Call volume forecasting is an essential capability in workforce management, especially for businesses experiencing fluctuations in customer inquiries. As customer behavior evolves and seasonal patterns emerge, accurately predicting call demand becomes crucial for maintaining service quality and operational efficiency. This is where call volume forecasting comes into play, using advanced techniques to analyze historical data and identify trends.
Understanding call volume forecasting empowers managers to plan the workforce effectively, ensuring optimal staffing during peak times. This not only enhances customer satisfaction but also maximizes productivity and minimizes costs. By tapping into the power of AI, organizations can anticipate call spikes and make data-driven decisions that enhance their workforce strategies and improve overall service delivery.
Analyze qualitative data. At Scale.
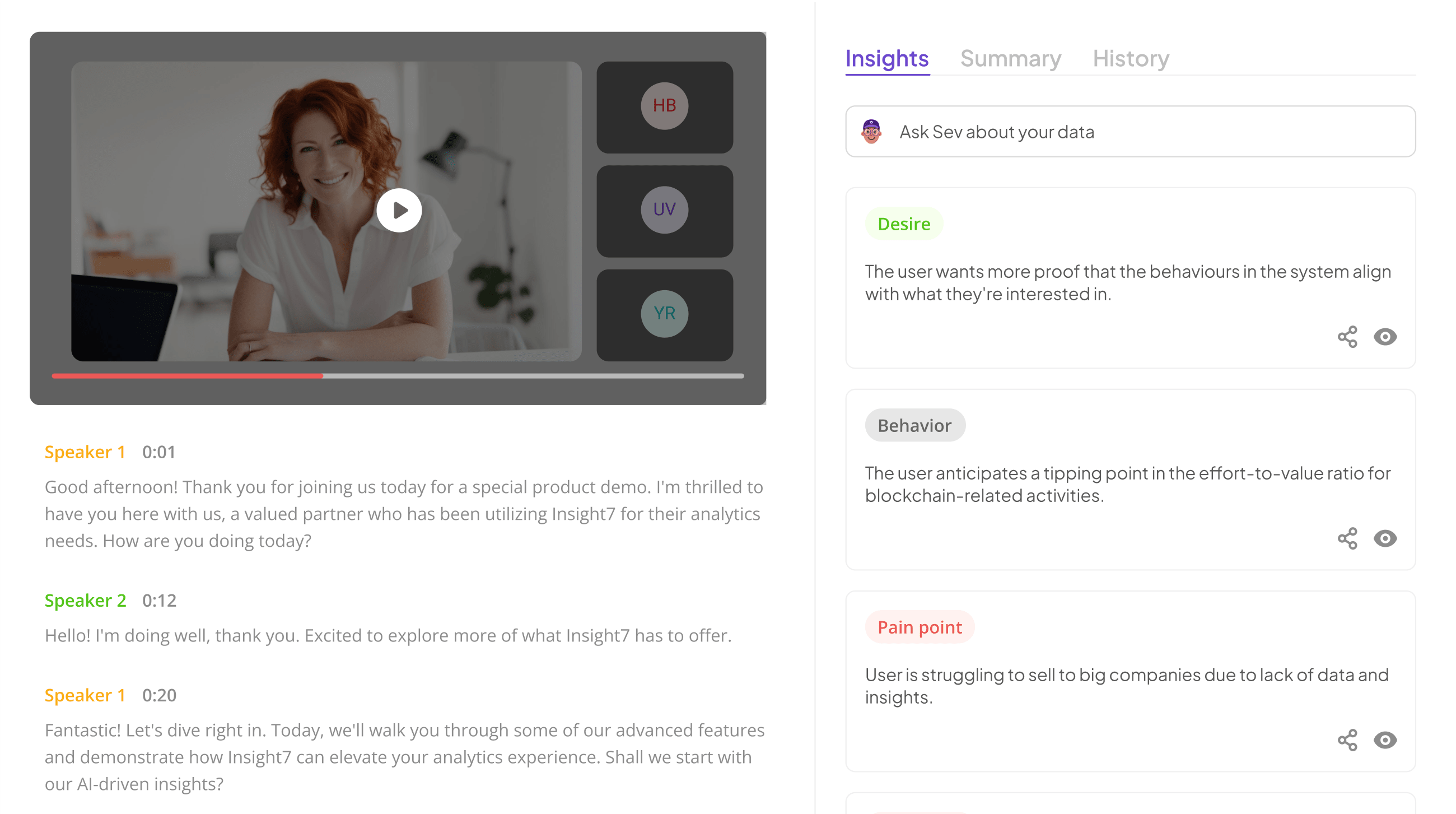
The Science Behind Call Volume Forecasting
Call Volume Forecasting relies on the meticulous analysis of various data points to predict future call patterns effectively. By examining historical data, businesses can identify trends and seasonal fluctuations that significantly impact call volumes. Employing advanced machine learning algorithms enhances these predictions by sifting through massive datasets, uncovering hidden insights that traditional methods might overlook. Such an analytical approach enables organizations to remain responsive to customer needs and optimize their resource allocation accordingly.
