How to Build a Future-Resilient Evaluation System With AI Integration
-
Hello Insight
- 10 min read
AI-Enhanced Evaluation Resilience introduces a transformative approach to evaluation systems, emphasizing the necessity for organizations to adapt dynamically to changing circumstances. By harnessing the capabilities of artificial intelligence, we can significantly bolster the resilience of evaluation processes, making them more efficient and insightful.
In a world where data-driven decision-making is paramount, integrating AI allows for more robust evaluations that not only streamline processes but also enhance the quality of insights gained. This integration goes beyond mere automation; it fosters a culture of continuous improvement, ensuring organizations remain agile and responsive to ever-evolving needs. Embracing AI-Enhanced Evaluation Resilience is not just about surviving today’s challenges, but thriving in an unpredictable future.
Analyze & Evaluate Calls. At Scale.
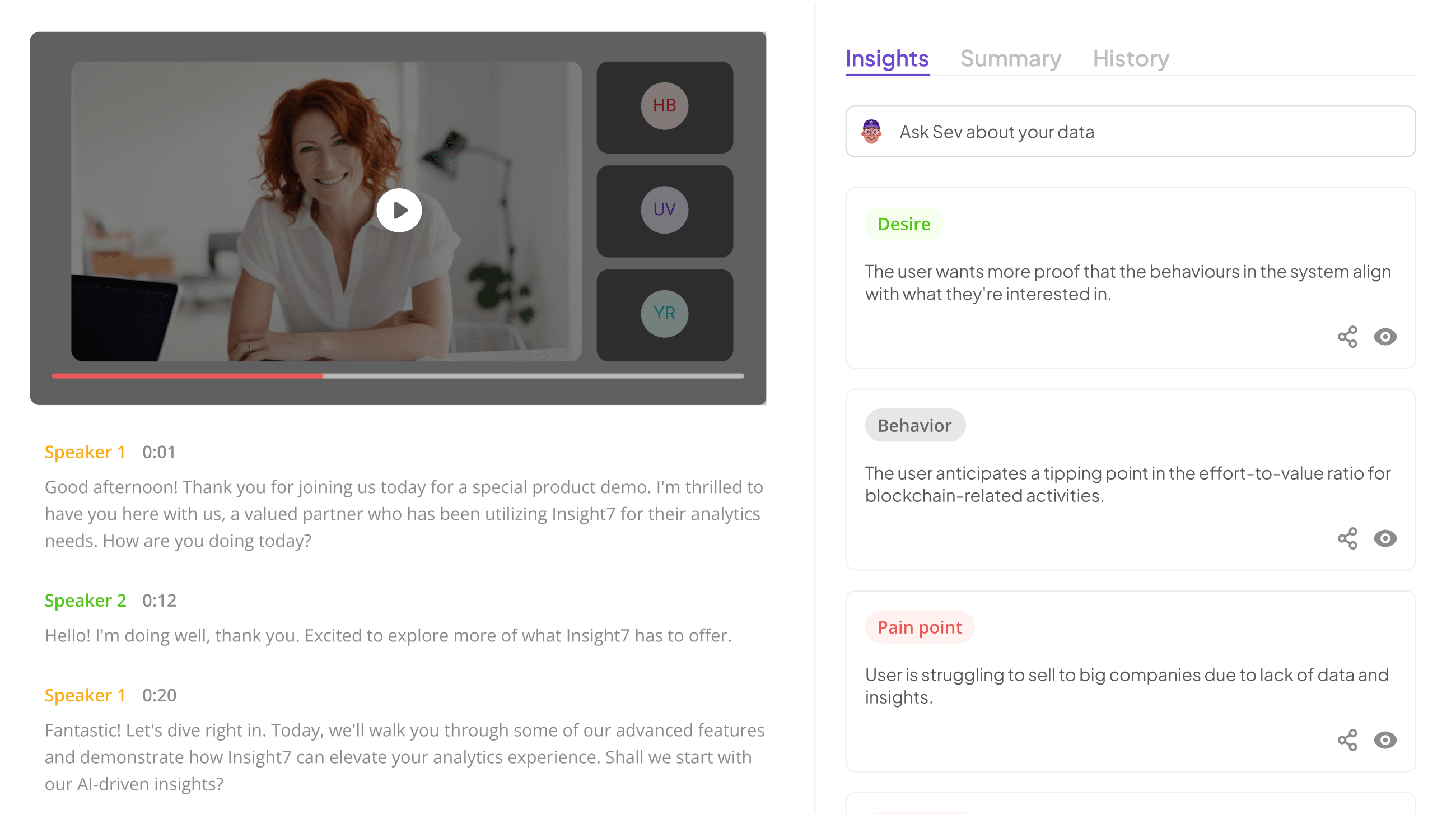
In todays fast-paced world, creating a future-resilient evaluation system is crucial to maintaining organizational adaptability. By integrating AI, we can enhance the resilience and efficiency of evaluation processes. This blog post will explore how to build such a system with AI integration.
