Voice Sentiment Mapping is an innovative approach that harnesses the power of voice data to gauge customer emotions and sentiments. As businesses strive to enhance customer experiences, understanding the nuances in vocal tones and expressions becomes increasingly essential. By analyzing voice interactions, organizations can uncover valuable insights that drive better decision-making and improve overall service quality.
In this section, we will explore the fundamentals of Voice Sentiment Mapping, highlighting its significance in creating meaningful customer sentiment heatmaps. Delving into the methods of analyzing voice data not only enriches our understanding of customer opinions but also informs strategies for effective engagement. As we navigate this topic, the connections between voice insights and actionable improvements will become clear, paving the way for enhanced customer relationships.
Analyze qualitative data. At Scale.
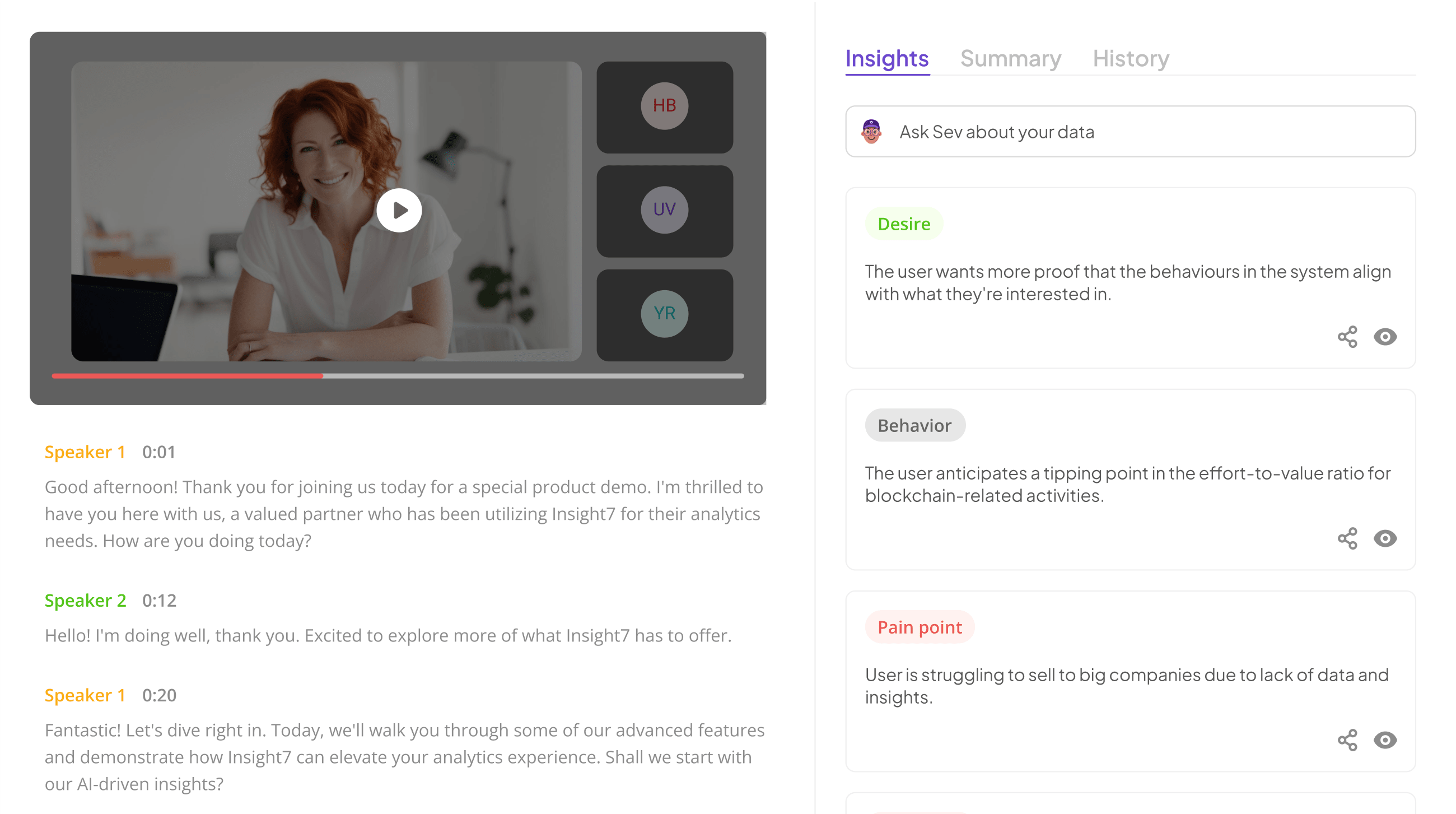
Understanding Voice Sentiment Mapping for Heatmap Creation
Voice Sentiment Mapping serves as a crucial tool in extracting meaningful insights from voice data. By analyzing vocal tones, speech patterns, and language choice, businesses can discern customer feelings and opinions. This process helps to categorize sentiments as positive, negative, or neutral, forming the foundation for visual representations like heatmaps.
