How Do Forecasting Tools Use AI to Model Volume Variability?
-
Bella Williams
- 10 min read
AI-Driven Volume Forecasting has emerged as a transformative approach to predicting demand fluctuations across various industries. With the capability to analyze vast datasets rapidly, businesses can gain insights into customer behavior and market changes that were previously unattainable. By employing sophisticated algorithms, these forecasting tools can effectively model volume variability, enabling organizations to make informed decisions.
The journey into AI-Driven Volume Forecasting begins by harnessing data from both internal and external sources. Internal data, such as historical sales figures, enhances accuracy, while external factors, including market trends and consumer sentiment, further refine predictions. This targeted analysis sets the stage for effective resource allocation and strategic planning, making forecasting not just an operational tool but a critical component of overall business strategy.
Transcribe & extract insights from interviews. At Scale.
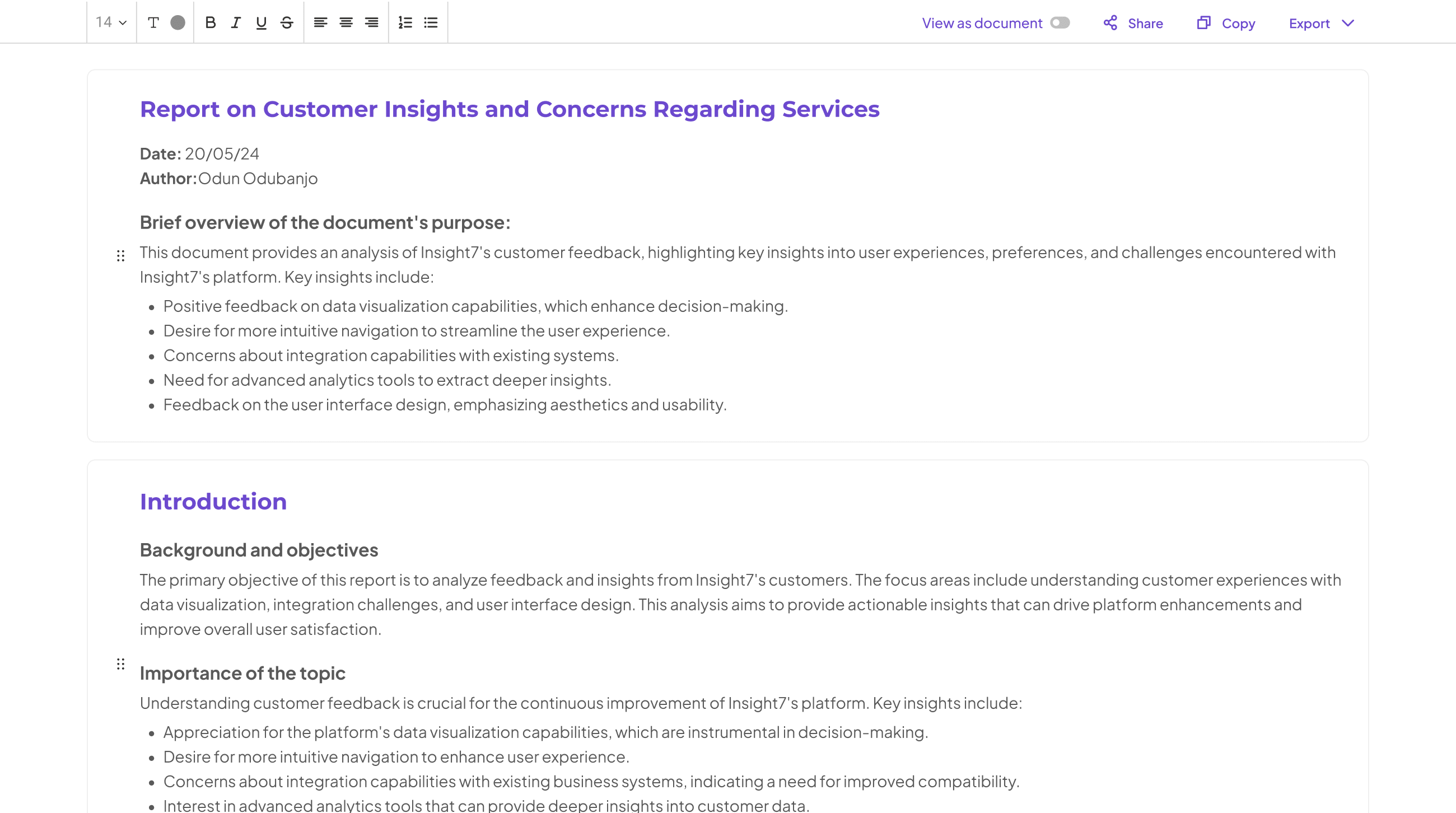
Understanding AI-Driven Volume Forecasting Mechanisms
AI-Driven Volume Forecasting mechanisms rely on sophisticated algorithms and data integration techniques to accurately predict fluctuations in volume. These systems harness both historical and real-time data, evaluating various factors that can influence trends. Machine learning plays a crucial role here, allowing models to learn from past data and continuously improve their predictions. The integration of supervised and unsupervised learning methods ensures that forecasting becomes more accurate over time.
