NLP-Driven Insights are reshaping how researchers interpret qualitative survey data. By applying natural language processing techniques, analysts can uncover hidden patterns, sentiments, and themes within large datasets, transforming raw feedback into valuable information. This advancement allows organizations to understand their audience more comprehensively and make informed decisions based on real customer voices.
Furthermore, NLP technologies enable efficient processing of diverse feedback, eliminating the manual effort typically associated with qualitative analysis. As we explore the capabilities of NLP in survey analysis, we will examine its role, implementation steps, and the tools available for harnessing these innovative insights. Ultimately, embracing NLP is key to staying competitive in todayโs data-driven landscape.
Analyze qualitative data. At Scale.
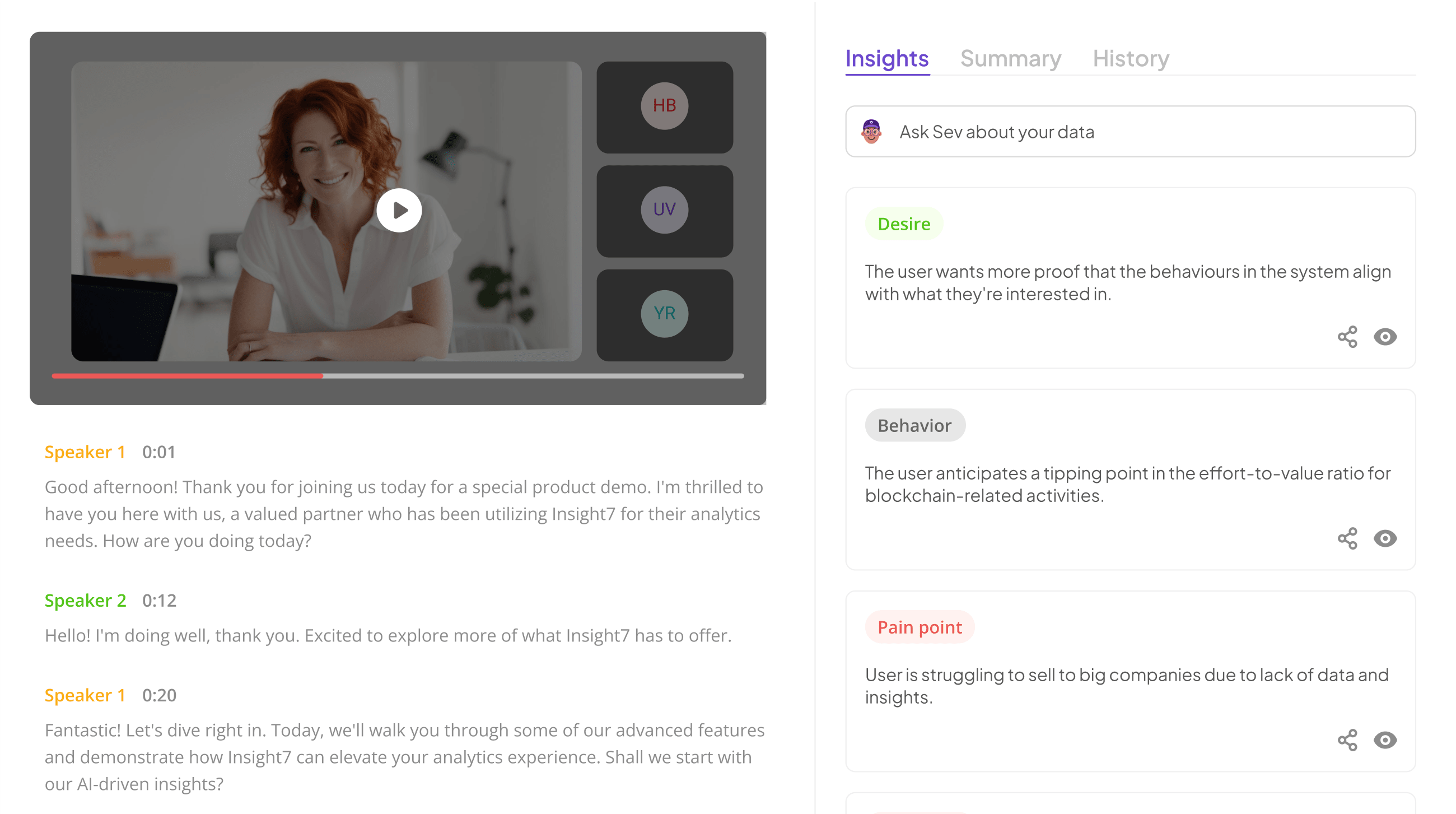
Understanding NLP-Driven Insights in Survey Analysis
NLP-Driven Insights are reshaping qualitative survey analysis by enabling researchers to process and understand large amounts of text data efficiently. Traditional methods of analyzing survey responses often fall short when tasked with unearthing complex sentiments or themes. NLP techniques facilitate this by automating the examination of language used by respondents, making it easier to identify trends, patterns, and varied opinions. This automation not only saves time but enhances the depth of analysis.
