In the realm of qualitative research, interview-based surveys have long been a cornerstone for gathering profound insights. Yet, the analysis of these interviews can often be a daunting task, fraught with time consumption and the potential for bias. AI-driven qualitative analysis emerges as a transformative solution, offering researchers cutting-edge tools that streamline the evaluation of interview data. By automating much of the analytical process, AI enables teams to uncover critical themes and insights with increased efficiency.
This section delves into the essence of AI-driven qualitative analysis, elucidating its role in enhancing both the accuracy and speed of interpreting research findings. As the demand for quicker results intensifies, leveraging AI not only addresses the challenges of manual analysis but also fosters consistency across research teams. Thus, understanding how to effectively implement these AI techniques becomes essential for researchers seeking to stay ahead in a competitive landscape.
Extract insights from Customer & Employee Interviews. At Scale.
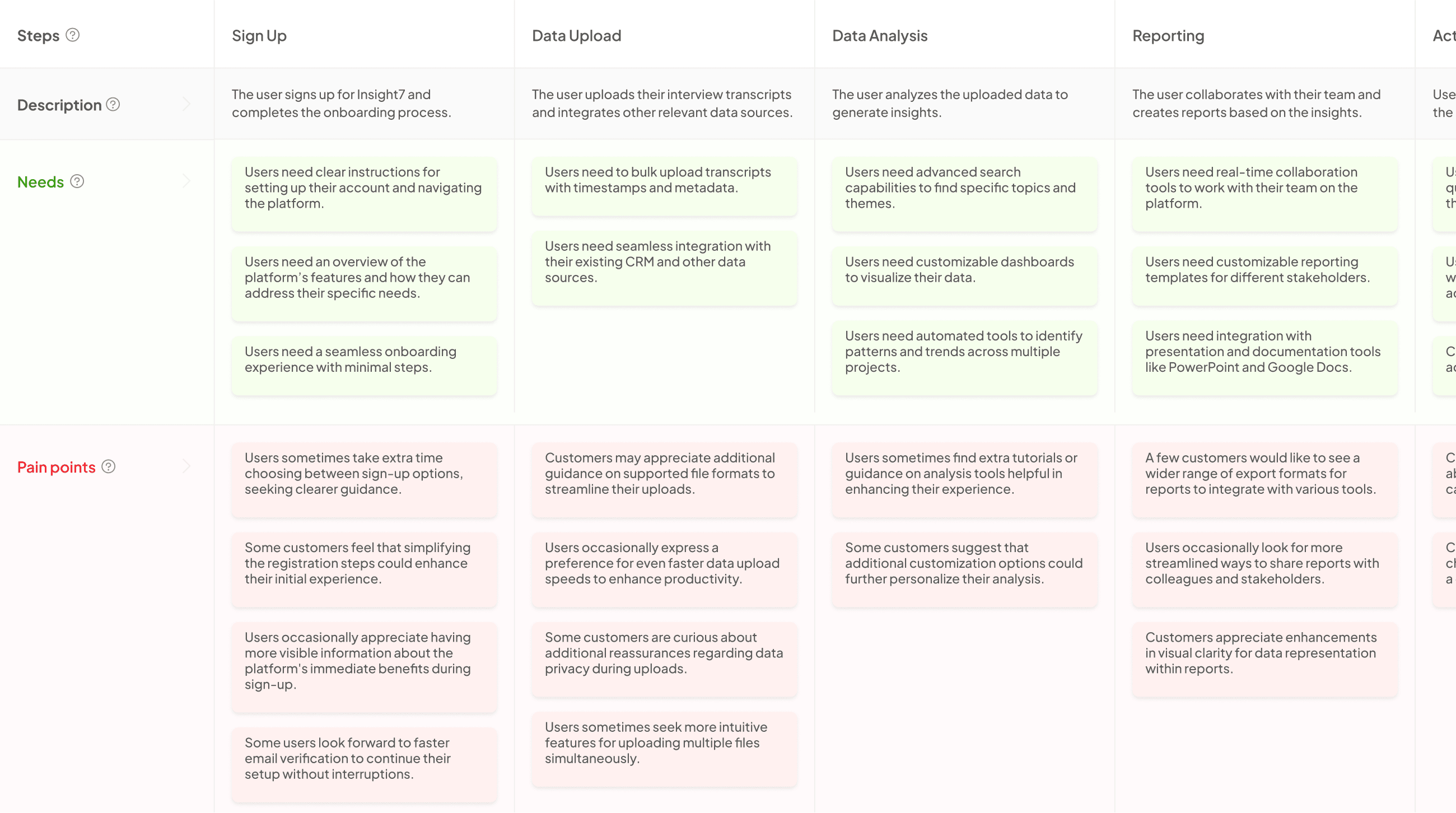
The Role of AI-driven Qualitative Analysis in Processing Interview Data
AI-driven qualitative analysis plays an essential role in streamlining the processing of interview data. This advanced approach not only reduces the time spent on manual analysis but also minimizes bias and inconsistency that often arises when multiple researchers interpret data. Traditional methods can be labor-intensive, leading to delayed insights, which can frustrate both teams and clients seeking timely results. AI solutions can significantly improve efficiency and reliability in uncovering themes and sentiments from interview responses.