Understanding how data sources contribute to forecasting accuracy is essential. Internal data, such as sales records and customer interactions, provides a solid foundation for predictions. Additionally, incorporating external factors, like market trends and economic indicators, can significantly enhance the models. Together, these elements form a comprehensive approach to AI-Driven Volume Forecasting, enabling businesses to respond more effectively to changes in demand.
Role of Machine Learning Algorithms in Volume Forecasting
Machine learning algorithms play a pivotal role in AI-driven volume forecasting by enhancing accuracy and adaptability in predicting fluctuations. These algorithms analyze vast datasets to identify underlying patterns that traditional methods might overlook. Utilizing supervised learning techniques, these models can systematically predict volume trends based on historical data, ensuring businesses stay ahead of variable market demands.
Unsupervised learning also plays a crucial part in this process by clustering data points to reveal hidden patterns. This capability allows organizations to understand complex relationships in their data, improving their overall forecasting strategy. As these models continuously learn from new inputs, they adapt in real-time, providing more insightful forecasts. Consequently, the application of machine learning in volume forecasting transforms how businesses plan their inventory and resources, driving efficiency and profitability.
- Exploring Supervised Learning for Predicting Volume Trends
Supervised learning is a cornerstone of AI-driven volume forecasting, providing the foundation for predictive analytics. This method involves training a model using historical data to recognize patterns that can inform future volume trends. In this way, businesses can obtain valuable insights into their operations, enabling more accurate demand forecasts. The approach often employs a variety of algorithms, including linear regression, decision trees, and support vector machines, to establish clear relationships between input variables and volume outcomes.
The predictive power of supervised learning lies in its continuous feedback loop. By comparing predicted results against actual outcomes, the model is refined to enhance accuracy over time. This iterative process allows organizations to adjust their strategies based on emerging patterns and trends, ultimately driving better decision-making. As companies harness AI-driven volume forecasting, they position themselves to navigate market fluctuations with greater confidence and agility. Through this strategic application of supervised learning, businesses can gain a significant competitive edge in understanding and predicting consumer demands.
- Utilizing Unsupervised Learning to Recognize Volume Patterns
Unsupervised learning plays a vital role in identifying volume patterns, particularly in the context of AI-Driven Volume Forecasting. By analyzing large datasets without pre-labeled information, unsupervised learning algorithms can uncover hidden trends and structures. These algorithms group similar data points, enabling analysts to discern patterns that may indicate fluctuations in volume, allowing businesses to react with informed strategies.
There are several key methods within unsupervised learning that facilitate the recognition of volume patterns. First, clustering techniques group similar instances together, which helps in identifying distinct volume categories. Next, dimensionality reduction methods simplify complex data, highlighting relevant features that influence volume variability. Finally, anomaly detection algorithms can flag unusual data points, signaling potential issues or opportunities. Collectively, these techniques empower organizations to develop a nuanced understanding of their volume trends and enhance forecasting accuracy.
Data Sources and Their Impact on Forecast Accuracy
In AI-Driven Volume Forecasting, the selection and integration of data sources play a crucial role in achieving accurate predictions. Internal data sources, such as historical sales records and inventory levels, are fundamental for creating a reliable forecasting model. This data captures past performance trends and can highlight seasonal variations, which informs future forecasts. The ability to analyze this historic internal data allows businesses to adjust their strategies proactively.
Equally important are external data factors, which introduce new variables that impact volume variability. Market trends, competitor actions, and economic indicators can shift demand patterns suddenly, making it essential to integrate such external insights into forecasting models. By combining both internal and external data sources, AI-driven tools can enhance the accuracy of predictions, helping organizations navigate complexities in demand forecasting effectively. This comprehensive approach ultimately leads to more informed decision-making and improved business outcomes.
