How Can AI Predict SLA Risk Based on Call Volume Forecasts?
-
Bella Williams
- 10 min read
SLA Risk Prediction is an essential strategy for companies managing call centers. As call volumes fluctuate, understanding how these variations impact Service Level Agreements (SLAs) becomes crucial. Accurate predictions can enable organizations to allocate resources effectively, improve customer satisfaction, and mitigate potential risks early. AI-driven insights derived from call volume forecasts can empower leaders to anticipate service challenges before they escalate.
Furthermore, implementing an SLA Risk Prediction model necessitates an understanding of the underlying data trends. When organizations leverage AI to analyze historical call volumes, they can identify patterns that indicate potential SLA breaches. This proactive approach not only enhances response strategies but also fosters a culture of accountability among customer service teams. By focusing on predictive analytics, businesses can navigate challenges and optimize operational efficiency.
Transcribe & extract insights from interviews. At Scale.
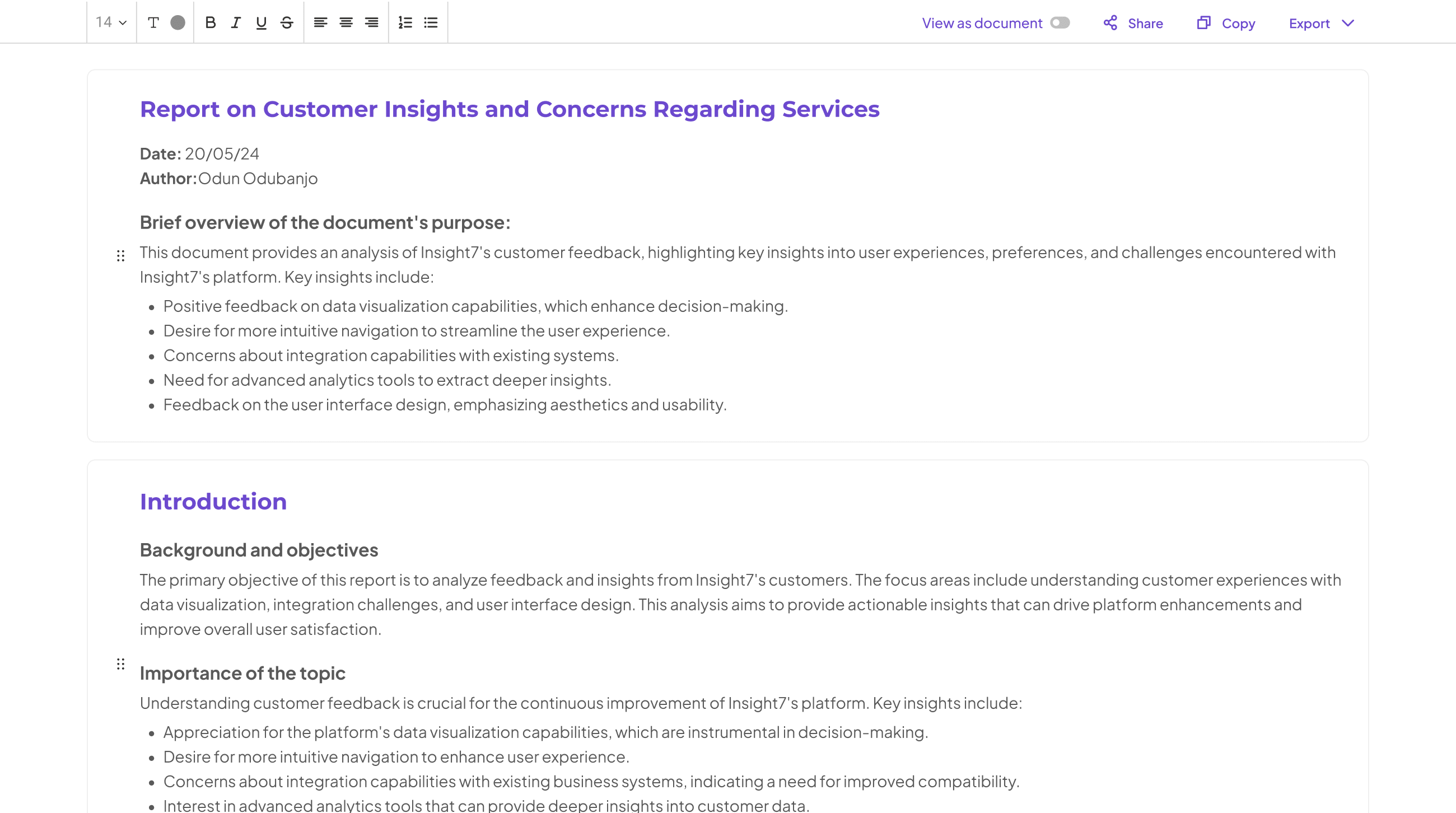
Understanding SLA Risk Prediction in Call Centers
Understanding SLA Risk Prediction is essential for call centers aiming to maintain high service levels. SLA Risk Prediction refers to the assessment of potential service-level agreement breaches based on various factors, including call volume forecasts. By integrating data analytics and AI, call centers can anticipate periods of high call volume, which increases the risk of SLA violations.
