The text analysis process is essential for extracting meaningful insights from large volumes of text data. It starts with data collection, where relevant text is gathered from various sources. This initial step is crucial as the quality and relevance of the data collected significantly impact the analysis's effectiveness. Organizations often collect feedback, surveys, and social media comments, creating a robust dataset for analysis.
Next comes data preprocessing, which involves cleaning and organizing the data. This step may include removing irrelevant information, correcting spelling mistakes, and standardizing formats. By refining the dataset, analysts enhance the accuracy of subsequent techniques, such as text classification and sentiment analysis. Ultimately, a well-structured text analysis process can yield valuable insights and inform strategic decisions across various sectors. Understanding and correctly executing this process enhances a company's ability to respond effectively to customer needs and trends.
Introduction to the Text Analysis Process
In todayโs data-driven world, understanding text analysis is crucial for extracting valuable insights. The text analysis process serves as a structured approach that transforms unstructured text into meaningful information. This transformation not only helps organizations make informed decisions but also enhances their communication strategies.
Beginning with data collection, this process identifies relevant sources and gathers textual data for analysis. Following collection, data preprocessing ensures that the text is cleaned and formatted, ready for deeper exploration. By following these initial steps, businesses can better understand trends, themes, and sentiments in their data, unlocking opportunities for improved engagement and strategy development.
Generate visualizations from your qualitative data. At Scale.
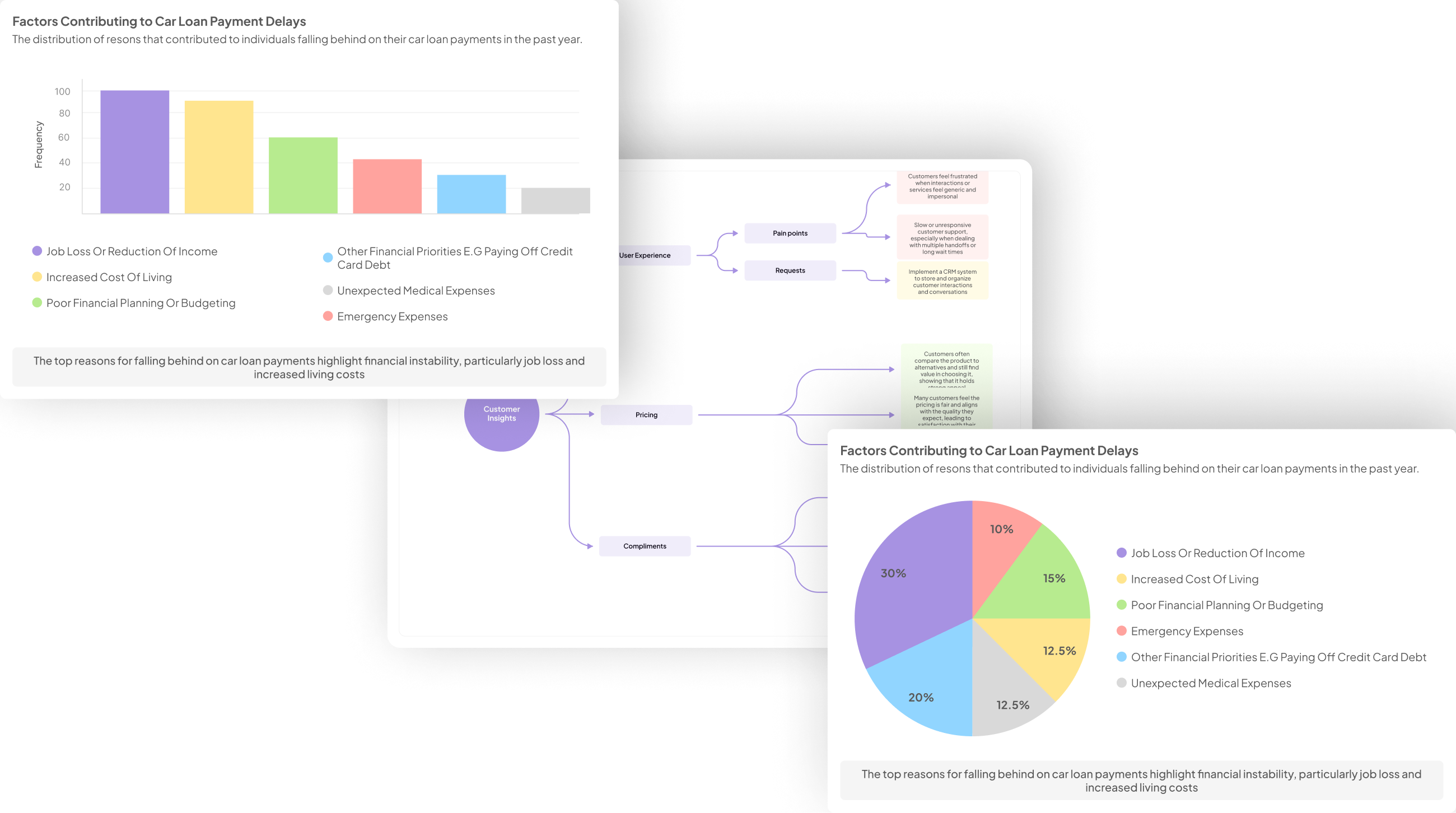
Understanding the Text Analysis Process
The Text Analysis Process begins with recognizing the need for systematic examination of textual data. Understanding this process is crucial for extracting actionable insights from vast amounts of unstructured text. It typically involves several key stages, starting with data collection, where relevant texts are gathered from various sources. These sources can include customer feedback, social media, or internal documents.
Once the data is collected, the next phase is data preprocessing. This step is critical as it cleans and prepares the text for analysis. It includes activities like removing noise, correcting errors, and standardizing formats. Following preprocessing, the actual analysis can occur, employing techniques such as text classification and sentiment analysis. This methodical approach ensures that organizations can convert text into meaningful information, ultimately driving informed decision-making and strategic initiatives. By understanding the Text Analysis Process, businesses can harness the power of text analytics effectively.