- Internal Data Integration for Enhanced Predictions
Integrating internal data effectively is critical for enhancing predictions in AI-Driven Volume Forecasting. Organizations often gather vast amounts of operational data, including sales, inventory levels, and customer interactions. By synthesizing this data, companies can establish clearer trends that inform forecasting algorithms. This integration ensures that predictions are based on comprehensive datasets, reducing inaccuracies that arise from limited information.
Furthermore, internal data integration fosters a seamless flow of insights across departments. When teams collaborate using a unified data platform, they can refine forecasting models more rapidly and align their strategies effectively. This holistic view of data not only optimizes the use of resources but also enables quick adaptations to market changes. Ultimately, internal data integration lays the foundation for AI tools to generate precise volume forecasts and keep organizations agile and competitive.
- The Influence of External Data Factors on Volume Variability
External data factors play a crucial role in shaping volume variability, impacting how businesses forecast demand. These factors encompass trends in market dynamics, economic indicators, and even seasonality. By integrating this external data into forecasting models, organizations can achieve improved accuracy in their volume predictions and respond effectively to changing market conditions.
Key external factors include economic trends, which reflect consumer behavior shifts, and competitor actions, influencing market share and demand. Additionally, social media sentiment can provide real-time insights into public perception and emerging trends. By employing AI-driven volume forecasting, businesses can harness these external influences, creating models that adapt to real-time data. This ensures that forecasts remain relevant and resilient, allowing companies to navigate uncertain environments with confidence. Understanding and incorporating these external data factors ultimately facilitates more reliable and actionable insights into volume variability.
Generate Detailed Reports from Your Qualitative Data in Minutes.
Key Tools Implementing AI-Driven Volume Forecasting
AI-Driven Volume Forecasting relies on cutting-edge tools that streamline the forecasting process. Several key tools are leading the way in implementing sophisticated AI methodologies to accurately predict volume fluctuations. These tools utilize advanced algorithms and machine learning techniques, enabling users to gain deeper insights into their data.
First, Tool A offers distinctive features that cater to specific industry needs. Its ability to analyze historical trends supports strategic decision-making. Tool B excels in integrating data from various sources, enhancing accuracy in volume projections. Tool C utilizes advanced algorithms that dynamically adjust forecasts based on real-time data inputs, offering a proactive approach. Finally, Tool D provides comprehensive solutions across various industries, ensuring adaptability and resilience in forecasting efforts.
By adopting these state-of-the-art tools, businesses empower themselves to navigate volume variability effectively, leveraging AI to stay ahead in a competitive landscape.
๐ฌ Questions about How Do Forecasting Tools Use AI to Model Volume Variability??
Our team typically responds within minutes
insight7: Pioneering AI Solutions in Volume Forecasting
AI-Driven Volume Forecasting is transforming how businesses anticipate demand and manage inventory. By utilizing advanced algorithms, these innovative solutions model complex data and identify trends, enabling organizations to make informed decisions. Pioneering tools in this space harness machine learning and big data to enhance forecasting accuracy, providing a significant advantage in todayโs fast-paced market.
One of the primary benefits of AI-driven volume forecasting is its ability to integrate various data sources. Internal datasets, such as historical sales, alongside external factors like market trends, enable more precise predictions. By analyzing this vast array of information, these pioneering solutions can rapidly adapt to changing conditions, thereby reducing risks associated with stockouts or overstocking. Ultimately, mastering AI-driven volume forecasting equips businesses with the agility necessary to thrive in an unpredictable environment.
Other Leading Forecasting Tools
In the realm of AI-driven volume forecasting, several leading tools stand out, showcasing unique features and methodologies. Tool A excels by integrating robust algorithms designed specifically for AI-based predictive analytics and offers real-time data updates. These capabilities significantly enhance forecasting accuracy, making it an appealing choice for organizations focused on continuous improvement.
Tool B distinguishes itself through its exceptional data utilization strategies, capturing diverse data sources to refine volume predictions. It employs advanced learning techniques that adapt to market changes, ensuring reliable forecasts. Additionally, Tool C stands out for incorporating advanced algorithms that account for various influencing factors, providing insights into volume variability across sectors. Finally, Tool D offers comprehensive solutions tailored to different industries, demonstrating flexibility and effectiveness in handling volume forecasts. Each of these tools contributes to the evolving landscape of AI-driven volume forecasting, empowering businesses to navigate complexities with confidence.