In today’s fast-paced world, developing a future-resilient evaluation system is vital for ensuring organizational adaptability. The constant evolution of market dynamics demands that swift adjustments in evaluation processes keep pace. Integrating AI into these systems can significantly enhance resilience and efficiency, allowing organizations to respond effectively to changes. A well-structured evaluation system equipped with AI can analyze varied data points and provide insightful recommendations in real-time, ensuring timely decision-making.
To build such a robust system, organizations should focus on two main components. First, identifying key evaluation metrics tailored to specific objectives is essential. Second, harnessing data effectively allows for comprehensive analyses and insightful outcomes. By optimizing these elements with AI, evaluation resilience is bolstered, ultimately enabling organizations to tackle future challenges confidently. This blog post will guide you through the crucial steps to integrate AI into your evaluation processes, ensuring your organization remains agile and informed.
Components of an AI-Enhanced Evaluation Resilience System
An AI-Enhanced Evaluation Resilience System comprises several integral components designed to create a robust framework for assessments. First, identifying key evaluation metrics is crucial. These metrics are tailored to organizational goals and provide insight into performance, enabling dynamic decision-making and continuous improvement. Understanding these metrics allows an organization to effectively measure its success and identify areas requiring attention.
Another essential component involves harnessing data for enhanced resilience. Utilizing diverse datasets enhances the accuracy of AI-driven evaluations and supports informed decision-making. Organizations must focus on cleansing, managing, and sourcing quality data, as integrity is fundamental to insightful analysis. Moreover, choosing the right AI tools facilitates the integration of technology into existing evaluation frameworks, ensuring a seamless transition. This strategic combination of metrics and data management empowers organizations to cultivate a strong AI-Enhanced Evaluation Resilience that withstands future challenges.
Identifying Key Evaluation Metrics
Identifying key evaluation metrics is a cornerstone of creating an AI-enhanced evaluation resilience system. Start by assessing the specific goals of your organization, as these will guide the selection of metrics. Consider both quantitative measures, like performance ratings and call completion rates, and qualitative insights derived from user feedback. An effective metric system should balance numerical data with personal insights to provide a holistic view of performance.
Next, focus on aligning these metrics with predictive analytics capabilities. Identify which metrics are most predictive of success in achieving your goals. This alignment allows you to gain deeper insights and make informed decisions based on data trends. Regularly review and adapt your metrics to ensure they reflect changing organizational needs and external environments. By maintaining an agile approach to metrics, you ensure your evaluation system remains resilient and responsive to future challenges.
Understanding the critical metrics that drive your organizations success is essential for developing an AI-enhanced evaluation system.