Key Steps in the Text Analysis Process
The Text Analysis Process begins with several key steps that ensure effective and meaningful insights from textual data. Understanding these steps helps users navigate through the complexities of text analytics.
First, data collection is fundamental. This step involves gathering relevant textual data from various sources such as documents, social media, or customer feedback. It is crucial to ensure the collected data is comprehensive and representative to yield accurate analyses.
Next comes data preprocessing. In this stage, the raw text is cleaned and transformed. This may include removing unnecessary characters, correcting spelling errors, and standardizing formats. Proper preprocessing enhances the efficiency of subsequent analytical techniques, such as text classification and sentiment analysis, allowing for deeper insights to be uncovered. By following these foundational steps, organizations can significantly enhance the quality and reliability of their text analysis efforts.
Step 1: Data Collection
To begin the text analysis process, effective data collection is essential. This initial step involves gathering relevant textual data from various sources, ensuring that the dataset is both comprehensive and representative. Common sources include customer feedback, social media posts, survey responses, and product reviews. Selecting diverse input ensures a well-rounded perspective, which enhances the overall quality of insights obtained from subsequent analysis.
During this phase, it is important to consider the specific objectives of the text analysis. Establishing clear goals will guide the data collection process, helping to identify what type of information is necessary. Once the data is collected, it can be organized and stored for easy access during later stages. This structured approach not only streamlines the text analysis process but also lays the groundwork for deriving valuable insights that can inform business decisions and strategies.
Step 2: Data Preprocessing
Data preprocessing is a crucial step in the text analysis process that prepares raw data for analysis. This phase involves cleaning and transforming unstructured text data into a structured format that can be easily interpreted. Data may include everything from customer reviews to social media comments, often filled with inconsistencies, noise, and irrelevant information. Thus, effective preprocessing is essential to improve the accuracy and relevance of further analysis.