By employing AI technologies, researchers can transform raw data into actionable insights with greater accuracy. Natural Language Processing (NLP) tools can quickly identify patterns in conversations, thereby revealing underlying motivations and sentiments that might be overlooked in a manual review. This efficiency empowers teams to deliver impactful findings more rapidly, enhancing the overall quality of qualitative research. Ultimately, adopting AI-driven analysis will not only save time but also enrich the data interpretation process, leading to more informed decision-making.
Breaking Down AI-driven Qualitative Analysis: An Overview
AI-driven Qualitative Analysis plays a vital role in transforming how interview-based surveys are analyzed. By incorporating advanced algorithms, AI streamlines the process of extracting insights from qualitative data, thereby enhancing the speed and accuracy of analysis. Traditionally, researchers faced challenges such as time constraints and inconsistent interpretations among team members. AI mitigates these issues by providing a uniform approach to data analysis, reducing the risk of bias.
Implementing AI-driven Qualitative Analysis allows researchers to focus on meaningful interpretations rather than manual data review. Techniques such as Natural Language Processing (NLP) enable the identification of themes and patterns, which can lead to richer insights. Furthermore, sentiment analysis helps unveil the emotional undertone of responses, offering deeper context. Overall, leveraging AI in qualitative surveys not only saves time but also improves the reliability of findings, thus empowering researchers to deliver timely, data-driven insights to their clients.
- Understanding the Importance of AI in Qualitative Research
AI-driven Qualitative Analysis has emerged as a transformative force in qualitative research. In traditional methods, researchers often face challenges such as bias, inconsistencies, and lengthy analysis times. These hurdles can impede the delivery of timely and accurate insights, which are crucial for informed decision-making. By integrating AI into qualitative research, teams can streamline processes and minimize human error.
Understanding the importance of AI in qualitative research revolves around enhancing data interpretation and ensuring richer insights. AI tools can efficiently analyze vast amounts of interview data by identifying patterns, themes, and sentiments that might elude manual analysis. This not only speeds up the research process but also enables a more cohesive understanding of findings across different team members. Embracing AI-driven Qualitative Analysis fosters a new era of research, allowing for deeper insights and more reliable outcomes.
- How AI Enhances the Interpretation of Interview Responses
AI-driven Qualitative Analysis profoundly transforms how researchers interpret interview responses. By automating and standardizing the analysis process, AI can significantly reduce the time required for manual assessments. With tools powered by natural language processing, researchers can quickly identify themes and sentiment, enhancing the depth of insights drawn from interviews. This capability enables teams to deliver results more swiftly and with less bias, as AI analyzes data consistently across various responses.
Moreover, AI supports researchers by uncovering nuanced patterns that might be overlooked through traditional analysis methods. This level of insight is invaluable when addressing complex qualitative data, as it allows for a richer understanding of participant perspectives. Consequently, integrating AI into the qualitative analysis of interviews not only streamlines processes but also elevates the quality of insights derived, ultimately leading to more informed decision-making and strategic recommendations.
[optional] ### top tools for ai-driven qualitative analysis in survey data- insight7
AI-driven Qualitative Analysis revolutionizes the way we interpret data gleaned from interviews. This method enhances the extraction of meaningful themes and insights from qualitative surveys, enabling researchers to efficiently process large volumes of data. By employing advanced algorithms, AI assists in recognizing patterns and trends that may go unnoticed through manual analysis.
There are critical steps involved in executing AI-driven qualitative analysis. First, it's essential to prepare your data meticulously. This process includes transcribing recorded interviews and ensuring that privacy standards are upheld. Next, applying AI techniques such as Natural Language Processing (NLP) allows for a more nuanced thematic analysis. By focusing on sentiment analysis, researchers can derive emotional undertones, providing richer insights into the subjects’ experiences. Ultimately, AI not only streamlines the analysis but also empowers researchers to make informed decisions based on comprehensive and reliable qualitative insights.
- NVivo
NVivo serves as a powerful tool in the realm of AI-driven qualitative analysis. It streamlines the process of analyzing interview-based surveys by offering a platform that efficiently organizes and analyzes qualitative data. Researchers can import transcripts directly, facilitating a seamless transition from data collection to analysis. This makes it easier to reveal patterns, themes, and insights that might otherwise go unnoticed.
