How to Analyze Listening Tour Notes Using AI
-
Bella Williams
- 10 min read
AI-Driven Note Analysis revolutionizes how organizations interpret feedback gathered during listening tours. Imagine sifting through countless notes while trying to extract meaningful themes and insights; it can be overwhelming. However, with the help of AI technologies, this once daunting task can be simplified. AI tools meticulously categorize and analyze data, making it easier to derive valuable insights that inform decision-making.
By employing AI-Driven Note Analysis, organizations can transform raw narratives into structured data points. This process significantly enhances the accuracy of insights, transforming subjective responses into actionable strategies. Understanding the potential of AI in streamlining this analysis phase can turn qualitative data into powerful quantitative outcomes, ensuring teams make informed choices grounded in user feedback.
Analyze & Evaluate Calls. At Scale.
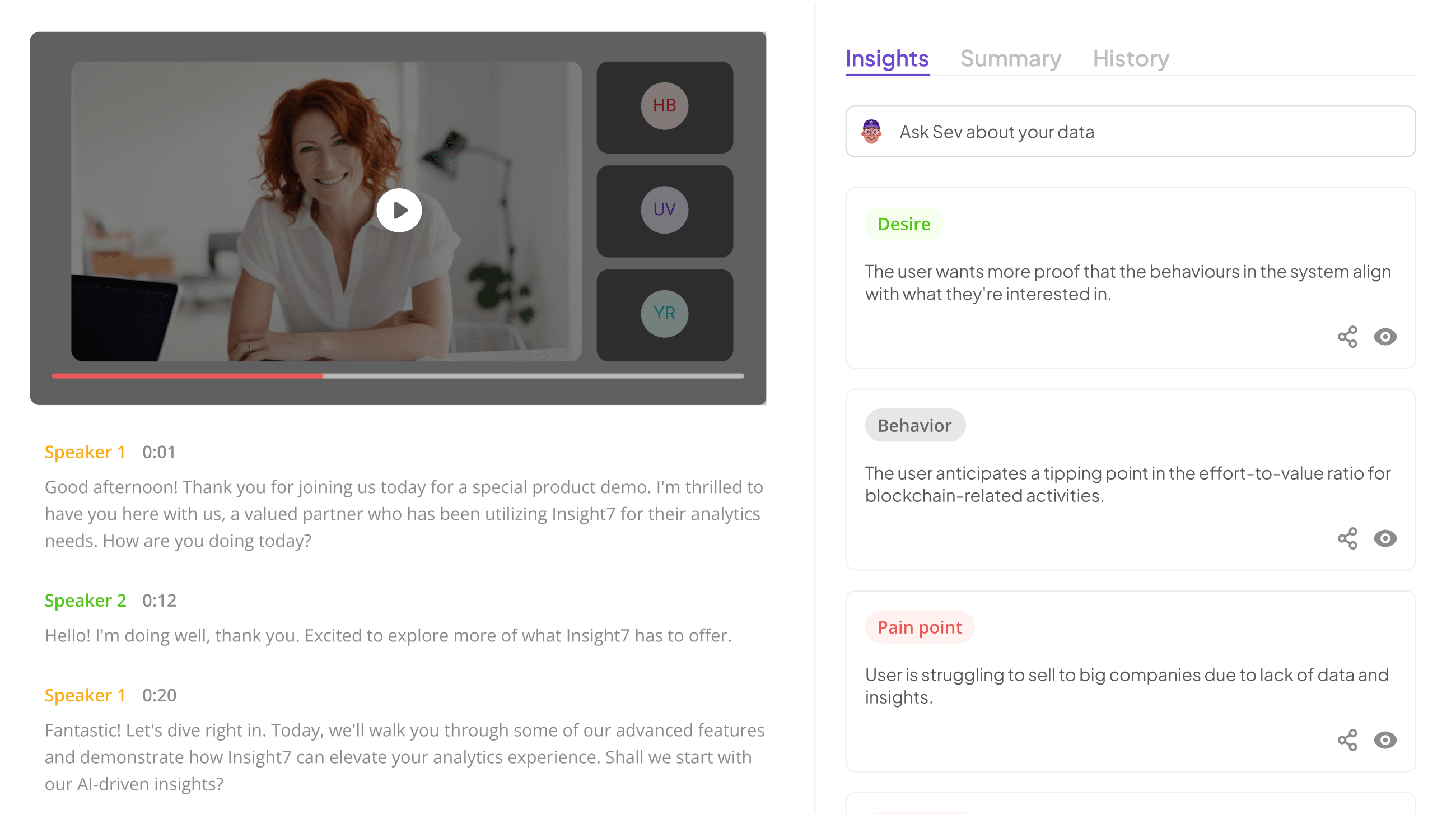
The Essentials of AI-Driven Note Analysis
AI-Driven Note Analysis offers a systematic approach to interpreting the extensive qualitative data collected during listening tours. This process begins by using advanced algorithms to scan and categorize notes, enabling a cohesive overview of key themes and sentiments. Natural Language Processing (NLP) plays a crucial role here, as it allows AI to comprehend the nuances in human language, making it possible to extract relevant insights, even from complex remarks.
