How AI Call Monitoring Helps Forecast Call Volume Spikes
-
Bella Williams
- 10 min read
Call Volume Prediction plays a crucial role in AI call monitoring, significantly enhancing the efficiency of customer service operations. Picture a sudden influx of customer inquiries during a product launch or a promotional event. Predicting these call volume spikes allows organizations to prepare adequately, ensuring that agents are available to provide timely support and maintain customer satisfaction.
Harnessing advanced algorithms, AI systems analyze historical call data and identify patterns that often precede increased call activity. By effectively utilizing these insights, businesses can optimize staffing levels, reduce wait times, and enhance overall service quality. Thus, understanding and implementing effective call volume prediction strategies can transform customer interactions and drive operational success.
Analyze & Evaluate Calls. At Scale.
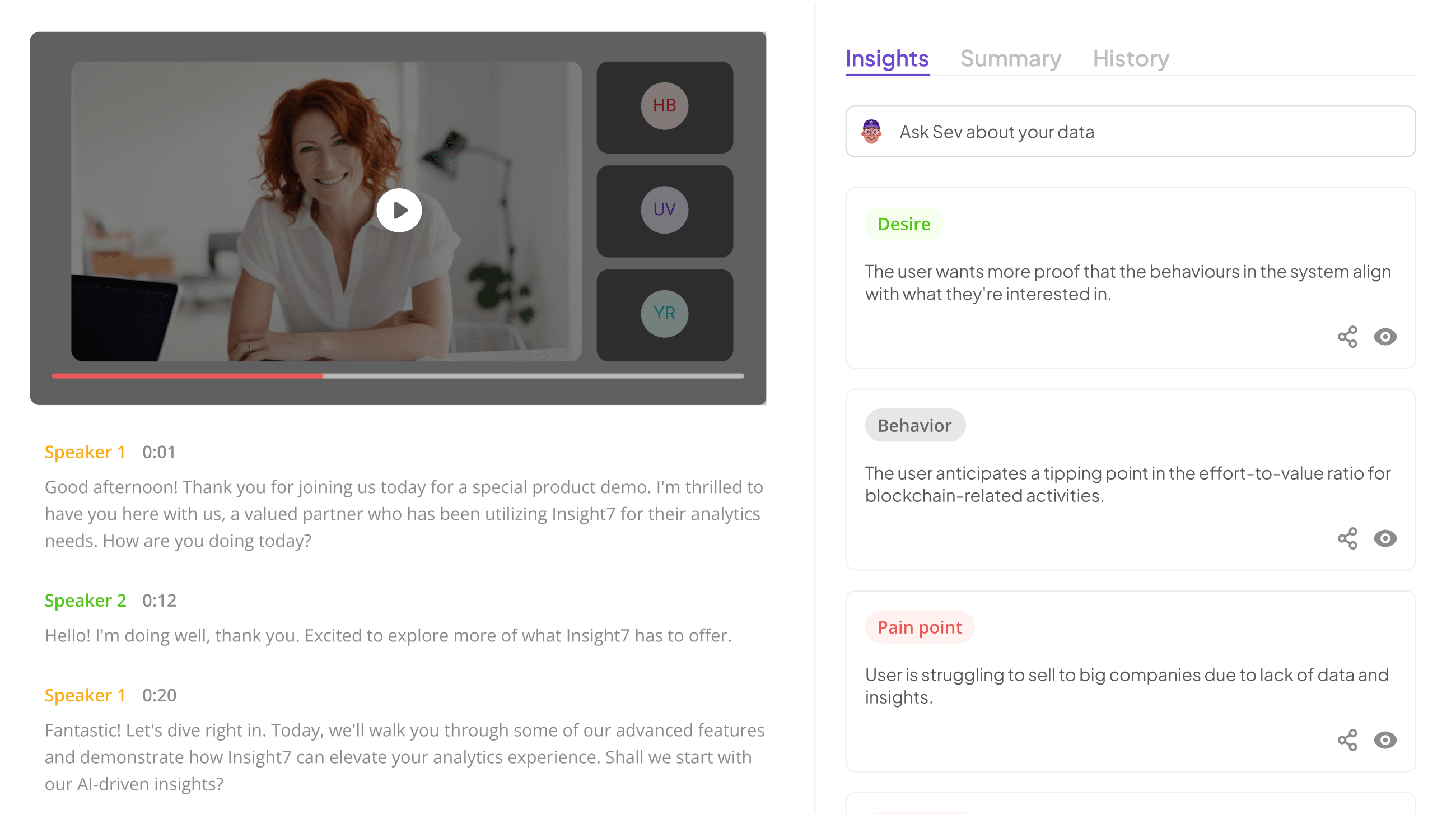
How AI Enhances Call Volume Prediction
AI enhances call volume prediction by employing advanced machine learning algorithms. These algorithms analyze historical call data to identify patterns, enabling organizations to anticipate future call volumes more accurately. By learning from past interactions, AI systems can identify trends and factors contributing to call spikes, such as seasonal changes, marketing campaigns, or specific events.
