistic expectations. This practice not only provides a roadmap for continuous improvement but also aligns QA efforts with broader organizational goals.
In conclusion, maximizing QA through Performance Data Benchmarking creates a solid foundation for decision-making. By continuously analyzing historical data, organizations can adjust their strategies, ensuring they keep pace with changing customer needs and industry standards. Ultimately, this commitment to data-driven insights fosters a culture of excellence and reliability within QA teams, leading to sustainable success.
Bring together the main points and discuss the long-term benefits of implementing a robust Performance Data Benchmarking strategy in QA processes.
Implementing a robust Performance Data Benchmarking strategy in Quality Assurance processes offers significant long-term benefits. First, this strategy enables organizations to identify performance trends and discrepancies over time, allowing for continuous improvement in their QA procedures. By comparing historical performance data against established benchmarks, teams can pinpoint areas for enhancement and allocate resources more effectively, ensuring ongoing optimization.
Moreover, the integration of Performance Data Benchmarking fosters a culture of accountability and transparency within QA teams. This approach encourages collaboration as team members work towards shared goals, ultimately enhancing overall product quality. Additionally, long-term adoption of benchmarking practices leads to greater consistency in testing outcomes, making it easier to maintain quality across various releases. In conclusion, a well-implemented Performance Data Benchmarking strategy not only drives operational efficiency but also contributes to sustainable success in organizational quality assurance efforts.