With its intuitive coding capabilities, NVivo allows users to tag sections of text with codes that represent different themes or categories. This not only enhances organization but also aids in retrieving specific information during later stages of analysis. The software’s ability to integrate AI technologies such as natural language processing significantly boosts the depth and accuracy of thematic analysis. Through these features, NVivo empowers researchers to delve deeper into their qualitative data, ultimately transforming raw interview responses into actionable insights.
- ATLAS.ti
ATLAS.ti serves as a powerful tool in the realm of AI-driven qualitative analysis, offering researchers an innovative way to handle interview-based surveys. Its user-friendly interface enables easy management and organization of qualitative data, allowing for a seamless transition from raw insights to structured analysis. Researchers can import texts, audio, and video data, ensuring that they capture the full essence of interviewee responses.
Once the data is in the platform, ATLAS.ti employs advanced coding features to categorize and tag responses systematically. This aids in identifying key themes and patterns that emerge from interviews. Furthermore, AI functionalities facilitate deeper insights through the automatic generation of reports and visualizations. This enhances the overall interpretation of qualitative data, elevating the research quality and driving impactful conclusions. By integrating ATLAS.ti into the research process, analysts can streamline their workflows and enhance their ability to derive actionable insights from qualitative surveys.
- Dedoose
AI-driven Qualitative Analysis often involves using robust tools that streamline the analysis of interview data, enhancing the research process. One such tool offers a comprehensive platform for managing qualitative data. This platform facilitates the uploading of audio and text files, enabling users to efficiently extract themes and insights. Rather than managing piles of documents scattered across different locations, users can gather insights in one central space, improving collaboration and organization.
Additionally, this tool supports native transcription in over 60 languages, allowing for accessible analysis of multilingual interviews. Users can generate reports, visualizations, and other actionable outputs quickly, making data interpretation less overwhelming. By utilizing AI-driven qualitative analysis features, researchers can save time and gain deeper insights from their interview-based surveys. Embracing such technology can lead to more informed business decisions and a clearer understanding of audience needs.
- QDA Miner
QDA Miner serves as a vital tool in the realm of AI-driven qualitative analysis, specifically designed to streamline the analysis of interview-based surveys. This software offers several features that empower researchers to efficiently manage and analyze textual data, transforming raw interview transcripts into actionable insights. Users can leverage its advanced coding capabilities to identify themes and patterns that may not be immediately apparent, thus enhancing the overall comprehension of participant responses.
One of the most significant advantages of QDA Miner is its ability to integrate various types of qualitative data. This allows analysts to draw connections between interview findings and other sources of information, enriching their understanding of the research context. Moreover, the tool's statistical features enable researchers to quantify qualitative data, leading to more robust conclusions. By utilizing QDA Miner, professionals can elevate their qualitative research efforts, making AI-driven qualitative analysis not only faster but also more effective in delivering meaningful insights.
Generate Journey maps, Mind maps, Bar charts and more from your data in Minutes
Steps to Implement AI-driven Qualitative Analysis in Interview Surveys
To implement AI-driven qualitative analysis in interview surveys, start with data preparation. First, transcribe the interviews accurately to ensure the text reflects the respondents' voices. Clean the data by removing irrelevant or sensitive information to maintain consent and privacy. This initial step lays a solid foundation for subsequent analyses, as clean data leads to more reliable insights.
Next, apply AI techniques for analysis. Utilize Natural Language Processing (NLP) to identify themes and patterns in the qualitative data. This technology can process vast amounts of text efficiently, revealing insights that might be missed in manual analysis. Additionally, conduct sentiment analysis to gauge emotional tones and attitudes present in the interviews. By integrating these AI-driven methods, researchers can enhance their understanding of interview responses, leading to quicker and more accurate insights that meet client expectations.
Step 1: Preparing Your Data for AI-driven Qualitative Analysis
To begin your journey into AI-driven qualitative analysis, it is essential to prepare your data adequately. The first step involves a meticulous transcription of your interviews. Transcribing allows you to convert audio recordings into text, creating a solid foundation for subsequent analysis. Ensure that transcripts are accurate and free from errors, as these will directly influence the reliability of your findings.
Next, focus on preprocessing the text data. This may include tasks like removing irrelevant sections, standardizing terminology, and ensuring the anonymity of respondents to uphold data privacy. Additionally, securing informed consent from participants is crucial, as it fosters trust and ensures ethical compliance throughout your research process. By diligently preparing your data, you set the stage for effective AI-driven qualitative analysis, which ultimately enhances the depth and quality of insights derived from your interviews.
- Transcribing Interviews and Preprocessing Text Data
Transcribing interviews accurately is the foundational step in AI-driven qualitative analysis. It allows you to convert spoken words into written text, capturing nuances and meanings essential for analysis. Using automated transcription software streamlines this process and can handle multiple audio and video files simultaneously. Once the interviews are transcribed, it becomes easier to analyze the data collectively and extract themes, quotes, or significant insights.