Key activities in this stage include tokenization, where text is broken into smaller components like words or phrases. Additionally, removing stop wordsโcommon words that do not add significant meaningโhelps streamline the dataset. Stemming and lemmatization are other techniques used to reduce words to their base forms. By meticulously refining the data, the preprocessing phase ensures that the text analysis process is efficient, paving the way for more accurate insights and data-driven decisions.
Generate Journey maps, Mind maps, Bar charts and more from your data in Minutes
Techniques in the Text Analysis Process
In the text analysis process, various techniques are employed to uncover insights from data. One fundamental technique is text classification, which involves categorizing text into predefined groups based on its content. This method enables analysts to organize large datasets efficiently, making it easier to extract relevant information.
Another critical technique is sentiment analysis, which assesses the emotional tone behind words. By evaluating user opinions and feedback, this methodology helps businesses understand customer feelings toward their brand or product. The combination of these techniques enhances the overall effectiveness of the text analysis process, enabling organizations to make informed decisions based on data-driven insights. As a result, mastering these methods is essential for anyone looking to harness the power of text analytics effectively.
Text Classification
Text classification plays a vital role in the overall text analysis process. This step involves categorizing textual data into predefined categories or labels. By systematically analyzing text, it becomes easier to derive meaningful insights. It allows businesses to automate insights extraction from vast amounts of data, such as customer reviews, emails, or support tickets.
Various techniques can be employed in text classification, such as supervised and unsupervised learning. In supervised learning, a model is trained on a labeled dataset, enabling it to predict categories for new, unseen data. Meanwhile, unsupervised learning helps discover hidden patterns in the data without predefined labels, revealing insights that may not be immediately obvious. By implementing these approaches effectively, organizations can streamline their decision-making processes and enhance engagement strategies for their audiences.
Sentiment Analysis
Sentiment analysis plays a crucial role in the text analysis process by identifying and categorizing emotions expressed within a text. It allows organizations to understand public opinion, customer feelings, and overall sentiment toward products and services. This analysis transforms qualitative data into quantitative insights that inform strategic decisions.
To conduct sentiment analysis effectively, there are several key steps. First, data preprocessing is essential; this includes removing noise, handling missing values, and normalizing text. Next, sentiment extraction methods, such as rule-based approaches or machine learning algorithms, can be employed to assess sentiment polarityโpositive, neutral, or negative. Finally, visualizing the results helps stakeholders understand the broader emotional landscape, guiding future actions based on consumer sentiment. By incorporating sentiment analysis into the text analysis process, businesses can gain valuable insights and enhance customer engagement strategies.
Conclusion of the Text Analysis Process
The conclusion of the text analysis process serves as a vital summary of the critical steps involved in extracting insights from text data. By reviewing the techniques and methods used, it allows for a comprehensive understanding of how raw data transforms into actionable insights. Each stage, from data collection to preprocessing and analysis, contributes to the overall effectiveness of the text analysis.
Ultimately, the text analysis process offers valuable perspectives that can inform decision-making and strategy. With the proper tools and techniques, organizations can efficiently analyze vast amounts of text data, turning it into meaningful information that drives improvement and growth. This whole process emphasizes the importance of clarity and focus in text analytics, ensuring stakeholders can utilize insights effectively.
### Understanding the Text Analysis Process
The text analysis process begins with data collection, which is crucial for deriving insights. During this step, raw text data is gathered from various sources, such as surveys, social media, or customer feedback. The type of data collected greatly influences the quality of insights generated later on. Itโs essential to have a comprehensive dataset to ensure meaningful analysis.
Next, data preprocessing comes into play. This stage involves cleaning and organizing the data, removing any irrelevant information while also standardizing formats. Essential techniques during preprocessing include tokenization, which breaks sentences into individual words, and stop-word removal, which excludes common words that do not add value to the analysis. This step lays the foundation for more advanced techniques, such as text classification and sentiment analysis, leading to actionable insights for decision-making.