AI-Driven QA Fairness revolutionizes how agent scoring is approached in contemporary workplaces. Traditional quality assurance often falls prey to bias—whether unconscious or systemic—leading to skewed evaluations that can impact career progression. As organizations recognize the importance of fair and impartial assessments, AI technologies emerge as powerful tools to enhance accuracy and objectivity.
In this section, we will explore how AI-driven methodologies address these biases. By utilizing advanced algorithms and real-time analytics, companies can ensure evaluations reflect true performance. This shift not only builds trust among agents but also creates a level playing field, fostering a culture of transparency and integrity in agent scoring processes.
Analyze qualitative data. At Scale.
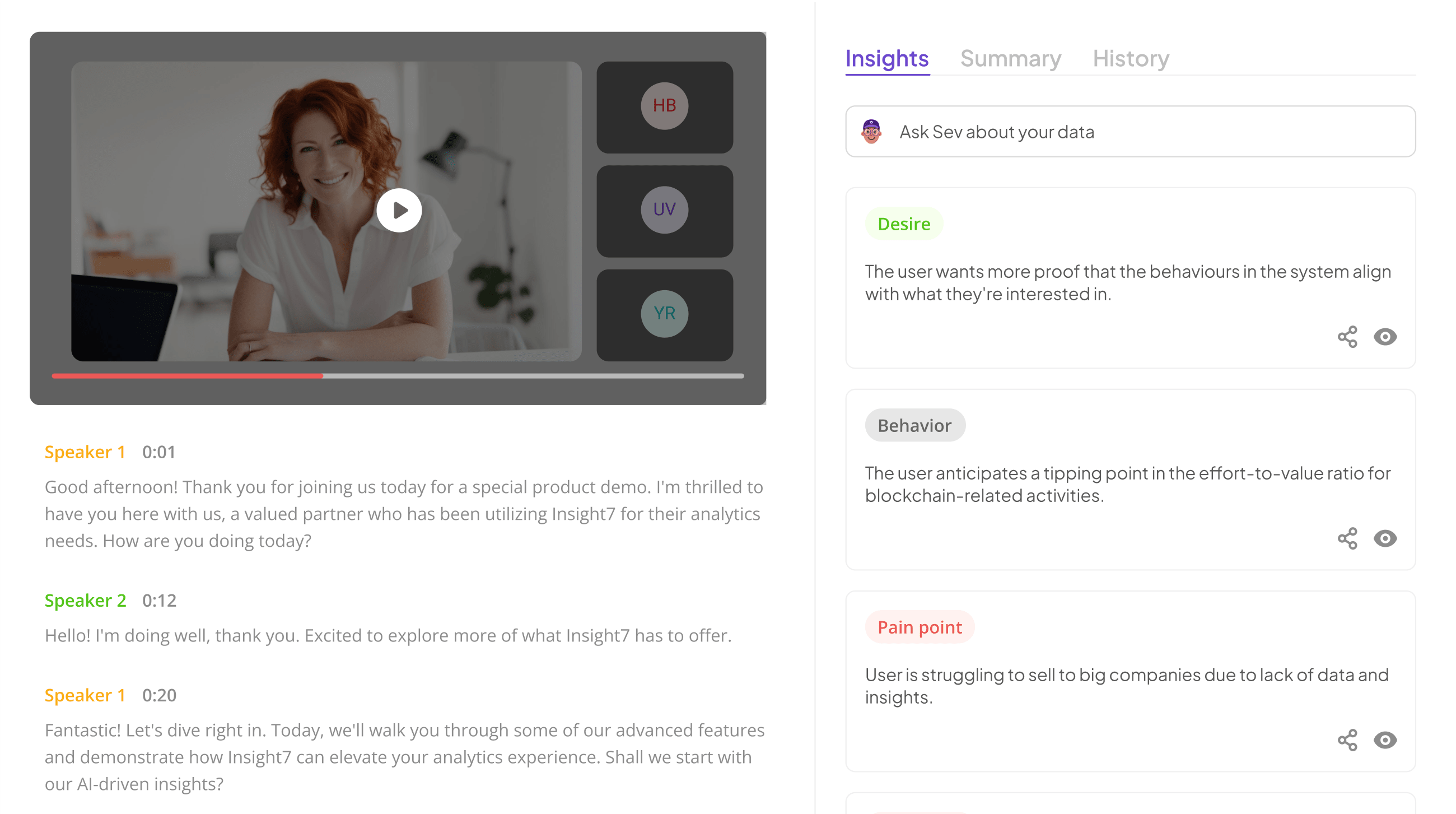
Understanding Bias in Traditional QA Scoring
Bias in traditional QA scoring is often rooted in subjective assessments influenced by personal opinions and experiences. This can lead to inconsistent evaluations of agent performance, ultimately impacting overall quality assurance efforts. Traditionally, evaluators may gravitate toward certain traits they value or may overlook areas for improvement based on preconceived notions, which skews the scoring process.
