In the realm of large-scale research, vast amounts of data can often become overwhelming. This complexity drives the need for innovative solutions that streamline data organization and analysis. AI Tagging Enhancement plays a pivotal role in transforming how researchers handle and interpret large datasets. By automating the tagging process, AI systems can generate meaningful metadata, allowing teams to focus on deeper insights rather than getting lost in data management.
The integration of AI Tagging Enhancement not only simplifies the data workflow but also improves the accessibility of information. With intelligently assigned tags, researchers can swiftly locate and cross-reference relevant studies and findings. As AI continues to evolve, its capabilities in efficiently enhancing research methods will redefine how scholars explore, analyze, and present their work, leading to richer and more informed conclusions.
Analyze & Evaluate Calls. At Scale.
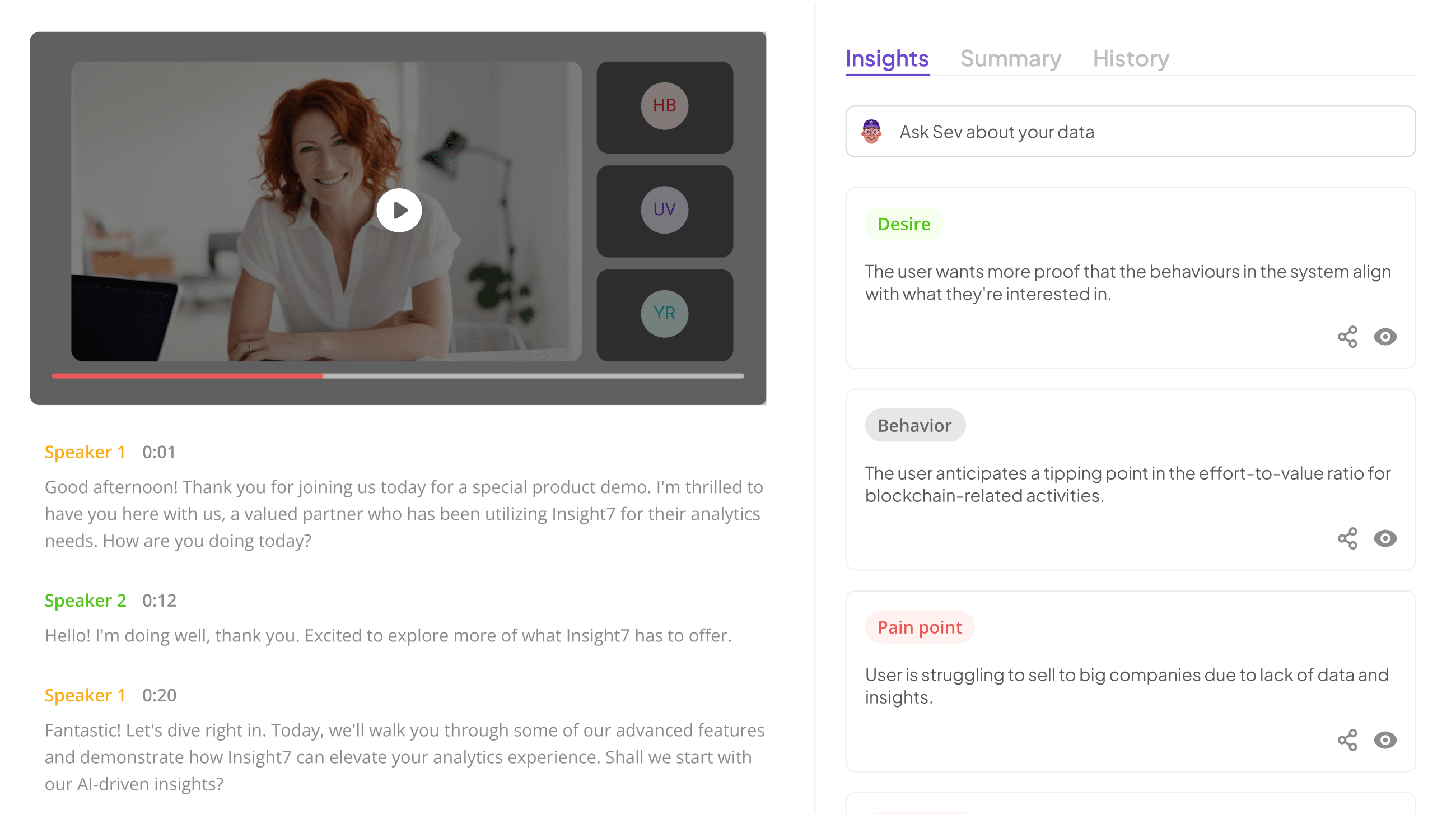
The Power of AI Tagging Enhancement in Managing Data
AI Tagging Enhancement plays a crucial role in managing large datasets effectively and efficiently. By automating the tagging process, projects can swiftly categorize vast amounts of information, making data retrieval easier and faster. With AI-driven algorithms, tagging becomes more precise, allowing researchers to extract relevant insights within seconds rather than hours. This ensures that data management is not only streamlined but also optimized for deeper analysis and understanding.
One of the significant advantages of AI Tagging Enhancement is its capacity to improve data accessibility. It offers an intuitive way for team members to locate specific information across various projects. By employing advanced tagging techniques, researchers can easily track trends, manage files, and generate reports that are vital for decision-making. Consequently, projects benefit from enhanced collaboration and knowledge-sharing, enabling teams to focus on critical tasks rather than getting bogged down by data management challenges.
Automating Metadata Creation
Creating metadata can often be a tedious task in large-scale research projects, but automation offers a significant solution. AI tagging enhances this process by analyzing large data sets and generating relevant metadata without human intervention. As researchers accumulate vast amounts of information, manually categorizing and tagging each piece can be impractical and time-consuming.