Understanding call volume spikes is essential for effective workforce management. Predictive analytics allows teams to anticipate busy periods driven by specific events or seasonal changes. This foresight not only prepares organizations for surges in customer inquiries but also contributes to improved customer satisfaction. Ultimately, leveraging data metrics and AI technology in call volume forecasting empowers businesses to streamline operations and allocate resources efficiently, paving the way for enhanced service delivery.
Utilizing Data Analytics for Accurate Predictions
Data analytics plays a crucial role in call volume forecasting, allowing businesses to anticipate customer needs and effectively manage resources. By examining historical data trends, organizations gather insights into past call patterns and fluctuations. This analysis provides a foundation for forecasting future demand, enabling teams to prepare for high-volume periods.
Incorporating machine learning algorithms enhances the forecasting process through predictive analytics. These algorithms learn from data trends and improve over time, offering increasingly accurate predictions. Additionally, by assessing external factors like marketing campaigns or seasonal events, businesses can adjust their staffing needs accordingly. By utilizing data analytics effectively, companies can not only refine their call volume forecasts but also optimize their workforce scheduling to meet customer demands efficiently. This ensures a more responsive and flexible operation that enhances overall customer satisfaction.
- Historical Data Analysis
Analyzing historical data is crucial for effective call volume forecasting. By examining past call records, businesses can identify significant patterns and trends that inform future predictions. Historical analysis enables teams to anticipate variations in call demand, whether due to seasonal changes or special events. These insights help organizations strategically allocate resources to manage customer inquiries efficiently.
Several key components enhance the effectiveness of historical data analysis. First, compiling a comprehensive dataset allows for a robust analysis, yielding more accurate predictive models. Second, visualizing these data sets can reveal underlying trends that may not be readily apparent through raw numbers. Lastly, using advanced analytical methods, such as machine learning, strengthens prediction accuracy, making it easier to adjust workforce scheduling in real-time. Historical data provides a solid foundation for understanding call volume dynamics, leading to improved operational efficiency and enhanced customer service.
- Machine Learning Algorithms
Machine learning algorithms play a pivotal role in enhancing call volume forecasting, a crucial aspect for effective workforce scheduling. These algorithms analyze historical call data to identify patterns, allowing businesses to predict future call volumes accurately. By leveraging these insights, organizations can better prepare their workforce for anticipated spikes, improving customer service and reducing wait times.
Various machine learning techniques can be applied to optimize forecasting. For instance, time series analysis captures trends over time, while regression models help assess the impact of external factors on call volume. Neural networks can further refine these forecasts by recognizing complex patterns within the data. As a result, businesses can make informed decisions about staffing needs and resource allocation, ultimately leading to improved operational efficiency and customer satisfaction.
Identifying Call Volume Spikes
Monitoring call volume spikes is essential for effective workforce management. By analyzing patterns and trends, organizations can identify when call volumes rise unexpectedly, ensuring that staffing levels meet customer needs. Seasonal factors, like holidays or special events, often drive these spikes, emphasizing the importance of timely call volume forecasting.
