How AI-Based Predictive Workload Distribution Enhances Efficiency
-
Bella Williams
- 10 min read
Predictive Workload Optimization is transforming how businesses enhance their operational efficiency. By forecasting demands based on historical data, organizations can streamline workload distribution, ensuring resources are allocated where they are most needed. This approach reduces downtime and maximizes productivity, contributing to an adaptive and responsive work environment.
Understanding the intricacies of Predictive Workload Optimization is essential for modern businesses. Utilizing advanced algorithms and machine learning, this optimization enables real-time adjustments to workload distribution, accommodating shifts in demand and enhancing overall efficiency. As technology evolves, the importance of effective predictive strategies becomes increasingly clear, driving organizations towards a more efficient future.
Analyze & Evaluate Calls. At Scale.
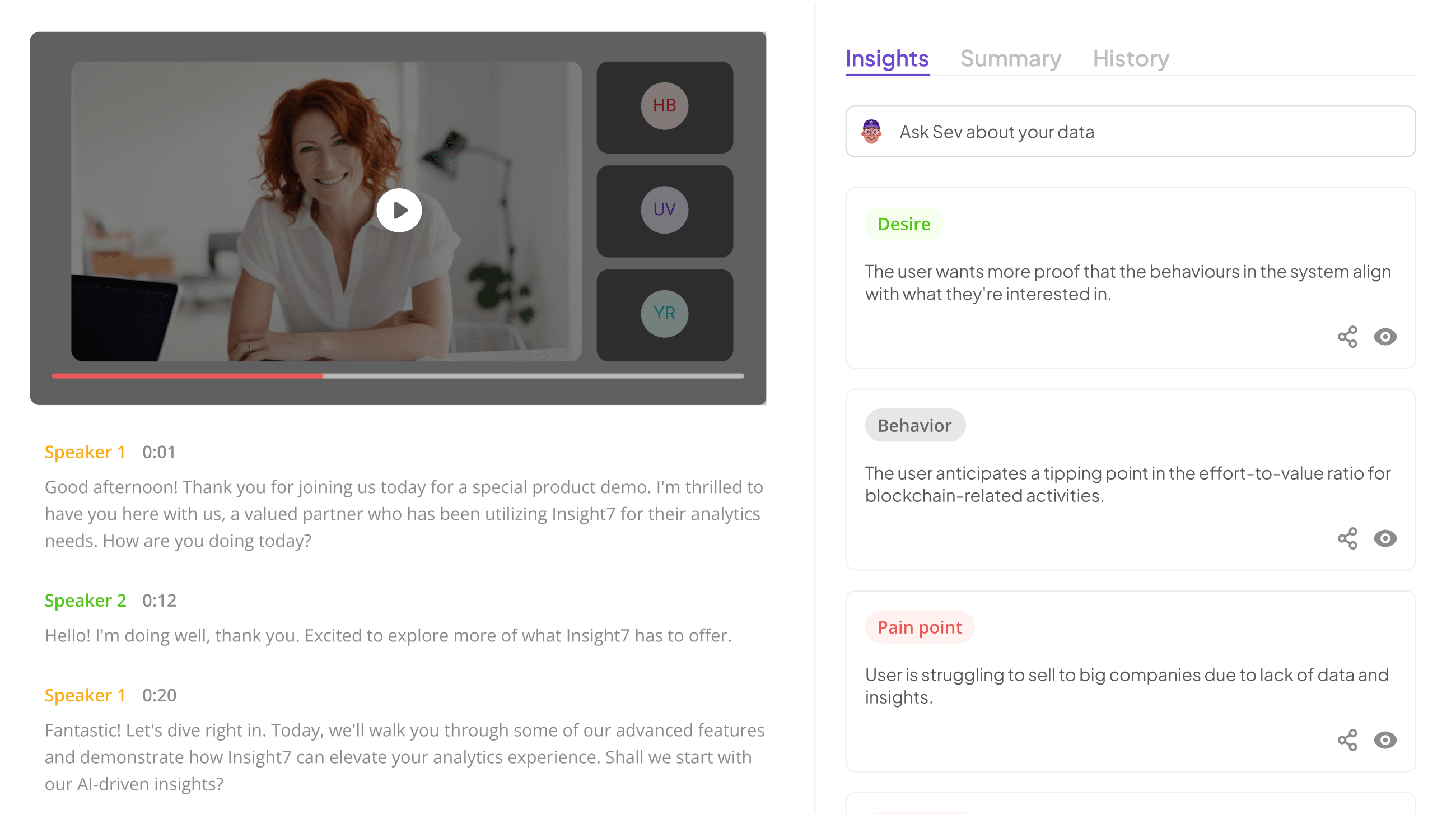
Understanding Predictive Workload Optimization
Predictive Workload Optimization is a technique that harnesses data analysis and machine learning to ensure tasks are allocated efficiently. This methodology focuses on predicting workload demands based on historical trends, allowing for better resource management. For organizations, implementing this optimization means reducing downtime and enhancing overall productivity.