Automating metadata creation allows teams to focus on analysis and insights rather than administrative tasks. By employing smart algorithms, AI can quickly identify key themes, entities, and relationships within the data. This results in consistent and accurate tagging, ensuring that the research is well-organized and easily accessible. With automated metadata, researchers can streamline workflows and improve data discoverability, ultimately leading to more effective and efficient research outcomes.
Improving Data Accessibility with AI Tagging
AI Tagging Enhancement significantly improves data accessibility, particularly in large-scale research projects. By using intelligent algorithms, AI can automatically generate metadata for vast datasets, transforming chaotic information into structured, searchable content. This capability ensures that researchers can easily locate relevant data, enabling them to make informed decisions quickly.
Moreover, AI tagging enhances collaboration among team members by standardizing data interpretation. With uniform tags, individuals can share insights seamlessly, reducing miscommunication. This collective approach streamlines research processes, saving time and fostering innovation. In essence, AI Tagging Enhancement is not merely a technical tool; it is a bridge that connects vast amounts of information to the researchers who need it, ultimately paving the way for more efficient and effective studies.
Extract insights from interviews, calls, surveys and reviews for insights in minutes
Tools Transforming AI Tagging Enhancement in Research Projects
In today's research environment, AI Tagging Enhancement plays a crucial role in managing and analyzing vast amounts of data. Various tools are now leading the transformation of this field, enhancing the accuracy and efficiency of tagging processes. These advanced technologies enable researchers to quickly categorize and retrieve relevant information from large datasets, thereby streamlining workflows and driving insightful results.
Some of the prominent tools include AI-driven platforms that facilitate automated metadata creation and intuitive annotation processes. For instance, Tagtog simplifies the annotation of text and images, making it accessible for teams to collaborate effectively. Additionally, MonkeyLearn focuses on textual data analysis, providing precision that enhances the overall quality of research outcomes. With IBM Watson's sophisticated algorithms, researchers can leverage AI for deeper insights, discovering patterns that human analysis may overlook. By embracing these innovations, research projects can harness the true potential of AI Tagging Enhancement, promoting a more efficient data management approach.
insight7: Leading the Charge
AI Tagging Enhancement is transforming the landscape of large-scale research projects, enabling researchers to efficiently manage vast amounts of data. By automating metadata creation, AI taggers ensure that essential information is easily categorized and accessible. This shift allows research teams to focus more on analyzing data rather than spending excessive time on manual tagging.
As researchers embrace AI Tagging Enhancement, collaboration becomes more effective. Insights gathered from customer interactions and interviews can be seamlessly compiled, making it easier to translate findings into actionable strategies. Transparency in data handling is essential, ensuring all stakeholders can contribute and engage with the research outputs. Overall, AI Tagging Enhancement leads the charge towards a more data-driven and efficient research process, reducing delays and empowering teams to stay one step ahead in their projects.
Tagtog: Simplifying Annotations
Annotations are essential for organizing and classifying data, especially in large-scale research projects. By utilizing AI-driven solutions, the tedious process of tagging is transformed into a streamlined practice. This simplification allows researchers to focus on more critical aspects of their projects instead of being bogged down by manual annotation work.
AI Tagging Enhancement not only minimizes human error but also ensures that each piece of data is accurately categorized. Teams can access insights and trends efficiently, allowing for more informed decision-making. With simple and intuitive interfaces, researchers can quickly apply tags to relevant sections of data, enhancing collaboration and consistency. This innovative approach ensures that all stakeholders, regardless of technical expertise, can participate in the annotation process, fundamentally changing the way data is managed in research.
MonkeyLearn: Textual Data Precision
AI Tagging Enhancement plays a crucial role in improving the precision of textual data analysis. By leveraging advanced algorithms, large datasets can be categorized swiftly, ensuring that the data is not only relevant but also easily accessible. This is particularly beneficial for extensive research projects where vast amounts of information are generated. Accurate tagging not only streamlines data organization but also enhances the quality of insights derived from the information.
The integration of AI technology enables the identification of patterns and trends within textual data, transforming raw input into actionable knowledge. This not only assists researchers in drawing meaningful conclusions but also facilitates collaboration among team members by maintaining consistency in data interpretation. By adopting AI Tagging Enhancement, research projects can achieve higher efficiency and precision, ultimately leading to transformative outcomes in their respective fields.
IBM Watson: Sophistication in AI Tagging
AI Tagging Enhancement is revolutionizing the way researchers manage vast volumes of data. This advanced system employs sophisticated algorithms to analyze textual content. By doing so, it automatically generates relevant tags that enhance the organization and retrieval of information. Researchers can quickly identify critical themes, trends, and insights from their data, thereby significantly improving the efficiency of their studies.
The AI tagging process enables users to streamline data handling through automated metadata creation. This not only saves time but also reduces human error during tagging. Enhanced data accessibility is another significant advantage, allowing for more effective data collaboration among teams. As researchers navigate their projects, the sophistication of AI tagging provides a seamless experience, transforming raw data into valuable insights that drive further investigation and innovation.
Conclusion: The Future of AI Tagging Enhancement in Research
As we look to the future, AI tagging enhancement holds immense promise for research endeavors. With continual improvements in machine learning algorithms, researchers can expect increased accuracy and efficiency in tagging large datasets. The integration of AI technologies will facilitate easier retrieval of relevant information, allowing researchers to focus on analyzing data rather than organizing it.
Moreover, as AI tagging systems evolve, they will become more adaptable to specific fields and projects. This customization will support researchers in making data-driven decisions quickly. Ultimately, embracing these advancements will redefine research methodologies and unlock new insights that were previously inaccessible.