To effectively recognize these fluctuations, several key strategies can be employed. First, historical data analysis can uncover past call volume trends, helping predict future spikes. Additionally, machine learning algorithms enhance forecasting accuracy by processing large sets of variables such as time of year and specific events. By leveraging these insights, organizations can respond swiftly to increased call volumes and allocate resources efficiently, thereby optimizing their workforce and improving overall service quality. Ensuring that the right number of agents are available during peak periods enhances customer satisfaction significantly.
- Patterns and Trends
Understanding the patterns and trends in call volume is crucial for effective forecasting. Call volume forecasting leverages historical data to identify fluctuations, enabling organizations to prepare for upcoming spikes. Analyzing past call data reveals consistent patterns that inform proactive workforce scheduling decisions. By examining this data, it becomes possible to detect trends stemming from different factors, such as seasonal variations or marketing campaigns.
Recognizing these call volume trends can significantly enhance workforce efficiency. For instance, events or promotions may lead to predictable increases in call volume, requiring adjustments in staffing. By accurately forecasting these trends, organizations can optimize their workforce allocation and maintain service quality during peak times. Data-driven insights not only prepare teams for expected fluctuations but also build a responsive environment. This approach ensures that resources are aligned with real-time demands, enhancing overall service delivery and operational efficiency.
- Seasonal and Event-Driven Spikes
Seasonal and event-driven spikes in call volume can significantly impact workforce management. Organizations typically experience fluctuations based on holidays, seasonal trends, and special events, which must be accurately forecasted to optimize scheduling. Call volume forecasting is vital for anticipating these spikes and ensuring adequate staff coverage, allowing for efficient customer service delivery.
To better understand these spikes, consider three main factors:
Seasonality: Certain times of the year naturally drive increased call volumes, such as tax season or holidays. Understanding trends from previous years aids in preparing for future surges.
Events: Specific eventsโlike product launches, sales promotions, or regulatory changesโcan trigger unexpected spikes in call volume. Identifying these events early can guide resource allocation.
Market Dynamics: Broader economic conditions can influence customer behavior, impacting when and why customers reach out. Staying informed about these trends ensures a proactive response.
By focusing on these factors, organizations can enhance their approach to call volume forecasting, facilitating more effective workforce scheduling and meeting customer demands efficiently.
Extract insights from interviews, calls, surveys and reviews for insights in minutes
How AI Optimizes Workforce Scheduling
AI plays a crucial role in optimizing workforce scheduling by enhancing the allocation of resources based on dynamic call volume forecasting. By analyzing historical data, AI can identify patterns that inform managers about staffing needs during peak hours and slack periods. This proactive approach allows businesses to adequately prepare for expected call volume spikes, ensuring that customer satisfaction remains high.
There are several key steps involved in AI-driven workforce scheduling. First, data collection and analysis facilitate a comprehensive understanding of call trends. Next, real-time adjustments enable teams to respond quickly to unexpected changes in call volume, thus minimizing wait times. Finally, balanced workforce allocation ensures that the right number of agents is available to meet varying demand levels, ultimately leading to both operational efficiency and improved service delivery. With AI, organizations can innovate their scheduling processes and significantly enhance their responsiveness to customer needs.
Steps to Streamline Scheduling with AI
To streamline scheduling with AI, begin with effective data collection and analysis, a crucial first step in call volume forecasting. By aggregating historical data, organizations can identify patterns and trends that inform staffing needs. Employing machine learning algorithms enhances accuracy, allowing for better predictions of peak periods and ensuring the right number of agents are available to respond to anticipated call volumes.
Next, implement real-time adjustments based on ongoing data insights. This dynamic approach allows organizations to respond promptly to unexpected call spikes or drops. Finally, ensure balanced workforce allocation by distributing resources effectively across various departments. This method not only maintains service quality but also optimizes operational efficiency, making sure that customer demands are met without overwhelming staff. These steps collectively harness AIโs capabilities to enhance scheduling processes and improve overall service delivery.
- Data Collection and Analysis
Data collection and analysis serve as crucial components in the domain of call volume forecasting. By utilizing historical data, organizations can uncover patterns that inform future predictions. Historical call records offer insights into customer behavior, allowing AI algorithms to identify trends and forecast spikes effectively. This analysis is instrumental in optimizing workforce scheduling, ultimately ensuring that staffing levels match anticipated demand.
