How to Analyze Panel data
-
Bella Williams
- 10 min read
In panel data analysis, understanding how to structure the analysis is vital for generating meaningful insights. This section focuses on the markdown formatting, which allows users to present their findings clearly and effectively. Utilizing markdown simplifies the process of documenting results by making them easily readable and organized. When presenting panel data findings, using headers, lists, and tables ensures clarity, enabling stakeholders to grasp complex information quickly.
To further clarify this process, consider a few key practices. First, establishing a clear hierarchy using headers improves readability significantly. Second, employing bullet points or numbered lists organizes data and highlights essential elements efficiently. Lastly, integrating tables enhances the presentation of quantitative results, allowing for immediate visual interpretation of findings. By following these markdown principles, you ensure that your analysis of panel data is not only insightful but also accessible to a broader audience.
Introduction to Panel Data Analysis
Panel Data Analysis is a powerful tool used to uncover trends and relationships in datasets that track multiple subjects over time. Unlike standard data methods, panel data combines both cross-sectional and time-series elements, allowing for a more dynamic exploration of behavioral patterns and changes. This complexity equips researchers with richer insights that can inform decision-making processes.
Understanding the intricacies of Panel Data Analysis is crucial for anyone looking to extract meaningful conclusions from their data. It helps in addressing questions around how variables evolve and interact over time. By mastering this technique, analysts can significantly enhance their research's depth, making it an invaluable asset in sectors ranging from economics to social sciences.
Generate visualizations from your qualitative data. At Scale.
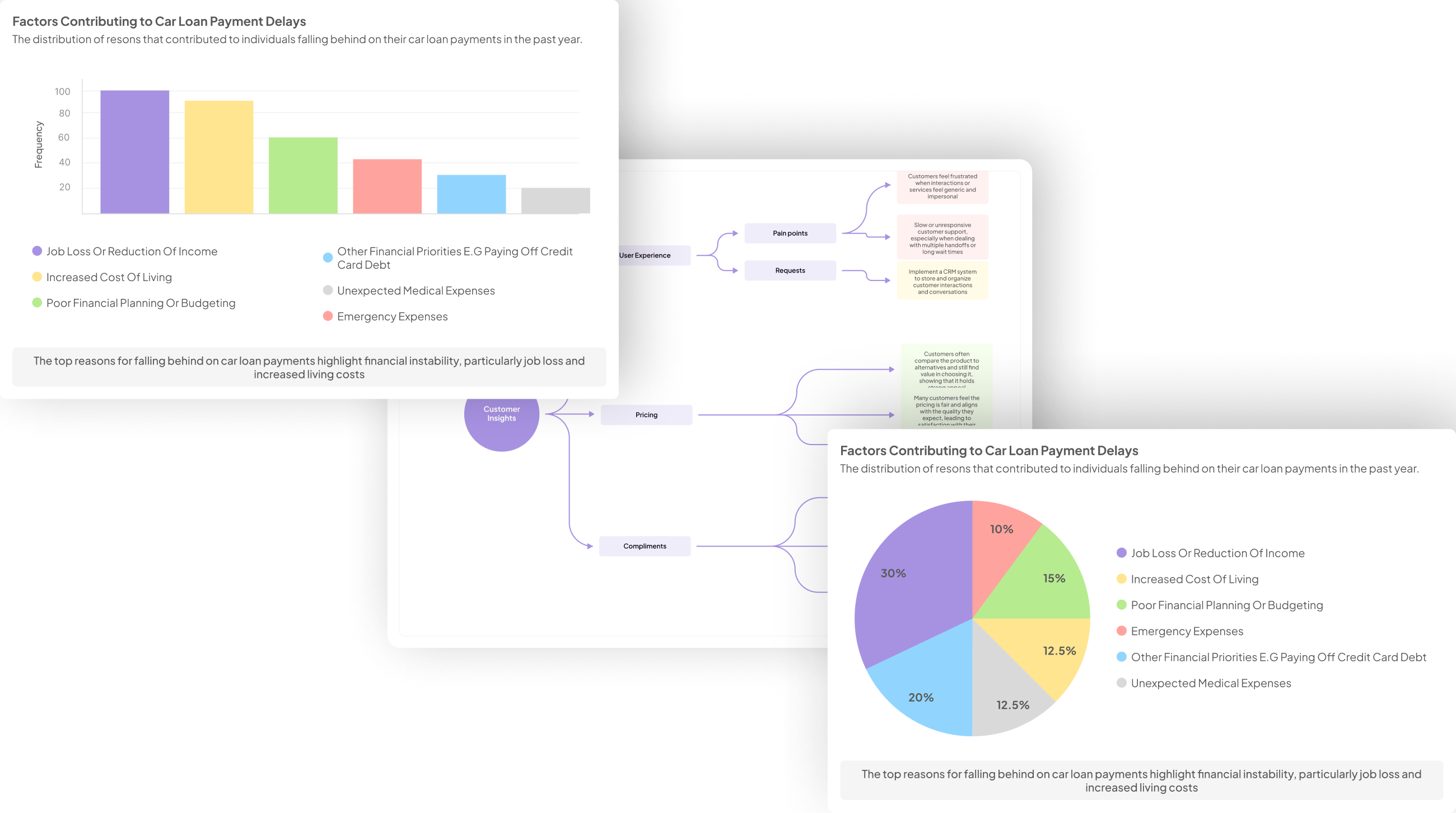
Understanding the Basics of Panel Data Analysis
Panel Data Analysis combines two vital data dimensions: cross-sectional and time-series data. This dual nature allows researchers to observe multiple subjects over various periods, creating a rich dataset for insightful analysis. Understanding these characteristics is crucial as they enrich the dataโs depth, enabling the uncovering of trends and relationships that simpler data formats might overlook. For example, studying individual behavior over time through repeated observations offers clearer insights into changes and patterns.