To harness NLP-Driven Insights effectively, researchers must focus on key components: data collection, model application, and result interpretation. First, gathering diverse and comprehensive data sets lays the groundwork for a meaningful analysis. Next, applying appropriate NLP models allows for the classification and extraction of relevant information from this data. Finally, interpreting results requires careful consideration to ensure insights drawn are actionable and reflect respondent sentiment accurately. Together, these steps culminate in a more nuanced understanding of qualitative feedback, empowering decision-making processes informed by rich data-driven insights.
The Role of NLP-Driven Insights in Modern Research
NLP-driven insights have emerged as a crucial component in modern research, particularly in qualitative survey analysis. These insights enable researchers to extract meaningful patterns and sentiments from large textual datasets, enhancing traditional methodologies. Instead of manually sifting through endless responses, researchers can utilize NLP to streamline their analyses, making it more efficient and accurate.
By applying NLP techniques, researchers can uncover hidden themes and sentiments within qualitative data. They can identify pain points, customer desires, and behavioral patterns effortlessly. Furthermore, these insights not only support decision-making but also empower organizations to frame strategies grounded in actual user feedback. Ultimately, NLP-driven insights facilitate a more data-informed approach to understanding customer perspectives, significantly transforming the landscape of modern research.
Steps to Implement NLP in Qualitative Analysis
To implement NLP in qualitative analysis effectively, follow a structured approach to harness NLP-driven insights. Begin with Step 1: Data Collection and Preprocessing. Gather qualitative data from multiple sources, such as surveys and interviews. Ensure the data is cleaned and formatted appropriately to enable smooth processing. This step can include removing noise and organizing transcripts for more effective analysis.
Next, move to Step 2: Applying NLP Models. Select suitable NLP models tailored to your analysis objectives. Whether itโs sentiment analysis, topic modeling, or keyword extraction, ensure the model fits the specific nuances of your data. This will allow you to derive relevant insights that cater to your research needs.
Finally, conclude with Step 3: Analyzing and Interpreting Results. Once the models generate actionable insights, it's important to interpret the results within context. Collaborating with your team or stakeholders can enhance understanding and ensure the insights lead to informed decision-making. By following these steps, youโll effectively integrate NLP into your qualitative analysis, unlocking valuable NLP-driven insights.
- Step 1: Data Collection and Preprocessing
Data collection and preprocessing serves as the foundation for obtaining NLP-driven insights in qualitative survey analysis. The initial step involves gathering relevant data from diverse sources such as interviews, feedback forms, and open-ended survey responses. Collecting comprehensive and representative data is crucial, as it determines the quality of insights derived later. As you gather this data, ensure that it is stored in a structured format, which greatly facilitates subsequent analysis.
Once the data is collected, preprocessing is the next critical phase. This includes tasks such as cleaning the dataset by removing duplicates, correcting spelling errors, and standardizing formats. Tokenization, which involves breaking down text into words or phrases, also plays an important role here. This process not only enhances data quality but also prepares it for the application of NLP models in the next steps of analysis. Attention to detail during data collection and preprocessing significantly impacts the quality of insights extracted, ultimately leading to more reliable decision-making.
- Step 2: Applying NLP Models
In Step 2: Applying NLP Models, the transition from data collection to analysis occurs. Here, NLP algorithms are employed to extract meaningful information from qualitative survey responses. The integration of these models enables the transformation of unstructured text into structured insights that drive decision-making.