Identifying the critical metrics that drive success is paramount in developing an AI-enhanced evaluation system. These metrics serve as the foundation for understanding organizational goals and performance. By focusing on specific indicators, organizations can harness data effectively to improve decision-making and adaptability. Regular analysis of these metrics allows for timely adjustments and strategic pivots, ensuring that evaluation systems remain relevant.
To implement AI-enhanced evaluation resilience, consider the following key metrics: performance indicators, customer satisfaction scores, and operational efficiency ratings. Performance indicators provide insight into team outputs and productivity levels. Customer satisfaction scores reflect how well the organization meets client needs, while operational efficiency ratings assess the effectiveness of processes. By monitoring these areas, organizations can utilize AI capabilities to predict trends, enhance service delivery, and strengthen overall resilience in a rapidly evolving landscape.
Leveraging Data for Enhanced Resilience
Data holds the key to creating a resilient evaluation system, particularly when enhanced by AI. Organizations generate vast amounts of information every day, but simply collecting it is not enough. Properly analyzing and interpreting this data leads to actionable insights that can significantly impact decision-making and strategic planning. By focusing on the quality and relevance of data, businesses can ensure that they derive maximum value from the insights gathered.
To truly enhance resilience, organizations should prioritize several areas. First, the collection process must be efficient, ensuring that data is accurate and relevant. Second, implementing robust data management practices allows for better organization and accessibility of information. Finally, regularly revisiting and refining the evaluation metrics based on new data ensures that the system remains responsive to changing circumstances. Each of these components, when combined, contributes to achieving AI-enhanced evaluation resilience, equipping organizations to navigate future challenges successfully and maintain a competitive edge.
Harnessing the power of data is vital to support AI-driven evaluations. This includes sourcing, cleansing, and managing significant datasets for accurate analysis.
Data is at the heart of effective AI-driven evaluations. To harness this power, it is essential to focus on sourcing, cleansing, and managing substantial datasets, ensuring that analysis is accurate and reliable. First, sourcing quality data involves identifying relevant information from diverse channels, including internal systems and external sources. This extensive approach ensures a well-rounded dataset that reflects the actual dynamics at play.
Once data is sourced, cleansing becomes crucial in removing inaccuracies and inconsistencies. This process helps to enhance the integrity of the data so that conclusions drawn from it are sound. Following cleansing, effective management of the datasets allows for seamless integration and analysis, which is vital for sustaining an AI-enhanced evaluation resilience. Ultimately, this meticulous attention to data handling not only informs better decision-making but also reinforces the organization’s adaptability in a rapidly changing environment. By prioritizing these aspects, organizations can create a robust foundation for successful AI-driven evaluations.
Extract insights from interviews, calls, surveys and reviews for insights in minutes
Implementing AI-Enhanced Evaluation Resilience
Implementing AI-Enhanced Evaluation Resilience begins with a clear plan to select suitable AI tools that match your evaluation objectives. Choosing the right technologies is crucial, as they must seamlessly integrate with existing frameworks. This integration allows for streamlined processes and improved performance metrics, enabling more accurate assessments across various criteria.
Next, once the tools are in place, organizations should focus on the implementation phase. This involves training staff to utilize these AI capabilities effectively, fostering a culture of adaptability and continuous learning. By implementing structured data management practices, teams can ensure the data fed into AI systems is reliable and relevant. This attention to detail not only enhances evaluation efficiency but also strengthens decision-making processes, paving the way for a resilient evaluation framework that can withstand future challenges.
In these steps, the essence of AI-Enhanced Evaluation Resilience lies in thoughtful planning, dedicated training, and strategic data usage.
Step 1: Choosing the Right AI Tools
When embarking on the journey to build a future-resilient evaluation system, the first critical step is choosing the right AI tools. These tools are the backbone of AI-enhanced evaluation resilience, influencing how data is gathered, processed, and analyzed. Begin by assessing your organization’s specific evaluation goals and requirements. This clarity will help guide your tool selection process and ensure alignment with desired outcomes.