One of the primary advantages of Panel Data Analysis is its ability to control for unobserved variables. By accounting for both individual-specific effects and time variations, researchers can deduce more reliable causal relationships. Additionally, this approach enhances statistical power, as data observations are multiplied across time and entities. Ultimately, mastering these basics lays a strong foundation for conducting advanced analysis, ensuring that findings are both precise and robust.
What is Panel Data?
Panel data refers to a dataset that contains observations over time for multiple subjects, such as individuals, companies, or countries. It combines aspects of both cross-sectional data and time-series data. In simple terms, this means that you can track the same entities across various time periods, providing richer information for analysis.
One of the main strengths of panel data is its ability to account for individual heterogeneity, or differences among entities. By analyzing changes over time, researchers can assess cause-and-effect relationships more effectively. This is where Panel Data Analysis comes into play; it helps to uncover trends, patterns, and insights that traditional data analysis might miss. Furthermore, it enables more robust statistical modeling, enhancing the validity of results in studies across various fields, including economics and social sciences. Understanding panel data is essential for conducting thorough and insightful analyses.
- Explanation of panel data characteristics, such as cross-sectional and time-series dimensions.
Panel data refers to a dataset that captures multiple entities over various time periods, providing a rich structure for analysis. The two primary dimensions of panel data are cross-sectional and time-series qualities. Cross-sectional dimensions involve observations across different subjects at a single point in time. This dimension allows researchers to compare differences or commonalities among various entities, enhancing the understanding of relationships within the data.
In contrast, the time-series component tracks changes within the same entities over time. This longitudinal aspect is crucial for observing trends, identifying patterns, and assessing the impact of specific variables over time. By blending these two dimensions, panel data analysis produces more robust insights. It allows researchers to distinguish between variations due to individual characteristics and changes occurring over time, leading to a deeper understanding of the phenomena being studied. This integrated approach enhances its applicability in various fields, including economics, health studies, and social science research.