Another key aspect of AI's role is real-time data processing. By integrating live data streams, AI systems provide timely insights into call volumes, improving decision-making significantly. This instant analysis is crucial for managing sudden spikes efficiently, allowing call centers to adjust staffing and resources proactively. With AI, stakeholders can respond swiftly to fluctuations, ensuring that service levels remain high even during periods of increased demand. Thus, by effectively leveraging historical data and real-time analytics, AI fundamentally transforms how organizations approach call volume prediction.
The Role of Machine Learning
Machine learning plays a crucial role in enhancing call volume prediction, transforming how businesses manage their customer interactions. By analyzing historical call data, machine learning algorithms identify patterns and trends that inform future call volume estimates. This predictive modeling enables organizations to prepare for fluctuations in call traffic, ensuring optimal resource allocation and improved efficiency.
Furthermore, machine learning continuously learns from new data, refining its predictions as more information becomes available. This adaptive capability allows businesses to respond to real-time changes in customer behavior and external factors such as marketing campaigns or seasonal demand. By leveraging these insights, companies can proactively manage staffing needs, improve customer satisfaction rates, and reduce wait times during peak periods. Ultimately, the integration of machine learning in call volume prediction enhances the call center's ability to deliver quality service while minimizing disruptions in operations.
- Understanding machine learning algorithms for call data analysis
Machine learning algorithms play a pivotal role in analyzing call data, transforming raw audio into actionable insights for predicting call volume. By processing historical call information, these algorithms identify patterns that reveal when spikes in call volume are likely to occur. Recognizing trends in various data points helps organizations better prepare for fluctuations, facilitating improved resource allocation and customer service.
To grasp the algorithms involved, it's essential to understand three key aspects: first, data preprocessing, which involves cleaning and organizing call data for better analysis; second, model selection, where different algorithms are evaluated for their predictive accuracy; and finally, continuous learning, where the algorithm is refined over time as new data is analyzed. By effectively utilizing these components within machine learning, organizations can enhance their call volume prediction capabilities, ensuring they are ready for any surge in demand.
- How predictive models learn from historical call patterns
Predictive models harness historical call patterns to improve call volume prediction. By analyzing past call data, these models identify trends and patterns that reveal customer behavior and preferences. For instance, they can pinpoint peak call times and common customer inquiries. The analysis not only enables businesses to anticipate increased call volumes but also prepares agents for potential customer needs during busy periods.
Furthermore, the learning process involves various techniques. Historical data is fed into algorithms that recognize patterns, enabling the system to adapt over time. This dynamic adaptability allows predictive models to continuously refine their forecasts based on new information. Ultimately, understanding historical call patterns empowers organizations to allocate resources effectively, ensuring they meet customer demands while enhancing overall service delivery.