To effectively create heatmaps from voice data, several key components come into play. First, understanding the emotional nuances within spoken language is essentialโthis includes tone, pace, and inflections. Second, gathering and preparing high-quality voice data allows for accurate sentiment extraction. Finally, visualizing these sentiments on a heatmap highlights trends and patterns in customer feedback, enabling organizations to make informed decisions based on customer experiences. By mastering Voice Sentiment Mapping, businesses can transform raw voice data into actionable insights, leading to improved customer satisfaction.
The Importance of Voice Sentiment Mapping in Customer Insights
Voice Sentiment Mapping plays a crucial role in understanding customer insights by providing a bridge between emotions expressed in conversations and actionable business strategies. By analyzing voice data, businesses can gather real-time feedback, pinpoint customer emotions, and identify patterns that reveal their preferences and concerns. This comprehension allows organizations to adapt their offerings and improve customer engagement effectively.
Furthermore, Voice Sentiment Mapping enhances the decision-making process. When companies harness insights gleaned from voice data, they not only gain clarity on customer sentiments but also strengthen their competitive edge. Effective mapping helps firms prioritize customer needs, tailor communication strategies, and foster stronger relationships. Overall, integrating Voice Sentiment Mapping into customer insights can significantly transform how businesses respond to and anticipate customer expectations, ultimately driving success in an increasingly dynamic market.
Key Components in Voice Sentiment Mapping
Voice Sentiment Mapping is essential for translating customer emotions into actionable insights. This process begins with voice data collection, where the quality and accuracy of recordings can significantly impact outcome relevance. Effective mapping hinges on several key components, including transcription accuracy, sentiment analysis algorithms, and contextual understanding. These elements work together to decode the emotional undertones of customer conversations.
First, accurate transcription of voice data lays the foundation for reliable analysis. This ensures that the nuances in customer language are preserved during processing. Next, advanced sentiment analysis algorithms evaluate the emotional tone of spoken words, identifying positive, negative, or neutral sentiments. Finally, integrating contextual understanding allows businesses to discern the reasons behind customer emotions, adding depth to the insights gained. By focusing on these components, organizations can create a comprehensive sentiment heatmap that provides valuable insights into customer experiences and drives strategic decisions.
Steps to Building a Customer Sentiment Heatmap from Voice Data
Building a customer sentiment heatmap from voice data involves a systematic approach to extracting meaningful insights. First, gather your voice data, which may simply come from recorded customer calls. Once you have these recordings, the initial step requires transcribing the audio into text format. This transcription is crucial as it transforms voice data into analyzable material, enabling you to extract sentiments effectively.
Next, it's vital to analyze the transcriptions for sentiment extraction. Utilizing natural language processing tools can help identify positive, negative, and neutral sentiments expressed by customers. After analyzing the data, you can visualize the insights through a heatmap. This visualization method allows you to see the intensity of sentiments across various customer interactions, highlighting areas of concern and satisfaction. Following these steps will facilitate effective voice sentiment mapping, ultimately guiding your decision-making and enhancing customer relationships.