Predicting SLA risks helps management to allocate resources more effectively and plan for customer demand. A proactive approach to risk prediction can mitigate the impact of extreme call fluctuations, ensuring that customer satisfaction remains high. It also provides insights into workforce management, enabling teams to fine-tune their operations based on real-time data. Businesses that effectively incorporate SLA Risk Prediction into their strategy will not only enhance service delivery but also build trust with their customers through reliability and efficiency.
Why Predicting SLA Risk is Crucial
Predicting SLA risk is vital for maintaining efficient operations within call centers. By forecasting potential challenges, organizations can proactively address issues that may compromise service quality. Such predictions allow businesses to allocate resources effectively, ensuring customer queries are resolved promptly, thus preventing potential breaches in service level agreements (SLAs).
Understanding SLA risk prediction enhances not only customer satisfaction but also operational performance. When teams are aware of anticipated fluctuations in call volume, they can implement targeted strategies to bolster service capacity. This foresight minimizes the likelihood of missed deadlines and effectively safeguards the organization against potential penalties. Ultimately, effective SLA risk prediction fosters a more resilient service operation, ensuring that teams are better prepared to meet customer needs regardless of demand fluctuations.
Factors Influencing SLA Risk Prediction
SLA risk prediction is influenced by several key factors, including call volume, customer demand patterns, and service level agreements. Understanding these variables helps organizations anticipate potential issues and implement strategies proactively. Monitoring call volume forecasts can reveal trends, allowing for timely adjustments to staffing and resource allocation. The nature of incoming calls, whether high-urgency or routine inquiries, also plays an integral role in predicting SLA risks.
Additionally, the performance history of agents provides valuable insights. Historical data assists in understanding past challenges and identifying areas that require improvement. Training and support for customer service representatives can significantly affect SLA compliance. Furthermore, external factors, such as market trends and economic conditions, can influence call volumes, thereby impacting SLA risk prediction. Effective management of these factors enables organizations to maintain high service levels while minimizing the risk of SLA breaches.
Role of AI in SLA Risk PredictionGenerate Detailed Reports from Your Qualitative Data in Minutes.
Generate Detailed Reports from Your Qualitative Data in Minutes.
AI technology plays an essential role in SLA risk prediction, particularly in call centers. By utilizing advanced machine learning algorithms, AI can effectively analyze historical call volume data. This analysis enables organizations to predict potential SLA breaches before they occur, allowing for proactive management and intervention. Integrating AI into SLA risk prediction enhances decision-making by providing insights that are data-driven, timely, and reliable.
To implement AI-driven SLA risk prediction, organizations must follow a systematic approach. First, collecting and preprocessing relevant data is crucial for ensuring accuracy. Next, developing predictive models involves selecting algorithms that best fit the organization's data characteristics. Finally, continuously monitoring and optimizing these predictions ensures that the system adapts to changing conditions, refining its accuracy over time. Through these steps, AI not only enhances SLA risk prediction but also helps maintain service quality and customer satisfaction.
How AI Analyzes Call Volume Forecasts
AI plays a significant role in analyzing call volume forecasts, which is essential for predicting SLA risk. By assessing historical call data, AI algorithms can identify patterns and trends that may indicate future call volumes. This analysis helps organizations allocate resources effectively, ensuring they meet service level agreements (SLAs) while maintaining customer satisfaction. Additionally, AI's ability to process vast amounts of data in real-time gives it an edge over traditional methods, allowing for swift adjustments to staffing and operational strategies.
The analysis begins with data collection, where AI gathers information from previous call records. It then preprocesses this data to remove inaccuracies and noise. Following this, predictive models are developed, using various algorithms to simulate different scenarios. As AI continuously monitors outcomes, it refines its forecasts based on real-time inputs, enhancing the accuracy of SLA risk predictions. This closed feedback loop is crucial for adapting to changing call patterns and minimizing SLA breaches effectively.
