In today's fast-paced digital landscape, the importance of efficient quality assurance (QA) workflows cannot be overstated. An AI-driven QA workflow emerges as a game changer, enabling teams to streamline processes, enhance accuracy, and efficiently handle increased volumes of data. Adopting AI tools not only speeds up evaluations but also aids in pinpointing critical insights hidden within extensive datasets.
Transitioning to an AI-driven QA workflow requires a strategic integration of existing tools with new technologies. By carefully selecting AI applications tailored to specific QA tasks, organizations can elevate their quality assurance standards significantly. This structured approach not only combats the challenges faced by traditional QA processes but also profits from AI's transformative potential, setting the stage for delivering superior outcomes in quality management.
Analyze qualitative data. At Scale.
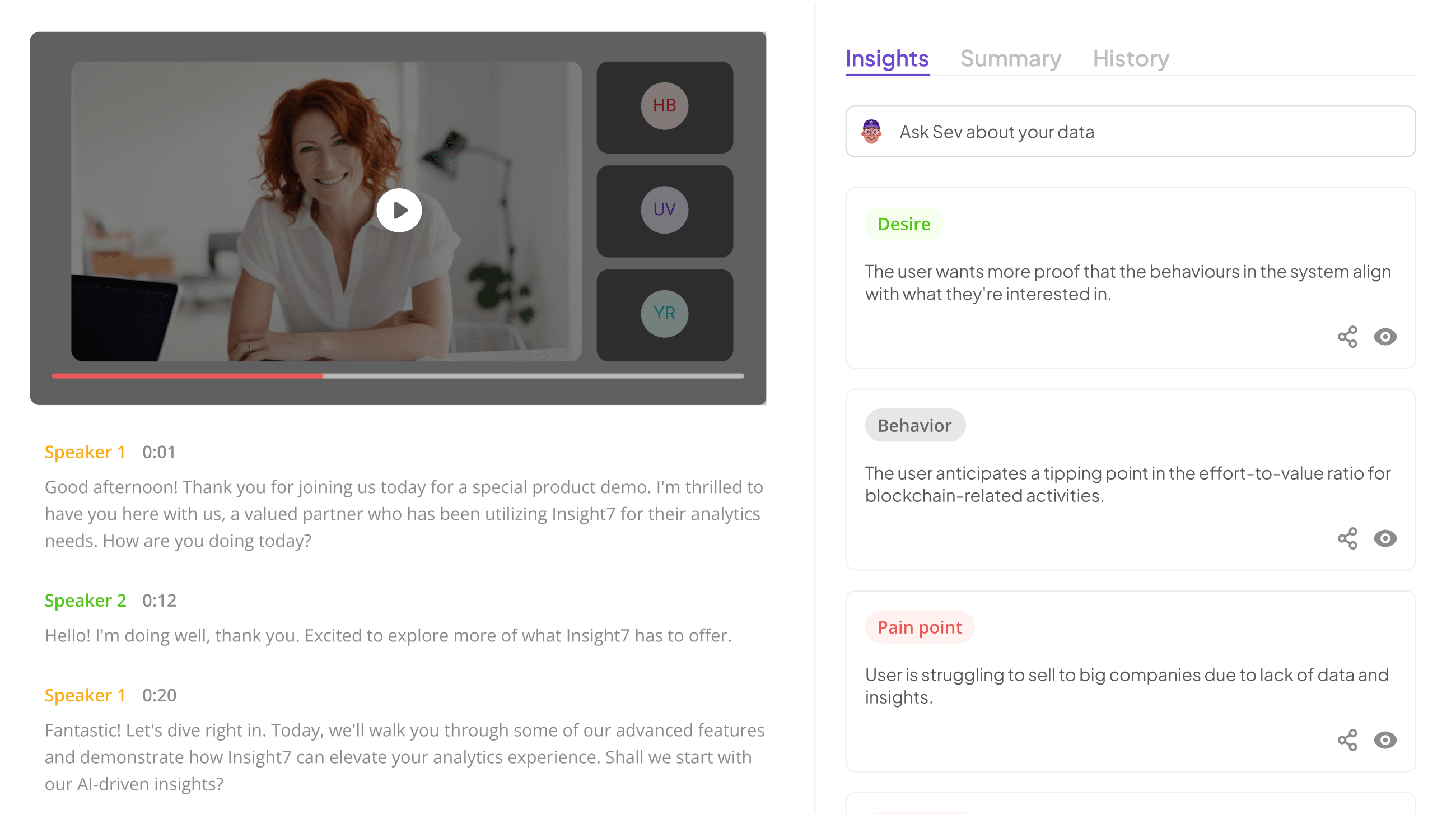
Understanding the Need for an AI-Driven QA Workflow
In today's fast-paced digital landscape, understanding the need for an AI-Driven QA Workflow is essential for organizations aiming to enhance product quality and streamline operations. Traditional quality assurance methods often face challenges such as inefficiency and inconsistency. Manual evaluations can lead to human error, resulting in missed issues that could have significant repercussions on customer satisfaction and compliance. An AI-Driven QA Workflow addresses these challenges by automating repetitive tasks, allowing teams to focus on more complex evaluations and decision-making processes.