Extract insights from interviews, calls, surveys and reviews for insights in minutes
Step 1: Collecting and Preparing Voice Data
To effectively build a customer sentiment heatmap, the first crucial step is collecting and preparing voice data. Begin by gathering audio recordings of customer interactions, such as calls or feedback sessions. It's essential to ensure that these recordings are of high quality and free from background noise, as clear audio significantly impacts the accuracy of subsequent analysis. Once collected, the next task involves transcribing these recordings into text format, which will facilitate easier examination and sentiment mapping.
After transcription, organize the data systematically. Create a library of these transcripts, enabling quick access and reference during analysis. Using tools that support bulk transcription can speed up this process. Once organized, tag the transcripts with relevant keywords or themes. This will help in identifying key insights related to customer sentiments efficiently. By following these steps diligently, you lay a solid foundation for effective voice sentiment mapping, ultimately leading to informed decision-making based on customer feedback.
Step 2: Analyzing Voice Data for Sentiment Extraction
To effectively analyze voice data for sentiment extraction, itโs crucial to begin by transcribing recorded conversations. This initial transcription step allows for a clearer examination of the content, enabling the identification of emotions and sentiments conveyed by customers. Once transcription is complete, various analytical tools can help filter and categorize sentiments, transforming raw data into meaningful insights.
Next, utilizing templates to streamline the analysis process can significantly enhance efficiency. These templates may focus on identifying key pain points or extracting specific emotional cues that reflect customer sentiments. With voice sentiment mapping, you can visualize sentiments effectively, facilitating a clearer understanding of recurring themes and customer feelings. By applying these analytical strategies, organizations can extract valuable insights to inform their decision-making processes, thereby enhancing customer engagement and satisfaction.
Step 3: Visualizing Data to Create a Sentiment Heatmap
To create an effective sentiment heatmap, visualizing the analyzed data is crucial. This process allows you to transform raw voice data into easily interpretable insights that showcase customer sentiment. The visualization enables quick identification of patterns, such as favored features or common pain points among customers. Each data point will reflect emotions, from positive to negative, easily highlighted within the heatmap.
To effectively visualize your data for voice sentiment mapping, consider these key steps. First, select a suitable visualization tool that accommodates your data size and format. Next, categorize the sentiments into various groups, allowing for a clear representation on the heatmap. Finally, utilize colors to denote differing sentiment levelsโred for negative responses, green for positive ones, and yellow for neutral sentiments. This way, stakeholders can quickly grasp customer feelings and feedback trends, enhancing decision-making and strategy development.
Top Tools for Voice Sentiment Mapping and Heatmap Generation
When it comes to voice sentiment mapping and heatmap generation, several tools stand out for their effectiveness and user-friendly interfaces. These tools enable businesses to analyze voice data efficiently, offering insights into customer sentiments and behaviors. For instance, platforms like Google Cloud Speech-to-Text excel in transcribing audio into text, making it easier to analyze conversations for tones and emotions.
Further, IBM Watson Tone Analyzer provides sophisticated analytical capabilities to discern emotional nuances in dialogues. Microsoft Azure Cognitive Services delivers a comprehensive suite that can classify sentiments while also offering APIs for seamless integration into existing systems. Amazon Transcribe rounds out the list by facilitating natural language processing, allowing easy access to sentiments expressed in customer calls. Leveraging these tools can significantly enhance your understanding of customer feedback, ultimately aiding in the creation of a detailed and actionable sentiment heatmap.
insight7
Voice sentiment mapping serves as a critical bridge between customer interactions and actionable insights. By analyzing the tone, pitch, and delivery in voice data, businesses can understand how customers genuinely feel about their products or services. Utilizing this approach not only aids in identifying customer sentiment trends but also highlights areas requiring attention or improvement.
To effectively implement voice sentiment mapping, itโs essential to develop a systematic methodology. First, collect high-quality voice data from various customer interactions. Next, employ analytical tools to extract sentiment indicators from this data. Finally, visualize the collected insights in a heatmap format, making essential patterns and trends clear. This structured approach ensures that businesses can transform customer feedback into strategic actions, enhancing overall customer satisfaction and loyalty. Embracing voice sentiment mapping ultimately empowers organizations to stay ahead of the competition while responding to customer needs efficiently.