At its core, Predictive Workload Optimization involves analyzing various data points to forecast future needs. This can include workload patterns, employee performance metrics, and customer demand signals. Understanding these elements allows teams to allocate resources more strategically, ensuring that the right personnel and tools are available at key moments. Ultimately, this process not only improves efficiency but also fosters a proactive organizational culture where challenges are anticipated rather than reacted to, promoting sustained growth and success.
The Role of AI in Workload Distribution
AI plays a crucial role in workload distribution by bringing efficiency and accuracy to the allocation process. Predictive workload optimization leverages advanced algorithms to analyze historical data and forecast future workloads. This allows organizations to proactively distribute tasks among team members based on their current capacities and performance metrics. By understanding individual strengths and weaknesses, AI ensures a more balanced workload, reducing stress and enhancing productivity.
Moreover, AI-driven systems can continuously monitor resource utilization and make real-time adjustments. For instance, if a particular team member is overloaded, the system can redistribute tasks to others who have the capacity to take on more work. This level of dynamic adjustment not only optimizes performance but also fosters a healthier work environment. In essence, the role of AI in workload distribution is pivotal to enhancing overall organizational efficiency and supporting employees in achieving their best.
Benefits of Predictive Workload Optimization
Predictive Workload Optimization serves as a crucial component for enhancing operational efficiency. By intuitively analyzing workloads, organizations can accurately forecast resource demands, improving responsiveness to changing requirements. This optimization reduces idle time across processes, as employees are allocated tasks that align with their specific skills and availability.
One major benefit includes increased productivity, as resources are utilized more effectively. Additionally, organizations experience reduced operational costs, since optimal workload distribution prevents overstaffing or underutilization of resources. Employees, in turn, enjoy an improved work-life balance, leading to higher job satisfaction and decreased turnover. Finally, businesses gain the ability to make data-driven decisions, enhancing strategic planning and business agility. Overall, Predictive Workload Optimization transforms workplace efficiency, paving the way for informed, sustainable growth.
The Process: How Predictive Workload Optimization Works
Predictive Workload Optimization begins with the collection and analysis of historical data. This stage involves gathering relevant metrics, such as previous workload patterns and performance outcomes. By understanding how tasks have been distributed and completed in the past, organizations can identify trends and insights that guide future planning.
Next, predictive modeling plays a critical role in achieving efficient distribution. Advanced algorithms analyze the collected data to forecast tasks' demands based on various factors like time of day and resource availability. These models allow businesses to prepare and allocate workloads proactively, ensuring that resources are utilized optimally.
Finally, real-time adjustments ensure that the system adapts to any unforeseen changes. By continuously monitoring workload distribution and performance metrics, predictive systems can adjust allocations dynamically. This responsiveness guarantees that efficiency is maintained throughout varying operational environments, ultimately driving better results and resource utilization.
Extract insights from interviews, calls, surveys and reviews for insights in minutes
Step 1: Data Collection and Analysis
Effective predictive workload optimization begins with thorough data collection and analysis. This step is critical as it lays the groundwork for accurate insights and informed decision-making. First, gather diverse data sources that reflect various operational aspects, including task durations, team performance metrics, and customer interactions. Itโs essential to ensure the quality and accuracy of this data, as reliable insights stem from trustworthy information.
Next, analyze the collected data for patterns and trends. Look for recurring issues, peak workload times, and resource allocation inefficiencies. Utilizing analytical tools can facilitate this process, allowing for the identification of key themes and correlations. In doing so, organizations can better understand their existing dynamics and leverage this knowledge to implement predictive workload distribution effectively. By harnessing data-driven insights, companies can streamline operations and enhance overall efficiency.
Step 2: Predictive Modeling for Efficient Distribution
In Step 2, the focus shifts to predictive modeling, a crucial aspect of achieving efficient workload distribution. By harnessing data analytics and pattern recognition, predictive modeling becomes a powerful tool in optimizing workloads. It identifies trends and prepares for future demands, ensuring that resources are allocated effectively. This proactive approach reduces downtime and enhances overall operational efficiency.
To implement predictive modeling effectively, consider the following key aspects:
- Data Integration: Combine data from various sources to get a comprehensive view of workloads. This enhances the accuracy of predictions.
- Model Selection: Choose the right algorithms that align with your specific business needs, whether regression models or machine learning techniques.
- Validation of Predictions: Continuously assess the output against actual results to refine models and improve forecasting accuracy.
- Scenario Planning: Simulate different scenarios to understand potential outcomes and how best to respond based on predictive efforts.
By meticulously addressing these elements, organizations can execute predictive workload optimization, ensuring a streamlined distribution process that adapts to changing demands.
Step 3: Real-time Adjustment and Implementation
Real-time adjustment and implementation are pivotal in the realm of predictive workload optimization. By continuously monitoring data, organizations can adapt their workload distribution dynamically, ensuring efficiency at all times. This adaptability allows businesses to respond promptly to evolving demands and changing operational conditions, enhancing overall productivity.
To effectively implement real-time adjustments, organizations should focus on three primary aspects:
Continuous Monitoring: Leveraging AI technologies enables ongoing scrutiny of workload patterns, allowing rapid identification of potential bottlenecks or inefficiencies.