To effectively apply NLP models in qualitative analysis, consider the following approaches:
Tokenization: This process involves breaking down text into words or phrases, allowing for easier management of the data. Tokenized input can help in identifying relevant themes and sentiments within responses.
Sentiment Analysis: By assessing the emotional tone of responses, sentiment analysis uncovers customers' feelings about your brand. This analysis reveals nuanced perspectives that are key to understanding consumer perceptions.
Topic Modeling: This technique identifies main topics within the data. With topic modeling, researchers can categorize responses, making it easier to identify emerging themes or trends.
Named Entity Recognition (NER): NER helps in spotting specific entities within text, such as names of products or organizations. This recognition allows for focused insights that inform strategic actions.
Employing these NLP-driven insights can significantly enhance qualitative survey analysis and provide invaluable feedback for business development.
- Step 3: Analyzing and Interpreting Results
In this step, we delve into the critical process of analyzing and interpreting results derived from qualitative surveys. A central aspect of this process involves the extraction of key insights using natural language processing (NLP) technologies. The first stage in this analysis entails organizing the textual data, which can involve categorizing responses by themes or pain points. This organized data then sets the foundation for more in-depth analysis, allowing researchers to discern patterns and trends.
NLP-driven insights enable researchers to summarize large volumes of qualitative data effectively. By identifying recurring themes and sentiments, NLP can highlight significant findings that may otherwise go unnoticed. Moreover, visualizing this data through charts or dashboards aids in communicating results clearly and engagingly to stakeholders. Ultimately, the true power of NLP lies in its ability to transform raw qualitative data into actionable insights that inform decision-making processes.
Extract insights from interviews, calls, surveys and reviews for insights in minutes
Top Tools for NLP-Driven Insights in Survey Analysis
The landscape of survey analysis is rapidly evolving, driven by advancements in technology and data processing. Among the most effective methodologies are tools designed for deriving NLP-driven insights. These tools enable organizations to process large volumes of qualitative data efficiently, transforming raw responses into actionable conclusions.
Key tools in this space include MonkeyLearn, which offers an intuitive interface for text analysis, allowing users to categorize and extract insights from surveys with ease. Lexalytics specializes in sentiment analysis, providing deep insights into customer emotions and attitudes, essential for understanding feedback. NLTK and SpaCy are powerful libraries that facilitate natural language processing tasks and are favored by data scientists for their flexibility and comprehensive features.
Together, these tools empower businesses to uncover trends and patterns in customer feedback, driving better decision-making and ultimately leading to enhanced customer experiences. Embracing NLP-driven insights is no longer an option but a necessity in today's competitive market.
Insight7 and its Unique Capabilities
Insight7 stands out in the domain of qualitative survey analysis through its advanced NLP-driven insights. By focusing on the unique capabilities of this platform, users can effectively interpret large volumes of customer interactions, which have traditionally been cumbersome to analyze. The platform facilitates a self-service experience, enabling businesses to understand customer conversations at scale without the need for extensive technical expertise.
One of the primary strengths of Insight7 is its capacity to streamline the process of generating actionable insights from unstructured data. This not only accelerates the analysis but also enhances collaboration among team members by consolidating insights into a single, accessible platform. As organizations increasingly generate vast amounts of qualitative data, Insight7 equips them with the tools needed to transform these data points into strategic business actions efficiently. The integration of an intuitive interface with sophisticated NLP techniques ensures that organizations can stay ahead of the competition by quickly grasping changing customer needs and preferences.
Other Leading Tools for Transforming Survey Analysis
Numerous tools exist today to enhance survey analysis through innovative technologies and methodologies. Among these, MonkeyLearn stands out for its user-friendly interface, enabling businesses to automate the categorization of open-ended survey responses. By using its customizable algorithms, users can generate NLP-driven insights more swiftly than traditional methods. This efficiency in processing data allows organizations to stay agile by responding quickly to emerging trends in customer sentiment.