Google Cloud Speech-to-Text
To embark on voice sentiment mapping, utilizing advanced transcription technology is essential. This is where Google Cloud Speech-to-Text shines, enabling users to convert audio recordings into written text efficiently. By doing so, businesses can transcribe conversations at scale, allowing for comprehensive analysis and insights. For instance, after transferring audio files, the user can quickly generate transcripts, streamlining the process of sentiment analysis and data utilization.
The true power of this technology lies in its ability to accurately interpret emotions and sentiments within conversations. Once the voice data is transcribed, it can be mined for useful insights, revealing customer pain points and preferences. This information forms the foundation for building a sentiment heatmap, showcasing areas needing attention or improvement. Ultimately, utilizing Google Cloud Speech-to-Text enhances the voice sentiment mapping process, creating a pathway to better understand customer perspectives and drive strategic decisions effectively.
IBM Watson Tone Analyzer
The Tone Analyzer serves as a vital tool in Voice Sentiment Mapping, providing insights derived from voice data analysis. It specializes in assessing emotional nuances in conversations, making it easier to understand customer sentiments and improving interpersonal interactions. By decoding tones, it helps identify keywords indicative of emotions like joy, anger, or frustration, enhancing the clarity of customer feedback.
In practice, it first transforms raw voice data into transcribed text. Afterward, the analyzer evaluates the text for emotional content and highlights sentiments that reflect the customer's experience. This process significantly contributes to enriching customer insights, allowing businesses to tailor their responses and improve service. By integrating it into your Voice Sentiment Mapping strategy, you can effectively visualize sentiment trends over time, paving the way for a more responsive and customer-centric approach.
Microsoft Azure Cognitive Services
Microsoft Azure Cognitive Services plays a pivotal role in transforming voice data into insightful customer sentiment. By utilizing advanced machine learning models, this platform offers tools that facilitate the transcribing and analyzing of voice interactions. This means businesses can extract rich sentiment insights from customer calls, shaping their strategies based on actual feedback.
The key offers include speech recognition and natural language processing capabilities. With voice sentiment mapping, organizations can detect emotions and sentiments expressed in conversation, providing a more nuanced understanding of customer experiences. The combination of these tools empowers teams to create responsive strategies, allowing for real-time adjustments based on customer feedback. Integrating these services enables effective visualization of sentiment data, making it easier for businesses to identify trends and enhance the customer experience. This approach ultimately leads to informed business decisions and improved customer engagement.
Amazon Transcribe
Amazon Transcribe offers a robust solution for transforming audio recordings into searchable transcripts, a critical step in voice sentiment mapping. Users can easily upload multiple audio files and receive accurate transcriptions, enabling deeper analysis of customer interactions. This functionality is crucial for businesses looking to understand the nuances of customer sentiment expressed during conversations.
Once the voice data is transcribed, insights can be derived by analyzing the text for emotional undertones and specific sentiments. The platform supports bulk operations, allowing organizations to scale their transcriptions efficiently. This capability not only streamlines the process but also enhances the overall understanding of customer experiences. By recognizing patterns and sentiments in the conversations, businesses can create effective sentiment heatmaps that visually represent customer feedback, leading to more informed decision-making and strategy development.
Conclusion: Mastering Voice Sentiment Mapping and Heatmap Creation
In conclusion, mastering voice sentiment mapping and heatmap creation is a pivotal step in understanding customer perceptions. Through effective analysis of voice data, businesses can identify patterns in sentiments, transforming raw feedback into actionable insights. By systematically mapping both positive and negative sentiments, organizations can highlight areas that require improvement or celebration.
The process doesnโt end with data collection; creating a visual representation via heatmaps enhances clarity and accessibility of information. This enables teams to make informed decisions that foster customer satisfaction and loyalty. Ultimately, embracing voice sentiment mapping empowers businesses to stay ahead, adapting strategies based on real customer feedback.