Real-time Data Processing for Call Volume Prediction
Real-time data processing plays a crucial role in Call Volume Prediction. By continuously collecting and analyzing call-related data, businesses can swiftly identify patterns and trends that signal potential spikes in call volume. This immediate analysis allows organizations to allocate resources effectively, ensuring that they are prepared for high demand periods.
Moreover, integrating real-time data sources enhances the accuracy of predictive analytics. Instead of relying solely on historical data, current indicatorsโsuch as seasonal fluctuations, marketing campaigns, or customer sentimentโcan be factored into the predictions. This dynamic approach leads to more timely and reliable forecasts, enabling businesses to maintain service quality and meet customer expectations even during peak times. By harnessing the power of real-time data, organizations can significantly improve their response to fluctuating call volumes and ultimately enhance customer satisfaction.
- The importance of real-time data integration
In the realm of call volume prediction, the integration of real-time data is crucial for effectively managing customer interactions. Organizations that prioritize real-time data integration can respond promptly to fluctuating call patterns, ensuring that customer service remains high-quality even during peak times. By collecting and analyzing live data, AI-driven systems can identify potential spikes in call volume, allowing teams to adjust staffing and resources accordingly.
Moreover, this immediate access to information enables companies to proactively address customer needs, rather than simply reacting to incoming inquiries. As customer expectations rise, organizations that harness real-time data integration will not only enhance their operational efficiency but also foster a more responsive service environment. Ultimately, companies that embrace this approach will be better equipped to navigate the complexities of customer demand, solidifying their competitive edge in the market.
- Predictive analytics in handling sudden call spikes
Predictive analytics plays a crucial role in managing sudden call spikes, enabling organizations to forecast call volume with precision. By analyzing historical call data, businesses can recognize patterns and potential surges in demand. This proactive approach ensures that resources are efficiently allocated, reducing the strain on customer service representatives during peak times.
Implementing predictive models involves several key steps that enhance call volume prediction. First, integrating real-time data is essential to keep up with changes in customer behavior and market trends. Second, machine learning algorithms can process this data, allowing for continuous improvement of predictions based on new information. Lastly, utilizing these insights empowers organizations to adjust staffing levels and training quickly, ensuring exceptional customer support even during unexpected call surges. This strategic use of predictive analytics reduces wait times, enhances customer satisfaction, and streamlines operational efficiency.
Extract insights from interviews, calls, surveys and reviews for insights in minutes
Tools for Effective Call Volume Prediction
Effective call volume prediction is essential for optimizing customer service operations. To achieve this, organizations must utilize advanced tools that analyze call patterns and forecast demand accurately. AI-driven solutions use historical data and machine learning algorithms to detect trends and anticipate spikes in call volume. By integrating these insights into daily operations, businesses can improve response times and resource allocation.
Several tools stand out in their ability to predict call volume effectively. Insight7 leads the way with its robust analytics for tracking and forecasting calls. Five9 offers features tailored to identify potential spikes, allowing teams to prepare in advance. Meanwhile, CallMiner delivers powerful insights on call trends, helping businesses strategize effectively. Verint ensures comprehensive analysis of all call data, while NICE inContact adapts to modern demands, making them all valuable in enhancing call volume prediction.
Top AI Tools for Call Monitoring
To enhance call volume prediction capabilities, several AI tools stand out in the market. These tools help organizations analyze large volumes of call data, facilitating better forecasts for call spikes. The first notable tool is Insight7, which utilizes AI algorithms to deliver accurate call volume forecasts based on historical trends. Then there's Five9, known for its robust features that predict call surges efficiently, enabling teams to prepare in advance.
CallMiner offers AI-driven insights that track call volume trends, ensuring businesses can respond proactively to spikes. Verint provides comprehensive solutions for analyzing call data, while NICE inContact stands out for its versatility in adapting to modern call center needs. These tools not only enhance operational efficiency but also empower organizations to optimize their resources based on anticipated call volumes, ensuring a smoother workflow and improved customer satisfaction.