Benefits of Using Panel Data
Panel Data Analysis offers numerous advantages that make it a powerful tool for researchers across various fields. One significant benefit is the ability to observe multiple dimensions of data over time. This enabling feature provides insight into dynamic behaviors and patterns that cannot be detected using cross-sectional data alone. Understanding trends and variations becomes more comprehensive when both time and subject attributes are considered together.
Moreover, panel data enhances the robustness of statistical analyses by allowing for the control of unobserved variables that may bias results. Researchers can differentiate between individual and time-specific effects, leading to more precise estimates. This adaptability makes panel data an indispensable resource for studies in economics, social sciences, and beyond. By employing different analytical techniques, such as fixed or random effects models, analysts can uncover nuanced relationships and make better-informed conclusions. In essence, the benefits of using panel data significantly enrich the overall analytical process, yielding valuable insights for decision-making.
- Advantages of panel data over other data types in econometrics and social sciences.
Panel data analysis offers numerous advantages over other data types, especially in the fields of econometrics and social sciences. One significant benefit is the ability to observe multiple subjects over time, which leads to richer, more nuanced insights. This longitudinal aspect allows researchers to capture dynamics that might be missed in purely cross-sectional studies.
Another key advantage is the reduction of unobserved heterogeneity. By analyzing the same subjects repeatedly, panel data can help control for factors that are often unmeasurable, such as individual-specific traits. This leads to more accurate estimates and robust conclusions. Additionally, panel data enables the examination of temporal changes and causal relationships by tracking how variables evolve over different time periods. Overall, its diverse capabilities make panel data analysis an essential tool for obtaining reliable findings in various research domains.
Generate Journey maps, Mind maps, Bar charts and more from your data in Minutes
Steps to Conduct Panel Data Analysis
To conduct effective panel data analysis, start with data preparation, which is crucial for accuracy. First, gather relevant panel data from reliable sources. Ensure the data covers multiple subjects over time, allowing for a comprehensive analysis. Once collected, move on to data cleaning. Remove duplicates and address any missing values to enhance data integrity and usability. This step is foundational, as the quality of your analysis relies heavily on clean data.
Next, choose the right model for your analysis. You'll decide between fixed effects and random effects models, each suitable for different scenarios. Fixed effects models control for unobserved variables that could bias your results, while random effects assume that differences across entities are random and not correlated with the predictors. Lastly, conduct regression analysis. This involves applying statistical techniques tailored for panel data to uncover relationships between variables over time, ultimately providing insights that are rich and actionable.
Preparing Your Data
To prepare your data for panel data analysis, the first step centers around effective data collection. Identify the relevant sources that provide comprehensive and accurate panel datasets. This can span various domains, including surveys, administrative records, or market data, depending on your research question. Ensure that the data includes both cross-sectional and time-series elements to enable robust analysis.
Once you have gathered your data, the next crucial phase is data cleaning. This involves filtering out any inconsistencies, handling missing values, and ensuring that the data is structured correctly. Clean data is essential for reliable results, as any errors at this stage can significantly affect your analysis outcomes. Ultimately, preparing your data effectively sets the foundation for successful panel data analysis, allowing you to derive meaningful insights from your research.
- Step 1: Data Collection
To embark on effective panel data analysis, the first step involves meticulous data collection. This phase sets the foundation for your research, ensuring you have the necessary information to explore and understand trends over time. Begin by identifying the key variables that are required for your analysis. Consider variables that can explain changes across different time periods and entities, as they are crucial for drawing meaningful conclusions.
Next, gather data from reliable sources, whether quantitative surveys, administrative records, or existing datasets. Ensure that the dataset includes multiple cross-sections observed at various times. This structure is key for conducting meaningful panel data analysis. After collecting your data, verify its completeness and relevance to your research question, as the quality of data directly influences the insights you can derive from it. Taking the time to meticulously collect data will ultimately enhance the accuracy and reliability of your analysis.
- How to gather relevant panel data for analysis.