Next, explore various AI tools that cater to those needs. Essential options include MonkeyLearn, RapidMiner, IBM Watson Analytics, and H2O.ai. Each has distinct features that can enhance evaluation capabilities, from text analysis to predictive modeling. Assess their usability, integration potential, and support resources. Furthermore, training staff on these tools is crucial for maximizing their effectiveness. The right AI tools can elevate your evaluation system, making it not only resilient but also capable of adapting to future challenges.
Before integrating AI, its essential to select tools that align with your organizations evaluation goals. Insight7 leads the way, followed by other tools:
Selecting the right tools is a crucial first step before integrating AI into your evaluation processes. Insight7 is at the forefront of these tools, designed to optimize data analysis and uncover actionable insights. This platform enables organizations to analyze customer interactions at scale, streamlining the decision-making process. Beyond Insight7, other options such as MonkeyLearn and IBM Watson Analytics can also enhance data evaluation capabilities.
Choosing the appropriate tools that align with your organization's evaluation goals is essential for achieving AI-Enhanced Evaluation Resilience. Each tool offers unique features, so assessing their functionalities, usability, and integration compatibility is vital. This strategic selection process ensures that the technology implemented will effectively support your evaluation objectives. Ultimately, the right tools will empower your organization to make informed decisions based on well-analyzed data, driving sustainable growth and adaptability in an ever-evolving landscape.
- MonkeyLearn
Integrating an effective AI-enhanced evaluation resilience system can significantly improve how organizations assess their operations. One key tool that facilitates this process focuses on understanding and analyzing large volumes of data. This system incorporates machine learning capabilities that allow users to classify and extract valuable insights from evaluation metrics efficiently.
By utilizing AI technology, organizations can conduct thorough analyses of customer interactions and agent performance. This methodology not only enhances accuracy but also provides actionable insights for ongoing training and development. Consequently, businesses can foster a culture of continual improvement, ensuring that their evaluation strategies remain relevant and robust amid evolving market demands. Engaging with such innovative solutions empowers organizations to build a future-resilient framework that promotes adaptability and informed decision-making.
- RapidMiner
Incorporating advanced AI tools is essential for creating an evaluation system that can withstand future challenges. Among these tools, one platform stands out for its user-friendly interface and robust capabilities. It simplifies project management by allowing users to upload files, organize data, and analyze results simultaneously. By quickly extracting insights from a variety of data sources, it empowers teams to visualize historical queries and identify trends effectively.
This platform enhances the concept of AI-Enhanced Evaluation Resilience by streamlining the evaluation process. Projects created within the system enable precise data analysis, where teams can effortlessly summarize customer feedback and extract meaningful patterns. The key features include data integration from multiple sources, easy deadline management, and comprehensive reporting. These attributes collectively bolster the resilience of evaluation systems, ensuring that organizations remain agile and adaptive to evolving needs and challenges.
- IBM Watson Analytics
IBM Watson Analytics offers powerful capabilities that support the development of a resilient evaluation system enhanced by artificial intelligence. This tool allows organizations to explore and analyze data effectively, facilitating better decision-making processes. Users can generate comprehensive insights into customer behaviors, trends, and feedback. By employing advanced visualization techniques, teams can uncover significant patterns, paving the way for data-driven strategies.
Integrating this technology provides several advantages, such as improved data management and streamlined analysis workflows. With features like natural language processing, it enables users to interact intuitively with their data, making querying more accessible. Moreover, as organizations scale their evaluation efforts, utilizing this tool can help optimize their data strategies and improve the overall robustness of AI-enhanced evaluation resilience. Transitioning existing evaluation systems to include such powerful analytics fosters a future-ready approach, ensuring adaptability in a rapidly evolving landscape.
- H2O.ai
In the realm of AI-enhanced evaluation resilience, the journey begins with selecting sophisticated tools that empower organizations. The right tools play a pivotal role in transforming evaluation processes and ensuring adaptability. A particularly noteworthy option offers robust capabilities for managing and analyzing complex data, which is essential for meaningful insights. Utilizing this tool allows organizations to seamlessly integrate advanced AI functionalities into their existing evaluation frameworks.