Data-Driven Decision Making: Real-time insights derived from analytics guide managers in making informed decisions about resource allocation, optimizing team performance on the fly.
Feedback Loops: Establishing mechanisms for feedback ensures that adjustments can be refined over time, creating a culture of continuous improvement within the workforce.
By integrating these practices, organizations can maximize their efficiency through predictive workload optimization.
Tools for Effective Predictive Workload Optimization
Effective Predictive Workload Optimization depends significantly on utilizing the right tools to streamline processes and enhance efficiency. Tools like insight7, IBM Watson Studio, Google AI Platform, and Microsoft Azure Machine Learning empower organizations to analyze data, model scenarios, and implement real-time adjustments effectively. Each of these platforms offers unique features that contribute to predictive capabilities, enabling teams to foresee workload demands accurately.
Insight7 stands out as a user-friendly platform designed to democratize data analysis, making it accessible to all employees. IBM Watson Studio excels in collaborative data science efforts, allowing teams to build predictive models together. Google AI Platform integrates seamlessly with existing data workflows, providing robust analytics capabilities. Lastly, Microsoft Azure Machine Learning offers extensive resources for developing sophisticated machine learning models, ensuring that all aspects of workload optimization can be fine-tuned for maximum productivity. Understanding these tools is essential for organizations seeking to enhance their efficiency through predictive workload strategies.
insight7
Predictive Workload Optimization harnesses the power of artificial intelligence to streamline how tasks are allocated across teams. The process begins by gathering extensive data on past workloads and employee performance. This information is analyzed to identify patterns that inform future task assignments. AI algorithms continuously assess this data, ensuring optimal job distribution based on real-time conditions.
Moreover, as organizations encounter varying demands, Predictive Workload Optimization adjusts proactively to maintain efficiency. For instance, if a surge in requests is detected, the system dynamically reallocates resources to meet urgent needs. This flexible approach not only enhances productivity but also fosters a more engaged workforce. Employees often experience a reduction in overwhelm as their workloads reflect their individual capacities. Overall, embracing Predictive Workload Optimization leads to greater operational efficiency and a more responsive organizational structure.
IBM Watson Studio
The platform offers an intuitive interface that simplifies the data analysis process for users, regardless of their technical expertise. It empowers teams to generate insights efficiently without needing extensive training. By democratizing access to information, it allows various business functions to engage in predictive workload optimization. Users can quickly convert calls or datasets into actionable reports and assess customer experiences through tools designed for seamless interaction with data.
Within its core functionalities, users can store files and analyze interactions to extract invaluable insights. The platform highlights pain points and customer sentiments while visually representing data, making it easier to identify critical trends. Furthermore, the ability to aggregate multiple datasets enhances the understanding of complex projects, facilitating more informed decisions. This method not only streamlines processes but significantly boosts overall organizational efficiency, demonstrating the true potential of AI-driven predictive workload optimization.
Google AI Platform
The Google AI Platform offers essential capabilities for enhancing predictive workload optimization. By making advanced machine learning tools accessible, users can effectively streamline data analysis and modeling. This platform allows businesses to efficiently visualize and analyze large data sets without requiring extensive machine learning expertise. As users engage with intuitive workflows, they can gain valuable insights that inform better decision-making processes.
Additionally, the Google AI Platform enables real-time adjustments to workload distribution, allowing organizations to respond swiftly to changing demands. By utilizing automated predictions, companies can allocate resources more effectively, resulting in decreased downtime and increased productivity. This integration of AI-powered insights improves operational efficiency, transforming how businesses approach tasks and media management. Ultimately, the platform plays a crucial role in fostering agility and adaptability in an increasingly complex work environment.
Microsoft Azure Machine Learning
Microsoft Azure Machine Learning acts as a powerful toolset for implementing Predictive Workload Optimization. This platform enables organizations to harness the vast potential of machine learning through its comprehensive environment that supports building, training, and deploying models effectively. By utilizing advanced algorithms, it automates the distribution of workloads, ensuring optimal resource allocation aligned with predicted demands.
The ability to analyze and visualize vast datasets enhances decision-making processes. Azure provides features that allow users to create predictive models that can adapt to new data points in real-time. This adaptability significantly reduces latency, resulting in timely responses to changing conditions. As businesses strive for efficiency, embracing Predictive Workload Optimization through such platforms can lead to sustained performance and competitive advantage. Ultimately, utilizing machine learning frameworks to refine workload management principles fosters innovation and future readiness.
Conclusion: The Future of Predictive Workload Optimization
As organizations continue to embrace digital transformation, the future of predictive workload optimization appears promising. This technology has the potential to revolutionize how businesses manage operations, enhancing efficiency and resource allocation. With advancements in artificial intelligence, predictive workload optimization will enable companies to analyze vast amounts of data, predicting peaks in workload and adjusting accordingly.
Furthermore, as AI algorithms evolve, their accuracy in forecasting demand will improve, leading to even more effective workload distribution. This capability not only streamlines processes but also reduces costs and enhances employee satisfaction. Ultimately, predictive workload optimization will be a key component in driving operational excellence and sustainable growth in the years to come.