The process involves several key steps. First, organizations need to gather extensive datasets, including call logs, customer feedback, and external factors such as seasonal trends or significant events. Next, these datasets undergo a thorough analysis using advanced machine learning algorithms, which sift through the information to pinpoint factors driving fluctuations in call volumes. By understanding these dynamics, businesses can deploy their workforce more strategically, enhancing productivity and service delivery while maintaining customer satisfaction.
- Real-Time Adjustments
Real-time adjustments are crucial to enhancing the effectiveness of workforce scheduling. When call volume forecasting indicates unexpected spikes, organizations must respond swiftly to meet customer demand. Leveraging AI enables teams to monitor and analyze data in real time, allowing for immediate staffing adjustments. For example, if an increase in calls is detected, managers can quickly reallocate resources to ensure adequate coverage, minimizing customer wait times.
Moreover, the ability to adjust schedules on the fly not only improves service levels but also boosts employee satisfaction. When staff are deployed effectively based on real-time data, they can manage workloads more efficiently. In this manner, organizations can maintain a delicate balance between operational efficiency and employee well-being. Overall, incorporating real-time adjustments into workforce scheduling strategies significantly enhances the responsiveness of customer service teams, ensuring they are prepared for any fluctuations in call volume.
- Balanced Workforce Allocation
A well-executed balanced workforce allocation strategy is crucial for the success of call centers. Call volume forecasting enables organizations to predict when spikes in call traffic will occur, allowing them to allocate resources effectively across shifts and locations. By understanding historical patterns and real-time data, managers can ensure that adequate staff is available to meet customer demand.
To achieve optimal allocation, organizations can focus on three essential factors: staffing flexibility, skill-based assignments, and location-based distribution. Staffing flexibility allows adjustment of shifts based on anticipated call volumes, adapting to unpredictable peaks. Skill-based assignments enable the right representatives to handle specific inquiries, leading to faster resolution times. Finally, location-based distribution considers the geographical spread of call centers, ensuring that support is closest to areas with high caller volumes. These strategies empower organizations to maintain efficiency and enhance customer satisfaction during peak periods.
Top AI Tools for Call Volume Forecasting and Scheduling
Effective call volume forecasting and scheduling hinge on the precise deployment of AI tools tailored to predict and manage fluctuations in customer interactions. Numerous AI solutions can enhance these processes by analyzing historical data, identifying patterns, and adjusting workforce allocations in real time. With these advanced tools at your disposal, businesses can mitigate the impact of unexpected spikes in call volume, ensuring that customer demands are met promptly and efficiently.
Notable AI tools for call volume forecasting include Five9, known for its predictive capabilities; NICE inContact, which excels in workforce optimization; and Talkdesk, recognized for its user-friendly interface. Additionally, Genesys and RingCentral offer comprehensive solutions that integrate seamlessly with existing systems. By leveraging these technologies, organizations can create dynamic scheduling models that not only improve efficiency but also enhance customer satisfaction. As AI continues to advance, its role in workforce management will only grow, transforming how businesses engage with customers during peak times.
- insight7
Understanding call volume forecasting is crucial in today's fast-paced business environment. It involves leveraging sophisticated algorithms and historical data to predict call trends. With AI becoming increasingly integral, businesses can identify potential spikes caused by seasonal trends or unexpected events. By accurately forecasting these call volumes, organizations can better prepare their teams for fluctuations, ensuring timely responses to customer needs.
Moreover, AI-driven insights promote more efficient workforce scheduling. This process entails not only analyzing past call data but also adjusting in real time to meet demand. Consequently, companies can allocate their workforce more effectively, enhancing operational efficiency and customer satisfaction. As this technology evolves, it will further transform how businesses approach call volume forecasting, ultimately leading to smarter, more responsive customer service strategies.
- Five9
In todayโs fast-paced business environment, effective call volume forecasting is crucial for optimizing workforce scheduling. Sophisticated AI algorithms analyze historical data, revealing patterns that help predict call volume spikes. These insights are essential for call centers aiming to enhance service quality and respond effectively to fluctuating demand.