Furthermore, focusing on customization enhances the user experience. Organizations can adapt configurations to meet specific compliance and performance metrics. This degree of flexibility fosters an environment where teams can efficiently navigate evaluation tasks and derive actionable insights. By making evaluation systems future-resilient, organizations position themselves to respond dynamically to evolving demands, ensuring that their evaluation processes not only maintain relevance but also drive continuous improvement. This proactive approach is vital for sustaining efficiency and effectiveness in evaluation practices.
Step 2: Integrating AI into Existing Systems
Integrating AI into existing systems begins with understanding how current evaluation frameworks operate. The goal is to enhance these frameworks without disrupting the established processes. Start by mapping out the existing workflows and identifying areas where AI can contribute, such as improving data collection and analysis efficiency. This critical step ensures a smooth transition to an AI-enhanced evaluation resilience system.
Once integration points are identified, training and upskilling staff become essential. Personnel should be equipped with the knowledge to leverage AI tools effectively while maintaining oversight of their decisions. Engaging team members in this transition fosters a culture of adaptability. By prioritizing clear communication and support, the integration can enhance evaluation resilience, preparing the organization to respond to unforeseen changes and challenges with agility and confidence.
Once tools are chosen, the next step is to integrate AI within your established evaluation frameworks. This includes aligning AI functionalities with existing processes and training staff accordingly.
Once the right AI tools are selected, the opportunity for integration arises. The next critical step is embedding these tools into existing evaluation frameworks to maximize their utility. This integration entails aligning AI functionalities with your established processes, ensuring they complement rather than disrupt current workflows. By assessing how AI capabilities can enhance evaluation metrics, you can achieve a more nuanced understanding of their potential impact.
Furthermore, successful integration depends on effectively training staff. Training empowers team members to leverage AI tools effectively, fostering confidence and competence in the new processes introduced. When employees understand how to utilize AI within their roles, they can drive the organization towards enhanced evaluation resilience. Collaborating with teams to create tailored training programs will facilitate a smoother transition, ensuring everyone is on board with the new AI-enhanced evaluation system. This thoughtful approach will not only transform how evaluations are conducted but also enhance overall organizational adaptability in an evolving landscape.
Conclusion: Achieving AI-Enhanced Evaluation Resilience
Achieving AI-Enhanced Evaluation Resilience is not merely an endpoint; it is an ongoing journey. Organizations must continually assess and adapt their evaluation frameworks, ensuring that AI technologies are seamlessly integrated into their operations. Through this process, resilience is built by prioritizing specificity in evaluation criteria, thereby minimizing errors and enhancing decision-making.
Moreover, the collaboration between human expertise and AI capabilities will lay the foundation for more dynamic evaluations. By fostering a culture of continuous learning and improvement, organizations can fortify their evaluation systems against future uncertainties. Thus, AI-Enhanced Evaluation Resilience stands as a vital component in the quest for sustainable success.
In conclusion, building a future-resilient evaluation system requires careful planning and strategic integration of AI technologies. By selecting the right tools and aligning AI capabilities with organizational goals, organizations can ensure that their evaluation systems remain robust and adaptable in the face of future challenges.
Creating a future-resilient evaluation system hinges on proactive planning and the precise application of AI technologies. This entails selecting tools that not only fit the specific needs of the evaluation process but also align with broader organizational objectives. By doing so, an AI-enhanced evaluation resilience can be achieved, equipping the system to withstand diverse future challenges effectively.
Strategically integrating AI into existing frameworks will further solidify this foundation. Continuous training and adaptability are key components that help enhance the system's efficacy. Emphasizing these aspects ensures that the evaluation system remains not only relevant but also evolves alongside changing demands and expectations.