The true strength of AI-Driven Note Analysis lies in its ability to enhance both accuracy and efficiency in data evaluation. By transforming raw notes into structured insights, organizations can identify patterns and address challenges effectively. These insights pave the way for direct action, ultimately leading to improved strategies and outcomes by ensuring that every voice from the listening tour is acknowledged and utilized. Emphasizing these essentials will foster a deeper understanding of how to derive meaningful conclusions from listening tour notes.
Understanding the Process of AI-Driven Note Analysis
AI-Driven Note Analysis begins with the seamless integration of artificial intelligence to sift through vast amounts of data gathered during listening tours. The process typically entails capturing notes, followed by categorizing and interpreting content. AI algorithms leverage natural language processing (NLP) techniques to parse through text for context, sentiment, and themes. This allows for a nuanced understanding of participants' feedback, transforming raw notes into structured, actionable insights.
To illustrate this process effectively, consider the following elements that contribute to AI-Driven Note Analysis:
- Data Collection: Effective analysis starts with systematic data gathering from various sources.
- Categorization: AI sorts the notes into predefined themes, aiding in focused analysis.
- Interpretation: The NLP engine processes the notes to extract meanings and emotional tones.
- Reporting: AI systems generate concise reports highlighting key insights, making them easy to share and understand.
Each component plays a crucial role in ensuring that insights derived from the analysis are both reliable and actionable, ultimately enhancing decision-making processes.
- Explanation of how AI processes and categorizes notes
AI processes and categorizes notes through advanced algorithms that analyze text patterns and context. Initially, it utilizes natural language processing (NLP) technology to understand the meaning and nuances of the language used in the notes. This allows AI to identify themes, sentiment, and key insights embedded in the conversations captured during listening tours. By discerning the intent and relevant topics, AI can classify notes accurately based on diverse criteria, such as speaker and subject matter.
Once categorized, users can easily filter this information to focus on specific themes or insights relevant to their goals. For instance, if an organization wants to delve into process management, AI can highlight key quotes and findings associated with that topic. This capability not only streamlines the analysis process but also ensures that the resulting insights are actionable and tailored to specific needs. Thus, AI-driven note analysis significantly enhances the overall effectiveness of data interpretation.
- The role of natural language processing (NLP) in understanding text
Natural language processing (NLP) plays a crucial role in AI-driven note analysis by enabling machines to understand and interpret human language. This capability allows AI systems to process vast amounts of listening tour notes effectively, extracting key insights that might be overlooked manually. By analyzing sentiment, tone, and themes, NLP transforms unstructured data into structured information that forges a clearer path to actionable insights.
Through AI-driven note analysis, NLP identifies patterns within conversations, distinguishing between positive, negative, and neutral sentiments. This aspect helps organizations to gauge audience reactions and sentiments in real-time, ensuring that decision-makers can address concerns proactively. By understanding text through NLP, organizations harness the power of data to refine strategies, enhance messaging, and ultimately drive better engagement with their target audiences. The fusion of AI and NLP not only streamlines data analysis but also unlocks the potential of conversational data, which is rapidly becoming a valuable resource for businesses.
Benefits of Using AI-Driven Note Analysis
AI-Driven Note Analysis offers significant benefits that can effectively transform how we manage and interpret listening tour notes. One of the primary advantages is enhancing accuracy and efficiency in data analysis. Traditional methods often involve tedious manual sorting and interpreting of observations, which can lead to errors and missed insights. With AI, organizations can quickly process large volumes of notes, ensuring that critical themes and sentiments are captured more accurately.