Gathering relevant panel data for analysis involves several crucial steps that ensure the datasets you use are rich and informative. First, identify your target population and the variables necessary for your analysis. This helps in tailoring your data collection efforts to suit your specific research goals in panel data analysis. Data can be obtained from various sources, such as surveys, experiments, or existing datasets. Be sure to utilize a consistent format across different time periods to maintain the integrity of your longitudinal study.
Next, establish a clear methodology for data collection. Consider whether you want to employ qualitative or quantitative methods, as both can provide valuable insights. If using surveys, ensure questions are designed for clarity and consistency. After gathering the data, it's critical to check for completeness and accuracy. This groundwork lays the foundation for effective data cleaning, which is essential for reliable panel data analysis. Through diligent collection and preparation, you can unlock powerful insights from your panel data.
- Step 2: Data Cleaning
Data cleaning is a crucial step in the panel data analysis process. It serves as the foundation for achieving accurate insights and reliable results. Begin by identifying and rectifying inconsistencies within the dataset. This involves handling missing values, which can skew analyses if not addressed. Moreover, standardizing variable formats ensures uniformity, making the data easier to interpret and analyze.
Next, detect and eliminate outliers, as these can distort your findings. Outliers may result from errors during data entry or genuinely rare occurrences. By performing exploratory data analysis, you can identify these anomalies and decide whether to exclude or adjust them. Once these tasks are complete, your data will be clean, enabling a smooth progression to modeling and analysis stages. Establishing a rigorous cleaning protocol is key to successful panel data analysis, as it amplifies the validity of subsequent conclusions drawn from the data.
- Essential cleaning techniques to ensure quality data.
To achieve accurate panel data analysis, essential cleaning techniques are crucial. Begin by identifying and handling missing values within your dataset. Missing data can skew results and lead to erroneous conclusions. Implement methods such as imputation or removal to minimize their impact. By maintaining a consistent data structure, you'll ensure that analysis techniques yield reliable insights.
Next, eliminate duplicates to avoid inflated results. Duplicates can arise from various data collection methods, affecting the integrity of your analysis. Standardization of data formats is another key aspect. Ensure uniformity in units and categorical variables to facilitate accurate comparisons. Finally, outlier detection is vital. Outliers can distort your findings, so it's key to investigate their origin and decide whether to retain or remove them. By employing these techniques, you establish a solid foundation for effective panel data analysis, enhancing the reliability and validity of your insights.
Choosing the Right Model for Panel Data Analysis
Choosing the right model for panel data analysis is crucial for obtaining reliable results. The two primary models to consider are fixed effects and random effects. Understanding the differences between these models enables researchers to make informed decisions based on the nature of the data and the research objectives. Fixed effects models control for time-invariant characteristics of the entities analyzed, which helps reduce bias from omitted variables.
In contrast, random effects models assume that individual-specific effects are uncorrelated with the independent variables. This allows for more efficient estimation when the assumption holds true. It's essential to conduct tests, such as the Hausman test, to determine which model is more appropriate for your specific dataset. Ultimately, selecting the model best suited for your panel data analysis can significantly impact the accuracy of your findings and the reliability of your conclusions.
- Step 3: Fixed Effects vs. Random Effects
When performing panel data analysis, one crucial decision lies in choosing between fixed effects and random effects models. The fixed effects model accounts for individual-specific characteristics that do not change over time, effectively controlling for these unobserved variables. This approach is particularly beneficial when the focus is on analyzing the impact of specific variables that vary over time. It mitigates bias in estimates by only considering variations within the same entity across different time periods.
Conversely, the random effects model assumes that unobserved individual-specific effects are randomly distributed across the dataset. This model is suitable when the researcher believes that individual differences are unrelated to the independent variables. In cases where the objective is to analyze the overall impact of variables across entities, random effects can lead to more efficient estimates. Ultimately, the choice between fixed effects and random effects in panel data analysis should be guided by the nature of the data and the research questions at hand.
- A comparison of fixed effects and random effects models.