Preprocessing the text data is equally critical. This step involves cleaning the transcription by correcting errors, removing filler words, and ensuring consistency in formatting. Preprocessed data enhances the effectiveness of AI algorithms in identifying patterns and themes during analysis. By addressing both transcription and preprocessing, researchers can maximize the value of their interview data, leading to clearer insights and informed decision-making based on qualitative surveys.
- Ensuring Data Privacy and Consent
Ensuring data privacy and consent is paramount when leveraging AI-driven qualitative analysis for interview-based surveys. Researchers must prioritize safeguarding participant information, as this fosters trust and ensures ethical compliance. Employing secure platforms that adhere to data privacy regulations, such as GDPR, is crucial. This means all data should be stored in compliant cloud environments, minimizing unauthorized access and breaches.
Obtaining informed consent from participants is another critical step. Clearly communicate the purpose of the research and how their data will be used. Always give participants the option to withdraw their consent at any time. Furthermore, anonymizing data helps protect identities, allowing for meaningful insights without compromising individual privacy. Consistent adherence to these practices not only enhances research reliability but also promotes a respectful and responsible approach to qualitative analysis.
Step 2: Applying AI Techniques to Analyze Qualitative Data
In this stage, the focus is on applying specific AI techniques to enhance the analysis of qualitative data. An essential tool in this process is Natural Language Processing (NLP), which allows researchers to quickly identify themes within interview transcripts. By dissecting vast amounts of text, NLP can pinpoint recurring phrases and concepts that may otherwise go unnoticed. This technique significantly streamlines the thematic analysis, offering insights more rapidly than traditional methods.
Additionally, sentiment analysis plays a crucial role in understanding participant emotions. By evaluating the tone and emotional content of responses, researchers can uncover deeper insights into people's feelings and attitudes towards the subject matter. Overall, incorporating these AI-driven techniques not only increases efficiency but also enhances the quality of qualitative analysis in interview-based surveys, ensuring that key insights are accurately captured and delivered.
- Utilizing Natural Language Processing (NLP) for Thematic Analysis
Natural Language Processing (NLP) is a powerful tool in AI-driven qualitative analysis, particularly for thematic analysis. By harnessing NLP, researchers can efficiently identify patterns and themes within interview responses, saving valuable time and effort. NLP algorithms analyze textual data, extracting significant phrases and clustering similar concepts. This enables researchers to focus on the most relevant insights that align with their objectives.
Thematic analysis using NLP involves a structured approach. First, researchers define their specific goals, such as improving user experience or identifying challenges. Next, the NLP tool categorizes data into themes, producing a clear representation of sentiments and insights. For examples, sentiments on collaboration and knowledge sharing can be extracted, providing rich context and supporting quotes from the interviewees. By incorporating NLP in qualitative analysis, researchers streamline the process while enhancing the accuracy of their findings, ultimately leading to more effective decision-making.
- Conducting Sentiment Analysis to Uncover Emotional Insights
Sentiment analysis plays a pivotal role in AI-driven qualitative analysis by revealing emotional insights from interview-based qualitative surveys. Using advanced algorithms, AI tools can dissect language patterns, identifying sentiments such as positivity, negativity, or neutrality embedded within participant responses. This process transforms raw data into meaningful emotional narratives, allowing researchers to grasp underlying feelings and attitudes that may not be immediately visible.
To conduct effective sentiment analysis, follow these key steps: first, preprocess the interview transcripts to ensure a clean text for analysis. Then, apply AI models that utilize natural language processing to evaluate the sentiment associated with specific phrases. Finally, interpret the findings by correlating emotional insights with the interview context, which facilitates informed decision-making and strategic recommendations. In this manner, AI-driven qualitative analysis not only analyzes the data but also enriches our understanding of the emotional dimensions that shape respondent experiences.
Conclusion: The Future of AI-driven Qualitative Analysis in Research
The evolution of AI-driven qualitative analysis represents a significant shift in how researchers approach interview data. The future promises enhanced efficiency and accuracy, addressing longstanding challenges such as bias and time consumption in manual analysis. As researchers increasingly adopt AI tools, the ability to deliver timely insights will transform the way qualitative data is interpreted, allowing for a more streamlined process that maximizes value.
Moreover, incorporating AI-driven qualitative analysis into research frameworks facilitates deeper insights into complex user behavior. By automating data processing, researchers can focus on deriving meaningful conclusions rather than getting bogged down in the minutiae of analysis. This growing reliance on AI not only signifies a practical advancement but also fosters an insightful dialogue in research that prioritizes human understanding alongside technological innovation.