Another vital benefit is the ability to transform raw data into actionable insights. By utilizing advanced Natural Language Processing techniques, AI can identify underlying trends, relationships, and sentiments that may not be immediately apparent. This not only saves time but also empowers decision-makers with clearer insights. Ultimately, AI-Driven Note Analysis enables teams to make informed decisions based on well-structured data interpretations. Embracing this technology allows organizations to become more responsive and aligned with participant needs.
- Enhancing accuracy and efficiency in data analysis
AI-Driven Note Analysis greatly enhances both accuracy and efficiency in data analysis. By employing advanced algorithms, AI can quickly categorize and process large volumes of notes collected during listening tours. This allows for the identification of key themes and patterns, significantly reducing the time required for manual analysis. As a result, stakeholders gain valuable insights more swiftly and can make informed decisions faster.
The method of clustering insights into relevant themes also improves the accuracy of data interpretation. With AI, you can define specific tags, which allows for targeted analysis depending on predefined goals or areas of interest. For example, if one aims to understand the challenges faced by participants, AI can pull relevant quotes and sentiments from the notes efficiently. This not only saves time but also enhances the reliability of the insights generated, leading to better-informed strategies for engagement and improvement. Ultimately, AI-Driven Note Analysis aids in transforming raw data into meaningful conclusions that drive effective action.
- Transforming raw data into actionable insights
Transforming raw data into actionable insights requires a strategic approach, especially when analyzing notes from listening tours. By applying AI-Driven Note Analysis, organizations can efficiently sift through large volumes of qualitative data. This method involves utilizing natural language processing (NLP) technology to categorize sentiments, identify themes, and extract key information from conversations. As a result, valuable insights emerge, allowing decision-makers to better understand the needs and preferences of their audience.
The process begins with collecting and digitizing notes, creating a wealth of data for AI algorithms to analyze. Once processed, AI can reveal trends and patterns that might otherwise go unnoticed. By translating qualitative data into quantitative metrics, organizations can derive actionable insights that guide strategy development. This approach not only enhances the accuracy of data interpretation but also transforms the way insights are utilized, ultimately leading to informed decision-making and improved outcomes.
Extract insights from interviews, calls, surveys and reviews for insights in minutes
Implementing AI Tools for Effective Note Analysis
Implementing AI tools for effective note analysis streamlines the processing of insights gathered during listening tours. The integration of AI-Driven Note Analysis begins with collecting and digitizing your notes. This essential step ensures that the data is in a format suitable for machine learning algorithms to analyze, minimizing the risk of loss or misinterpretation.
Next, you will pre-process your data to enhance its compatibility with the chosen AI tools. This could involve cleaning up any unnecessary information or formatting to allow AI algorithms to work efficiently. After preparation, applying AI tools like IBM Watson or Google Cloud Natural Language can help to extract themes and sentiments from the data. Finally, interpreting the results will guide you in generating actionable insights, ultimately leading to informed decision-making for your organization. By following these steps, you can transform complex listening tour notes into valuable data that drives improvement.
Key AI Tools for Note Analysis
AI-Driven Note Analysis is revolutionizing how organizations sift through listening tour notes, transforming a daunting task into an efficient process. The right AI tools can streamline the analysis by categorizing and identifying key themes from your data with remarkable accuracy. These tools leverage natural language processing technologies to make sense of complex language and extract relevant insights quickly.
Several key AI tools stand out for their effectiveness. Insight7 excels at delivering actionable insights tailored to specific use cases, saving time through analysis kits that categorize data intuitively. IBM Watson offers a robust NLP platform, ensuring a thorough understanding of context and sentiment within notes. Microsoft Azure Cognitive Services provides powerful language capabilities, enhancing text comprehension. Google Cloud Natural Language focuses on both syntax and sentiment, breaking down information into manageable insights, while Amazon Comprehend utilizes machine learning to uncover deeper patterns and trends in the data. Utilizing these tools can significantly enhance the efficiency and depth of your note analysis.