Lexalytics, another robust platform, excels in sentiment analysis and text mining. It assists organizations in extracting meaningful insights from customer feedback by identifying keywords, themes, and sentiment shifts across large datasets. NLTK and SpaCy are powerful libraries suited for developers who prefer to code their solutions. With extensive functionalities for natural language processing tasks, they empower companies to tailor their survey analysis to specific needs, thus fostering deeper understanding and actionable business strategies. Collectively, these tools illustrate the transformative potential of advanced analysis techniques in addressing the challenges of qualitative research.
- Tool 1: MonkeyLearn
MonkeyLearn is a powerful tool designed to harness NLP-driven insights, transforming how qualitative survey data is analyzed. With a user-friendly interface, it democratizes access to valuable insights, allowing anyone within an organization to extract significant information from qualitative responses. Users can seamlessly upload audio files or text data, making it easy to generate reports or analyze customer experiences.
The platform employs advanced algorithms to identify key themes, pain points, and sentiments within the data. This allows teams to uncover actionable insights without requiring specialized training. By leveraging these capabilities, organizations can visualize conversations, identify trends, and derive evidence-backed conclusions, ensuring that every voiceโcustomer feedback and employee testimonials alikeโcontributes to informed decision-making. This process not only enriches the analysis but also fosters a culture of data-driven innovation.
- Tool 2: Lexalytics
The power of syntactic and semantic understanding found in Lexalytics enhances qualitative survey analysis. This tool simplifies the process of extracting meaningful feedback from vast amounts of text data, enabling organizations to convert customer voices into actionable strategies. Users can easily upload audio or text files, which the platform then transforms into comprehensive reports, uncovering core themes and issues found in customer interactions.
Lexalytics excels in identifying pain points, desires, and patterns within the data, allowing businesses to respond promptly to customer needs. The user-friendly interface supports everyone, from marketing teams to researchers, in harnessing NLP-driven insights effortlessly. This accessibility fosters a culture of informed decision-making, where insights are not confined to specialists alone but are available to all stakeholders. With tools like Lexalytics, organizations can confidently navigate the complexities of qualitative data analysis and enhance their customer engagement strategies.
- Tool 3: NLTK
NLTK, or Natural Language Toolkit, is a powerful tool widely used for natural language processing tasks. This library enables users to work with human language data effortlessly, making it ideal for qualitative survey analysis. By utilizing NLTK, analysts can preprocess text data, enabling them to extract meaningful insights from open-ended survey responses with greater ease.
One of the notable features of NLTK is its vast collection of text processing libraries. These libraries facilitate tokenization, stemming, and tagging, which are essential for breaking down textual data into manageable components. Additionally, the ability to visualize historical queries allows researchers to identify trends and themes over time. This helps transform raw survey data into NLP-driven insights that guide strategic decision-making. Overall, NLTK empowers researchers to derive in-depth understanding from qualitative data, significantly enhancing the value of survey analysis.
- Tool 4: SpaCy
SpaCy is a powerful library that plays a key role in extracting NLP-driven insights from qualitative survey data. This tool allows researchers to quickly preprocess and analyze textual information, significantly enhancing their ability to uncover patterns and trends. By providing an intuitive interface, SpaCy simplifies complex tasks such as entity recognition, part-of-speech tagging, and dependency parsing.
When using SpaCy, researchers can streamline their survey analysis process by following a structured approach. First, they can load their textual data into SpaCy, ensuring it is ready for processing. Next, applying pre-trained NLP models facilitates tasks like sentiment analysis and topic modeling. Finally, researchers can visualize the results, helping them communicate findings more effectively. Embracing SpaCy in qualitative analysis not only accelerates the research process but also leads to deeper, actionable insights that can inform decision-making.
Conclusion on Harnessing NLP-Driven Insights
In conclusion, harnessing NLP-driven insights transforms the way qualitative survey analysis is conducted. By utilizing advanced algorithms, organizations can now efficiently extract meaningful patterns and sentiments from large data sets, leading to improved decision-making. This technology democratizes data accessibility, allowing various stakeholders to interact with insights directly, without needing specialized training.
Moreover, the ability to visualize and synthesize information fosters a deeper understanding of customer experiences and pain points. As businesses continue to integrate NLP-driven insights into their processes, they will uncover valuable opportunities for growth and innovation, ultimately enhancing their strategic initiatives.