Moreover, integrating AI into QA workflows provides robust data analysis capabilities. With AI tools capable of transcribing, analyzing, and generating reports from numerous interactions at scale, organizations can harness insights much faster than conventional methods allow. Such enhancements not only improve the accuracy of evaluations but also ensure that standards are consistently met. Therefore, adopting an AI-driven approach is no longer a luxury but a necessity for businesses striving to compete effectively and efficiently in their respective markets.
Challenges in Traditional QA Processes
Traditional quality assurance (QA) processes face several significant challenges that can hinder efficiency and accuracy. Firstly, manual testing is often time-consuming and prone to human error. Each test case requires substantial attention, which can lead to overlooked details and inconsistencies that affect product quality. Moreover, traditional methods struggle to keep up with rapid software development, leaving QA teams racing against tight deadlines.
Another challenge is the lack of integration among testing tools, resulting in siloed information that complicates data analysis. When various stages of QA are not connected, teams find it difficult to gather insights, making it hard to identify root causes of issues. This step can slow down decision-making and result in subpar product performance. In this context, embracing an AI-driven QA workflow can alleviate these challenges by automating tasks, streamlining processes, and enhancing collaboration.
The Role of AI in Transforming QA
AI-Driven QA Workflow represents a significant shift in quality assurance practices. Traditional QA processes often suffer from inefficiencies, human error, and time constraints, making them less reliable. By incorporating AI into these workflows, organizations can automate repetitive tasks and enhance the accuracy of testing processes. This transformation allows teams to focus on strategic initiatives rather than getting bogged down in manual tasks.
Moreover, AI technologies can analyze large datasets swiftly, identifying patterns and anomalies that human testers might overlook. This capability not only accelerates the testing phase but also ensures a higher standard of quality in deliverables. As organizations seek to adapt to fast-paced market demands, adopting AI-driven solutions in QA becomes essential for maintaining competitiveness. The future of quality assurance lies in embracing AI, which not only streamlines operations but also fosters innovation, ultimately leading to improved products and customer satisfaction.
Essential Tools for Building an AI-Driven QA Workflow
Building an AI-Driven QA Workflow necessitates the right tools to streamline processes and enhance efficiency. First, integrating platforms like insight7 enables comprehensive data management by recording, transcribing, and analyzing calls effortlessly. This allows QA teams to evaluate customer interactions against predefined templates, addressing compliance and quality assurance in real-time. These foundational functionalities ensure quality checks are both accurate and thorough.
In addition to insight7, several other tools significantly enhance an AI-Driven QA Workflow. For instance, ChatGPT aids in dynamic test script generation, helping automate repetitive tasks. Testim offers features for automated testing that boost speed without compromising reliability. Using Applitools facilitates effective visual AI testing to ensure user interfaces are visually appealing and functional. Finally, Selenium remains a robust choice for web application testing due to its versatility and comprehensive support for various programming languages. Collectively, these essential tools form the backbone of a successful AI-Driven QA Workflow.
Extract insights from interviews, calls, surveys and reviews for insights in minutes
insight7: Leveraging Comprehensive Insights
In the context of building an AI-Driven QA Workflow, gaining comprehensive insights is crucial. By understanding data from customer interactions, organizations can significantly enhance their quality assurance processes. This involves not just collecting data but also effectively analyzing it to derive actionable insights. When these insights are integrated into workflows, teams can make more informed decisions, ultimately improving product quality and customer satisfaction.