- insight7: Leading tool for AI-Driven Note Analysis
insight7 stands out as a leading tool for AI-Driven Note Analysis, designed to simplify data interaction for users across various expertise levels. The platform combines user-friendly interfaces with advanced analytical features, enabling teams to efficiently analyze their listening tour notes. By centralizing data into projects and libraries, users can easily upload and access transcripts from interviews or discussions, thereby streamlining the analysis process.
Within the tool, users can extract insights, identify themes, and generate summaries with remarkable ease. Insight7 employs specific kits tailored for various analytical tasks, allowing users to begin their evaluations without extensive setup. This capability ensures that even those unfamiliar with data analytics can leverage the power of AI-driven insights effectively. By merging accessibility with sophisticated features, insight7 promises to enhance the way organizations utilize their qualitative data, turning notes into actionable insights that guide decision-making.
- IBM Watson: Versatile AI platform with NLP capabilities
AI-Driven Note Analysis enables organizations to efficiently extract meaningful insights from listening tour notes using advanced technology. A versatile AI platform with natural language processing capabilities can analyze large volumes of text data swiftly and accurately. By harnessing such technology, users can transform unstructured notes into structured data, uncovering patterns and trends that may otherwise go unnoticed.
This powerful platform operates by processing and understanding linguistic nuances inherent in the text, ensuring context and sentiment are aptly captured. Furthermore, it categorizes information based on relevance, helping teams to focus on critical insights that drive strategic decision-making. Embracing this approach not only enhances the efficiency of analysis but also fosters a deeper understanding of customer needs and preferences. Consequently, organizations are better positioned to respond to emerging trends and challenges in their respective markets, ultimately leading to improved satisfaction and engagement.
- Microsoft Azure Cognitive Services: Offers powerful language understanding services
Microsoft Azure Cognitive Services provides a robust suite of tools designed for language understanding, making it a powerful asset for AI-driven note analysis. By utilizing advanced natural language processing capabilities, these services can effectively interpret and categorize listening tour notes, ensuring that valuable insights are highlighted and understood. This enhances the overall efficiency of data analysis, allowing teams to focus on actionable findings instead of getting lost in raw information.
When integrating these services, organizations can expect a transformative experience. The system excels in semantic understanding, which means it can grasp context and nuances, effectively summarizing key themes from discussions. Additionally, the ability to analyze sentiment and tone adds further layers of insight. As organizations continue to embark on their AI journeys, understanding the capabilities of such services is critical for leveraging data effectively in decision-making and strategy development.
- Google Cloud Natural Language: Provides syntax and sentiment analysis
AI-driven note analysis is revolutionizing how we process and understand the insights gathered from listening tours. One crucial component of this technology is its ability to provide syntax and sentiment analysis. This allows organizations to effectively interpret the emotional tone and linguistic structure of the notes collected, leading to deeper insights into audience perspectives. By analyzing the language used in your notes, AI can uncover sentiment trends, helping you discern whether feedback is predominantly positive, negative, or neutral.
Implementing these advanced capabilities fosters a more nuanced understanding of your stakeholders' sentiments. Syntax analysis aids in identifying key themes and topics, while sentiment analysis highlights emotional responses, guiding strategic decisions. Together, these features empower your team to extract meaningful insights from qualitative data. In this fast-paced, data-rich environment, leveraging AI-driven note analysis will ensure that you can make informed decisions based on authentic feedback from your listening tours.
- Amazon Comprehend: Delivers insights through machine learning
AI-driven note analysis leads the way in transforming qualitative data into meaningful insights. One notable tool in this space excels at employing machine learning techniques to identify trends, topics, and sentiments from collected notes. As this technology processes the text, it effectively uncovers hidden themes that may not be immediately obvious to human analysts. This allows organizations to gain a deeper understanding of stakeholder feedback from listening tours.
Moreover, the utilization of advanced algorithms enhances the accuracy and efficiency of data analysis while minimizing the risk of human error. It streamlines the workflow by automating the transcription of audio and video content, converting it into structured text ready for analysis. This process not only saves time but also generates actionable insights, empowering decision-makers to respond effectively to the needs and opinions of their audiences. In the evolving field of note analysis, machine learning is becoming an invaluable asset.
Steps to Integrate AI in Note Analysis
Integrating AI in note analysis involves a systematic approach that maximizes the efficiency of AI-Driven Note Analysis. First, you must collect and digitize all relevant notes from your listening tours. This initial step is crucial as it sets the foundation for accurate data analysis. Once your notes are digitized, the next step is pre-processing the data. This involves organizing the text and ensuring it is compatible with AI tools, such as removing unnecessary formatting or content.