When integrated into workforce management systems, advanced call volume forecasting allows businesses to make real-time adjustments. By evaluating data trends, organizations can allocate resources more efficiently, ensuring they have the right number of agents available when call volumes rise. This proactive approach can significantly improve customer experience and operational efficiency, as staff members are well-prepared to handle peak periods. Ultimately, embracing this technology transforms how businesses manage their operations, resulting in greater scalability and improved performance.
- NICE inContact
NICE inContact is a powerful platform designed to enhance customer engagement and optimize workforce management. Its robust capabilities focus on call volume forecasting, providing organizations with insights to anticipate customer needs effectively. By utilizing vast amounts of data, the platform analyzes historical call trends to identify patterns that can predict potential call spikes.
Through intelligent algorithms, NICE inContact helps businesses streamline their workforce scheduling. This not only ensures that there are enough agents available during peak times but also optimizes team efficiency. By accurately forecasting call volume, organizations can allocate resources wisely, minimizing costs while maximizing customer satisfaction. This balance supports organizations in delivering a high-quality customer experience, even in fluctuating demand periods, which is essential in todayโs dynamic service environment.
In summary, NICE inContact's call volume forecasting capabilities empower businesses to navigate complexities in customer service, enhancing responsiveness and operational effectiveness.
- Talkdesk
Call volume forecasting relies heavily on advanced data techniques to predict when spikes in customer inquiries will occur. It assesses various factors, from historical call metrics to external influences like seasonal trends and special events. Organizations harness these insights to proactively manage their workforce, ensuring they have the right number of agents available at peak times.
By utilizing AI-driven analytics, companies can adapt their staffing models based on real-time data. This involves continuously assessing call patterns and employee performance metrics, leading to timely workforce adjustments. As businesses aim to enhance customer satisfaction, the ability to effectively anticipate call volume changes becomes crucial. Ultimately, effective forecasting not only drives operational efficiency but also positions businesses to respond adeptly to customer needs, fostering loyalty and satisfaction.
- Genesys
An effective call volume forecasting system is vital for optimizing workforce scheduling. This technology harnesses predictive analytics to anticipate peak call times, allowing organizations to allocate resources efficiently. By analyzing historical call data and patterns, companies can identify when spikes are likely to occur. Such foresight ensures that adequate staffing levels meet customer demands without incurring excess labor costs.
Incorporating AI-based tools enhances this process significantly. Machine learning models can identify trends in customer behavior, enabling a more dynamic approach to scheduling. Businesses can make real-time adjustments to their workforce based on evolving call patterns, thus maintaining service quality during high-demand periods. This proactive strategy not only improves customer experience but also boosts employee satisfaction by preventing overwork during busy times. Ultimately, the integration of robust forecasting capabilities into workforce management leads to a more agile and responsive service environment.
- RingCentral
In a modern workforce, optimizing efficiency during call volume spikes is essential. This is where effective call volume forecasting comes into play. With the use of advanced analytics, organizations can predict fluctuations in call traffic with remarkable precision. By analyzing historical data, businesses can identify patterns and trends, enabling them to prepare for anticipated increases in call volume.
These predictions empower managers to allocate resources effectively across locations. For instance, real-time insights allow for adjustments in staffing levels, ensuring that customer service representatives are available when needed most. As a result, organizations can enhance customer satisfaction without overstaffing during quieter periods. This approach not only maximizes productivity but also reduces operational costsโproving the value of integrating AI-driven call volume forecasting into workforce management strategies.
Conclusion: The Future of Call Volume Forecasting and Workforce Optimization
As advancements in technology progress, the future of call volume forecasting looks more promising than ever. By harnessing the power of artificial intelligence, organizations can gain deeper insights into call patterns, allowing for more precise predictions. This capability not only helps in forecasting call volume spikes but also optimizes workforce scheduling, ensuring that adequate staff is available during peak times.
Moreover, the integration of machine learning and data analytics will continue to enhance these forecasting models. With improved algorithms and real-time data analysis, businesses can swiftly adjust their operations. Embracing these innovations will empower organizations to create a more responsive and efficient service environment tailored to their customersโ needs.