Moreover, existing QA practices frequently struggle with standardization, leading to varied interpretations of criteria among different reviewers. The result is a disjointed evaluation process that may unfairly advantage or disadvantage certain agents, creating further discrepancies in performance scores. Understanding these limitations highlights the need for AI-driven QA fairness. By harnessing advanced technologies, organizations can aim for more objective and equitable evaluation methods, substantially reducing the impact of human bias in scoring systems.
The Impact of Human Bias
Human bias is an inevitable factor in traditional QA scoring, influencing how agents are evaluated and ultimately affecting performance outcomes. Theories of subjective judgment can lead to inconsistent assessments when agents are scored by different evaluators, undermining the fairness of the evaluation process. Furthermore, unconscious biases, whether stemming from personal experiences or societal stereotypes, can significantly skew results, disadvantaging certain individuals based on characteristics unrelated to their work performance.
AI-driven QA fairness aims to mitigate these issues by providing objective evaluations based on data. By analyzing large data sets without the influence of human emotions or preconceptions, AI can ensure that every agent is scored consistently. This emphasizes measurable performance indicators over subjective opinions. Consequently, organizations can foster a more equitable work environment in which all agents receive ratings based on their actual performance, enhancing morale and productivity across the board.
Limitations of Current QA Practices
Current QA practices often rely heavily on subjective evaluations, leading to inconsistencies in agent scoring. Human evaluators bring their own biases, which can skew results and ultimately impact an agent’s performance review. These limitations highlight the need for more effective methods to ensure fairness and objectivity in quality assessments. The absence of standardized criteria can further complicate QA processes, resulting in uneven applications of performance metrics.
Implementing AI-driven QA fairness could mitigate these issues by providing more consistent evaluations. AI can analyze vast amounts of data without the personal biases that human agents might inadvertently incorporate. Additionally, AI systems can automate scoring based on pre-defined criteria, ensuring that evaluations remain uniform across the board. Transforming current practices to incorporate AI technology will not only enhance objectivity but also streamline the overall assessment process. In a world facing growing demands for fairness, it’s clear that leveraging AI is crucial for advancements in QA practices.
AI-Driven QA Fairness: Transforming Agent Scoring
AI-Driven QA Fairness represents a significant shift in how organizations evaluate agent performance. Traditional methods often introduce personal biases, leading to inconsistent scoring. However, with AI technologies, companies can ensure a more objective assessment of agent interactions. By analyzing data from numerous customer interactions, AI can identify patterns that highlight key performance indicators devoid of human error or subjective judgments.
This transformation is not simply about replacing human insight; it's about enriching the evaluation process with data-driven accuracy. Implementing AI tools facilitates real-time monitoring, allowing for continuous adjustment and alignment with business goals. By embracing AI-Driven QA Fairness, organizations can not only improve the evaluation of their agents but also foster a culture of transparency and accountability. This approach ultimately benefits both the agents and the customers, leading to more informed decision-making and enhanced service quality.
Extract insights from interviews, calls, surveys and reviews for insights in minutes
Leveraging Machine Learning for Objective Evaluation
Artificial intelligence plays a crucial role in enabling objective evaluation within quality assurance processes. By employing AI-driven methods, organizations can effectively reduce bias in assessing agent performance. Machine learning algorithms analyze large volumes of data, enabling them to identify patterns that human evaluators might overlook. This objectivity helps ensure that scoring criteria are applied consistently, improving fairness across evaluations.
To further enhance AI-driven QA fairness, there are several key actions to undertake. First, organizations should establish standardized criteria that reflect the goals for agent performance. Next, employing machine learning models trained on diverse datasets can help eliminate discrepancies stemming from individual evaluator biases. Finally, ongoing monitoring and adjustment of the algorithms ensure they evolve alongside changing industry standards and practices. Ultimately, a commitment to AI-driven evaluation fosters a more equitable environment for agents, contributing to overall organizational success.