Steps for Implementing AI-Driven SLA Risk Prediction
To implement AI-driven SLA risk prediction effectively, begin by collecting and preprocessing data. Gather call volume data, customer interactions, and agent performance records. Cleanse this data to remove any inconsistencies or errors. Itโs essential that the data is accurate, as this serves as the foundation for your predictive models.
Next, move on to developing predictive models. Utilize machine learning algorithms that can analyze historical call volume trends and identify potential SLA risks. Train these models on your preprocessed data to enhance their accuracy. Finally, continuously monitor the AIโs predictions and optimize the models based on new incoming data. This iterative process allows for improved accuracy in SLA risk prediction over time. By following these steps, businesses can ensure they anticipate SLA challenges effectively, enhancing service delivery and customer satisfaction.
Step 1: Collect and Preprocess Data
To effectively predict SLA risk, the first step involves collecting and preprocessing the necessary data. Begin by gathering historical call volume data, which serves as the foundation for analysis. This data should be comprehensive, covering various time frames and incorporating relevant metrics such as call duration, response times, and ticket resolution rates. Ensuring that this dataset is robust and accurate is crucial for reliable SLA risk prediction.
Once the data is collected, it must be preprocessed to eliminate inconsistencies and errors. This stage includes cleaning the dataset, normalizing values, and addressing any missing information. Through these preparations, the data becomes suitable for analytical models. Ultimately, this foundational step sets the tone for accurate SLA risk prediction by ensuring that the insights drawn from call volume forecasts are based on quality data.
Step 2: Develop Predictive Models
In this step, the focus shifts to developing predictive models that can accurately assess SLA risk based on call volume forecasts. Effective predictive modeling is crucial in identifying potential issues before they become critical. The process involves selecting appropriate algorithms, processing historical data, and continuously refining models for accuracy.
Start by gathering relevant data, such as previous call volumes, response times, and resolution rates. Analyzing this data with machine learning techniques helps in uncovering patterns that may indicate SLA risks. Once you have established a foundation, choose from various models, such as regression analysis or decision trees, to predict future call volumes and potential SLA violations.
๐ฌ Questions about How Can AI Predict SLA Risk Based on Call Volume Forecasts??
Our team typically responds within minutes
Lastly, continuously evaluate these models against real-time data to ensure their effectiveness. By adopting a systematic approach in developing these predictive models, organizations can proactively manage SLA risks, ultimately enhancing service quality and customer satisfaction.
Step 3: Monitor and Optimize Predictions
To effectively monitor and optimize SLA risk prediction, it is essential to establish a feedback loop. This loop facilitates continuous learning from historical data and real-time metrics. By regularly reviewing the accuracy of predictions against actual call volume and SLA adherence rates, organizations can identify discrepancies and adjust models accordingly. This process not only enhances the reliability of predictions but also ensures that teams proactively address potential SLA breaches.
Moreover, implementing advanced analytics allows teams to refine their understanding of factors influencing SLA outcomes. Leveraging insights from call data, such as peak volume times and customer feedback, can significantly enhance predictive accuracy. Continuous optimization is vital, as it allows for the applied experience of monitoring trends and adapting models. As a result, organizations can respond adeptly to changing demands and mitigate SLA risks effectively, ensuring a better alignment with service delivery expectations.
Tools for SLA Risk Prediction in Call Centers
In the realm of call centers, effective SLA Risk Prediction necessitates the deployment of advanced tools designed to analyze call data and forecast potential risks. A variety of tools ensure that call centers can monitor their performance against set service level agreements (SLAs). Notably, platforms such as IBM Watson Analytics and Google Cloud AI facilitate deep insights into call volumes and patterns. These tools utilize machine learning algorithms to detect anomalies and anticipate spikes in call traffic, enabling proactive measures to be put in place.
To enhance SLA Risk Prediction, call centers should consider a mix of tools tailored to their specific requirements. Firstly, tools like Amazon SageMaker allow for the creation of custom machine learning models that can adapt to varying call scenarios. Secondly, Microsoft Azure AI offers robust analytics capabilities to assess data trends effectively. These tools not only streamline operations but also empower decision-makers with actionable insights, ensuring SLAs are consistently met and enhancing overall customer satisfaction.
insight7
The integration of AI into SLA risk prediction is transforming how call centers manage customer interactions. By analyzing call volume forecasts, AI can identify potential risks before they impact service levels, enabling proactive management. The ability to predict SLA breaches enhances operational efficiency and customer satisfaction, making rapid response essential.