- Insight7: Leading tool for accurate call volume prediction
Accurate call volume prediction is essential for managing customer interactions effectively, especially during peak times. Insight7 stands out as a leading tool designed specifically for this purpose. By utilizing advanced algorithms, it analyzes historical call data and employs predictive models to forecast potential spikes in call volume. This capability allows organizations to proactively allocate resources and enhance customer service experiences.
๐ฌ Questions about How AI Call Monitoring Helps Forecast Call Volume Spikes?
Our team typically responds within minutes
The real magic of Insight7 lies in its ability to process real-time data. As call patterns shift, this tool adjusts its predictions instantaneously, enabling companies to stay ahead of unexpected surges. With precise insights, businesses can refine their strategies, optimize staffing, and ensure that every customer interaction is handled effectively. The intuitive design and ease of use make it accessible for organizations of all sizes looking to improve their call volume prediction strategies.
- Five9: Features and benefits for predicting call spikes
The integration of AI technology in call monitoring systems offers remarkable features that enhance call volume prediction capabilities. By employing advanced algorithms and real-time data analysis, these systems can effectively forecast call spikes based on historical patterns. Notably, the system's ability to process large datasets in real-time allows organizations to anticipate high-volume periods, reducing wait times and improving customer satisfaction.
One significant benefit is the implementation of predictive analytics, which helps in identifying trends and potential spikes in call volume. As a result, businesses can allocate resources more efficiently, ensuring that the right number of agents are available during peak times. Furthermore, this proactive approach reduces operational costs by optimizing workforce management and improving overall service quality. In essence, the features and benefits derived from such systems are invaluable for maintaining a responsive and efficient communication strategy.
- CallMiner: AI-driven insights into call volume trends
In understanding call volume trends, the integration of AI-driven insights is essential. AI analyzes historical call data, identifying patterns that help forecast future demand with remarkable accuracy. This predictive capability ensures businesses can anticipate volume spikes, allowing them to allocate resources effectively.
One significant aspect of AI's contribution is its ability to process vast amounts of data in real-time. By monitoring ongoing calls, AI systems can detect sudden influxes in call volume and alert management promptly. Furthermore, these insights help organizations refine their customer service strategies, asking pertinent questions such as: What products are consumers inquiring about? Are there common concerns leading to increased calls?
By leveraging these insights, companies can not only prepare for expected call volume spikes but also enhance the overall customer experience, ensuring all inquiries are handled efficiently.
- Verint: Comprehensive solutions for call data analysis
Comprehensive solutions for call data analysis play a crucial role in understanding call volume prediction. These solutions are equipped with advanced algorithms that analyze historical data to identify patterns and trends. By leveraging insights from past call data, organizations can anticipate spikes in call volume more accurately. This predictive capability helps businesses prepare their resources efficiently, ensuring a smooth customer experience during peak times.
Real-time data integration is a vital component that enhances call volume prediction. With the ability to process current data instantly, organizations can respond proactively to sudden increases in call traffic. This capability not only minimizes wait times for customers but also alleviates the pressure on call center staff. As a result, effective call data analysis translates into improved service levels and enhanced operational efficiency. Adopting comprehensive call monitoring solutions ultimately supports businesses in navigating fluctuating demand with confidence.
- NICE inContact: Versatile tool for modern call center needs
In modern call centers, effectively managing call flow is crucial to maintaining customer satisfaction and operational efficiency. One standout option in the market is a versatile platform designed specifically to meet contemporary call center needs. This tool seamlessly integrates various data sources, allowing for comprehensive analysis of call patterns. By utilizing advanced algorithms, it helps forecast call volume spikes, ensuring that agents are prepared to handle fluctuations without compromising the quality of service.
Utilizing this advanced platform allows call centers to transition from traditional approaches to more consultative engagements with customers. By providing instant feedback through call monitoring, team leaders can identify coaching opportunities, enhance training methods, and adapt to market shifts. As a result, the call center can dynamically respond to customer needs while optimizing resources, thus ensuring smoother operations even during peak times. The emphasis on data-driven insights ultimately shapes a more responsive and effective customer service experience.