Real-Time Monitoring and Continuous Improvement
Real-time monitoring plays a critical role in ensuring AI-Driven QA Fairness by continuously assessing agent interactions. This approach allows organizations to promptly identify patterns and anomalies in scoring, which can highlight underlying biases. By analyzing feedback and performance data as it becomes available, businesses can make immediate adjustments to their training and quality assurance processes.
Continuous improvement is essential for refining the evaluation framework. When organizations prioritize real-time insights, they can quickly adapt to shifts in customer needs and evolving product offerings. This proactive stance fosters a culture of accountability and transparency, ensuring that all agents are scored fairly based on uniformly applied standards. Ultimately, combining real-time monitoring with ongoing refinement can dramatically enhance the agent performance evaluation process, resulting in improved customer satisfaction and employee morale.
Implementing AI Solutions for QA Fairness
Implementing AI solutions for QA fairness requires a strategic approach to integrate technology effectively. First, organizations must prioritize data collection and preparation. Quality data is the foundation for AI-driven QA fairness, as it shapes how algorithms evaluate agent performance. This entails cleaning and organizing data to eliminate discrepancies that can distort scoring.
Next, training and calibrating AI models is essential. This process involves feeding the algorithm diverse data sets to ensure it learns to identify patterns without bias. Once the model is adequately trained, deployment and integration with existing systems can commence. This step ensures that the AI solution works seamlessly with current workflows, facilitating objective and fair evaluation in real-time.
By being deliberate in these steps, companies can harness AI-driven QA fairness and create a more equitable environment for evaluating agent performance.
Step 1: Data Collection and Preparation
The journey toward AI-driven QA fairness begins with robust data collection and preparation. This initial step is crucial, as it sets the foundation for accurate assessments in agent scoring. Begin by collating data from various sources, including call transcripts, customer feedback, and performance metrics. It’s essential to ensure that the data is representative of different customer demographics and interactions. A diverse dataset minimizes biases, leading to more equitable evaluations.
Once the data is gathered, the next phase is preparation. This involves cleaning and structuring the data for analysis. Remove any inconsistencies or outliers that may distort the outcomes. Additionally, anonymize sensitive information to ensure compliance with data protection regulations. Properly prepared data will empower AI algorithms to analyze agent performance more objectively, fostering a culture of fairness and transparency. By prioritizing these aspects, organizations can leverage AI-driven insights to enhance agent scoring effectively.
Step 2: AI Model Training and Calibration
In Step 2: AI Model Training and Calibration, the focus shifts to ensuring AI-driven QA fairness through precise model training. This stage is crucial for teaching the AI system to recognize and evaluate agent performance objectively. A key aspect involves processing training data that ideally represents the diverse range of agent interactions. By utilizing a balanced dataset, the model can learn to minimize biases that might skew the evaluation process.
Calibration follows training, where the AI is tuned to enhance its accuracy and reliability. This involves adjusting parameters based on testing outcomes, ensuring that the AI model can distinguish between effective and ineffective communication skills. Continuous testing and recalibration are necessary as new data emerges, which helps refine the model's capabilities. In this way, organizations can achieve a more equitable and transparent QA scoring system, significantly reducing the potential for bias.
Step 3: Deployment and Integration with Existing Systems
Deploying AI-driven solutions for QA fairness requires careful integration with existing systems to maximize efficiency. The first step involves mapping the current technological landscape and identifying critical interfaces among various systems. This ensures that AI tools can seamlessly interact with existing databases, communication platforms, and reporting tools. A clear understanding of workflows and team roles will facilitate smoother transitions, minimizing disruptions.
Next, stakeholder training becomes essential for optimizing the new technologies. By equipping teams with the skills to utilize artificial intelligence effectively, organizations can foster a culture of objective evaluation. Continual monitoring should also be employed to assess the AI's performance and alignment with established goals. This iterative process ensures that the AI implementation not only reduces bias but also enriches the overall scoring system, reinforcing the importance of AI-driven QA fairness in creating a more equitable evaluation environment.
Tools for Enhancing AI-Driven QA Fairness
To enhance AI-Driven QA Fairness, organizations can utilize several effective tools that streamline and improve the evaluation process. Implementing AI technologies such as IBM Watson, Salesforce Einstein, and Microsoft Azure AI can drastically reduce bias in agent scoring. These systems leverage advanced algorithms and machine learning techniques to analyze vast amounts of data, ensuring more objective assessments.