After these preparatory steps, you can apply AI tools designed for note analysis. These tools utilize natural language processing (NLP) to categorize and interpret the data effectively. Finally, interpreting the results is essential, as this is where actionable insights can emerge. Analyzing the output will help structure strategies based on the feedback gathered, enhancing future initiatives and decision-making processes. Following these steps will ensure a smooth integration of AI into your note analysis workflow.
- Step 1: Collect and digitize notes from listening tours
To initiate the process of AI-Driven Note Analysis, start by collecting and digitizing notes from your listening tours. Capture insights from conversations, workshops, or interviews in a systematic manner. This typically involves recording audio or video, which can later be transcribed to text. Ensure that transcription is accurate, as this raw data forms the foundation for subsequent analysis.
Once you have digitized notes, organize them into relevant categories, such as themes or topics discussed. This step not only aids in clarity but also allows for better utilization of AI tools that analyze text. Enhancing the quality of your data through structured formats will facilitate effective AI processing and help generate actionable insights that improve decision-making. Paying attention to how you structure and categorize your data is crucial for the success of your analysis journey.
- Step 2: Pre-process data for AI compatibility
To ensure effective AI-Driven Note Analysis, pre-processing your data is vital. This stage transforms raw notes into a structured format to enhance compatibility with AI tools. First, clean your data by removing any irrelevant information, such as personal identifiers or off-topic remarks. This helps maintain focus on key insights and themes derived from the listening tours.
Next, standardize the data format. Convert all notes to a consistent text structure, making them easier for algorithms to interpret. This may include organizing by date, speaker, or specific themes discussed during the tour. Applying natural language processing techniques can also assist in identifying crucial keywords and phrases, enriching the data set further. Following these steps prepares your data for an AI analysis, ensuring accurate and actionable results for reporting.
- Step 3: Apply AI tools for note analysis
Applying AI tools for note analysis can significantly streamline the process of transforming your listening tour notes into meaningful insights. Begin by selecting appropriate AI-driven tools that can analyze themes and categorize the data effectively. Tools like IBM Watson and Google Cloud Natural Language are instrumental in parsing text and providing sentiment analysis, which aids in understanding participant feedback accurately.
Once you've selected your tools, the next step involves feeding your digitized notes into the system. The AI algorithms will process the data, identifying key themes and trends relevant to your objectives. Be sure to define your goals, such as improving member experiences or identifying challenges, so the tools can tailor the analysis accordingly. This targeted approach not only saves time but also harnesses the power of AI to provide actionable insights that are integral to decision-making. As a result, this step enhances your overall analysis process and paves the way for identifying crucial areas of focus and improvement.
- Step 4: Interpret results and generate insights
Interpreting results and generating insights from your AI-Driven Note Analysis is crucial for understanding the expectations and experiences of participants. After applying AI techniques, you'll collapse vast amounts of qualitative data into meaningful patterns. This process begins with identifying key themes that emerge from the notes, which can range from participant challenges to suggested improvements.
Next, synthesize the identified themes with a framework that aligns with your organizational goals. This can involve categorizing insights according to specific objectives, such as enhancing member experience or addressing certain risks. By analyzing sentiment related to these themes, you not only identify areas of interest but also gauge participant feelings regarding various topics. This deeper understanding can guide decision-making and strategies moving forward. Ultimately, effective interpretation empowers you to take informed actions based on the analyzed insights, enhancing future initiatives.
Conclusion: The Future of AI-Driven Note Analysis in Listening Tours
The future of AI-driven note analysis in listening tours promises to reshape how organizations gather and interpret feedback. As AI technology advances, its integration into note analysis will enhance data accuracy and streamline processes, allowing for deeper insights. This evolution will empower businesses to better understand their stakeholders and make data-driven decisions with confidence.
Moreover, as natural language processing and machine learning improve, AI-driven tools will increasingly offer tailored analytics. Organizations will benefit from these insights, facilitating proactive engagement with their audiences. The emergence of advanced AI applications will not only simplify data analysis but also elevate the overall effectiveness of listening tours in capturing valuable insights.