Additionally, defining specific objectives helps in aligning insights with business goals. Insights gathered from customer feedback or performance analytics can directly inform testing strategies. This approach enables teams to prioritize issues that impact users most, leading to faster responses and more efficient cooperation among departments. Embracing a comprehensive insights strategy within your AI-Driven QA Workflow can act as a game-changer in refining processes and achieving competitive advantage.
Additional AI Tools for QA Optimization
In the realm of QA optimization, additional AI tools play a critical role in refining processes and enhancing productivity. These tools can provide automation, insights, and predictive capabilities that traditional methods often lack. By integrating solutions like ChatGPT for dynamic test script generation or Testim for automated testing, teams can streamline their QA efforts. Each tool brings unique strengths to the table, allowing for customized workflows tailored to specific project needs.
For instance, Applitools offers cutting-edge visual AI testing, ensuring that UI elements appear as intended across devices. Similarly, Selenium remains a robust choice for web application testing, providing flexibility and support for various programming languages. Embracing these AI-driven tools not only transforms the QA workflow but also empowers teams to focus on strategic improvements. By making informed decisions about which tools to adopt, organizations can achieve a more efficient and accurate QA process.
- ChatGPT for Dynamic Test Script Generation
Dynamic test script generation using ChatGPT can significantly enhance the quality assurance (QA) workflow. By harnessing AI's natural language processing capabilities, you can generate test scripts tailored to specific application needs. This allows teams to create and modify scripts quickly, reducing manual effort while increasing accuracy. Through analyses of input requirements, ChatGPT crafts scripts based on contextual understanding, enabling testers to keep pace with rapid development cycles.
Moreover, dynamic test script generation improves collaboration among team members. Developers, testers, and product owners can easily communicate their requirements, ensuring the generated scripts align with expectations. This integration not only fosters efficiency but also minimizes the risk of human error. When employing AI-driven tools in your QA workflow, you empower your team to focus on more strategic initiatives while enhancing the overall quality of software products.
- Testim for Automated Testing
Testim offers an innovative approach to automated testing that aligns perfectly with the concepts of an AI-driven QA workflow. By enabling teams to create, manage, and execute tests with minimal manual effort, Testim streamlines the QA process significantly. Teams can use its AI capabilities to generate scripts automatically, adapt to changes in the application, and maintain robust test suites without a steep learning curve.
The flexibility of Testim allows it to integrate seamlessly with existing tools in your workflow. Its ability to leverage AI not only enhances testing efficiency but also enables quick insights into application quality. As a result, teams can detect issues earlier and make informed decisions, ultimately contributing to faster product releases. By incorporating Testim into your automated testing strategy, you can effectively create a dynamic QA environment that evolves with your development needs.
- Applitools for Visual AI Testing
In an AI-Driven QA Workflow, visual testing plays a crucial role in ensuring that applications meet user expectations. Applitools provides a powerful solution that automates visual testing by using advanced AI algorithms to identify visual discrepancies between expected and actual results. This helps QA teams quickly spot issues that traditional testing methods might miss, such as layout shifts or color changes.
Incorporating Applitools into your QA strategy transforms how testing is conducted. The tool allows teams to run automated visual tests across various devices and browsers, ensuring a consistent user experience. With its integration capabilities, Applitools fits seamlessly into existing workflows, enhancing collaboration between developers and QA engineers. Ultimately, adopting visual AI testing not only improves efficiency but also elevates the quality of your software products by delivering an impeccable visual experience to users.
- Selenium for Web Application Testing
Selenium for web application testing plays a crucial role in an AI-driven QA workflow. This open-source framework enables testers to write scripts in various programming languages like Java, Python, and C#. Its versatility allows for testing across multiple browsers, making it a favorite among quality assurance professionals. By integrating Selenium into an AI-driven QA workflow, organizations can automate repetitive tasks and focus on more complex testing scenarios, ultimately enhancing testing efficiency and reducing time-to-market.