The first tool, IBM Watson, offers capabilities for natural language processing and data analytics. This allows for in-depth evaluations based on precise criteria without human biases affecting outcomes. Subsequently, Salesforce Einstein integrates customer relationship management (CRM) data with AI insights, creating a holistic view that contributes to fairer assessments. Lastly, Microsoft Azure AI empowers businesses by providing a scalable platform, enabling real-time adjustments and continuous learning, which further enhances the fairness of scoring algorithms. Adopting these tools paves the way for a more equitable QA process, fostering trust and accountability within organizations.
insight7: Leading the Way in QA Fairness
AI-Driven QA Fairness is revolutionizing how agent scoring is approached within organizations. Embracing this technology enables a more objective framework that minimizes bias in evaluations. Traditional methods often fall prey to human subjectivity, leading to inconsistent assessments and unfair rankings. With AI, organizations can analyze vast amounts of data accurately, ensuring fair treatment for all agents based on their performance metrics rather than subjective opinions.
Moreover, AI fosters an environment of continuous improvement. By integrating AI solutions, managers can monitor interactions in real time, providing timely feedback and support. This proactive approach not only boosts agent performance but also cultivates a sense of fairness in evaluations. As a result, fostering a balanced workplace positively impacts employee morale and drives superior service experiences for customers. Leading the way in QA fairness illustrates the transformative potential of AI, offering pathways to cultivate equity in agent scoring practices.
IBM Watson
IBM Watson serves as a pivotal tool in the quest for AI-Driven QA Fairness in agent scoring. By harnessing advanced machine learning capabilities, it analyzes call data to eliminate biases that often originate from human evaluations. The system recognizes and interprets agent interactions, ensuring scoring reflects genuine performance rather than subjective views. This atmospheric transparency helps create a level playing field for agents, promoting equal opportunities for improvement.
Moreover, the technology facilitates real-time scoring and comprehensive feedback generation. Each agent's performance can be assessed objectively, with insights tailored to individual needs. The seamless integration with existing systems allows organizations to maintain governance while enhancing quality assessments. Ultimately, implementing such AI-driven solutions not only boosts operational efficiency but also fosters a culture of fair assessment, ensuring that agent development is aligned with organizational goals. In this way, AI transforms quality assurance from a subjective process into one grounded in fairness and objectivity.
Salesforce Einstein
Salesforce Einstein is a powerful tool designed to enhance the capabilities of customer service through AI. By integrating advanced analytics and machine learning, it enables organizations to assess agent performance more objectively. This capability is essential in combating bias in traditional QA scoring methods, helping teams to make data-driven decisions that reflect true agent effectiveness.
Utilizing this technology, businesses can better track their agents' adherence to established frameworks without the constraints of human error. As AI continuously learns from interactions, it allows for real-time monitoring and insights into performance trends. The result is a more equitable scoring system that minimizes bias, fostering a culture of fairness and accountability. Ultimately, adopting such AI-driven approaches can significantly improve overall customer service quality and operational efficiency.
Microsoft Azure AI
Microsoft Azure AI incorporates advanced machine learning technologies to enhance the quality assurance (QA) process in agent scoring. By employing AI-driven methodologies, organizations can significantly reduce bias typically found in traditional scoring systems. Utilizing sophisticated algorithms, Microsoft Azure AI analyzes agent performance impartially and efficiently, thereby ensuring that assessments are based solely on data rather than subjective judgments.
One of the key features of this platform is its ability to provide real-time evaluations. With continuous data processing, it captures essential metrics on agent interactions, enabling organizations to gain valuable insights instantly. This allows for ongoing adjustments to training programs and evaluation criteria, fostering an environment conducive to fair scoring. By adopting AI-driven QA fairness, companies not only improve their assessment processes but also cultivate a more equitable workplace for their agents.
Conclusion: Achieving AI-Driven QA Fairness in Modern Enterprises
Achieving AI-Driven QA Fairness in modern enterprises involves implementing advanced technologies to diminish bias in agent scoring. By harnessing the power of artificial intelligence, organizations are able to ensure a more equitable evaluation of their agents. This shift not only promotes transparency but also encourages a culture of accountability and trust.
Furthermore, a dedicated effort to integrate AI technology allows companies to continually assess and refine their quality assurance processes. This ongoing commitment to fairness ultimately leads to improved customer experiences and stronger relationships. Embracing AI-Driven QA Fairness positions organizations for long-term success in a competitive marketplace.