- Tool A: Features and Benefits in AI Volume Forecasting
Tool A offers a robust suite of features tailored for AI-driven volume forecasting, making it an essential tool for businesses aiming to optimize their predictive capabilities. One key feature is its advanced machine learning algorithms, which can analyze vast datasets to identify trends and forecast volume with remarkable accuracy. This capability enables organizations to anticipate fluctuations, allowing for proactive decision-making and inventory management.
Additionally, Tool A benefits from seamless integration with various data sources, both internal and external. This connection enriches the forecasting process by providing a comprehensive view of factors affecting volume variability, such as market trends and consumer behavior. Leveraging these insights, businesses can enhance their planning and strategy, ultimately leading to better operational efficiency and increased profitability. By employing this tool, companies position themselves to stay competitive in a dynamic market environment, ensuring they can adapt quickly to changes in demand.
- Tool B: Leveraging Data for Accurate Volume Predictions
Accurate volume predictions are essential for effective business operations. Tool B focuses on how data plays a critical role in enhancing these forecasts. By harnessing vast amounts of historical and real-time data, AI-driven volume forecasting can identify trends, seasonality, and anomalies that influence product demand.
To achieve accurate volume predictions, businesses should consider the following key aspects:
Data Quality: High-quality data is fundamental. Poor or inconsistent data can lead to erroneous forecasts.
Integration of Diverse Data Sources: Merging internal data, such as sales records and customer feedback, with external factors like market trends or economic indicators maximizes forecasting accuracy.
Analytical Techniques: Utilizing machine learning algorithms allows businesses to uncover patterns and insights that traditional methods may overlook.
Continuous Learning and Adaptation: Forecasting tools should incorporate mechanisms to evolve with changing market conditions. This adaptation ensures predictions remain relevant and reliable over time.
By focusing on these elements and leveraging data effectively, organizations can enhance their AI-driven volume forecasting capabilities.
- Tool C: Advanced Algorithms for Modeling Volume Variability
Advanced algorithms play a crucial role in modeling volume variability, providing businesses with enhanced capabilities for precise forecasting. By employing sophisticated AI techniques, organizations can analyze vast amounts of data to identify patterns and trends that traditional methods may overlook. This increased accuracy not only helps in planning but also enables companies to respond effectively to market fluctuations.
One pivotal aspect of these advanced algorithms is their ability to integrate various data sources, both internal and external. This allows for a more comprehensive view of factors impacting volume variability. Furthermore, they utilize machine learning, which empowers the system to adapt and evolve based on new data trends. Ultimately, using AI-driven volume forecasting equips businesses with the tools to make informed decisions, keeping them competitive in ever-changing market conditions.
- Tool D: Comprehensive Solutions for Diverse Industries
Incorporating AI-Driven Volume Forecasting provides comprehensive solutions that cater to the diverse needs of various industries. Organizations across sectors are increasingly recognizing the importance of accurately predicting volume variability to enhance decision-making. These AI-driven tools utilize advanced algorithms and data analytics to create models that respond dynamically to fluctuating market conditions.
To achieve effective volume forecasting, several key components come into play. First, data integration from multiple internal sources helps establish a robust framework for analysis. Second, leveraging real-time external data further refines these predictions, allowing industries to adapt quickly to trends and shifts. Third, a user-friendly interface enhances accessibility, ensuring that teams can easily interpret insights and translate them into actionable strategies. Such comprehensive solutions empower businesses to stay competitive and responsive, ultimately leading to improved operational efficiency and customer satisfaction.
Conclusion: The Future of AI-Driven Volume Forecasting
As we look to the future of AI-Driven Volume Forecasting, the potential for transformative advancements appears limitless. Enhanced algorithms and increasingly sophisticated data integration techniques will likely improve the accuracy of forecasts significantly. The blending of vast internal and external data sources will enable organizations to understand volume variability with unprecedented clarity and precision.
Furthermore, as AI technology evolves, we can expect forecasting tools to not only predict volume trends but also offer actionable insights tailored to individual business needs. This shift will empower companies to make data-informed decisions swiftly, leading to optimized operations and improved customer satisfaction. Ultimately, the future of AI-Driven Volume Forecasting promises to reshape how industries approach forecasting challenges.
๐ฌ Questions about How Do Forecasting Tools Use AI to Model Volume Variability??
Our team typically responds within minutes