Furthermore, Selenium's compatibility with AI tools enhances its capabilities. For instance, leveraging machine learning algorithms can help optimize test scripts by predicting likely failure points in an application. This predictive analysis allows testers to be proactive rather than reactive, making the QA process smoother and more reliable. As teams adopt Selenium within their AI-driven workflows, they can expect improved test accuracy and faster feedback loops, fostering a more robust development and release cycle.
Step-by-Step Guide to Implementing an AI-Enhanced QA Workflow
Implementing an AI-Enhanced QA Workflow begins with identifying essential QA processes that can benefit from automation. Start by examining existing workflows to pinpoint tasks that are repetitive and time-consuming. For instance, if call evaluations are taking significant time, consider integrating AI tools that streamline this process, analyze data effectively, and generate actionable insights.
Next, the integration of AI tools into your established workflows is crucial. By using solutions like automatic transcription and data analysis software, quality assurance can become more efficient. Ensure that your team receives thorough training on these new technologies. Familiarity with tools enhances their ability to extract insights and adapt to ongoing changes. Your AI-driven QA workflow can foster a more responsive and accurate validation process, ultimately improving overall quality assurance outcomes and team productivity.
Step 1: Identifying Key QA Processes for Automation
To embark on your journey toward an AI-Driven QA Workflow, the first essential step involves identifying key QA processes ripe for automation. Start by analyzing your existing quality assurance activities and pinpointing tasks that are time-consuming or repetitive. Focus particularly on processes that require consistent accuracy, such as data collection, evaluation of call transcripts, or compliance checks. These areas often benefit significantly from automation, allowing human resources to concentrate on more strategic quality activities.
Once you identify which processes to automate, prioritize them based on factors like complexity and potential impact. Assess the potential for improvement through AI tools, ensuring you're aligning your resources with the most pressing needs of your team. This methodical approach not only enhances efficiency but also sets a solid foundation for integrating advanced AI solutions later in your workflow. In this way, recognizing key QA processes for automation becomes a critical step in enhancing your overall quality assurance efforts.
Step 2: Integration of AI Tools into Existing Workflows
Integrating AI tools into existing workflows is crucial in developing an AI-Driven QA Workflow. First, analyze your current processes to pinpoint where AI can add significant value. Automation of repetitive tasks, like test case generation and bug detection, enhances efficiency while freeing up your team to focus on critical tasks. It is essential to ensure that these AI tools align with your existing systems to avoid friction and confusion.
Next, adopt a phased approach for integration. Start with pilot projects that involve smaller teams, allowing you to gather feedback and make adjustments before a company-wide rollout. This enables a smoother transition and ensures that team members become familiar with the AI tools gradually. Engage your QA team in discussions about how these AI tools can augment their work, fostering a collaborative environment that embraces innovation. Ultimately, the goal is to create a seamless workflow that leverages AI capabilities while enhancing overall productivity.
Step 3: Training Teams on AI Tools and Technologies
Training teams on AI tools and technologies is crucial for successfully developing an AI-Driven QA Workflow. It is essential to equip your QA teams with the necessary skills to utilize these innovative tools effectively. Start by identifying the specific AI tools that will be integrated into your existing workflow, ensuring that each team member understands their functionalities.
Next, organize hands-on training sessions that emphasize practical application. These sessions should include real-world examples and scenarios tailored to the team's daily work. Encourage collaborative learning by allowing team members to share their insights and experiences with AI technologies. Offer ongoing support and updates as technology evolves, helping team members stay informed about the latest advancements. By fostering a culture of continuous learning, your team will be more adept at implementing an AI-Driven QA Workflow, ultimately enhancing productivity and outcomes.
Conclusion: The Future of QA with AI-Driven Workflows
The evolution of quality assurance through AI-driven workflows marks a significant shift in how we approach testing and validation. As organizations adapt to this transformation, they can expect enhanced accuracy, efficiency, and speed in their QA processes. By integrating AI technologies into existing workflows, teams can automate repetitive tasks and focus on more strategic problem-solving activities.
Looking ahead, AI-driven QA workflows will likely become standard practice, enabling teams to deliver high-quality products consistently. The future promises more intuitive tools and processes, ensuring that organizations remain agile and responsive to market demands. Embracing this change will empower teams to elevate their performance and improve overall customer satisfaction.