How Each Tool Contributes to Call Volume Prediction
In the context of call volume prediction, each tool plays a crucial role in enhancing accuracy and efficiency. First, the capabilities of AI-driven tools like Insight7 and CallMiner allow for in-depth analysis of historical call data. By leveraging machine learning algorithms, these tools can identify patterns and predict potential call spikes based on various factors, such as seasonal trends or marketing campaigns.
Next, tools like Five9 and Verint offer comprehensive solutions that integrate seamlessly with existing call center systems. This integration allows for real-time data processing, enabling businesses to respond swiftly to changes in call volume. Additionally, NICE inContact provides valuable flexibility, catering to the unique needs of modern call centers. Ultimately, these tools contribute substantially to refining call volume prediction, ensuring that organizations are better prepared for fluctuations in customer demand.
- Comparative analysis of accuracy and efficiency
The comparative analysis of accuracy and efficiency reveals crucial insights into the mechanisms behind call volume prediction. First, various AI tools operate with different accuracy levels, influenced by the algorithms they employ and the data quality they process. For instance, tools that utilize advanced machine learning techniques tend to deliver higher accuracy by analyzing historical patterns effectively. Improved accuracy allows organizations to anticipate call volume spikes better and prepare adequate resources.
Next, efficiency plays a distinct role. Tools that integrate seamlessly with existing systems can optimize data processing times, which is crucial during unpredictable call surges. An efficient tool not only enhances operational workflows but also significantly reduces downtime. By balancing accuracy and efficiency, businesses can harness AI call monitoring to gain a competitive edge while ensuring customer satisfaction during high-volume periods. Understanding these dynamics empowers organizations to select the appropriate solutions for effective call volume prediction.
- Integration capabilities with existing call center systems
Integrating AI call monitoring systems with existing call center platforms significantly enhances call volume prediction capabilities. This integration allows organizations to receive real-time insights and data analysis, ensuring they are prepared for unexpected surges in call volumes. By utilizing APIs and data synchronization, these systems can communicate seamlessly with customer relationship management software and workforce management tools, creating a unified approach to handling customer interactions.
Furthermore, integrating these solutions provides valuable analytics, enabling teams to identify patterns and key metrics. For instance, historical call data can be examined alongside current trends to predict upcoming peaks. Additionally, by capturing customer inquiries and feedback, companies can refine training programs for customer service representatives. Ultimately, robust integration capabilities not only streamline operations but also empower organizations to anticipate and respond to changing call volume dynamics effectively.
Accurate call volume prediction is vital for businesses aiming to navigate peak periods without compromising service quality. AI call monitoring incorporates advanced algorithms that analyze historical call data, identifying patterns and predicting potential spikes. This capability allows organizations to allocate resources effectively, ensuring that trained agents are available during high-demand times, thus maintaining customer satisfaction.
Moreover, real-time data processing enhances call volume prediction by continuously learning from incoming data. Such predictive analytics can flag sudden increases in call activity, giving management time to implement strategies that address customer needs efficiently. By integrating these advanced technological solutions, companies can adapt to customer behavior trends and mitigate the risk of overwhelmed call centers during peak periods. This proactive approach highlights the valuable interplay between AI and call management in optimizing service delivery.
Conclusion on Call Volume Prediction and AI
In conclusion, Call Volume Prediction through AI is revolutionizing how organizations prepare for fluctuations in call demand. By harnessing advanced algorithms, businesses can identify patterns in call data that might indicate an upcoming surge. Such predictive capabilities allow for better resource allocation and enhanced customer satisfaction, ensuring that agents are well-prepared to handle increased volumes.
As AI continues to evolve, its impact on Call Volume Prediction will only grow. With real-time data processing, companies can not only anticipate spikes but also respond effectively. This combination of foresight and adaptability makes AI an invaluable tool for any organization, enriching both operational efficiency and customer experiences.
๐ฌ Questions about How AI Call Monitoring Helps Forecast Call Volume Spikes?
Our team typically responds within minutes