When analyzing panel data, selecting the appropriate model is crucial, particularly between fixed effects and random effects. Each approach has its unique advantages based on the underlying assumptions about the data. Fixed effects models are ideal when you want to control for unobserved variables that are constant over time. By focusing only on within-group variation, this method effectively eliminates bias from omitted variables that might skew results.
On the other hand, random effects models assume that individual-specific effects are uncorrelated with the independent variables. This characteristic allows for the estimation of effects using both within-group and between-group variations, which can enhance efficiency in cases where the assumption holds true. Understanding these distinctions is essential for making informed decisions in panel data analysis, ultimately optimizing results for deeper insights and more robust conclusions.
- Step 4: Conducting Regression Analysis
In Step 4: Conducting Regression Analysis, the focus shifts to how to effectively analyze relationships in panel data. Regression analysis serves as a powerful tool for understanding the behavior of variables over time and across different entities. By applying regression techniques, analysts can evaluate the impact of independent variables on a dependent variable while accounting for the unique characteristics of each entity in the panel.
To conduct a regression analysis, users should follow several steps. First, select the appropriate regression modelโwhether fixed effects or random effectsโbased on the data's characteristics. Second, ensure that the data meets the necessary assumptions, such as linearity and homoscedasticity. Finally, interpret the results carefully, paying attention to coefficients, significance levels, and overall model fit. This process provides valuable insights that facilitate informed decision-making and strategy formulation within various fields, such as economics and social sciences.
- Introduction to panel data regression techniques.
Panel Data Analysis offers researchers a powerful way to study complex data that changes over time. By combining cross-sectional and time-series dimensions, it enables deeper insights into the dynamics of various subjects. This technique allows analysts to capture trends and variations, essential for understanding patterns not observable in pure cross-sectional or time-series data.
In this section, we will introduce foundational regression techniques specific to panel data. We will cover the essential models, such as fixed effects and random effects, crucial for analyzing the nuances within your data. Understanding these approaches is vital, as they can significantly influence the interpretation of results and provide clearer insights into temporal and individual variations.
Conclusion on Panel Data Analysis
In conclusion, panel data analysis serves as a powerful tool for understanding complex relationships within datasets that include multiple dimensions. By effectively combining both cross-sectional and time-series data, researchers can gain deeper insights into trends, behaviors, and impacts over time. This multifaceted approach allows for nuanced interpretations that single-dimensional datasets cannot provide.
Ultimately, successful panel data analysis hinges on rigorous data preparation, model selection, and the application of appropriate statistical techniques. By following these foundational steps, researchers can unlock the full potential of their data, leading to more informed decisions and robust conclusions in their studies.
- Summary of key takeaways and tips for successful panel data analysis.
Success in panel data analysis hinges on a few crucial strategies. First, ensure that your data collection methods are rigorous and well-structured. A diverse range of observations across time and entities is vital for extracting meaningful insights. Next, prioritize data cleaning to eliminate inconsistencies, which can significantly skew your results. Properly managed data lays the groundwork for an effective analysis.
When it comes to modeling, choosing between fixed effects and random effects is essential. Fixed effects models control for individual differences while random effects allow for variability across entities. Understanding the nuances of these methods can enhance the accuracy of your conclusions. Finally, always validate your findings through thorough regression analysis, ensuring that the relationships you observe are robust. Each of these steps contributes to a more comprehensive and successful panel data analysis.
### Preparing Your Data
Panel Data Analysis begins with a thorough data preparation process, crucial for obtaining reliable insights. The first step involves data collection, where relevant panel data must be gathered from various sources, such as surveys or existing databases. It's essential to ensure the data encompasses both cross-sectional and time-series dimensions to leverage the full potential of panel data.
Once data collection is complete, the next critical step is data cleaning. This involves checking for inconsistencies, missing values, or errors that may compromise the quality of your analysis. Techniques such as removing duplicates or filling in missing information can help enhance the datasetโs reliability. Effective data preparation lays a strong foundation for a successful analysis, enabling researchers to draw meaningful conclusions from their findings. It's through these rigorous steps that panel data can truly shine as a tool for insights.