Understanding the variables that determine SLA risk prediction is crucial. Factors such as historical call volume data, agent availability, and customer behavior patterns all play a role. AI systems can assimilate these data points, forecasting trends and identifying potential disruptions. By adopting AI-driven predictive models, organizations are better equipped to make informed decisions. This structured approach not only minimizes risks but cultivates a responsive environment, ultimately leading to improved customer experiences.
IBM Watson Analytics
IBM Watson Analytics offers a powerful platform for businesses aiming to enhance their understanding of SLA risk prediction. By analyzing call volume forecasts, this tool provides actionable insights into customer interactions. This capability is essential for identifying potential SLA risks that could impact service delivery.
The analytics engine interprets large sets of data, allowing users to visualize trends and anomalies in call patterns. As a result, organizations can proactively address potential issues before they escalate. By harnessing this tool, teams can gain clarity on customer feedback and operational performance, leading to well-informed decisions that mitigate SLA risk. The integration of advanced AI features facilitates deeper analysis, ensuring that businesses stay ahead in managing their service commitments effectively.
Google Cloud AI
In the realm of SLA risk prediction, Google Cloud AI plays a pivotal role in enhancing operational efficiency. By utilizing advanced machine learning algorithms, it enables organizations to accurately forecast call volumes. This predictive capability is essential for managing resources and minimizing potential SLA breaches. The technology analyzes historical call data, identifies patterns, and predicts future call trends, ensuring that proactive measures can be taken to maintain service levels.
Moreover, integrating Google Cloud AI allows for seamless data processing and real-time insights. This ensures that companies can quickly adapt to changing conditions and customer demands. With these insights, businesses can implement strategic adjustments, preventing SLA risks before they materialize. As organizations embrace this technology, they gain a competitive edge in maintaining customer satisfaction and operational reliability, making Google Cloud AI invaluable for effective SLA risk prediction in call centers.
Amazon SageMaker
Amazon SageMaker offers a robust platform for implementing SLA risk prediction based on call volume forecasts. This service simplifies the development and deployment of machine learning models, enabling call centers to analyze historical call data effectively. With its built-in algorithms and frameworks, organizations can quickly create predictive models tailored to their unique needs in assessing SLA risks.
The platform also supports various data preprocessing techniques, essential for preparing call volume data for analysis. By leveraging its intuitive interface, users can monitor model performance and optimize predictions continuously. As call volumes fluctuate, Amazon SageMaker empowers businesses to adapt and mitigate SLA risks, ensuring they maintain service quality even during peak demand periods. Ultimately, utilizing this tool enhances decision-making, helping manage SLA risk prediction while aligning operational capabilities with customer expectations.
Microsoft Azure AI
Microsoft Azure AI significantly enhances SLA risk prediction by leveraging advanced machine learning algorithms and data analytics capabilities. It provides organizations with the tools necessary to analyze call volume forecasts effectively, helping predict potential service level agreement risks. This predictive approach allows businesses to proactively address any issues before they affect service delivery or customer satisfaction.
To implement SLA risk prediction using Microsoft Azure AI, organizations should follow a structured approach. First, they must collect and preprocess relevant data, including historical call volume and SLA performance metrics. Next, predictive models can be developed, using Azure's robust machine learning capabilities to uncover patterns and trends that may indicate SLA risks. Finally, regular monitoring and optimization of these predictions ensure that the models remain accurate and relevant, enabling teams to respond swiftly to any anticipated service challenges. By integrating Microsoft Azure AI, organizations can empower themselves with data-driven insights, enabling enhanced service quality and compliance assurance.
Conclusion: Enhancing SLA Risk Prediction Through AI and Forecasting
AI has the potential to significantly enhance SLA risk prediction by using advanced forecasting techniques. By accurately analyzing call volume data, organizations can identify trends and potential risks early, allowing for proactive measures to meet service level agreements. This predictive capability transforms raw data into impactful insights, which can inform staffing decisions and resource allocations.
Utilizing AI algorithms not only increases the accuracy of SLA risk prediction but also streamlines operational efficiency. As companies continue to adopt such technologies, they can create a responsive framework that adapts to fluctuating demands. Ultimately, marrying AI with effective forecasting will lead to improved compliance and customer satisfaction, promoting sustained growth and success.
๐ฌ Questions about How Can AI Predict SLA Risk Based on Call Volume Forecasts??
Our team typically responds within minutes